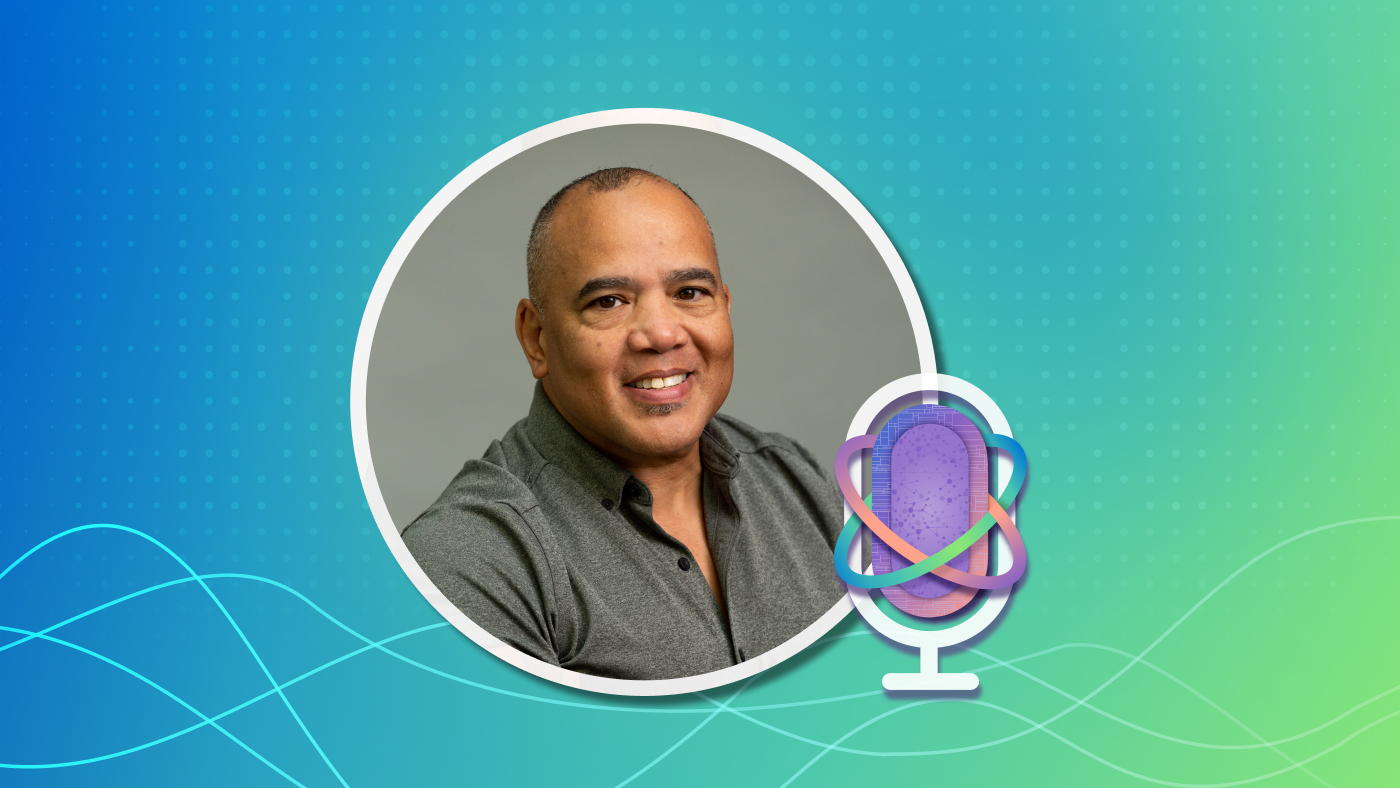
In the Microsoft Research Podcast series What’s Your Story, Johannes Gehrke explores the who behind the technical and scientific advancements helping to reshape the world. A systems expert whose 10 years with Microsoft spans research and product, Gehrke talks to members of the company’s research community about what motivates their work and how they got where they are today.
