The latest C2PA provenance technology aims to help people better understand how a particular piece of content was created and modified over time.Read More
CRISPR-Cas9 guide RNA efficiency prediction with efficiently tuned models in Amazon SageMaker
The clustered regularly interspaced short palindromic repeat (CRISPR) technology holds the promise to revolutionize gene editing technologies, which is transformative to the way we understand and treat diseases. This technique is based in a natural mechanism found in bacteria that allows a protein coupled to a single guide RNA (gRNA) strand to locate and make cuts in specific sites in the targeted genome. Being able to computationally predict the efficiency and specificity of gRNA is central to the success of gene editing.
Transcribed from DNA sequences, RNA is an important type of biological sequence of ribonucleotides (A, U, G, C), which folds into 3D structure. Benefiting from recent advance in large language models (LLMs), a variety of computational biology tasks can be solved by fine-tuning biological LLMs pre-trained on billions of known biological sequences. The downstream tasks on RNAs are relatively understudied.
In this post, we adopt a pre-trained genomic LLMs for gRNA efficiency prediction. The idea is to treat a computer designed gRNA as a sentence, and fine-tune the LLM to perform sentence-level regression tasks analogous to sentiment analysis. We used Parameter-Efficient Fine-Tuning methods to reduce the number of parameters and GPU usage for this task.
Solution overview
Large language models (LLMs) have gained a lot of interest for their ability to encode syntax and semantics of natural languages. The neural architecture behind LLMs are transformers, which are comprised of attention-based encoder-decoder blocks that generate an internal representation of the data they are trained from (encoder) and are able to generate sequences in the same latent space that resemble the original data (decoder). Due to their success in natural language, recent works have explored the use of LLMs for molecular biology information, which is sequential in nature.
DNABERT is a pre-trained transformer model with non-overlapping human DNA sequence data. The backbone is a BERT architecture made up of 12 encoding layers. The authors of this model report that DNABERT is able to capture a good feature representation of the human genome that enables state-of-the-art performance on downstream tasks like promoter prediction and splice/binding site identification. We decided to use this model as the foundation for our experiments.
Despite the success and popular adoption of LLMs, fine-tuning these models can be difficult because of the number of parameters and computation necessary for it. For this reason, Parameter-Efficient Fine-Tuning (PEFT) methods have been developed. In this post, we use one of these methods, called LoRA (Low-Rank Adaptation). We introduce the method in the following sections.
The following diagram is a representation of the Cas9 DNA target mechanism. The gRNA is the component that helps target the cleavage site.
The goal of this solution is to fine-tune a base DNABERT model to predict activity efficiency from different gRNA candidates. As such, our solution first takes gRNA data and processes it, as described later in this post. Then we use an Amazon SageMaker notebook and the Hugging Face PEFT library to fine-tune the DNABERT model with the processed RNA data. The label we want to predict is the efficiency score as it was calculated in experimental conditions testing with the actual RNA sequences in cell cultures. Those scores describe a balance between being able to edit the genome and not damage DNA that wasn’t targeted.
The following diagram illustrates the workflow of the proposed solution.
Prerequisites
For this solution, you need access to the following:
- A SageMaker notebook instance (we trained the model on an ml.g4dn.8xlarge instance with a single NVIDIA T4 GPU)
- transformers-4.34.1
- peft-0.5.0
- DNABERT 6
Dataset
For this post, we use the gRNA data released by researchers in a paper about gRNA prediction using deep learning. This dataset contains efficiency scores calculated for different gRNAs. In this section, we describe the process we followed to create the training and evaluation datasets for this task.
To train the model, you need a 30-mer gRNA sequence and efficiency score. A k-mer is a contiguous sequence of k nucleotide bases extracted from a longer DNA or RNA sequence. For example, if you have the DNA sequence “ATCGATCG” and you choose k = 3, then the k-mers within this sequence would be “ATC,” “TCG,” “CGA,” “GAT,” and “ATC.”
Efficiency score
Start with excel file 41467_2021_23576_MOESM4_ESM.xlsx from the CRISPRon paper in the Supplementary Data 1 section. In this file, the authors released the gRNA (20-mer) sequences and corresponding total_indel_eff
scores. We specifically used the data from the sheet named spCas9_eff_D10+dox. We use the total_indel_eff
column as the efficiency score.
