Breakthrough models AlphaProof and AlphaGeometry 2 solve advanced reasoning problems in mathematicsRead More
Unleash the Dragonborn: ‘Elder Scrolls V: Skyrim Special Edition’ Joins GeForce NOW
“Hey, you. You’re finally awake.”
It’s the summer of Elder Scrolls — whether a seasoned Dragonborn or a new adventurer, dive into the legendary world of Tamriel this GFN Thursday as The Elder Scrolls V: Skyrim Special Edition joins the cloud.
Epic adventures await, along with nine new games joining the GeForce NOW library this week.
Plus make sure to catch the GeForce NOW Summer Sale for 50% off new Ultimate and Priority memberships.
Unleash the Dragonborn
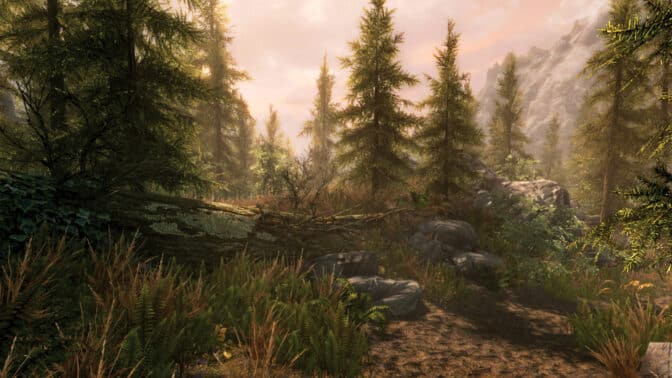
Experience the legendary adventures, breathtaking landscapes and immersive storytelling of the iconic role-playing game The Elder Scrolls V: Skyrim Special Edition from Bethesda Game Studios — now accessible on any device from the cloud. Become the Dragonborn and defeat Alduin the World-Eater, a dragon prophesied to destroy the world.
Explore a vast landscape, complete quests and improve skills to develop characters in the open world of Skyrim. The Special Edition includes add-ons with all-new features, including remastered art and effects. It also brings the adventure of Bethesda Game Studios creations, including new quests, environments, characters, dialogue, armor and weapons.
Get ready to embark on unforgettable quests, battle fearsome foes and uncover the rich lore of the Elder Scrolls universe, all with the power and convenience of GeForce NOW. “Fus Ro Dah” with an Ultimate membership to stream at up to 4K resolution and 120 frames per second with up to eight-hour gaming sessions for the ultimate immersive experience throughout the realms of Tamriel.
All Hands on Deck
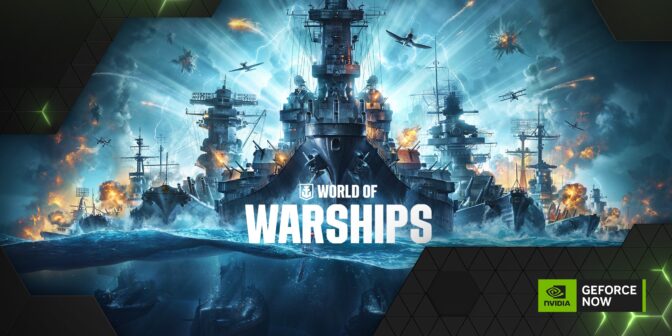
Wargaming is bringing back an in-game event exclusively for GeForce NOW members this week.
Through Tuesday, July 30, members who complete the quest while streaming World of Warships can earn up to five GeForce NOW one-day Priority codes — one for each day of the challenge. Aspiring admirals can learn more on the World of Warships blog and social channels.
Shiny and New
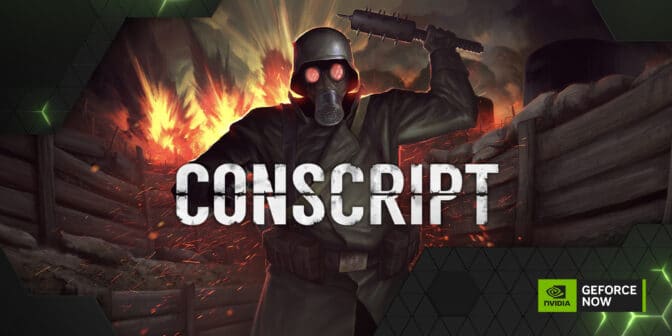
Take on classic survival horror in CONSCRIPT from Jordan Mochi and Team17. Inspired by legendary games in the genre, the game is set in 1916 during the Great War. CONSCRIPT blends all the punishing mechanics of older horror games into a cohesive, tense and unique experience. Play as a French soldier searching for his missing-in-action brother during the Battle of Verdun. Search through twisted trenches, navigate overrun forts and cross no-man’s-land to find him.
Here’s the full list of new games this week:
- Cataclismo (New release on Steam, July 22
- CONSCRIPT (New release on Steam, July 23)
- F1 Manager 2024 (New release on Steam, July 23)
- EARTH DEFENSE FORCE 6 (New release on Steam, July 25)
- The Elder Scrolls V: Skyrim (Steam)
- The Elder Scrolls V: Skyrim Special Edition (Steam, Epic Games Store and Xbox, available on PC Game Pass)
- Gang Beasts (Steam and Xbox, available on PC Game Pass)
- Kingdoms and Castles (Steam)
- The Settlers: New Allies (Steam)
What are you planning to play this weekend? Let us know on X or in the comments below.
ʏᴏᴜʀ ᴄʟᴏᴜᴅ ɢᴀᴍɪɴɢ ꜱᴋɪʟʟ ɪɴᴄʀᴇᴀꜱᴇᴅ
—
NVIDIA GeForce NOW (@NVIDIAGFN) July 24, 2024
LazyLLM: Dynamic Token Pruning for Efficient Long Context LLM Inference
This paper was accepted at the Efficient Systems for Foundation Models Workshop at ICML 2024
The inference of transformer-based large language models consists of two sequential stages: 1) a prefilling stage to compute the KV cache of prompts and generate the first token, and 2) a decoding stage to generate subsequent tokens. For long prompts, the KV cache must be computed for all tokens during the prefilling stage, which can significantly increase the time needed to generate the first token. Consequently, the prefilling stage may become a bottleneck in the generation process. An open question…Apple Machine Learning Research
Pre-Trained Foundation Model Representations to Uncover Breathing Patterns in Speech
The process of human speech production involves coordinated respiratory action to elicit acoustic speech signals. Typically, speech is produced when air is forced from the lungs and is modulated by the vocal tract, where such actions are interspersed by moments of breathing in air (inhalation) to refill the lungs again. Respiratory rate (𝑅𝑅) is a vital metric that is used to assess the overall health, fitness, and general well-being of an individual. Existing approaches to measure 𝑅𝑅 (number of breaths one takes in a minute) are performed using specialized equipment or training. Studies…Apple Machine Learning Research
Mistral Large 2 is now available in Amazon Bedrock
Mistral AI’s Mistral Large 2 (24.07) foundation model (FM) is now generally available in Amazon Bedrock. Mistral Large 2 is the newest version of Mistral Large, and according to Mistral AI offers significant improvements across multilingual capabilities, math, reasoning, coding, and much more.
In this post, we discuss the benefits and capabilities of this new model with some examples.
Overview of Mistral Large 2
Mistral Large 2 is an advanced large language model (LLM) with state-of-the-art reasoning, knowledge, and coding capabilities according to Mistral AI. It is multi-lingual by design, supporting dozens of languages, including English, French, German, Spanish, Italian, Chinese, Japanese, Korean, Portuguese, Dutch, Polish, Arabic, and Hindi. Per Mistral AI, a significant effort was also devoted to enhancing the model’s reasoning capabilities. One of the key focuses during training was to minimize the model’s tendency to hallucinate, or generate plausible-sounding but factually incorrect or irrelevant information. This was achieved by fine-tuning the model to be more cautious and discerning in its responses, making sure it provides reliable and accurate outputs. Additionally, the new Mistral Large 2 is trained to acknowledge when it can’t find solutions or doesn’t have sufficient information to provide a confident answer.