In this episode, Gehrke is joined by Lex Story, a model maker and fabricator whose craftsmanship has helped bring research to life through prototyping. He’s contributed to projects such as Jacdac, a hardware-software platform for connecting and coding electronics, and the biological monitoring and intelligence platform Premonition. Story shares how his father’s encouragement helped stoke a curiosity that has informed his pursuit of the sciences and art; how his experience with the Marine Corps intensified his desire for higher education; and how his heritage and a sabbatical in which he attended culinary school might inspire his next career move …
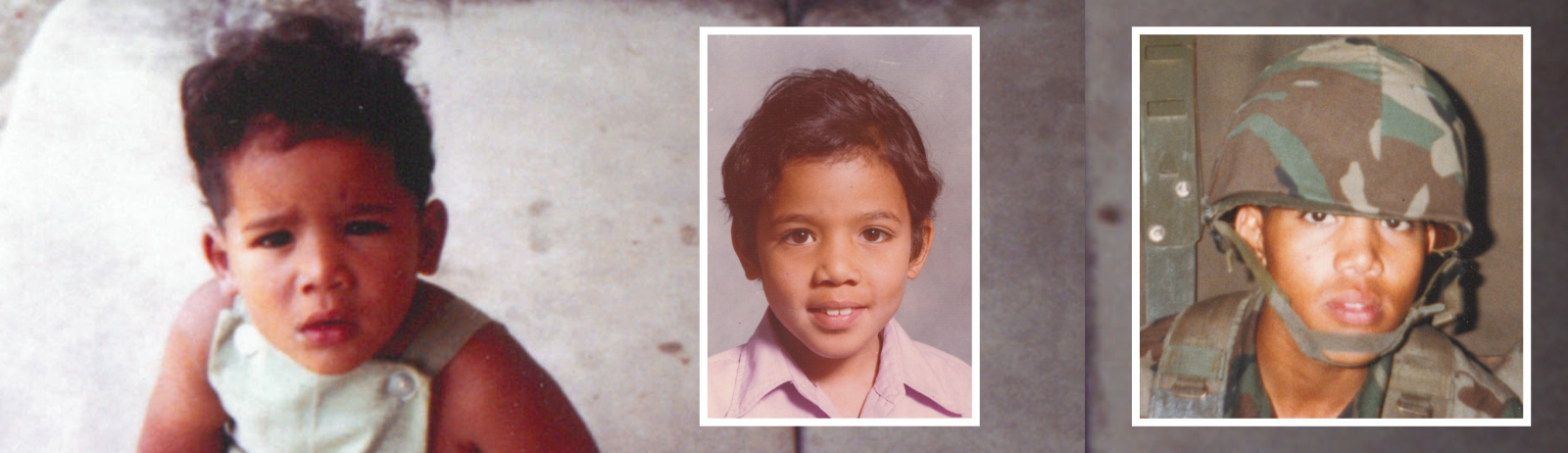
Learn about the projects Story has contributed to:
Subscribe to the Microsoft Research Podcast:
Transcript
[TEASER] [MUSIC PLAYS UNDER DIALOGUE]LEX STORY: Research is about iteration. It’s about failing and failing fast so that you can learn from it. You know, we spin on a dime. Sometimes, we go, whoa, we went the wrong direction. But we learn from it, and it just makes us better.
JOHANNES GEHRKE: Microsoft Research works at the cutting edge. But how much do we know about the people behind the science and technology that we create? This is What’s Your Story, and I’m Johannes Gehrke. In my 10 years with Microsoft, across product and research, I’ve been continuously excited and inspired by the people I work with, and I’m curious about how they became the talented and passionate people they are today. So I sat down with some of them. Now, I’m sharing their stories with you. In this podcast series, you’ll hear from them about how they grew up, the critical choices that shaped their lives, and their advice to others looking to carve a similar path.