Training and validation data
Given the 20-mers and the crispron scores (same as the total_indel_eff
scores) from earlier, complete the following steps to put together the training and validation data:
- Convert the sequences in the sheet “TRAP12K microarray oligos” into an .fa (fasta) file.
- Run the script
get_30mers_from_fa.py
(from the CRISPRon GitHub repository) to obtain all possible 23-mers and 30-mers from the sequences obtained from Step 1. - Use the
CRISPRspec_CRISPRoff_pipeline.py
script (from the CRISPRon GitHub repository) to obtain the binding energy for the 23-mers obtained from Step 2. For more details on how to run this script, check out the code released by the authors of the CRISPRon paper(check the scriptCRISPRon.sh
). - At this point, we have 23-mers along with the corresponding binding energy scores, and 20-mers along with the corresponding CRISPRon scores. Additionally, we have the 30-mers from Step 2.
- Use the script
prepare_train_dev_data.py
(from our released code) to create training and validation splits. Running this script will create two files:train.csv
anddev.csv
.
The data looks something like the following:
Model architecture for gRNA encoding
To encode the gRNA sequence, we used the DNABERT encoder. DNABERT was pre-trained on human genomic data, so it’s a good model to encode gRNA sequences. DNABERT tokenizes the nucleotide sequence into overlapping k-mers, and each k-mer serves as a word in the DNABERT model’s vocabulary. The gRNA sequence is broken into a sequence of k-mers, and then each k-mer is replaced by an embedding for the k-mer at the input layer. Otherwise, the architecture of DNABERT is similar to that of BERT. After we encode the gRNA, we use the representation of the [CLS] token as the final encoding of the gRNA sequence. To predict the efficiency score, we use an additional regression layer. The MSE loss will be the training objective. The following is a code snippet of the DNABertForSequenceClassification
model:
Fine-tuning and prompting genomic LLMs
Fine-tuning all the parameters of a model is expensive because the pre-trained model becomes much larger. LoRA is an innovative technique developed to address the challenge of fine-tuning extremely large language models. LoRA offers a solution by suggesting that the pre-trained model’s weights remain fixed while introducing trainable layers (referred to as rank-decomposition matrices) within each transformer block. This approach significantly reduces the number of parameters that need to be trained and lowers the GPU memory requirements, because most model weights don’t require gradient computations.
Therefore, we adopted LoRA as a PEFT method on the DNABERT model. LoRA is implemented in the Hugging Face PEFT library. When using PEFT to train a model with LoRA, the hyperparameters of the low rank adaptation process and the way to wrap base transformers models can be defined as follows:
Hold-out evaluation performances
We use RMSE, MSE, and MAE as evaluation metrics, and we tested with rank 8 and 16. Furthermore, we implemented a simple fine-tuning method, which is simply adding several dense layers after the DNABERT embeddings. The following table summarizes the results.
Method | RMSE | MSE | MAE |
LoRA (rank = 8) | 11.933 | 142.397 | 7.014 |
LoRA (rank = 16) | 13.039 | 170.01 | 7.157 |
One dense layer | 15.435 | 238.265 | 9.351 |
Three dense layer | 15.435 | 238.241 | 9.505 |
CRISPRon | 11.788 | 138.971 | 7.134 |
When rank=8, we have 296,450 trainable parameters, which is about 33% trainable of the whole. The performance metrics are “rmse”: 11.933, “mse”: 142.397, “mae”: 7.014.
When rank=16, we have 591,362 trainable parameters, which is about 66% trainable of the whole. The performance metrics are “rmse”: 13.039, “mse”: 170.010, “mae”: 7.157. There might have some overfitting issue here under this setting.
We also compare what happens when adding a few dense layers:
- After adding one dense layer, we have “rmse”: 15.435, “mse”: 238.265, “mae”: 9.351
- After adding three dense layers, we have “rmse”: 15.435, “mse”: 238.241, “mae”: 9.505
Lastly, we compare with the existing CRISPRon method. CRISPRon is a CNN based deep learning model. The performance metrics are “rmse”: 11.788, “mse”: 138.971, “mae”: 7.134.
As expected, LoRA is doing much better than simply adding a few dense layers. Although the performance of LoRA is a bit worse than CRISPRon, with thorough hyperparameter search, it is likely to outperform CRISPRon.