According to Mistral AI, the model is also proficient in coding, trained on over 80 programming languages such as Python, Java, C, C++, JavaScript, Bash, Swift, and Fortran. With its best-in-class agentic capabilities, it can natively call functions and output JSON, enabling seamless interaction with external systems, APIs, and tools. Additionally, Mistral Large 2 (24.07) boasts advanced reasoning and mathematical capabilities, making it a powerful asset for tackling complex logical and computational challenges.
Mistral Large 2 also offers an increased context window of 128,000 tokens. At the time of writing, the model (mistral.mistral-large-2407-v1:0) is available in the us-west-2
AWS Region.
Get started with Mistral Large 2 on Amazon Bedrock
If you’re new to using Mistral AI models, you can request model access on the Amazon Bedrock console. For more details, see Manage access to Amazon Bedrock foundation models.
To test Mistral Large 2 on the Amazon Bedrock console, choose Text or Chat under Playgrounds in the navigation pane. Then choose Select model and choose Mistral as the category and Mistral Large 24.07 as the model.
By choosing View API request, you can also access the model using code examples in the AWS Command Line Interface (AWS CLI) and AWS SDKs. You can use model IDs such as mistral.mistral-large-2407-v1:0
, as shown in the following code:
In the following sections, we dive into the capabilities of Mistral Large 2.
Increased context window
Mistral Large 2 supports a context window of 128,000 tokens, compared to Mistral Large (24.02), which had a 32,000-token context window. This larger context window is important for developers because it allows the model to process and understand longer pieces of text, such as entire documents or code files, without losing context or coherence. This can be particularly useful for tasks like code generation, documentation analysis, or any application that requires understanding and processing large amounts of text data.
Generating JSON and tool use
Mistral Large 2 now offers a native JSON output mode. This feature allows developers to receive the model’s responses in a structured, easy-to-read format that can be readily integrated into various applications and systems. With JSON being a widely adopted data exchange standard, this capability simplifies the process of working with the model’s outputs, making it more accessible and practical for developers across different domains and use cases. To learn more about how to generate JSON with the Converse API, refer to Generating JSON with the Amazon Bedrock Converse API.
To generate JSON with the Converse API, you need to define a toolSpec
. In the following code, we present an example for a travel agent company that will take passenger information and requests and convert them to JSON:
We get the following response:
Mistral Large 2 was able to correctly take our user query and convert the appropriate information to JSON.
Mistral Large 2 also supports the Converse API and tool use. You can use the Amazon Bedrock API to give a model access to tools that can help it generate responses for messages that you send to the model. For example, you might have a chat application that lets users find the most popular song played on a radio station. To answer a request for the most popular song, a model needs a tool that can query and return the song information. The following code shows an example for getting the correct train schedule:
We get the following response:
Mistral Large 2 was able to correctly identify the shinkansen tool and demonstrate its use.
Multilingual support
Mistral Large 2 now supports a large number of character-based languages such as Chinese, Japanese, Korean, Arabic, and Hindi. This expanded language support allows developers to build applications and services that can cater to users from diverse linguistic backgrounds. With multilingual capabilities, developers can create localized UIs, provide language-specific content and resources, and deliver a seamless experience for users regardless of their native language.
In the following example, we translate customer emails generated by the author into different languages such as Hindi and Japanese:
We get the following response:
Coding tasks
Mistral Large 2 has been trained on over 80 coding languages, including popular ones like Python, Java, C, C++, JavaScript, and Bash, as well as more specialized languages such as Swift and Fortran. This comprehensive language support empowers developers to tackle a wide range of coding tasks and projects across various domains and platforms. Whether you’re working on web development, mobile applications, scientific computing, or system programming, Mistral Large 2 can assist you with code generation, debugging, refactoring, and other coding-related tasks. For example, the following code requests the model to generate a Python function:
We get the following response:
Conclusion
Mistral AI’s Mistral Large 2 FM is now available on Amazon Bedrock in the US West (Oregon) Region. To get started with Mistral Large 2 in Amazon Bedrock, visit the Amazon Bedrock console.
Interested in diving deeper? Check out the Mistral-on-AWS repo. For more information about Mistral AI on Amazon Bedrock, refer to Mistral AI models now available on Amazon Bedrock.
About the Authors
Niithiyn Vijeaswaran is a Solutions Architect at AWS. His area of focus is generative AI and AWS AI Accelerators. He holds a Bachelor’s degree in Computer Science and Bioinformatics. Niithiyn works closely with the Generative AI GTM team to enable AWS customers on multiple fronts and accelerate their adoption of generative AI. He’s an avid fan of the Dallas Mavericks and enjoys collecting sneakers.
Armando Diaz is a Solutions Architect at AWS. He focuses on generative AI, AI/ML, and Data Analytics. At AWS, Armando helps customers integrating cutting-edge generative AI capabilities into their systems, fostering innovation and competitive advantage. When he’s not at work, he enjoys spending time with his wife and family, hiking, and traveling the world.
Preston Tuggle is a Sr. Specialist Solutions Architect working on generative AI.
LLM experimentation at scale using Amazon SageMaker Pipelines and MLflow
Large language models (LLMs) have achieved remarkable success in various natural language processing (NLP) tasks, but they may not always generalize well to specific domains or tasks. You may need to customize an LLM to adapt to your unique use case, improving its performance on your specific dataset or task. You can customize the model using prompt engineering, Retrieval Augmented Generation (RAG), or fine-tuning. Evaluation of a customized LLM against the base LLM (or other models) is necessary to make sure the customization process has improved the model’s performance on your specific task or dataset.
In this post, we dive into LLM customization using fine-tuning, exploring the key considerations for successful experimentation and how Amazon SageMaker with MLflow can simplify the process using Amazon SageMaker Pipelines.
LLM selection and fine-tuning journeys
When working with LLMs, customers often have different requirements. Some may be interested in evaluating and selecting the most suitable pre-trained foundation model (FM) for their use case, while others might need to fine-tune an existing model to adapt it to a specific task or domain. Let’s explore two customer journeys:
- Selecting and evaluating foundation models – You can evaluate the performance of different pre-trained FMs on relevant datasets and metrics specific to your use case. You can then select the best model based on the evaluation results. You can do this using services such as Amazon SageMaker JumpStart and Amazon SageMaker Clarify. It can also be done at scale, as explained in Operationalize LLM Evaluation at Scale using Amazon SageMaker Clarify and MLOps services. The following diagram illustrates an example architecture.
- Fine-tuning an LLM for a specific task or domain adaptation – In this user journey, you need to customize an LLM for a specific task or domain data. This requires fine-tuning the model. The fine-tuning process may involve one or more experiment, each requiring multiple iterations with different combinations of datasets, hyperparameters, prompts, and fine-tuning techniques, such as full or Parameter-Efficient Fine-Tuning (PEFT). Each iteration can be considered a run within an experiment.
Fine-tuning an LLM can be a complex workflow for data scientists and machine learning (ML) engineers to operationalize. To simplify this process, you can use Amazon SageMaker with MLflow and SageMaker Pipelines for fine-tuning and evaluation at scale. In this post, we describe the step-by-step solution and provide the source code in the accompanying GitHub repository.
Solution overview
Running hundreds of experiments, comparing the results, and keeping a track of the ML lifecycle can become very complex. This is where MLflow can help streamline the ML lifecycle, from data preparation to model deployment. By integrating MLflow into your LLM workflow, you can efficiently manage experiment tracking, model versioning, and deployment, providing reproducibility. With MLflow, you can track and compare the performance of multiple LLM experiments, identify the best-performing models, and deploy them to production environments with confidence.
You can create workflows with SageMaker Pipelines that enable you to prepare data, fine-tune models, and evaluate model performance with simple Python code for each step.