[MUSIC FADES]In this episode, I’m talking with model maker and fabricator Lex Story. His creativity and technical expertise in computer-aided industrial design and machining are on display in prototypes and hardware across Microsoft—from Jacdac, a hardware-software platform for connecting and coding electronics, to the biological monitoring and intelligence platform Microsoft Premonition. But he didn’t start out in research. Encouraged by his father, he pursued opportunities to travel, grow, and learn. This led to service in the Marine Corps; work in video game development and jewelry design; and a sabbatical to attend culinary school. He has no plans of slowing down. Here’s my conversation with Lex, beginning with hardware development at Microsoft Research and his time growing up in San Bernardino, California.
GEHRKE: Welcome, Lex.
LEX STORY: Oh, thank you.
GEHRKE: Really great to have you here. Can you tell us a little bit about what you’re doing here at MSR (Microsoft Research) …
STORY: OK.
GEHRKE: … and how did you actually end up here?
STORY: Well, um, within MSR, I actually work in the hardware prototype, hardware development. I find solutions for the researchers, especially in the areas of developing hardware through various fabrication and industrial-like methods. I’m a model maker. My background is as an industrial designer and a product designer. So when I attended school initially, it was to pursue a science; it was [to] pursue chemistry.
GEHRKE: And you grew up in California?
STORY: I grew up in California. I was born in Inglewood, California, and I grew up in San Bernardino, California. Nothing really too exciting happening in San Bernardino, which is why I was compelled to find other avenues, especially to go seek out travel. To do things that I knew that I would be able to look back and say, yes, you’ve definitely done something that was beyond what was expected of you having grown up in San Bernardino.
GEHRKE: And you even had that drive during your high school, or …
STORY: Yeah, high school just didn’t feel like … I think it was the environment that I was growing up in; it didn’t feel as if they really wanted to foster exceptional growth. And I had a father who was … had multiple degrees, and he had a lot of adversity, and he had a lot of challenges. He was an African American. He was a World War II veteran. But he had attained degrees, graduate degrees, in various disciplines, and that included chemical engineering, mechanical engineering, and electrical engineering.
GEHRKE: Wow. All three of them?
STORY: Yes. And so he was … had instilled into us that, you know, education is a vehicle, and if you want to leave this small town, this is how you do it you. But you need to be a vessel. You need to absorb as much as you can from a vast array of disciplines. And not only was he a man of science; he was also an artist. So he always fostered that in us. He said, you know, explore, gain new skills, and the collection of those skills will make you greater overall. He’s not into this idea of being such a specialist. He says lasers are great, but lasers can be blind to what’s happening around them. He says you need to be a spotlight. And he says then you have a great effect on a large—vast, vast array of things instead of just being focused on one thing.
GEHRKE: So you grew up in this environment where the idea was to, sort of, take a holistic view and not, like, a myopic view …
STORY: Yes, yes, yes.
GEHRKE: And so what is the impact of that on you?
STORY: Well, as soon as I went into [LAUGHS] the Marine Corps, I said, now I can attain my education. And …
GEHRKE: So right after school, you went to the …
STORY: I went directly into the Marine Corps right after high school graduation.
GEHRKE: And you told me many times, this is not the Army, right?
STORY: No, it’s the Marine Corps. It’s a big differentiation between … they’re both in military service. However, the Marine Corps is very proud of its traditions, and they instill that in us during boot camp, your indoctrination. It is drilled upon you that you are not just an arm of the military might. You are a professional. You are representative. You will basically become a reflection of all these other Marines who came before you, and you will serve as a point of the young Marines who come after you. So that was drilled into us from day one of boot camp. It was … but it was very grueling. You know, that was the one aspect, and there was a physical aspect. And the Marine Corps boot camp is the longest of all the boot camps. It was, for me, it was 12 weeks of intensive, you know, training. So, you know, the indoctrination is very deep.
GEHRKE: And then so it’s your high school, and you, sort of, have this holistic thinking that you want to bring in.
STORY: Yes.