When using SageMaker notebooks, you have the flexibility to save the work and data produced during the training, turn off the instance, and turn it back on when you’re ready to continue the work, without losing any artifacts. Turning off the instance will keep you from incurring costs on compute you’re not using. We highly recommend only turning it on when you’re actively using it.
Conclusion
In this post, we showed how to use PEFT methods for fine-tuning DNA language models using SageMaker. We focused on predicting efficiency of CRISPR-Cas9 RNA sequences for their impact in current gene-editing technologies. We also provided code that can help you jumpstart your biology applications in AWS.
To learn more about the healthcare and life science space, refer to Run AlphaFold v2.0 on Amazon EC2 or fine-tuning Fine-tune and deploy the ProtBERT model for protein classification using Amazon SageMaker.
About the Authors
Siddharth Varia is an applied scientist in AWS Bedrock. He is broadly interested in natural language processing and has contributed to AWS products such as Amazon Comprehend. Outside of work, he enjoys exploring new places and reading. He got interested in this project after reading the book The Code Breaker.
Yudi Zhang is an Applied Scientist at AWS marketing. Her research interests are in the area of graph neural networks, natural language processing, and statistics.
Erika Pelaez Coyotl is a Sr Applied Scientist in Amazon Bedrock, where she’s currently helping develop the Amazon Titan large language model. Her background is in biomedical science, and she has helped several customers develop ML models in this vertical.
Zichen Wang is a Sr Applied Scientist in AWS AI Research & Education. He is interested in researching graph neural networks and applying AI to accelerate scientific discovery, specifically on molecules and simulations.
Rishita Anubhai is a Principal Applied Scientist in Amazon Bedrock. She has deep expertise in natural language processing and has contributed to AWS projects like Amazon Comprehend, Machine Learning Solutions Lab, and development of Amazon Titan models. She’s keenly interested in using machine learning research, specifically deep learning, to create tangible impact.
Improve RAG performance using Cohere Rerank
This post is co-written with Pradeep Prabhakaran from Cohere.
Retrieval Augmented Generation (RAG) is a powerful technique that can help enterprises develop generative artificial intelligence (AI) apps that integrate real-time data and enable rich, interactive conversations using proprietary data.
RAG allows these AI applications to tap into external, reliable sources of domain-specific knowledge, enriching the context for the language model as it answers user queries. However, the reliability and accuracy of the responses hinges on finding the right source materials. Therefore, honing the search process in RAG is crucial to boosting the trustworthiness of the generated responses.
RAG systems are important tools for building search and retrieval systems, but they often fall short of expectations due to suboptimal retrieval steps. This can be enhanced using a rerank step to improve search quality.
RAG is an approach that combines information retrieval techniques with natural language processing (NLP) to enhance the performance of text generation or language modeling tasks. This method involves retrieving relevant information from a large corpus of text data and using it to augment the generation process. The key idea is to incorporate external knowledge or context into the model to improve the accuracy, diversity, and relevance of the generated responses.
Workflow of RAG Orchestration
The RAG orchestration generally consists of two steps:
- Retrieval – RAG fetches relevant documents from an external data source using the generated search queries. When presented with the search queries, the RAG-based application searches the data source for relevant documents or passages.
- Grounded generation – Using the retrieved documents or passages, the generation model creates educated answers with inline citations using the fetched documents.
The following diagram shows the RAG workflow.
Document retrieval in RAG orchestration
One technique for retrieving documents in a RAG orchestration is dense retrieval, which is an approach to information retrieval that aims to understand the semantic meaning and intent behind user queries. Dense retrieval finds the closest documents to a user query in the embedding, as shown in the following screenshot.
The goal of dense retrieval is to map both the user queries and documents (or passages) into a dense vector space. In this space, the similarity between the query and document vectors can be computed using standard distance metrics like cosine similarity or euclidean distance. The documents that match closest to the semantic meaning of the user query based on the calculated distance metrics are then presented back to the user.
The quality of the final responses to search queries is significantly influenced by the relevance of the retrieved documents. While dense retrieval models are very efficient and can scale to large datasets, they struggle with more complex data and questions due to the simplicity of the method. Document vectors contain the meaning of text in a compressed representation—typically 786-1536 dimension vectors. This often results in loss of information because information is compressed into a single vector. When documents are retrieved during a vector search the most relevant information is not always presented at the top of the retrieval.