Now you can use SageMaker managed MLflow to run LLM fine-tuning and evaluation experiments at scale. Specifically:
- MLflow can manage tracking of fine-tuning experiments, comparing evaluation results of different runs, model versioning, deployment, and configuration (such as data and hyperparameters)
- SageMaker Pipelines can orchestrate multiple experiments based on the experiment configuration
The following figure shows the overview of the solution.
Prerequisites
Before you begin, make sure you have the following prerequisites in place:
- Hugging Face login token – You need a Hugging Face login token to access the models and datasets used in this post. For instructions to generate a token, see User access tokens.
- SageMaker access with required IAM permissions – You need to have access to SageMaker with the necessary AWS Identity and Access Management (IAM) permissions to create and manage resources. Make sure you have the required permissions to create notebooks, deploy models, and perform other tasks outlined in this post. To get started, see Quick setup to Amazon SageMaker. Please follow this post to make sure you have proper IAM role confugured for MLflow.
Set up an MLflow tracking server
MLflow is directly integrated in Amazon SageMaker Studio. To create an MLflow tracking server to track experiments and runs, complete the following steps:
- On the SageMaker Studio console, choose MLflow under Applications in the navigation pane.
- For Name, enter an appropriate server name.
- For Artifact storage location (S3 URI), enter the location of an Amazon Simple Storage Service (Amazon S3) bucket.
- Choose Create.
The tracking server may require up to 20 minutes to initialize and become operational. When it’s running, you can note its ARN to use in the llm_fine_tuning_experiments_mlflow.ipynb
notebook. The ARN will have the following format:
For subsequent steps, you can refer to the detailed description provided in this post, as well as the step-by-step instructions outlined in the llm_fine_tuning_experiments_mlflow.ipynb notebook. You can Launch the notebook in Amazon SageMaker Studio Classic or SageMaker JupyterLab.
Overview of SageMaker Pipelines for experimentation at scale
We use SageMaker Pipelines to orchestrate LLM fine-tuning and evaluation experiments. With SageMaker Pipelines, you can:
- Run multiple LLM experiment iterations simultaneously, reducing overall processing time and cost
- Effortlessly scale up or down based on changing workload demands
- Monitor and visualize the performance of each experiment run with MLflow integration
- Invoke downstream workflows for further analysis, deployment, or model selection
MLflow integration with SageMaker Pipelines requires the tracking server ARN. You also need to add the mlflow and sagemaker-mlflow Python packages as dependencies in the pipeline setup. Then you can use MLflow in any pipeline step with the following code snippet:
Log datasets with MLflow
With MLflow, you can log your dataset information alongside other key metrics, such as hyperparameters and model evaluation. This enables tracking and reproducibility of experiments across different runs, allowing for more informed decision-making about which models perform best on specific tasks or domains. By logging your datasets with MLflow, you can store metadata, such as dataset descriptions, version numbers, and data statistics, alongside your MLflow runs.
In the preproccess step, you can log training data and evaluation data. In this example, we download the data from a Hugging Face dataset. We are using HuggingFaceH4/no_robots for fine-tuning and evaluation. First, you need to set the MLflow tracking ARN and experiment name to log data. After you process the data and select the required number of rows, you can log the data using the log_input API of MLflow. See the following code:
Fine-tune a Llama model with LoRA and MLflow
To streamline the process of fine-tuning LLM with Low-Rank Adaption (LoRA), you can use MLflow to track hyperparameters and save the resulting model. You can experiment with different LoRA parameters for training and log these parameters along with other key metrics, such as training loss and evaluation metrics. This enables tracking of your fine-tuning process, allowing you to identify the most effective LoRA parameters for a given dataset and task.
For this example, we use the PEFT library from Hugging Face to fine-tune a Llama 3 model. With this library, we can perform LoRA fine-tuning, which offers faster training with reduced memory requirements. It can also work well with less training data.
We use the HuggingFace class from the SageMaker SDK to create a training step in SageMaker Pipelines. The actual implementation of training is defined in llama3_fine_tuning.py
. Just like the previous step, we need to set the MLflow tracking URI and use the same run_id
:
While using the Trainer class from Transformers, you can mention where you want to report the training arguments. In our case, we want to log all the training arguments to MLflow:
When the training is complete, you can save the full model, so you need to merge the adapter weights to the base model:
The merged model can be logged to MLflow with the model signature, which defines the expected format for model inputs and outputs, including any additional parameters needed for inference:
Evaluate the model
Model evaluation is the key step to select the most optimal training arguments for fine-tuning the LLM for a given dataset. In this example, we use the built-in evaluation capability of MLflow with the mlflow.evaluate() API. For question answering models, we use the default evaluator logs exact_match, token_count, toxicity, flesch_kincaid_grade_level, and ari_grade_level.
MLflow can load the model that was logged in the fine-tuning step. The base model is downloaded from Hugging Face and adapter weights are downloaded from the logged model. See the following code:
These evaluation results are logged in MLflow in the same run that logged the data processing and fine-tuning step.
Create the pipeline
After you have the code ready for all the steps, you can create the pipeline:
You can run the pipeline using the SageMaker Studio UI or using the following code snippet in the notebook:
Compare experiment results
After you start the pipeline, you can track the experiment in MLflow. Each run will log details of the preprocessing, fine-tuning, and evaluation steps. The preprocessing step will log training and evaluation data, and the fine-tuning step will log all training arguments and LoRA parameters. You can select these experiments and compare the results to find the optimal training parameters and best fine-tuned model.
You can open the MLflow UI from SageMaker Studio.
Then you can select the experiment to filter out runs for that experiment. You can select multiple runs to make the comparison.
When you compare, you can analyze the evaluation score against the training arguments.
Register the model
After you analyze the evaluation results of different fine-tuned models, you can select the best model and register it in MLflow. This model will be automatically synced with Amazon SageMaker Model Registry.
Deploy the model
You can deploy the model through the SageMaker console or SageMaker SDK. You can pull the model artifact from MLflow and use the ModelBuilder
class to deploy the model:
Clean up
In order to not incur ongoing costs, delete the resources you created as part of this post:
- Delete the MLflow tracking server.
- Run the last cell in the notebook to delete the SageMaker pipeline:
Conclusion
In this post, we focused on how to run LLM fine-tuning and evaluation experiments at scale using SageMaker Pipelines and MLflow. You can use managed MLflow from SageMaker to compare training parameters and evaluation results to select the best model and deploy that model in SageMaker. We also provided sample code in a GitHub repository that shows the fine-tuning, evaluation, and deployment workflow for a Llama3 model.
You can start taking advantage of SageMaker with MLflow for traditional MLOps or to run LLM experimentation at scale.
About the Authors
Jagdeep Singh Soni is a Senior Partner Solutions Architect at AWS based in the Netherlands. He uses his passion for Generative AI to help customers and partners build GenAI applications using AWS services. Jagdeep has 15 years of experience in innovation, experience engineering, digital transformation, cloud architecture and ML applications.
Dr. Sokratis Kartakis is a Principal Machine Learning and Operations Specialist Solutions Architect for Amazon Web Services. Sokratis focuses on enabling enterprise customers to industrialize their ML and generative AI solutions by exploiting AWS services and shaping their operating model, such as MLOps/FMOps/LLMOps foundations, and transformation roadmap using best development practices. He has spent over 15 years inventing, designing, leading, and implementing innovative end-to-end production-level ML and AI solutions in the domains of energy, retail, health, finance, motorsports, and more.
Kirit Thadaka is a Senior Product Manager at AWS focused on generative AI experimentation on Amazon SageMaker. Kirit has extensive experience working with customers to build scalable workflows for MLOps to make them more efficient at bringing models to production.
Piyush Kadam is a Senior Product Manager for Amazon SageMaker, a fully managed service for generative AI builders. Piyush has extensive experience delivering products that help startups and enterprise customers harness the power of foundation models.