GEHRKE: And then you go to the Marines.
STORY: I go to the Marines. And the funny thing is that I finished my enlistment, and after my enlistment, I enroll in college, and I say, OK, great; that part of my … phase of life is over. However, I’m still active reserve, and the Desert Shield comes up. So I’m called back, and I said, OK, well, I can come back. I served in a role as an NBC instructor. “NBC” stands for nuclear, biological, chemical warfare. And one of the other roles that I had in the Marine Corps, I was also a nuke tech. That means I knew how to deploy artillery-delivered nuclear-capable warheads. So I had this very technical background mixed in with, like, this military, kind of, decorum. And so I served in Desert Shield, and then eventually that evolved into Operation Desert Storm, and once that was over, I was finally able to go back and actually finish my schooling.
GEHRKE: Mm-hmm. So you studied for a couple of years and then you served?
STORY: Oh, yes, yes.
GEHRKE: OK. OK.
STORY: I had done a four-year enlistment, and you have a period of years after your enlistment where you can be recalled, and it would take very little time for you to get wrapped up for training again to be operational.
GEHRKE: Well, that must be a big disruption right in the middle of your studies, and …
STORY: It was a disruption that …
GEHRKE: And thank you for your service.
STORY: [LAUGHS] Thank you. I appreciate that. It was a disruption, but it was a welcome disruption because, um, it was a job that I knew that I could do well. So I was willing to do it. And when I was ready for college again, it made me a little hungrier for it.
GEHRKE: And you’re already a little bit more mature than the average college student …
STORY: Oh, yes.
GEHRKE: … when you entered, and then now you’re coming back from your, sort of, second time.
STORY: I think it was very important for me to actually have that military experience because [through] that military experience, I had matured. And by the time I was attending college, I wasn’t approaching it as somebody who was in, you know, their teenage years and it’s still formative; you’re still trying to determine who you are as a person. The military had definitely shown me, you know, who I was as a person, and I actually had a few, you know, instances where I actually saw some very horrible things. If anything, being in a war zone during war time, it made me a pacifist, and I have … it increased my empathy. So if anything, there was a benefit from it. I saw some very horrible things, and I saw some amazing things come from human beings on both ends of the spectrum.
GEHRKE: And it’s probably something that’s influenced the rest of your life also in terms of where you went as your career, right?
STORY: Yes.
GEHRKE: So what were you studying, and then what were your next steps?
STORY: Well, I was studying chemistry.
GEHRKE: OK, so not only chemistry and mechanical engineering …
STORY: And then I went away, off to Desert Storm, and when I came back, I decided I didn’t want to study chemistry anymore. I was very interested in industrial design and graphic design, and as I was attending, at ArtCenter College of Design in Pasadena, California, there was this new discipline starting up, but it was only for graduates. It was a graduate program, and it was called computer-aided industrial design. And I said, wait a minute, what am I doing? This is something that I definitely want to do. So it was, like, right at the beginning of computer-generated imagery, and I had known about CAD in a very, very rudimentary form. My father, luckily, had introduced me to computers, so as I was growing up a child in the ’70s and the ’80s, we had computers in our home because my dad was actually building them. So his background and expertise—he was working for RCA; he was working for Northrop Grumman. So I was very familiar with those.
GEHRKE: You built PCs at home, or what, what … ?
STORY: Oh, he built PCs. I learned to program. So I …
GEHRKE: What was your first programming language?
STORY: Oh, it was BASIC …
GEHRKE: BASIC. OK, yup.
