This post is co-written by Danilo Tommasina and Andrei Voinov from Thomson Reuters.
Thomson Reuters (TR) is one of the world’s most trusted information organizations for businesses and professionals. TR provides companies with the intelligence, technology, and human expertise they need to find trusted answers, enabling them to make better decisions more quickly. TR’s customers span the financial, risk, legal, tax, accounting, and media markets.
Thomson Reuters Labs (TR Labs) is the dedicated applied research division within TR. TR Labs is focused on the research, development, and application of artificial intelligence (AI) and emerging trends in technologies that can be infused into existing TR products or new offerings. TR Labs works collaboratively with various product teams to experiment, prototype, test, and deliver AI-powered innovation in pursuit of smarter and more valuable tools for our customers. The TR Labs team includes over 150 applied scientists, machine learning specialists, and machine learning engineers.
In this post, we explore how TR Labs was able to develop an efficient, flexible, and powerful MLOps process by adopting a standardized MLOps framework that uses AWS SageMaker, SageMaker Experiments, SageMaker Model Registry, and SageMaker Pipelines. The goal being to accelerate how quickly teams can experiment and innovate using AI and machine learning (ML)—whether using natural language processing (NLP), generative AI, or other techniques. We discuss how this has helped decrease the time to market for fresh ideas and helped build a cost-efficient machine learning lifecycle. Lastly, we will go through the MLOps toolchain that TR Labs built to standardize the MLOps process for developers, scientists, and engineers.
The challenge
Machine learning operations (MLOps) is the intersection of people, for gaining business value from machine learning. An MLOps practice is essential for an organization with large teams of ML engineers and data scientists. Correctly using AI/ML tools to increase productivity directly influences efficiency and cost of development. TR Labs was founded in 1992 with a vision to be a world-leading AI/ML research and development practice, forming the core innovation team that works alongside the tax, legal and news divisions of TR to ensure that their offerings remain at the cutting edge of their markets.
The TR Labs team started off as a small team in its early days, with a team directive to spearhead ML innovation to help the company in various domains including but not limited to text summarization, document categorization, and various other NLP tasks. The team made remarkable progress from an early stage with AI/ML models being integrated into TR’s products and internal editorial systems to help with efficiency and productivity.
However, as the company grew, so did the team’s size and task complexity. The team had grown to over 100 people, and they were facing new challenges. Model development and training processes were becoming increasingly complex and challenging to manage. The team had different members working on different use cases, and therefore, models. Each researcher also had their own way of developing the models. This led to a situation where there was little standardization in the process for model development. Each researcher needed to configure all the underlying resources manually, and a large amount of boilerplate code was created in parallel by different teams. A significant portion of time was spent on tasks that could be performed more efficiently.
The TR Labs leadership recognized that the existing MLOps process wasn’t scalable and needed to be standardized. It lacked sufficient automation and assistance for those new to the platform. The idea was to take well architected practices for ML model development and operations and create a customized workflow specific to Labs that uses Amazon Web Services (AWS). The vision was to harmonize and simplify the model development process and accelerate the pace of innovation. They also aimed to set the path to quickly mature research and development solutions into an operational state that would support a high degree of automation for monitoring and retraining.
In this post, we will focus on the MLOps process parts involved in the research and model development phases.
The overview section will take you through the innovative solution that TR Labs created and how it helped lower the barrier to entry while increasing the adoption of AI/ML services for new ML users on AWS while decreasing time to market for new projects.
Solution overview
The existing ML workflow required a TR user to start from scratch every time they started a new project. Research teams would have to familiarize themselves with the TR Labs standards and deploy and configure the entire MLOps toolchain manually with little automation in place. Inconsistent practices within the research community meant extra work was needed to align with production grade deployments. Many research projects had to be refactored when handing code over to MLOps engineers, who often had to reverse engineer to achieve a similar level of functionality to make the code ready to deploy to production. The team had to create an environment where researchers and engineers worked on one shared codebase and use the same toolchain, reducing the friction between experimentation and production stages. A shared codebase is also a key element for long term maintenance—changes to the existing system should be integrated directly in the production level code and not reverse engineered and re-merged out of a research repository into the production codebase. This is an anti-pattern that leads to large costs and risks over time.