Discover insights from Amazon S3 with Amazon Q S3 connector
Amazon Q is a fully managed, generative artificial intelligence (AI) powered assistant that you can configure to answer questions, provide summaries, generate content, gain insights, and complete tasks based on data in your enterprise. The enterprise data required for these generative-AI powered assistants can reside in varied repositories across your organization. One common repository to store data is Amazon Simple Storage Service (Amazon S3), which is an object storage service that stores data as objects within storage buckets. Customers of all sizes and industries can securely index data from a variety of data sources such as document repositories, web sites, content management systems, customer relationship management systems, messaging applications, database, and so on.
To build a generative AI-based conversational application that’s integrated with the data sources that contain the relevant content an enterprise needs to invest time, money, and people, you need to build connectors to the data sources. Next you need to index the data to make it available for a Retrieval Augmented Generation (RAG) approach where relevant passages are delivered with high accuracy to a large language model (LLM). To do this you need to select an index that provides the capabilities to index the content for semantic and vector search, build the infrastructure to retrieve the data, rank the answers, and build a feature rich web application. You also need to hire and staff a large team to build, maintain and manage such a system.
Amazon Q Business is a fully managed generative AI-powered assistant that can answer questions, provide summaries, generate content, and securely complete tasks based on data and information in your enterprise systems. Amazon Q business can help you get fast, relevant answers to pressing questions, solve problems, generate content, and take actions using the data and expertise found in your company’s information repositories, code, and enterprise systems such as Atlassian Jira and others. To do this, Amazon Q provides native data source connectors that can index content into a built-in retriever and uses an LLM to provide accurate, well written answers. A data source connector within Amazon Q helps to integrate and synchronize data from multiple repositories into one index.
Amazon Q Business offers multiple prebuilt connectors to a large number of data sources, including Atlassian Jira, Atlassian Confluence, Amazon S3, Microsoft SharePoint, Salesforce, and many more and can help you create your generative AI solution with minimal configuration. For a full list of Amazon Q supported data source connectors, see Amazon Q connectors.
Now you can use the Amazon Q S3 connector to index your data on S3 and build a generative AI assistant that can derive insights from the data stored. Amazon Q generates comprehensive responses to natural language queries from users by analyzing information across content that it has access to. Amazon Q also supports access control for your data so that the right users can access the right content. Its responses to questions are based on the content that your end user has permissions to access.
This post shows how to configure the Amazon Q S3 connector and derive insights by creating a generative-AI powered conversation experience on AWS using Amazon Q while using access control lists (ACLs) to restrict access to documents based on user permissions.
Finding accurate answers from content in S3 using Amazon Q Business
After you integrate Amazon Q Business with Amazon S3, users can ask questions about the content stored in S3. For example, a user might ask about the main points discussed in a blog post on cloud security, the installation steps outlined in a user guide, findings from a case study on hybrid cloud usage, market trends noted in an analyst report, or key takeaways from a whitepaper on data encryption. This integration helps users to quickly find the specific information they need, improving their understanding and ability to make informed business decisions.
Secure querying with ACL crawling and identity crawling
Secure querying is when a user runs a query and is returned answers from documents that the user has access to and not from documents that the user does not have access to. To enable users to do secure querying, Amazon Q Business honors ACLs of the documents. Amazon Q Business does this by first supporting the indexing of ACLs. Indexing documents with ACLs is crucial for maintaining data security, because documents without ACLs are treated as public. Second, at query time the user’s credentials (email address) are passed along with the query so that only answers from documents that are relevant to the query and that the user is authorized to access are displayed.
A document’s ACL, included in the metadata.json or acl.json files alongside the document in the S3 bucket, contains details such as the user’s email address and local groups.
When a user signs in to a web application to conduct a search, their credentials (such as an email address) need to match what’s in the ACL of the document to return results from that document. The web application that the user uses to retrieve answers would be connected to an identity provider (IdP) or the AWS IAM Identity Center. The user’s credentials from the IdP or IAM Identity Center are referred to here as the federated user credentials. The federated user credentials are passed along with the query so that Amazon Q can return the answers from the documents that this user has access to. However, there are occasions when a user’s federated credentials might be absent from the S3 bucket ACLs. In these instances, only the user’s local alias and local groups are specified in the document’s ACL. Therefore, it’s necessary to map these federated user credentials to the corresponding local user alias and local group in the document’s ACL.
Any document or folder without an explicit ACL Deny clause is treated as public.
Solution overview
As an administrator user of Amazon Q, the high-level steps to set up a generative AI chat application are to create an Amazon Q application, connect to different data sources, and finally deploy your web experience. An Amazon Q web experience is the chat interface that you create using your Amazon Q application. Then, your users can chat with your organization’s Amazon Q web experience, and it can be integrated with IAM Identity Center. You can configure and customize your Amazon Q web experience using either the AWS Management Console for Amazon Q or the Amazon Q API.
Amazon Q understands and respects your existing identities, roles, and permissions and uses this information to personalize its interactions. If a user doesn’t have permission to access data without Amazon Q, they can’t access it using Amazon Q either. The following table outlines which documents each user is authorized to access for our use case. The documents being used in this example are a subset of AWS public documents. In this blog post, we will focus on users Arnav (Guest), Mary, and Pat and their assigned groups.
First name | Last name | Group | Document type authorized for access | |
1 | Arnav | Desai | Blogs | |
2 | Pat | Candella | Customer | Blogs, user guides |
3 | Jane | Doe | Sales | Blogs, user guides, and case studies |
4 | John | Stiles | Marketing | Blogs, user guides, case studies, and analyst reports |
5 | Mary | Major | Solutions architect | Blogs, user guides, case studies, analyst reports, and whitepapers |
Architecture diagram
The following diagram illustrates the solution architecture. Amazon S3 is the data source and documents along with the ACL information are passed to Amazon Q from S3. The user submits a query to the Amazon Q application. Amazon Q retrieves the user and group information and provides answers based on the documents that the user has access to.
In the upcoming sections, we will show you how to implement this architecture.
Prerequisites
For this walkthrough, you should have the following prerequisites:
- An AWS account.
- Amazon S3 and IAM Identity Center permissions.
- Privileges to create an Amazon Q application, AWS resources, and AWS Identity and Access Management (IAM) roles and policies.
- Basic knowledge of AWS services and working knowledge of S3.
- Follow the steps for Setting up for Amazon Q Business if you’re using Amazon Q Business for the first time.
Prepare your S3 bucket as a data source
In the AWS Region list, choose US East (N. Virginia) as the Region. You can choose any Region that Amazon Q is available in but ensure that you remain in the same Region when creating all other resources. To prepare an S3 bucket as a data source, create an S3 bucket. Note the name of the S3 bucket. Replace <REPLACE-WITH-NAME-OF-S3-BUCKET>
with the name of the bucket in the commands below. In a terminal with the AWS Command Line Interface (AWS CLI) or AWS CloudShell, run the following commands to upload the documents to the data source bucket:
The documents being queried are stored in an S3 bucket. Each document type has a separate folder: blogs, case-studies, analyst reports, user guides, and white papers. This folder structure is contained in a folder named Data as shown below:
Each object in S3 is considered a single document. Any <object-name>.metadata.json file and access control list (ACL) file is considered metadata for the object it’s associated with and not treated as a separate document. In this example, metadata files including the ACLs are in a folder named Meta. We use the Amazon Q S3 connector to configure this S3 bucket as the data source. When the data source is synced with the Amazon Q index, it crawls and indexes all documents and collects the ACLs and document attributes from the metadata files. To learn more about ACLs using metadata files, see Amazon S3 document metadata. Here’s the sample metadata JSON file:
Create users and groups in IAM Identity Center
In this section, you create the following mapping for demonstration:
User | Group name | |
1 | Arnav | |
2 | Pat | customer |
3 | Mary | AWS-SA |
To create users:
- Open the AWS IAM Identity Center
- If you haven’t enabled IAM Identity Center, choose Enable. If there’s a pop-up, choose how you want to enable IAM Identity Center. For this example, select Enable only in this AWS account. Choose Continue.