STORY: … of course. It was the only thing I could check out in the library that I could get up and running on. So I was surrounded with technology. While most kids went away, summer camp, I spent my summer in the garage with my father. He had metalworking equipment. I understood how to operate metal lathes. I learned how to weld. I learned how to rebuild internal combustion engines. So my childhood was very different from what most children had experienced during their summer break. And also at that time, he was working as a … in chemistry. So his job then, I would go with him and visit his job and watch him work in a lab environment. So it was very, very unique. But also the benefit of that is that being in a lab environment was connected to other sciences. So I got to see other departments. I got to see the geology department. I got to see … there was disease control in the same department that he was in. So I was exposed to all these things. So I was always very hungry and interested, and I was very familiar with sciences. So looking at going back into art school, I said, oh, I’m going to be an industrial designer, and I dabble in art. And I said, wait a minute. I can use technology, and I can create things, and I can guide machines. And that’s the CAM part, computer-aided machining. So I was very interested in that. And then having all of this computer-generated imagery knowledge, I did one of the most knuckleheaded things I could think of, and I went into video game development.
GEHRKE: Why is it knuckleheaded? I mean, it’s probably just the start of big video games.
STORY: Well, I mean … it wasn’t, it wasn’t a science anymore. It was just pursuit of art. And while I was working in video game development, it was fun. I mean, no doubt about it. And that’s how I eventually came to Microsoft, is the company I was working for was bought, purchased by Microsoft.
GEHRKE: But why is it only an art? I’m so curious about this because even computer games, right, there’s probably a lot of science about A/B testing, science of the infrastructure …
STORY: Because I was creating things strictly for the aesthetics.
GEHRKE: I see.
STORY: And I had the struggle in the back of my mind. It’s, like, why don’t we try to create things so that they’re believable, and there’s a break you have to make, and you have to say, is this entertaining? Because in the end, it’s entertainment. And I’ve always had a problem with that.
GEHRKE: It’s about storytelling though, right?
STORY: Yes, it is about storytelling. And that was one of the things that was always told to us: you’re storytellers. But eventually, it wasn’t practical, and I wanted to be impactful, and I couldn’t be impactful doing that. I could entertain you. Yeah, that’s great. It can add some levity to your life. But I was hungry for other things, so I took other jobs eventually. I thought I was going to have a full career with it, and I decided, no, this is not the time to do it.
GEHRKE: That’s a big decision though, right?
STORY: Oh, yeah. Yeah.
GEHRKE: Because, you know, you had a good job at a game company, and then you decided to …
STORY: But there was no, there was no real problem solving for me.
GEHRKE: I see. Mm-hmm.
STORY: And there was opportunity where there was a company, and they were using CAD, and they were running wax printers, and it was a jewel company. And I said, I can do jewelry.
GEHRKE: So what is a wax printer? Explain that.
STORY: Well, here’s … the idea is you can do investment casting.
GEHRKE: Yeah.
STORY: So if you’re creating all your jewelry with CAD, then you can be a jewelry designer and you can have something practical. The reason I took those jobs is because I wanted to learn more about metallurgy and metal casting. And I did that for a bit. And then, eventually, I—because of my computer-generated imagery background—I was able to find a gig with HoloLens. And so as I was working with HoloLens, I kept hearing about research, and they were like, oh yeah, look at this technology research created, and I go, where’s this research department? So I had entertained all these thoughts that maybe I should go and see if I can seek these guys out. And I did find them eventually. My previous manager, Patrick Therien, he brought me in, and I had an interview with him, and he asked me some really poignant questions. And he was a mechanical engineer by background. And I said, I really want to work here, and I need to show you that I can do the work. And he says, you don’t need to prove to me that you can do the work; you have to prove to me that you’re willing to figure it out.
GEHRKE: So how did you do that, or how did you show him?
STORY: I showed him a few examples. I came up with a couple of ideas, and then I demonstrated some solutions, and I was able to present those things to him during the interview. And so I came in as a vendor, and I said, well, if I apply myself, you know, rigorously enough, they’ll see the value in it. And, luckily, I caught the eye of …was it … Gavin [Jancke], and it was Patrick. And they all vouched for me, and they said, yeah, definitely, I have something that I can bring. And it’s always a challenge. The projects that come in, sometimes we don’t know what the solution is going to be, and we have to spend a lot of time thinking about how we’re going to approach it. And we also have to be able to approach it within the scope of what their project entails. They’re trying to prove a concept. They’re trying to publish. I want to make everything look like a car, a beautiful, svelte European designed … but that’s not always what’s asked. So I do have certain parameters I have to stay within, and it’s exciting, you know, to come up with these solutions. I’m generating a concept that in the end becomes a physical manifestation.