Regardless of the chosen model architecture, or even if the chosen model is a third-party provider for large language models (LLMs) without any fine tuning, a robust ML system requires validation on a relevant dataset. There are multiple testing methods, such as zero-shot learning, a machine learning technique that allows a model to classify objects from previously unseen classes, without receiving any specific training for those classes, with a transition to later introduce fine tuning to improve the model’s performance. How many iterations are necessary to obtain the expected initial quality and maintain or even improve the level over time depends on the use case and the model type being developed. However, when thinking about long-term systems, teams go through tens or even hundreds of repetitions. These repetitions will contain several recurring steps such as pre-processing, training, and post processing, which are similar, if not the same, no matter which approach is taken. Repeating the process manually without following a harmonized approach is also an anti-pattern.
This process inefficiency presented an opportunity to create a coherent set of MLOps tools that would enforce TR Labs standards for how to configure and deploy SageMaker services and expose these MLOps capabilities to a user by providing standard configuration and boilerplate code. The initiative was named TR MLTools and joined several MLOps libraries developed in TR Labs under one umbrella. Under this umbrella, the team provided a command line interface (CLI) tool that would support a standard project structure and deliver boilerplate code abstracting the underlying infrastructure deployment process and promoting a standardized TR ML workflow.
MLTools and MLTools CLI were designed to be flexible and extendable while incorporating a TR Labs-opinionated view on how to run MLOps in line with TR enterprise cloud platform standards.
MLTools CLI
MLTools CLI is a Python package and a command-line tool that promotes the standardization of TR Labs ML experiments workflow (ML model development, training, and so on) by providing code and configuration templates directly into the users’ code repository. At its core, MLTools CLI aims to connect all ML experiment-related entities (Python scripts, Jupyter notebooks, configuration files, data, pipeline definitions, and so on) and provide an easy way to bootstrap new experiments, conduct trials, and run user-defined scripts, testing them locally and remotely running them at scale as SageMaker jobs.
MLTools CLI is added as a development dependency to a new or existing Python project, where code for the planned ML experiments will be developed and tracked, for example in GitHub. As part of an initial configuration step, this source-code project is associated with specific AI Platform Machine Learning Workspaces. The users can then start using the MLTools CLI for running their ML experiments using SageMaker capabilities like Processing and Training jobs, Experiments, Pipelines, and so on.