- In the IAM Identity Center dashboard, choose Users in the navigation pane.
- Choose Add User.
- Enter the user details for Mary:
- Username: mary_major
- Email address: mary_major@example.com
Note: Use or create a real email address for each user to use in a later step. - First name: Mary
- Last name: Major
- Display name: Mary Major
- Skip the optional fields and choose Next to create the user.
- In the Add user to groups page, choose Next and then choose Add user. Follow the same steps to create users for Pat and Arnav (Guest user).
(You will assign users to groups at a later step.)
To create groups:
- Now, you will create two groups: AWS-SA and customer. Choose Groups on the navigation pane and choose Create group.
- For the group name, enter AWS-SA, add user Mary to the group,and choose Create group.
- Similarly, create a group name customer, add user Pat, and choose Create group.
- Now, add multi-factor authentication to the users following the instructions sent to the user email. For more details, see Multi-factor authentication for Identity Center users. When done, you will have the users and groups set up on IAM Identity Center.
Create and configure your Amazon Q application
In this step, you create an Amazon Q application that powers the conversation web experience:
- On the AWS Management Console for Amazon Q, in the Region list, choose US East (N. Virginia).
- On the Getting started page, select Enable identity-aware sessions. Once enabled, Amazon Q connected to IAM Identity Center should be displayed. Choose Subscribe in Q Business.
- On the Amazon Q Business console, choose Get started.
- On the Applications page, choose Create application.
- On the Create application page, enter Application name and leave everything else with default values.
- Choose Create.
- On the Select retriever page, for Retrievers, select Use native retriever.
- Choose Next. This will take you to the Connect data sources
Configure Amazon S3 as the data source
In this section, you walk through an example of adding an S3 connector. The S3 connector consists of blogs, user guides, case studies, analyst reports, and whitepapers.
To add the S3 connector:
- On the Connect data sources page, select Amazon S3 connector.
- For Data source name, enter a name for your data source.
- In the IAM role section, select Create new service role (Recommended).
- In Sync scope section, browse to your S3 bucket containing the data files.
- Under Advanced settings, for Metadata files prefix folder location, enter Meta/
- Choose Filter patterns. Under Include patterns, enter Data/ as the prefix and choose Add.
- For Frequency under Sync run schedule, choose Run on demand.
- Leave the rest as default and choose Add data source. Wait until the data source is added.
- On the Connect data sources page, choose Next. This will take you to the Add users and groups
Add users and groups in Amazon Q
In this section, you set up users and groups to showcase how access can be managed based on the permissions.
- On the Add users and groups page, choose Assign existing users and groups and choose Next.
- Enter the users and groups you want to add and choose Assign. You will have to enter the user names and groups in the search box and select the user or group. Verify that users and groups are correctly displayed under the Users and Groups tabs respectively.
- Select the Current subscription. In this example, we selected choose Q Business Lite for groups. Choose the same subscription for users under the Users tab. You can also update subscriptions after creating the application.
- Leave the Service role name as default and choose Create application.
Sync S3 data source
With your application created, you will crawl and index the documents in the S3 bucket created at the beginning of the process.
- Select the name of the application
- Go to the Data sources Select the radio button next to the S3 data source and choose Sync now.
- The sync can take from a few minutes to a few hours. Wait for the sync to complete. Verify the sync is complete and documents have been added.
Run queries with Amazon Q
Now that you have configured the Amazon Q application and integrated it with IAM Identity Center, you can test queries from different users based on their group permissions. This will demonstrate how Amazon Q respects the access control rules set up in the Amazon S3 data source.
You have three users for testing—Pat from the Customer group, Mary from the AWS-SA group, and Arnav who isn’t part of any group. According to the access control list (ACL) configuration, Pat should have access to blogs and user guides, Mary should have access to blogs, user guides, case studies, analyst reports, and whitepapers, and Arnav should have access only to blogs.
In the following steps, you will sign in as each user and ask various questions to see what responses Amazon Q provides based on the permitted document types for their respective groups. You will also test edge cases where users try to access information from restricted sources to validate the access control functionality.
- In the Amazon Q Business console, choose Applications on the navigation pane and copy the Web experience URL.
Sign in as Pat to the Amazon Q chat interface.
Pat is part of the Customer group and has access to blogs and user guides
When asked a question like “What is AWS?” Amazon Q will provide a summary pulling information from blogs and user guides, highlighting the sources at the end of each excerpt.
Try asking a question that requires information from user guides, such as “How do I set up an AWS account?” Amazon Q will summarize relevant details from the permitted user guide sources for Pat’s group.
However, if you, as Pat, ask a question that requires information from whitepapers, analyst reports, or case studies, Amazon Q will indicate that it could not find any relevant information from the sources she has access to.
Ask a question such as “What are the strategic planning assumptions for the year 2025?” to see this.
Sign in as Mary to the Amazon Q chat interface.
Sign out as user Pat. Start a new incognito browser session or use a different browser. Copy the web experience URL and sign in as user Mary. Repeat these steps each time you need to sign in as a different user.
Mary is part of the AWS-SA group, so she has access to blogs, case studies, analyst reports, and whitepapers.
When Mary asks the same question about strategic planning, Amazon Q will provide a comprehensive summary pulling information from all the permitted sources.
With Mary’s sign-in, you can ask various other questions related to AWS services, architectures, or solutions, and Amazon Q will effectively summarize information from across all the content types Mary’s group has access to.
Sign in as Arnav to the Amazon Q chat interface
Arnav is not part of any group and is able to access only blogs. If Arnav asks a question about Amazon Polly, Amazon Q will return blog posts.
When Arnav tries to get information from the user guides, access is restricted. If they ask about something like how to set up an AWS account, Amazon Q responds that it could not find relevant information.
This shows how Amazon Q respects the data access rules configured in the Amazon S3 data source, allowing users to gain insights only from the content their group has permissions to view, while still providing comprehensive answers when possible within those boundaries.
Troubleshooting
Troubleshooting your Amazon S3 connector provides information about error codes you might see for the Amazon S3 connector and suggested troubleshooting actions. If you encounter an HTTP status code 403 (Forbidden) error when you open your Amazon Q Business application, it means that the user is unable to access the application. See Troubleshooting Amazon Q Business and identity provider integration for common causes and how to address them.
Frequently asked questions
Q. Why isn’t Amazon Q Business answering any of my questions?
A. Verify that you have synced your data source on the Amazon Q console. Also, check the ACLs to ensure you have the required permissions to retrieve answers from Amazon Q.
Q. How can I sync documents without ACLs?
A. When configuring the Amazon S3 connector, under Sync scope, you can optionally choose not to include the metadata or ACL configuration file location in Advanced settings. This will allow you to sync documents without ACLs.
Q. I updated the contents of my S3 data source but Amazon Q business answers using old data.
A. After content has been updated in your S3 data source location, you must re-sync the contents for the updated data to be picked up by Amazon Q. Go to the Data sources Select the radio button next to the S3 data source and choose Sync now. After the sync is complete, verify that the updated data is reflected by running queries on Amazon Q.
Q. I am unable to sign in as a new user through the web experience URL.
A. Clear your browser cookies and sign in as a new user.
Q. I keep trying to sign in but am getting this error:
A. Try signing in from a different browser or clear browser cookies and try again.
Q. What are the supported document formats and what is considered a document in Amazon S3?
A. See Supported document types and What is a document? to learn more.
Call to action
Explore other features in Amazon Q Business such as:
- The Amazon Q Business document enrichment feature helps you control both what documents and document attributes are ingested into your index and also how they’re ingested. Using document enrichment, you can create, modify, or delete document attributes and document content when you ingest them into your Amazon Q Business index. For example, you can scrub personally identifiable information (PII) by choosing to delete any document attributes related to PII.