GEHRKE: Yeah, so how do you balance this? Because, I mean, from, you know, just listening to your story so far, which is really fascinating, is that there’s always this balance not only on the engineering side but also on the design and art side.
STORY: Yes!
GEHRKE: And then a researcher comes to you and says, I want x.
STORY: Yes, yes, yes. [LAUGHS]
GEHRKE: So how do you, how do you balance that?
STORY: It’s understanding my roles and responsibilities.
GEHRKE: OK.
STORY: It’s a tough conversation. It’s a conversation that I have often with my manager. Because in the end, I’m providing a service, and there are other outlets for me still. Every day, I draw. I have an exercise of drawing where I sit down for at least 45 minutes every day, and I put pen to paper because that is an outlet. I’m a voracious reader. I tackle things because—on a whim. It’s not necessarily that I’m going to become a master of it. So that’s why I attended culinary school. Culinary school fell into this whole curiosity with molecular gastronomy. And I said, wait a minute, I don’t want to be an old man …
GEHRKE: So culinary school is like really very, very in-depth understanding the chemistry of cooking. I mean, the way you understand it …
STORY: Yeah, the molecular gastronomy, the chemistry of cooking. Why does this happen? What is caramelization? What’s the Maillard effect?
GEHRKE: So it’s not about just the recipe for this cake, or so …
STORY: No … the one thing you learn in culinary school very quickly is recipes are inconsequential.
GEHRKE: Oh, really?
STORY: It’s technique.
GEHRKE: OK.
STORY: Because if I have a technique and I know what a roux is and what a roux is doing—and a roux is actually gelatinizing another liquid; it’s a carrier. Once you know these techniques and you can build on those techniques, recipes are irrelevant. Now, the only time recipes matter is when you’re dealing with specific ratios, but that’s still chemistry, and that’s only in baking. But everything else is all technique. I know how to break down the, you know, the connective tissue of a difficult cut of meat. I know what caramelization adds. I understand things like umami. So I look at things in a very, very different way than most people. I’m not like a casual cook, which drove me to go work for Cook’s Illustrated and America’s Test Kitchen, outside of Boston. Because it wasn’t so much about working in a kitchen; it was about exploration, a process. That all falls back into that maddening, you know, part of my personality … it’s like, what is the process? How can I improve that—how can I harness that process?
GEHRKE: So how was it to work there? Because I see food again as, sort of, this beautiful combination of engineering in some sense, creating the recipe. But then there’s also the art of it, right? The presentation.
STORY: Yes …
GEHRKE: And how do you actually put the different flavors together?
STORY: Well, a lot of that’s familiarity because it’s like chemistry. You have familiarity with reactions; you have familiarity and comparisons. So that all falls back into the science. Of course, when I plate it, that falls … I’m now borrowing on my aesthetics, my ability to create aesthetic things. So it fulfills all of those things. So, and that’s why I said, I don’t want to be an old man and say, oh, I wish I’d learned this. I wanted to attend school. I took a sabbatical, attended culinary school.
GEHRKE: So you took a sabbatical from Microsoft?
STORY: Oh, yes, when I was working in video games. Yeah.
GEHRKE: OK.
STORY: I took a sabbatical. I did that. And I was like, great. I got that out of the way. Who’s to say I don’t open a food truck?
GEHRKE: Yeah, I was just wondering, what else is on your bucket list, you know?
STORY: [LAUGHS] I definitely want to do the food truck eventually.
GEHRKE: OK, what would the food truck be about?
STORY: OK. My heritage, my background, is that I’m half Filipino and half French Creole Black.