Note: AI Platform Workspaces is an internal service, developed in TR, that provides secure access to Amazon Simple Storage Service (Amazon S3)-hosted data and AWS resources like SageMaker or SageMaker Studio Notebook instances for our ML researchers. You can find more information about the AI Platform Workspaces in this AWS blog: How Thomson Reuters built an AI platform using Amazon SageMaker to accelerate delivery of ML projects.
MLTools CLI acts effectively as a frontend or as a delivery channel for the set of capabilities (libraries, tools, and templates) that TR collectively refers to as MLTools. The following diagram shows a typical TR Labs ML experiments workflow, with a focus on the role of MLTools and MLTools CLI:
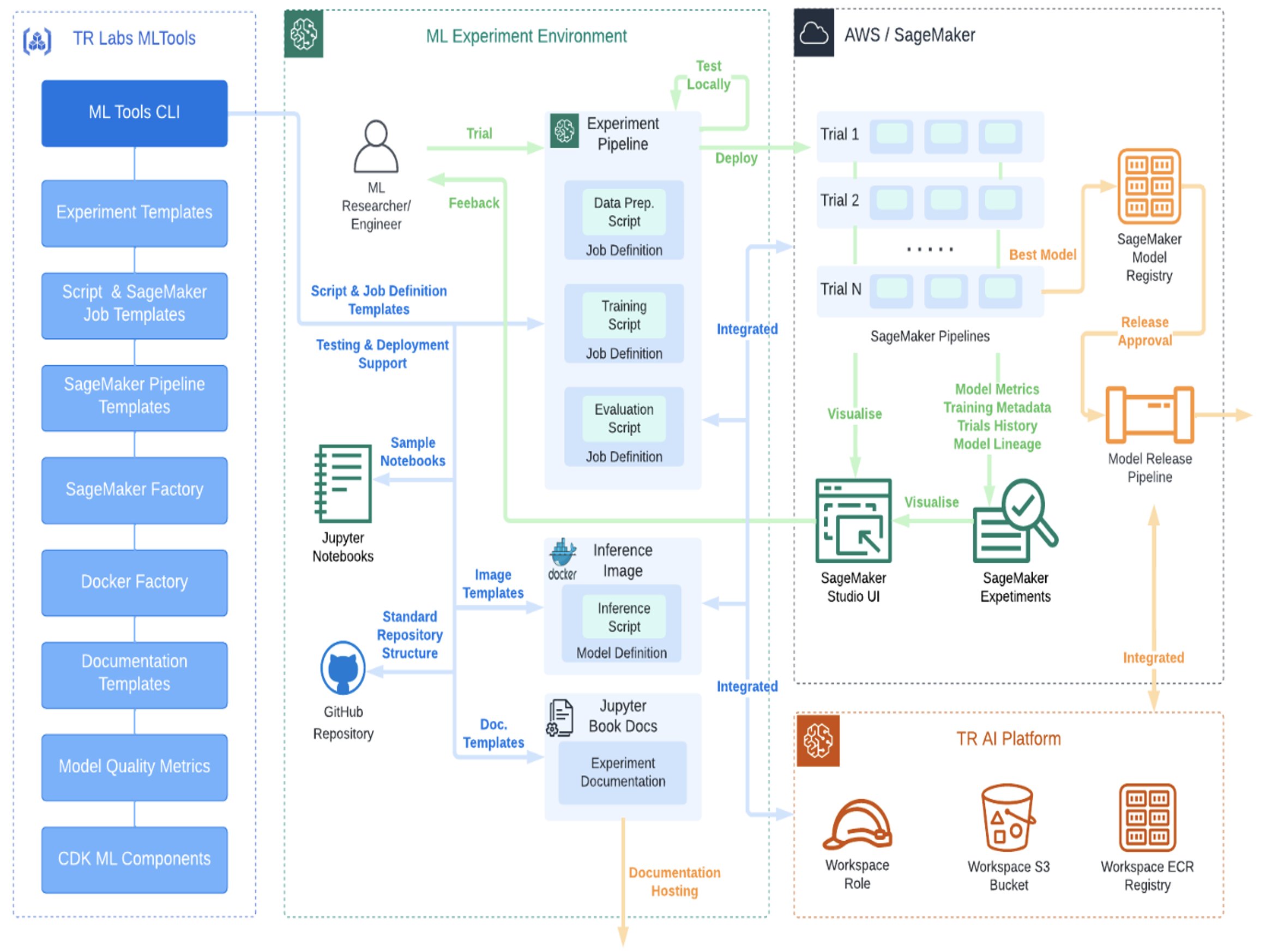
MLTools CLI offers various templates that can be generated using a command-line, including the following:
- Separate directory structure for new ML experiments and experiment trials.
- Script templates for launching SageMaker processing, training, and batch transform jobs.
- Complete experiment pipeline template based on SageMaker Pipeline, with user scripts as steps.
- Docker image templates for packaging user scripts. For example, for delivery to production.
MLTools CLI also provides the following features to support effective ML experiments:
- User scripts can be run directly as SageMaker jobs without the need to build Docker images.
- Each experiment runs in a sandboxed Poetry environment and can have its own code package and dependency tree.
- The main, project-level code package is shared and can be used by all project experiments and user scripts code, allowing re-use of common code with no copy-paste.
- Context-aware API resolves and loads experiment and trial metadata based on the current working directory.
- Created AWS resources are automatically tagged with the experiment metadata.
- Utilities to query these experiment-related AWS resources are available.
ML experiment workflow
After MLTools CLI is installed and configured on a laptop or notebook instance, a user can begin ML experimentation work. The first step is to create a new experiment using the MLTools CLI create-experiment
command:
> mltools-cli create-experiment –experiment-name my-demo-experiment
An experiment template is generated in a sub-directory of the user’s project. The generated experiment folder has a standard structure, including the initial experiment’s configuration, a sandboxed Poetry package, and sample Jupyter notebooks to help quickly bootstrap new ML experiments:
experiments
└── my_demo_experiment
├── data
├── notebooks
├── scripts
├── src
│ └── my_demo_experiment
│ └── __init__.py
├── config.yaml
├── poetry.toml
├── pyproject.toml
├── README.md
└── setup.sh
The user can then create script templates for the planned ML experiment steps:
> cd experiments/my_demo_experiments
> mltools-cli create-script –script-name preprocess --job-config PROCESS
> mltools-cli create-script –script-name train --job-config TRAIN
> mltools-cli create-script –script-name evaluate --job-config INFERENCE
Generated script templates are placed under the experiment directory:
experiments
└── my_demo_experiment
├── ...