- Amazon Q Business features
- Filtering using metadata – Use document attributes to customize and control users’ chat experience. Currently supported only if you use the Amazon Q Business API.
- Source attribution with citations – Verify responses using Amazon Q Business source attributions.
- Upload files and chat – Let users upload files directly into chat and use uploaded file data to perform web experience tasks.
- Quick prompts – Feature sample prompts to inform users of the capabilities of their Amazon Q Business web experience.
- To improve retrieved results and customize the user chat experience, you can map document attributes from your data sources to fields in your Amazon Q index. Learn more by exploring Amazon Q Business Amazon S3 data source connector field mappings.
Clean up
To avoid incurring future charges and to clean out unused roles and policies, delete the resources you created: the Amazon Q application, data sources, and corresponding IAM roles.
- To delete the Amazon Q application, go to the Amazon Q console and, on the Applications page, select your application.
- On the Actions drop-down menu, choose Delete.
- To confirm deletion, enter delete in the field and choose Delete. Wait until you get the confirmation message; the process can take up to 15 minutes.
- To delete the S3 bucket created in Prepare your S3 bucket as a data source, empty the bucket and then follow the steps to delete the bucket.
- Delete your IAM Identity Center instance.
Conclusion
This blog post has walked you through the steps to build a secure, permissions-based generative AI solution using Amazon Q and Amazon S3 as the data source. By configuring user groups and mapping their access privileges to different document folders in S3, it demonstrated that Amazon Q respects these access control rules. When users query the AI assistant, it provides comprehensive responses by analyzing only the content their group has permission to view, preventing unauthorized access to restricted information. This solution allows organizations to safely unlock insights from their data repositories using generative AI while ensuring data access governance.
Don’t let your data’s potential go untapped. Continue exploring how Amazon Q can transform your enterprise data to gain actionable insights. Join the conversation and share your thoughts or questions in the comments section below.
About the Author
Kruthi Jayasimha Rao is a Partner Solutions Architect with a focus in AI and ML. She provides technical guidance to AWS Partners in following best practices to build secure, resilient, and highly available solutions in the AWS Cloud.
Keagan Mirazee is a Partner Solutions Architect specializing in Generative AI to assist AWS Partners in engineering reliable and scalable cloud solutions.
Dipti Kulkarni is a Sr. Software Development Engineer for Amazon Q. Dipti is a passionate engineer building connectors for Amazon Q.
Boosting Salesforce Einstein’s code generating model performance with Amazon SageMaker
This post is a joint collaboration between Salesforce and AWS and is being cross-published on both the Salesforce Engineering Blog and the AWS Machine Learning Blog.
Salesforce, Inc. is an American cloud-based software company headquartered in San Francisco, California. It provides customer relationship management (CRM) software and applications focused on sales, customer service, marketing automation, ecommerce, analytics, and application development. Salesforce is building toward artificial general intelligence (AGI) for business, enabling predictive and generative functions within their flagship software-as-a-service (SaaS) CRM, and working toward intelligent automations using artificial intelligence (AI) as well as agents.
Salesforce Einstein is a set of AI technologies that integrate with Salesforce’s Customer Success Platform to help businesses improve productivity and client engagement. Einstein has a list of over 60 features, unlocked at different price points and segmented into four main categories: machine learning (ML), natural language processing (NLP), computer vision, and automatic speech recognition. Einstein delivers advanced AI capabilities into sales, service, marketing, and other functions, empowering companies to deliver more personalized and predictive customer experiences. Einstein has out-of-the-box AI features such as sales email generation in Sales Cloud and service replies in Service Cloud. They also have tools such as Copilot, Prompt, and Model Builder, three tools contained in the Einstein 1 Studio, that allow organizations to build custom AI functionality and roll it out to their users.
The Salesforce Einstein AI Platform team is the group supporting development of Einstein applications. They are committed to enhancing the performance and capabilities of AI models, with a particular focus on large language models (LLMs) for use with Einstein product offerings. These models are designed to provide advanced NLP capabilities for various business applications. Their mission is to continuously refine these LLMs and AI models by integrating state-of-the-art solutions and collaborating with leading technology providers, including open source communities and public cloud services like AWS and building it into a unified AI platform. This helps make sure Salesforce customers receive the most advanced AI technology available.
In this post, we share how the Salesforce Einstein AI Platform team boosted latency and throughput of their code generation LLM using Amazon SageMaker.
The challenge with hosting LLMs
In the beginning of 2023, the team started looking at solutions to host CodeGen, Salesforce’s in-house open source LLM for code understanding and code generation. The CodeGen model allows users to translate natural language, such as English, into programming languages, such as Python. Because they were already using AWS for inference for their smaller predictive models, they were looking to extend the Einstein platform to help them host CodeGen. Salesforce developed an ensemble of CodeGen models (Inline for automatic code completion, BlockGen for code block generation, and FlowGPT for process flow generation) specifically tuned for the Apex programming language. Salesforce Apex is a certified framework for building SaaS apps on top of Salesforce’s CRM functionality. They were looking for a solution that can securely host their model and help them handle a large volume of inference requests as well as multiple concurrent requests at scale. They also needed to be able to meet their throughput and latency requirements for their co-pilot application (EinsteinGPT for Developers). EinsteinGPT for Developers simplifies the start of development by creating smart Apex based on natural language prompts. Developers can accelerate coding tasks by scanning for code vulnerabilities and getting real-time code suggestions within the Salesforce integrated development environment (IDE), as shown in the following screenshot.
The Einstein team conducted a comprehensive evaluation of various tools and services, including open source options and paid solutions. After assessing these options, they found that SageMaker provided the best access to GPUs, scalability, flexibility, and performance optimizations for a wide range of scenarios, particularly in addressing their challenges with latency and throughput.
Why Salesforce Einstein chose SageMaker
SageMaker offered several specific features that proved essential to meeting Salesforce’s requirements:
- Multiple serving engines – SageMaker includes specialized deep learning containers (DLCs), libraries, and tooling for model parallelism and large model inference (LMI) containers. LMI containers are a set of high-performance Docker Containers purpose built for LLM inference. With these containers, you can use high performance open source inference libraries like FasterTransformer, TensorRT-LLM, vLLM and Transformers NeuronX. These containers bundle together a model server with open source inference libraries to deliver an all-in-one LLM serving solution. The Einstein team liked how SageMaker provided quick-start notebooks that get them deploying these popular open source models in minutes.
- Advanced batching strategies – The SageMaker LMI allows customers to optimize performance of their LLMs by enabling features like batching, which groups multiple requests together before they hit the model. Dynamic batching instructs the server to wait a predefined amount of time and batch up all requests that occur in that window with a maximum of 64 requests, while paying attention to a configured preferred size. This optimizes the use of GPU resources and balances throughput with latency, ultimately reducing the latter. The Einstein team liked how they were able to use dynamic batching through the LMI to increase throughput for their Codegen models while minimizing latency.
- Efficient routing strategy – By default, SageMaker endpoints have a random routing strategy. SageMaker also supports a least outstanding requests (LOR) strategy, which allows SageMaker to optimally route requests to the instance that’s best suited to serve that request. SageMaker makes this possible by monitoring the load of the instances behind your endpoint and the models or inference components that are deployed on each instance. Customers have the flexibility to choose either algorithm depending on their workload needs. Along with the capability to handle multiple model instances across several GPUs, the Einstein team liked how the SageMaker routing strategy ensures that traffic is evenly and efficiently distributed to model instances, preventing any single instance from becoming a bottleneck.
- Access to high-end GPUs – SageMaker provides access to top-end GPU instances, which are essential for running LLMs efficiently. This is particularly valuable given the current market shortages of high-end GPUs. SageMaker allowed the Einstein team to use auto-scaling of these GPUs to meet demand without manual intervention.
- Rapid iteration and deployment – While not directly related to latency, the ability to quickly test and deploy changes using SageMaker notebooks helps in reducing the overall development cycle, which can indirectly impact latency by accelerating the implementation of performance improvements. The use of notebooks enabled the Einstein team to shorten their overall deployment time and get their models hosted in production much faster.