GEHRKE: You also had a huge family. There’s probably a lot of really good cooking.
STORY: Oh, yeah. Well, I have stepbrothers and stepsisters from my Mexican stepmother, and she grew up cooking Mexican dishes. She was from the Sinaloa area of Mexico. And so I learned a lot of those things, very, very unique regional things that were from her area that you can’t find anywhere else.
GEHRKE: What’s an example? Now you’ve made me curious.
STORY: Capirotada. Capirotada is a Mexican bread pudding, and it utilizes a lot of very common techniques, but the ingredients are very specific to that region. So the preparation is very different. And I’ve had a lot of people actually come to me and say, I’ve never had capirotada like that. And then I have other people who say, that is exactly the way I had it. And by the way, my, you know, my family member was from the Sinaloa area. So, yeah, but from my Filipino heritage background, I would love to do something that is a fusion of Filipino foods. There’s a lot of great, great food like longganisa; there’s a pancit. There’s adobo. That’s actually adding vinegars to braised meats and getting really great results that way. It’s just a … but there’s a whole bevy of … but my idea eventually for a food truck, I’m going to keep that under wraps for now until I finally reveal it. Who’s, who’s to say when it happens.
GEHRKE: OK. Wow, that sounds super interesting. And so you bring all of these elements back into your job here at MSR in a way, because you’re saying, well, you have these different outlets for your art. But then you come here, and … what are some of the things that you’ve created over the last few years that you’re especially proud of?
STORY: Oh, phew … that would … Project Eclipse.
GEHRKE: Eclipse, uh-huh.
STORY: That’s the hyperlocal air-quality sensor.
GEHRKE: And this is actually something that was really deployed in cities …
STORY: Yes. It was deployed in Chicago …
GEHRKE: … so it had to be both aesthetically good and … to look nice, not only functional.
STORY: Well, it had not only … it had … first of all, I approached it from it has to be functional. But knowing that it was going to deploy, I had to design everything with a design for manufacturing method. So DFM—design for manufacturing—is from the ground up, I have to make sure that there are certain features as part of the design, and that is making sure I have draft angles because the idea is that eventually this is going to be a plastic-injected part.
GEHRKE: What is a draft angle?
STORY: A draft angle is so that a part can get pulled from a mold.
GEHRKE: OK …
STORY: If I build things with pure vertical walls, there’s too much even stress that the part will not actually extract from the mold. Every time you look at something that’s plastic injected, there’s something called the draft angle, where there’s actually a slight taper. It’s only 2 to 4 degrees, but it’s in there, and it needs to be in there; otherwise, you’re never going to get the part out of the mold. So I had to keep that in mind. So from the ground up, I had designed this thing—the end goal of this thing is for it to be reproduced in a production capacity. And so DFM was from day one. They came to me earlier, and they gave me a couple of parts that they had prototyped on a 3D printer. So I had to go through and actually re-engineer the entire design so that it would be able to hold the components, but …
GEHRKE: And to be waterproof and so on, right?
STORY: Well, waterproofing, that was another thing. We had a lot of iterations—and that was the other thing about research. Research is about iteration. It’s about failing and failing fast so that you can learn from it. Failure is not a four-lettered word. In research, we fail so that we use that as a steppingstone so that we can make discoveries and then succeed on that …
GEHRKE: We learn.
STORY: Yes, it’s a learning opportunity. As a matter of fact, the very first time we fail, I go to the whiteboard and write “FAIL” in big capital letters. It’s our very first one, and it’s our “First Attempt In Learning.” And that’s what I remember it as. It’s my big acronym. But it’s a great process. You know, we spin on a dime. Sometimes, we go, whoa, we went the wrong direction. But we learn from it, and it just makes us better.
GEHRKE: And sometimes you have to work under time pressure because, you know, there’s no …
STORY: There isn’t a single thing we don’t do in the world that isn’t under time pressure. Working in a restaurant … when I had to, as they say, grow my bones after culinary school, you work in a restaurant, and you gain that experience. And one of the …
GEHRKE: So in your sabbatical, you didn’t only go to culinary school; you actually worked in this restaurant, as well?