└── scripts
├── evaluate
│ ├── evaluate.py
│ ├── evaluate_job.py
│ └── requirements.txt
├── preprocess
│ ├── preprocess.py
│ ├── preprocess_job.py
│ └── requirements.txt
└── train
├── train.py
├── train_job.py
└── requirements.txt
Script names should be short and unique within their parent experiment, because they’re used to generate standardized AWS resource names. Script templates are supplemented by a job configuration for a specific type of job, as specified by the user. Templates and configurations for SageMaker processing, training, and batch transform jobs are currently supported by MLTools—these offerings will be expanded in the future. A requirements.txt
file is also included where users can add any dependencies required by the script code to be automatically installed by SageMaker at runtime. The script’s parent experiment and project packages are added to the requirements.txt
by default, so the user can import and run code from the whole project hierarchy.
The user would then proceed to add or adapt code in the generated script templates. Experiment scripts are ordinary Python scripts that contain common boilerplate code to give users a head start. They can be run locally while adapting and debugging the code. After the code is working, the same scripts can be launched directly as SageMaker jobs. The required SageMaker job configuration is defined separately in a <script_name>_job.py
file, and job configuration details are largely abstracted from the notebook experiment code. As a result, an experiment script can be launched as a SageMaker job with a few lines of code:
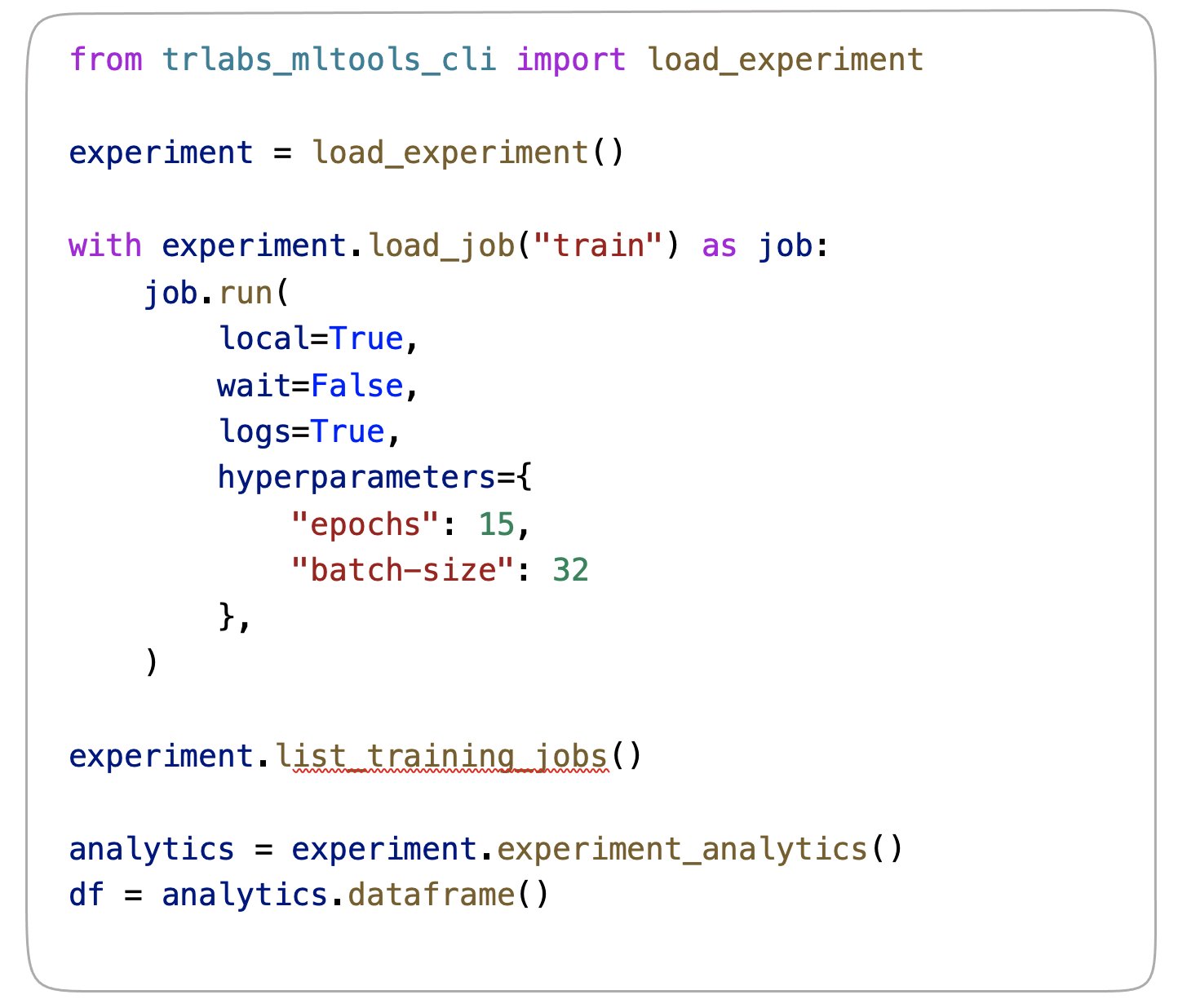
Let’s explore the previous code snippet in detail.