These features collectively help optimize the performance of LLMs by reducing latency and improving throughput, making Amazon SageMaker a robust solution for managing and deploying large-scale machine learning models.
One of the key capabilities was how using SageMaker LMI provided a blueprint of model performance optimization parameters for NVIDIA’s FasterTransformer library to use with CodeGen. When the team initially deployed CodeGen 2.5, a 7B parameter model on Amazon Elastic Compute Cloud (Amazon EC2), the model wasn’t performing well for inference. Initially, for a code block generation task, it could only handle six requests per minute, with each request taking over 30 seconds to process. This was far from efficient and scalable. However, after using the SageMaker FasterTransformer LMI notebook and referencing the advanced SageMaker-provided guides to understand how to optimize the different endpoint parameters provided, there was a significant improvement in model performance. The system now handles around 400 requests per minute with a reduced latency of approximately seven seconds per request, each containing about 512 tokens. This represents an over 6,500 percent increase in throughput after optimization. This enhancement was a major breakthrough, demonstrating how the capabilities of SageMaker were instrumental in optimizing the throughput of the LLM and reducing cost. (The FasterTransformer backend has been deprecated by NVIDIA; the team is working toward migrating to the TensorRT (TRT-LLM) LMI.)
To assess the performance of LLMs, the Einstein team focuses on two key metrics:
- Throughput – Measured by the number of tokens an LLM can generate per second
- Latency – Determined by the time it takes to generate these tokens for individual requests
Extensive performance testing and benchmarking was conducted to track these metrics. Before using SageMaker, CodeGen models had a lower token-per-second rate and higher latencies. With SageMaker optimization, the team observed significant improvements in both throughput and latency, as shown in the following figure.
Latency and throughput changes with different techniques for CodeGen1 and CodeGen2.5 models. CodeGen1 is the original version of CodeGen, which is a 16B model. CodeGen2.5 is the optimized version, which is a 7B model. For more information about CodeGen 2.5, refer to CodeGen2.5: Small, but mighty.
New challenges and opportunities
The primary challenge that the team faced when integrating SageMaker was enhancing the platform to include specific functionalities that were essential for their projects. For instance, they needed additional features for NVIDIA’s FasterTransformer to optimize their model performance. Through a productive collaboration with the SageMaker team, they successfully integrated this support, which initially was not available.
Additionally, the team identified an opportunity to improve resource efficiency by hosting multiple LLMs on a single GPU instance. Their feedback helped develop the inference component feature, which now allows Salesforce and other SageMaker users to utilize GPU resources more effectively. These enhancements were crucial in tailoring the platform to Salesforce’s specific needs.
Key takeaways
The team took away the following key lessons from optimizing models in SageMaker for future projects:
- Stay updated – It’s crucial to keep up with the latest inferencing engines and optimization techniques because these advancements significantly influence model optimization.
- Tailor optimization strategies – Model-specific optimization strategies like batching and quantization require careful handling and coordination, because each model might require a tailored approach.
- Implement cost-effective model hosting – You can optimize the allocation of limited GPU resources to control expenses. Techniques such as virtualization can be used to host multiple models on a single GPU, reducing costs.
- Keep pace with innovations – The field of model inferencing is rapidly evolving with technologies like Amazon SageMaker JumpStart and Amazon Bedrock. Developing strategies for adopting and integrating these technologies is imperative for future optimization efforts.
Conclusion
In this post, we shared how the Salesforce Einstein AI Platform team boosted latency and throughput of their code generation LLM using SageMaker, and saw an over 6,500 percent increase in throughput after optimization.
Looking to host your own LLMs on SageMaker? To get started, see this guide.
_______________________________________________________________________
About the Authors
Pawan Agarwal is the Senior Director of Software Engineering at Salesforce. He leads efforts in Generative and Predictive AI, focusing on inferencing, training, fine-tuning, and notebooking technologies that power the Salesforce Einstein suite of applications.
Rielah De Jesus is a Principal Solutions Architect at AWS who has successfully helped various enterprise customers in the DC, Maryland, and Virginia area move to the cloud. In her current role she acts as a customer advocate and technical advisor focused on helping organizations like Salesforce achieve success on the AWS platform. She is also a staunch supporter of Women in IT and is very passionate about finding ways to creatively use technology and data to solve everyday challenges.
Amazon Robotics names 2024 Day One Fellowship Program recipients
Program empowers uniquely merited scholars from backgrounds historically underrepresented in STEM to become industry leaders through scholarship, research, and career opportunities.Read More
Demystifying AI-Assisted Artistry With Adobe Apps Using NVIDIA RTX
Editor’s note: This post is part of the AI Decoded series, which demystifies AI by making the technology more accessible, and showcases new hardware, software, tools and accelerations for RTX PC users.
Adobe Creative Cloud applications, which tap NVIDIA RTX GPUs, are designed to enhance the creativity of users, empowering them to work faster and focus on their craft.
These tools seamlessly integrate into existing creator workflows, enabling greater productivity and delivering power and precision.
Look to the Light
Generative AI creates new data in forms such as images or text by learning from existing data. It effectively visualizes and generates content to match what a user describes and helps open up fresh avenues for creativity.
Adobe Firefly is Adobe’s family of creative generative AI models that offer new ways to ideate and create while assisting creative workflows using generative AI. They’re designed to be safe for commercial use and were trained, using NVIDIA GPUs, on licensed content, like Adobe Stock Images, and public domain content where copyright has expired.
Firefly features are integrated in Adobe’s most popular creative apps.
Adobe Photoshop features the Generative Fill tool, which uses simple description prompts to easily add content from images. With the latest Reference Image feature currently in beta, users can also upload a sample image to get image results closer to their desired output.
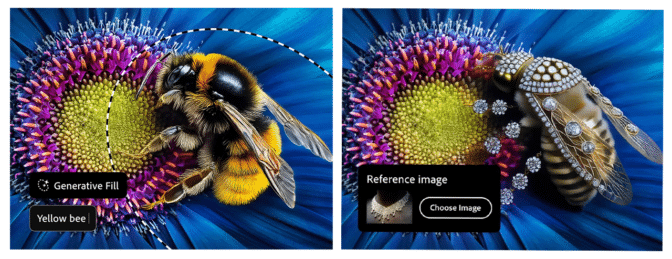
Generative Expand allows artists to extend the border of their image with the Crop tool, filling in bigger canvases with new content that automatically blends in with the existing image.
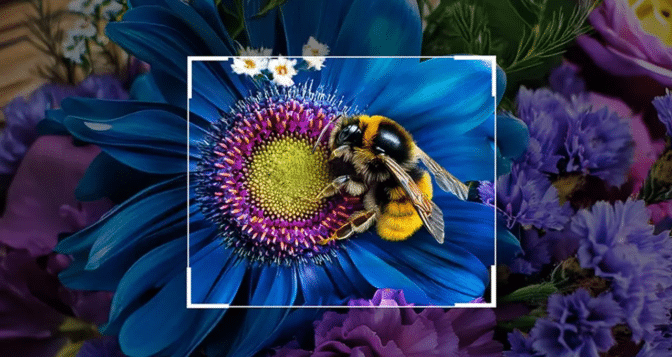
RTX-accelerated Neural Filters, such as Photo Restoration, enable complex adjustments such as colorizing black-and-white photos and performing style transfers using AI. The Smart Portrait filter, which allows non-destructive editing with filters, is based on work from NVIDIA Research.
The brand-new Generative Shape Fill (beta) in Adobe Illustrator, powered by the latest Adobe Firefly Vector Model, allows users to accelerate design workflows by quickly filling shapes with detail and color in their own styles. With Generative Shape Fill, designers can easily match the style and color of their own artwork to create a wide variety of editable and scalable vector graphic options.