Boost search accuracy with Cohere Rerank
To address the challenges with accuracy, search engineers have used two-stage retrieval as a means of increasing search quality. In these two-stage systems, a first-stage model (an embedding model or retriever) retrieves a set of candidate documents from a larger dataset. Then, a second-stage model (the reranker) is used to rerank those documents retrieved by the first-stage model.
A reranking model, such as Cohere Rerank, is a type of model that will output a similarity score when given a query and document pair. This score can be used to reorder the documents that are most relevant to the search query. Among the reranking methodologies, the Cohere Rerank model stands out for its ability to significantly enhance search accuracy. The model diverges from traditional embedding models by employing deep learning to evaluate the alignment between each document and the query directly. Cohere Rerank outputs a relevance score by processing the query and document in tandem, which results in a more nuanced document selection process.
In the following example, the application was presented with a query: “When was the transformer paper coauthored by Aidan Gomez published?” The top-k with k = 6 returned the results shown in the image, in which the retrieved result set did contain the most accurate result, although it was at the bottom of the list. With k = 3, the most relevant document would not be included in the retrieved results.
Cohere Rerank aims to reassess and reorder the relevance of the retrieved documents based on additional criteria, such as semantic content, user intent, and contextual relevance, to output a similarity score. This score is then used to reorder the documents by relevance of the query. The following image shows reorder results using Rerank.
By applying Cohere Rerank after the first-stage retrieval, the RAG orchestration can gain the benefits of both approaches. While first-stage retrieval helps to capture relevant items based on proximity matches within the vector space, reranking helps optimize search according to results by guaranteeing contextually relevant results are surfaced to the top. The following diagram demonstrates this improved efficiency.
The latest version of Cohere Rerank, Rerank 3, is purpose-built to enhance enterprise search and RAG systems. Rerank 3 offers state-of-the-art capabilities for enterprise search, including:
- 4k context length to significantly improve search quality for longer documents
- Ability to search over multi-aspect and semi-structured data (such as emails, invoices, JSON documents, code, and tables)
- Multilingual coverage of more than 100 languages
- Improved latency and lower total cost of ownership (TCO)
The endpoint takes in a query and a list of documents, and it produces an ordered array with each document assigned a relevance score. This provides a powerful semantic boost to the search quality of any keyword or vector search system without requiring any overhaul or replacement.
Developers and businesses can access Rerank on Cohere’s hosted API and on Amazon SageMaker. This post offers a step-by-step walkthrough of consuming Cohere Rerank on Amazon SageMaker.
Solution overview
This solution follows these high-level steps:
- Subscribe to the model package
- Create an endpoint and perform real-time inference
Prerequisites
For this walkthrough, you must have the following prerequisites:
- The cohere-aws notebook.
This is a reference notebook, and it cannot run unless you make changes suggested in the notebook. It contains elements that render correctly in the Jupyter interface, so you need to open it from an Amazon SageMaker notebook instance or in Amazon SageMaker Studio.
- An AWS Identity and Access Management (IAM) role with the AmazonSageMakerFullAccess policy attached. To deploy this machine learning (ML) model successfully, choose one of the following options:
- If your AWS account does not have a subscription to Cohere Rerank 3 Model – Multilingual, your IAM role needs to have the following three permissions, and you need to have the authority to make AWS Marketplace subscriptions in the AWS account used:
aws-marketplace:ViewSubscriptions
aws-marketplace:Unsubscribe
aws-marketplace:Subscribe
- If your AWS account has a subscription to Cohere Rerank 3 Model – Multilingual, you can skip the instructions for subscribing to the model package.
- If your AWS account does not have a subscription to Cohere Rerank 3 Model – Multilingual, your IAM role needs to have the following three permissions, and you need to have the authority to make AWS Marketplace subscriptions in the AWS account used:
Refrain from using full access in production environments. Security best practice is to opt for the principle of least privilege.
Implement Rerank 3 on Amazon SageMaker
To improve RAG performance using Cohere Rerank, use the instructions in the following sections.
Subscribe to the model package
To subscribe to the model package, follow these steps:
- In AWS Marketplace, open the model package listing page Cohere Rerank 3 Model – Multilingual
- Choose Continue to Subscribe.