First, the MLTools experiment context is loaded based on the current working directory using the load_experiment()
factory method. The experiment context concept is a central point of the MLTools API. It provides access to the experiment’s user configuration, the experiment’s scripts, and the job configuration. All project experiments are also integrated with the project-linked AI Platform workspace and therefore have access to the resources and metadata of this workspace. For example, the experiments can access the workspace AWS Identity and Access Management (IAM) role, S3 bucket and its default Amazon Elastic Container Registry (Amazon ECR) repository.
From the experiment, a job context can be loaded, providing one of the experiment’s script names—load_job("train")
in this instance. During this operation, the job configuration is loaded from the script’s <script_name>_job.py
module. Also, if the script code depends on the experiment or the project packages, they’re automatically built (as Python wheels) and pre-packaged together with the script code, ready to be uploaded to S3.
Next, the training script is launched as a SageMaker training job. In the background, the MLTools factory code ensures that the respective SageMaker estimator or processor instances are created with the default configuration and conform to the rules and best practices accepted in TR. This includes naming conventions, virtual private cloud (VPC) and security configurations, and tagging. Note that SageMaker local mode is fully supported (set in the example by local=True
) while its specific configuration details are abstracted from the code. Although the externalized job configuration provides all the defaults, these can be overwritten by the user. In the previous example, custom hyperparameters are provided.
SageMaker jobs that were launched as part of an experiment can be listed directly from the notebook using the experiment’s list_training_jobs()
and list_processing_jobs()
utilities. SageMaker ExperimentAnalytics data is also available for analysis and can be retrieved by calling the experiment’s experiment_analytics()
method.
Integration with SageMaker Experiments
For every new MLTools experiment, a corresponding entity is automatically created in SageMaker Experiments. Experiment names in SageMaker are standardized and made unique by adding a prefix that includes the associated workspace ID and the root commit hash of the user repository. For any job launched from within an MLTools experiment context (that is by using job.run()
as shown in the preceding code snippet), a SageMaker Experiments Run instance is created and the job is automatically launched within the SageMaker Experiments Run context. This means all MLTools job runs are automatically tracked in SageMaker Experiments, ensuring that all job run metadata is recorded. This also means that users can then browse their experiments and runs directly in the experiments browser in SageMaker Studio, create visualizations for analysis, and compare model metrics, among other tasks.