STORY: Oh, it’s required.
GEHRKE: It’s a requirement? OK.
STORY: Yeah, yeah, it’s a requirement that you understand, you familiarize yourself with the rigor. So one of the things we used to do is … there was a Denny’s next to LAX in Los Angeles. Because I was attending school in Pasadena. And I would go and sign up to be the fry cook at a Denny’s that doesn’t close. It’s 24 hours.
GEHRKE: Yup …
STORY: And these people would come in, these taxis would come in, and they need to eat, and they need to get in and out.
GEHRKE: As a student, I would go to Denny’s at absurd times …
STORY: Oh, my, it was like drinking from a fire hose. I was getting crushed every night. But after a while, you know, within two or three weeks, I was like a machine, you know. And it was just like, oh, that’s not a problem. Oh, I have five orders here of this. And I need to make sure those are separated from these orders. And you have this entire process, this organization that happens in the back of your mind, you know. And that’s part of it. I mean, every job I’ve ever had, there’s always going to be a time pressure.
GEHRKE: But it must be even more difficult in research because you’re not building like, you know, Denny’s, I think you can fry probably five or 10 different things. Whereas here, you know, everything is unique, and everything is different. And then you, you know, you learn and improve and fail.
STORY: Yes, yes. But, I mean, it’s … but it’s the same as dealing with customers. Everyone’s going to have a different need and a different … there’s something that everyone’s bringing unique to the table. And when I was working at Denny’s, you’re going to have the one person that’s going to make sure that, oh, they want something very, very specific on their order. It’s no different than I’m working with, you know, somebody I’m offering a service to in a research environment.
GEHRKE: Mm-hmm. Mm-hmm. That’s true. I hadn’t even thought about this. Next time when I go to a restaurant, I’ll be very careful with the special orders. [LAUGHTER]
STORY: That’s why I’m exceptionally kind to those people who work in restaurants because I’ve been on the other side of the line.
GEHRKE: So, so you have seen many sides, right? And you especially are working also across developers, PMs, researchers. How do you bridge all of these different gaps? Because all of these different disciplines come with a different history and different expectations, and you work across all of them.
STORY: There was something somebody said to me years ago, and he says, never be the smartest guy in the room. Because at that point, you stop learning. And I was very lucky enough to work with great people like Mike Sinclair, Bill Buxton—visionaries. And one of the things that was always impressed upon me was they really let you shine, and they stepped back, and then when you had your chance to shine, they would celebrate you. And when it was their time to shine, you step back and make sure that they overshined. So it’s being extremely receptive to every idea. There’s nothing, there’s no … what do they say? The only bad idea is the lack of …
GEHRKE: Not having any ideas …
STORY: … having any ideas.
GEHRKE: Right, right …
STORY: Yeah. So being extremely flexible, receptive, willing to try things that even though they are uncomfortable, that’s I think where people find the most success.
GEHRKE: That’s such great advice. Reflecting back on your super-interesting career and all the different things that you’ve seen and also always stretching the boundaries, what’s your advice for anybody to have a great career if somebody’s starting out or is even changing jobs?
STORY: Gee, that’s a tough one. Starting out or changing—I can tell you about how to change jobs. Changing jobs … strip yourself of your ego. Be willing to be the infant, but also be willing to know when you’re wrong, and be willing to have your mind changed. That’s about it.
GEHRKE: Such, such great advice.
STORY: Yeah.
GEHRKE: Thanks so much, Lex, for the great, great conversation.
STORY: Not a problem. You’re welcome.
[MUSIC]To learn more about Lex and to see pictures of him as a child or from his time in the Marines, visit aka.ms/ResearcherStories.
[MUSIC FADES]
The post What’s Your Story: Lex Story appeared first on Microsoft Research.