- On the Subscribe to this software page, review the End User License Agreement (EULA), pricing, and support terms and choose Accept Offer.
- Choose Continue to configuration and then choose a Region. You will see a Product ARN displayed, as shown in the following screenshot. This is the model package Amazon Resource Name (ARN) that you need to specify while creating a deployable model using Boto3. Copy the ARN corresponding to your Region and enter it in the following cell.
The code snippets included in this post are sourced from the aws-cohere notebook. If you encounter any issues with this code, refer to the notebook for the most up-to-date version.
!pip install --upgrade cohere-aws
# if you upgrade the package, you need to restart the kernel
from cohere_aws import Client
import boto3
On the Configure for AWS CloudFormation page shown in the following screenshot, under Product Arn, make a note of the last part of the product ARN to use as the value in the variable cohere_package
in the following code.
cohere_package = " cohere-rerank-multilingual-v3--13dba038aab73b11b3f0b17fbdb48ea0"
model_package_map = {
"us-east-1": f"arn:aws:sagemaker:us-east-1:865070037744:model-package/{cohere_package}",
"us-east-2": f"arn:aws:sagemaker:us-east-2:057799348421:model-package/{cohere_package}",
"us-west-1": f"arn:aws:sagemaker:us-west-1:382657785993:model-package/{cohere_package}",
"us-west-2": f"arn:aws:sagemaker:us-west-2:594846645681:model-package/{cohere_package}",
"ca-central-1": f"arn:aws:sagemaker:ca-central-1:470592106596:model-package/{cohere_package}",
"eu-central-1": f"arn:aws:sagemaker:eu-central-1:446921602837:model-package/{cohere_package}",
"eu-west-1": f"arn:aws:sagemaker:eu-west-1:985815980388:model-package/{cohere_package}",
"eu-west-2": f"arn:aws:sagemaker:eu-west-2:856760150666:model-package/{cohere_package}",
"eu-west-3": f"arn:aws:sagemaker:eu-west-3:843114510376:model-package/{cohere_package}",
"eu-north-1": f"arn:aws:sagemaker:eu-north-1:136758871317:model-package/{cohere_package}",
"ap-southeast-1": f"arn:aws:sagemaker:ap-southeast-1:192199979996:model-package/{cohere_package}",
"ap-southeast-2": f"arn:aws:sagemaker:ap-southeast-2:666831318237:model-package/{cohere_package}",
"ap-northeast-2": f"arn:aws:sagemaker:ap-northeast-2:745090734665:model-package/{cohere_package}",
"ap-northeast-1": f"arn:aws:sagemaker:ap-northeast-1:977537786026:model-package/{cohere_package}",
"ap-south-1": f"arn:aws:sagemaker:ap-south-1:077584701553:model-package/{cohere_package}",
"sa-east-1": f"arn:aws:sagemaker:sa-east-1:270155090741:model-package/{cohere_package}",
}
region = boto3.Session().region_name
if region not in model_package_map.keys():
raise Exception(f"Current boto3 session region {region} is not supported.")
model_package_arn = model_package_map[region]
Create an endpoint and perform real-time inference
If you want to understand how real-time inference with Amazon SageMaker works, refer to the Amazon SageMaker Developer Guide.
Create an endpoint
To create an endpoint, use the following code.
co = Client(region_name=region)
co.create_endpoint(arn=model_package_arn, endpoint_name="cohere-rerank-multilingual-v3-0", instance_type="ml.g5.2xlarge", n_instances=1)
# If the endpoint is already created, you just need to connect to it
# co.connect_to_endpoint(endpoint_name="cohere-rerank-multilingual-v3-0”)
After the endpoint is created, you can perform real-time inference.