As shown in the following diagram, the MLTools experiment workflow is fully integrated with SageMaker Experiments:
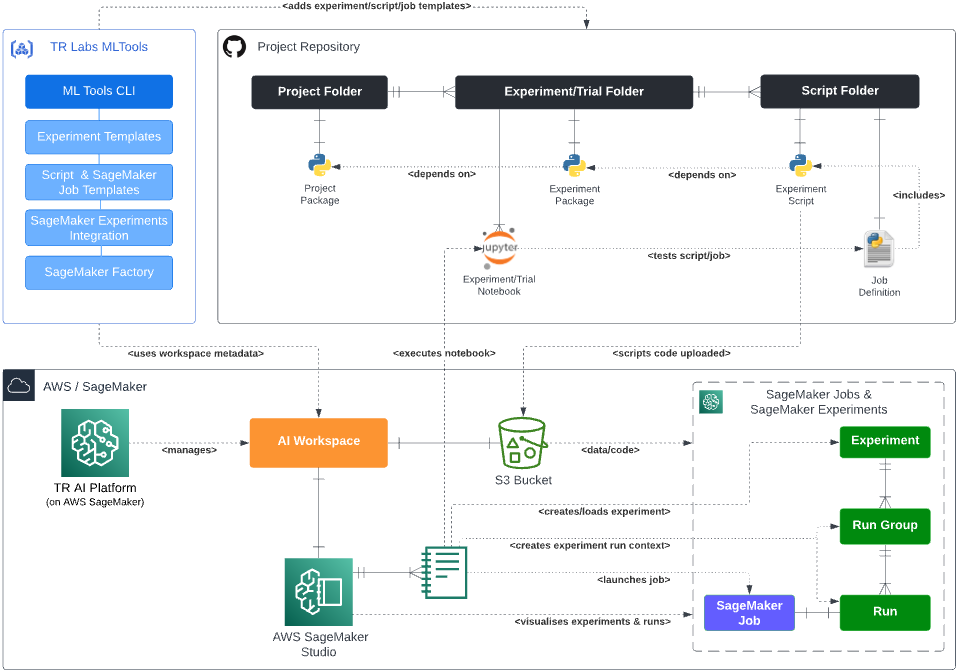
Integration with SageMaker Pipelines
Some of the important factors that make ML experiments scalable are their reproducibility and their operationalization level. To support this, MLTools CLI provides users with a capability to add a template with boilerplate code to link the steps of their ML experiment into a deployable workflow (pipeline) that can be automated and delivers reproducible results. The MLTools experiment pipeline implementation is based on AWS SageMaker Pipelines. The same experiment scripts that might have been run and tested as standalone SageMaker jobs can naturally form the experiment pipeline steps.
MLTools currently offers the following standard experiment pipeline template:
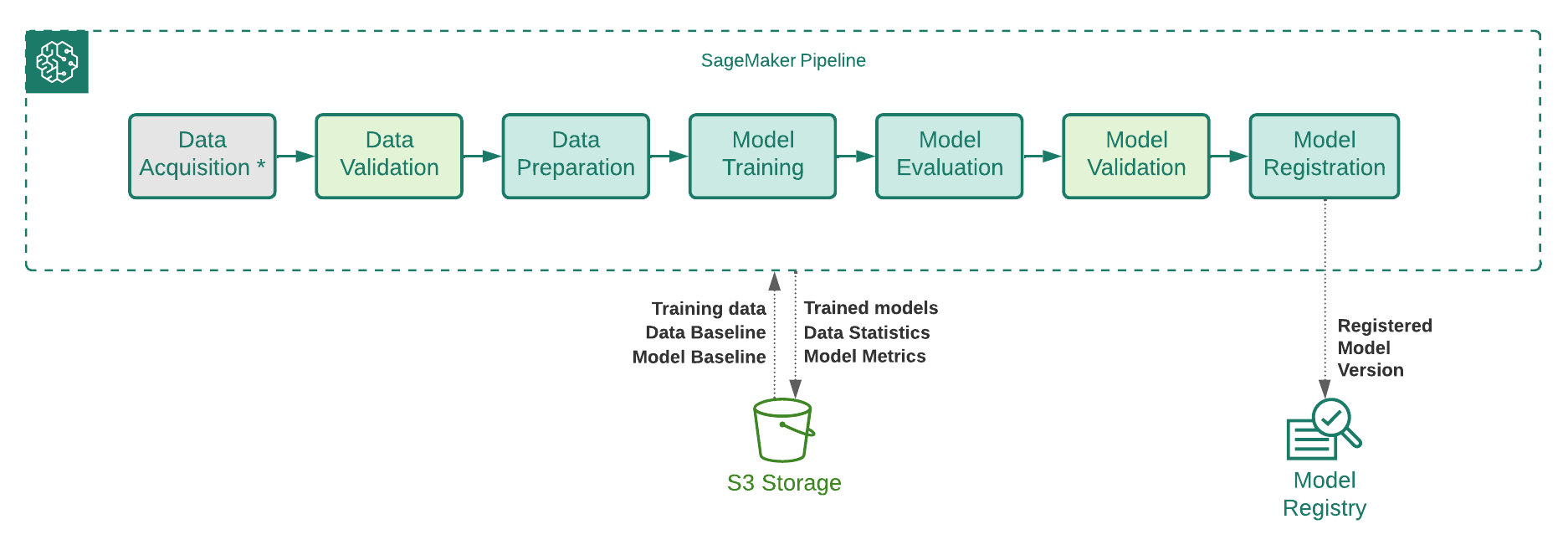
We made a deliberate design decision to offer a simple, linear, single-model experiment pipeline template with well-defined standard steps. Oftentimes our project work on multi-model solutions involved an ensemble of ML models that might be ultimately trained on the same set of training data. In such cases, pipelines with more complex flows, or even integrated multi-model experiment pipelines, can be perceived as more efficient. Nevertheless, from a reproducibility and standardization standpoint, a decision to develop a customized experiment pipeline would need to be justified and is generally better suited for the later stages of ML operations where efficient model deployment might be a factor.