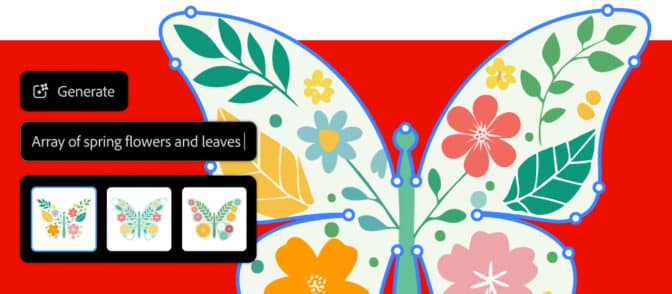
Adobe Illustrator’s Generative Recolor feature lets creators type in a text prompt to explore custom color palettes and themes for their vector artwork in seconds.
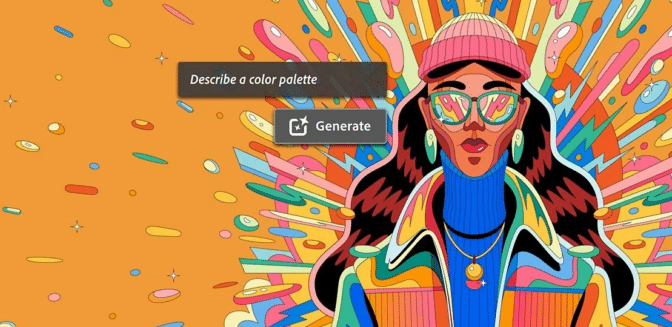
NVIDIA will continue working with Adobe to support advanced generative AI models, with a focus on deep integration into the apps the world’s leading creators use.
Making Moves on Video
Adobe Premiere Pro is one of the most popular and powerful video editing solutions.
Its Enhance Speech tool, accelerated by RTX, uses AI to remove unwanted noise and improve the quality of dialogue clips so they sound professionally recorded. It’s up to 4.5x faster on RTX PCs.
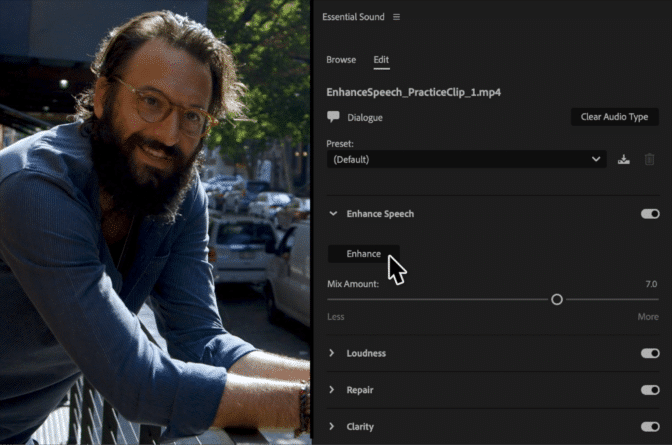
Auto Reframe, another Adobe Premiere feature, uses GPU acceleration to identify and track the most relevant elements in a video, and intelligently reframes video content for different aspect ratios. Scene Edit Detection automatically finds the original edit points in a video, a necessary step before the video editing stage begins.
Visual Effects
Separating a foreground object from a background is a crucial step in many visual effects and compositing workflows.
Adobe After Effects has a new feature that uses a matte to isolate an object, enabling capabilities including background replacement and the selective application of effects to the foreground.
Using the Roto Brush tool, artists can draw strokes on representative areas of the foreground and background elements. After Effects uses that information to create a segmentation boundary between the foreground and background elements, delivering cleaner cutouts with fewer clicks.
Creating 3D Product Shots
The Substance 3D Collection is Adobe’s solution for 3D material authoring, texturing and rendering, enabling users to rapidly create stunningly photorealistic 3D content, including models, materials and lighting.
Visualizing products and designs in the context of a space is compelling, but it can be time-consuming to find the right environment for the objects to live in. Substance 3D Stager’s Generative Background feature, powered by Adobe Firefly, solves this issue by letting artists quickly explore generated backgrounds to composite 3D models.
Once an environment is selected, Stager can automatically match the perspective and lighting to the generated background.
Material Authoring With AI
Adobe Substance 3D Sampler, also part of the Substance 3D Collection, is designed to transform images of surfaces and objects into photorealistic physically based rendering (PBR) materials, 3D models and high-dynamic range environment lights. With the recent introduction of new generative workflows powered by Adobe Firefly, Sampler is making it easier than ever for artists to explore variations when creating materials for everything from product visualization projects to the latest AAA games.
Sampler’s Text-to-Texture feature allows users to generate tiled images from detailed text prompts. These generated images can then be edited and transformed into photorealistic PBR materials using the machine learning-powered Image-to-Material feature or any Sampler filter.
Image-to-Texture similarly enables the creation of tiled textures from reference images, providing an alternate way to prompt and generate variations from existing visual content.
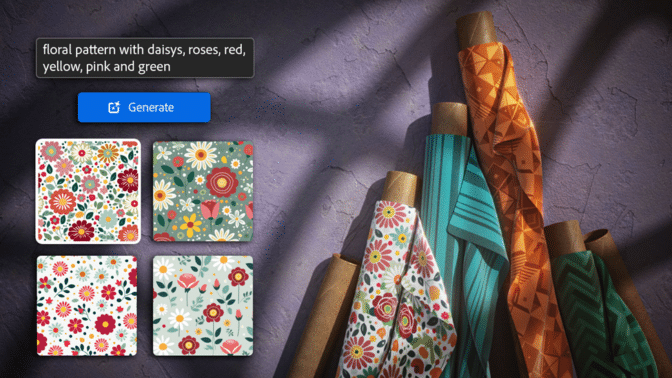
Sampler’s Text-to-Pattern feature uses text prompts to generate tiling patterns, which can be used as base colors or inputs for various filters, such as the Cloth Weave filter for creating original fabric materials.
All of these generative AI features in the Substance 3D Collection, supercharged with RTX GPUs, are designed to help 3D creators ideate and create faster.
Photo-tastic Features
Adobe Lightroom’s AI-powered Raw Details feature produces crisp detail and more accurate renditions of edges, improves color rendering and reduces artifacts, enhancing the image without changing its original resolution. This feature is handy for large displays and prints, where fine details are visible.
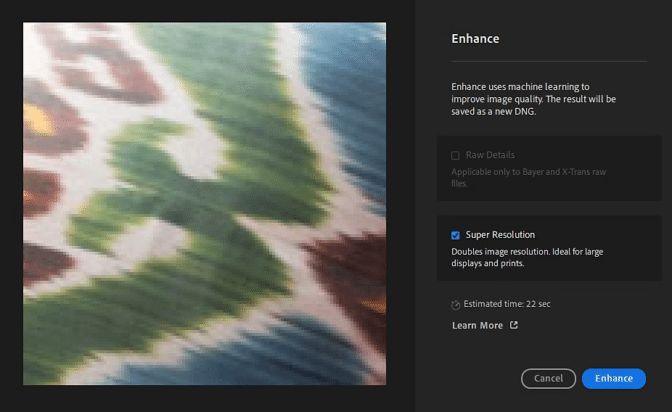
Super Resolution helps create an enhanced image with similar results as Raw Details but with 2x the linear resolution. This means that the enhanced image will have 2x the width and height of the original image — or 4x the total pixel count. This is especially useful for increasing the resolution of cropped imagery.
For faster editing, AI-powered, RTX-accelerated masking tools like Select Subject, which isolates people from an image, and Select Sky, which captures skies, enable users to create complex masks with the click of a button.
Visit Adobe’s AI features page for a complete list of AI features using RTX.
Looking for more AI-powered content creation apps? Consider NVIDIA Broadcast, which transforms any room into a home studio, free for RTX GPU owners.
Generative AI is transforming gaming, videoconferencing and interactive experiences of all kinds. Make sense of what’s new and what’s next by subscribing to the AI Decoded newsletter.