On the other hand, using the standard MLTools experiment pipeline template, users can create and start running their experiment pipelines in the early stages of their ML experiments. The underlying pipeline template implementation allows users to easily configure and deploy partial pipelines where only some of the defined steps are implemented. For example, a user can start with a pipeline that only has a single step implemented, such as a DataPreparation
step, then add ModelTraining
and ModelEvaluation
steps and so on. This approach aligns well with the iterative nature of ML experiments and allows for gradually creating a complete experiment pipeline as the ML experiment itself matures.
As shown in the following diagram, MLTools allows users to deploy and run their complete experiment pipelines based on SageMaker Pipelines integrated with SageMaker Model Registry and SageMaker Studio.
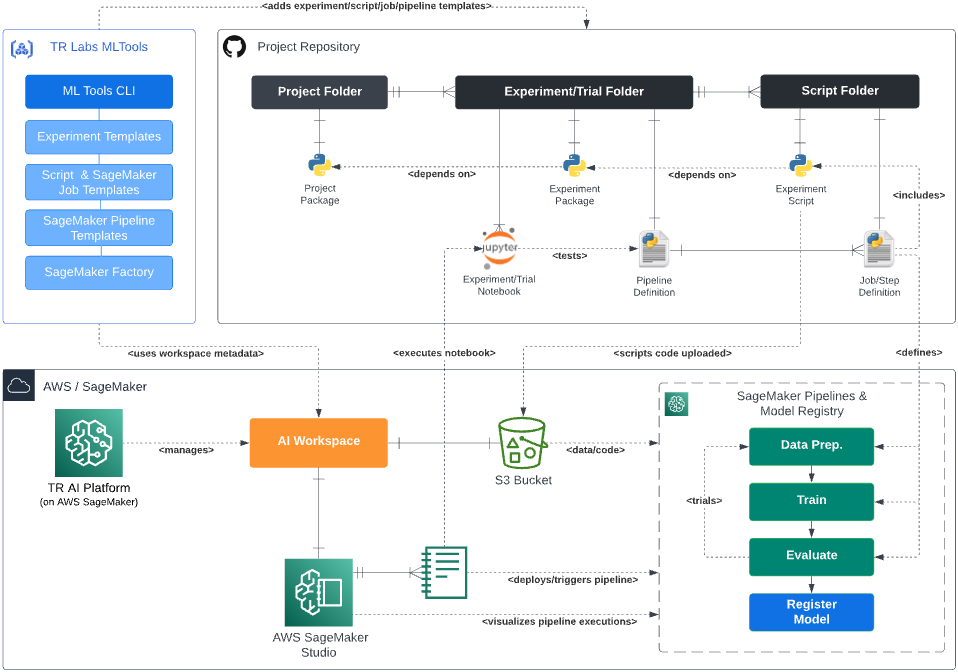
Results and future improvements
TR Labs’s successful creation of the MLTools toolchain helps to standardize the MLOps framework throughout the organization and provides several benefits—the first of these is faster model development times. With a consistent process, team members can now work more efficiently by using project templates that deliver a modular setup, facilitating all phases of the ML development process. The structure delivers out-of-the-box integration with TR’s AWS-based AI Platform and the ability to switch between phases of the development including research and data analysis, running experiments at scale, and delivering end-to-end ML pipeline automation. This allows the team to focus on the critical aspects of model development while technicalities are handled and provisioned in advance.
The toolchain is designed to support a close collaboration between researchers and engineers who can work on different aspects of an ML delivery while sharing a codebase that follows software development best practices.
By following a standardized MLOps process, the TR Labs team can also quickly identify issues and model performance drifts more efficiently. It becomes easier to pinpoint where errors are occurring and how to fix them. This can help to reduce downtime and improve the overall efficiency of the development and maintenance processes. The standardized process also ensures that researchers working in model development are using the same environment as ML engineers. This leads to a more efficient transition from ideation and development to deploying the output as models in production and entering the maintenance phase.
Standardizing the MLOps platform has also led to cost savings through efficiencies. With a defined process, the team can reduce the time and resources required to develop and deploy models. This leads to cost savings in the long run, making the development, and particularly the long-term maintenance processes, more cost-effective.
A difficulty the team observed was in measuring how much the toolchain improved time to market and reduced costs. Thoroughly evaluating this would require a dedicated study where independent teams would work on the same use cases with and without the toolchain and comparing the results. However, there are subjective components and possibly different approaches that you can take to resolve this question. Such an approach would be very costly and still contain a high degree of imprecision.
The TR Labs team found an alternate solution for how to measure success. At a yearly interval we run an assessment with the userbase of the toolchain. The assessment covers a variety of aspects ranging over the entire AI/ML lifecycle. Toolchain users are asked to provide subjective assessments on how much of their development time is considered “wasted” on infrastructure issues, configuration issues, or manual tasks that are repetitive. Other questions cover the level of satisfaction with the current toolchain and the perceived improvement in productivity comparing current and past work without the toolchain or earlier versions of the toolchain. The resulting values are averaged over the entire userbase, which includes a mix of job roles ranging from engineers to data scientists to researchers.
The reduction of time spent on inefficiencies, the increase in perceived productivity, and user satisfaction can be used to compute the approximate monetary savings, improvement in code quality, and reduction in time to market. These combined factors contribute to user satisfaction and improvement in the retention of talent within the ML community at TR.
As a measure of success, the TR Labs team was able to achieve reductions in accumulated time spent on inefficiencies and found that this ranges between 3 to 5 days per month per person. Measuring the impact over a period of 12 months, TR has seen improvements of up to 40 percent in perceived productivity in several areas of the lifecycle and a measurable increase in user satisfaction. These numbers are based on what the users of the toolchain reported in the self-assessments.
Conclusion
A standardized MLOps framework can lead to the reduction of bugs, faster model development times, faster troubleshooting of issues, faster reaction to model performance drifts, and cost savings gained through a more efficient end-to-end machine learning process that facilitates experimentation and model creating at scale. By adopting a standardized MLOps framework that uses AWS SageMaker, SageMaker Experiments, SageMaker Model Registry, and SageMaker Pipelines, TR Labs was able to ensure that their machine learning models were developed and deployed efficiently and effectively. This has resulted in a faster time to market and accelerated business value through development.
To learn more about how AWS can help you with your AI/ML and MLOps journey, see What is Amazon SageMaker.
About the Authors
Andrei Voinov is a Lead Software Engineer at Thomson Reuters (TR). He is currently leading a team of engineers in TR Labs with the mandate to develop and support capabilities that help researchers and engineers in TR to efficiently transition ML projects from inception, through research, integration, and delivery into production. He brings over 25 years of experience with software engineering in various sectors and extended knowledge both in the cloud and ML spaces.
Danilo Tommasina is a Distinguished Engineer at Thomson Reuters (TR). With over 20 years of experience working in technology roles ranging from Software Engineer, over Director of Engineering and now as Distinguished Engineer. As a passionate generalist, proficient in multiple programming languages, cloud technologies, DevOps practices and with engineering knowledge in the ML space, he contributed to the scaling of TR Labs’ engineering organization. He is also a big fan of automation including but not limited to MLOps processes and Infrastructure as Code principles.
Simone Zucchet is a Manager of Solutions Architecture at AWS. With close to a decade of experience as a Cloud Architect, Simone enjoys working on innovative projects that help transform the way organizations approach business problems. He helps support large enterprise customers at AWS and is part of the Machine Learning TFC. Outside of his professional life, he enjoys working on cars and photography.
Jeremy Bartosiewicz is a Senior Solutions Architect at AWS. With over 15 years of experience working in technology in multiple roles. Coming from a consulting background, Jeremy enjoys working on a multitude of projects that help organizations grow using cloud solutions. He helps support large enterprise customers at AWS and is part of the Advertising and Machine Learning TFCs.
Read More