Create the input payload
To create the input payload, use the following code.
documents = [
{"Title":"Contraseña incorrecta","Content":"Hola, llevo una hora intentando acceder a mi cuenta y sigue diciendo que mi contraseña es incorrecta. ¿Puede ayudarme, por favor?"},
{"Title":"Confirmation Email Missed","Content":"Hi, I recently purchased a product from your website but I never received a confirmation email. Can you please look into this for me?"},
{"Title":"أسئلة حول سياسة الإرجاع","Content":"مرحبًا، لدي سؤال حول سياسة إرجاع هذا المنتج. لقد اشتريته قبل بضعة أسابيع وهو معيب"},
{"Title":"Customer Support is Busy","Content":"Good morning, I have been trying to reach your customer support team for the past week but I keep getting a busy signal. Can you please help me?"},
{"Title":"Falschen Artikel erhalten","Content":"Hallo, ich habe eine Frage zu meiner letzten Bestellung. Ich habe den falschen Artikel erhalten und muss ihn zurückschicken."},
{"Title":"Customer Service is Unavailable","Content":"Hello, I have been trying to reach your customer support team for the past hour but I keep getting a busy signal. Can you please help me?"},
{"Title":"Return Policy for Defective Product","Content":"Hi, I have a question about the return policy for this product. I purchased it a few weeks ago and it is defective."},
{"Title":"收到错误物品","Content":"早上好,关于我最近的订单,我有一个问题。我收到了错误的商品,需要退货。"},
{"Title":"Return Defective Product","Content":"Hello, I have a question about the return policy for this product. I purchased it a few weeks ago and it is defective."}
]
Perform real-time inference
To perform real-time inference, use the following code.
response = co.rerank(documents=documents, query='What emails have been about returning items?', rank_fields=["Title","Content"], top_n=5)
Visualize output
To visualize output, use the following code.
print(f'Documents: {response}')
The following screenshot shows the output response.
Cleanup
To avoid any recurring charges, use the following steps to clean up the resources created in this walkthrough.
Delete the model
Now that you have successfully performed a real-time inference, you do not need the endpoint anymore. You can terminate the endpoint to avoid being charged.
co.delete_endpoint()
co.close()
Unsubscribe to the listing (optional)
If you want to unsubscribe to the model package, follow these steps. Before you cancel the subscription, make sure that you don’t have a deployable model created from the model package or using the algorithm. You can find this information by looking at the container name associated with the model.
Steps to unsubscribe from the product from AWS Marketplace:
- On the Your Software subscriptions page, choose the Machine Learning tab
- Locate the listing that you want to cancel the subscription for, and then choose Cancel Subscription
Summary
RAG is a capable technique for developing AI applications that integrate real-time data and enable interactive conversations using proprietary information. RAG enhances AI responses by tapping into external, domain-specific knowledge sources, but its effectiveness depends on finding the right source materials. This post focuses on improving search efficiency and accuracy in RAG systems using Cohere Rerank. RAG orchestration typically involves two steps: retrieval of relevant documents and generation of answers. While dense retrieval is efficient for large datasets, it can struggle with complex data and questions due to information compression. Cohere Rerank uses deep learning to evaluate the alignment between documents and queries, outputting a relevance score that enables more nuanced document selection.
Customers can find Cohere Rerank 3 and Cohere Rerank 3 Nimble on Amazon Sagemaker Jumpstart.
About the Authors
Shashi Raina is a Senior Partner Solutions Architect at Amazon Web Services (AWS), where he specializes in supporting generative AI (GenAI) startups. With close to 6 years of experience at AWS, Shashi has developed deep expertise across a range of domains, including DevOps, analytics, and generative AI.
Pradeep Prabhakaran is a Senior Manager – Solutions Architecture at Cohere. In his current role at Cohere, Pradeep acts as a trusted technical advisor to customers and partners, providing guidance and strategies to help them realize the full potential of Cohere’s cutting-edge Generative AI platform.
Accounting for cognitive bias in human evaluation of large language models
A position paper presented at ACL proposes a framework for more-accurate human evaluation of LLMs.Read More
A breakthrough in wildfire detection: How a new constellation of satellites can detect smaller wildfires earlier
FireSat is a new global satellite constellation designed to detect and track wildfires the size of a classroom within 20 minutes.Read More
Unlock AWS Cost and Usage insights with generative AI powered by Amazon Bedrock
Managing cloud costs and understanding resource usage can be a daunting task, especially for organizations with complex AWS deployments. AWS Cost and Usage Reports (AWS CUR) provides valuable data insights, but interpreting and querying the raw data can be challenging.
In this post, we explore a solution that uses generative artificial intelligence (AI) to generate a SQL query from a user’s question in natural language. This solution can simplify the process of querying CUR data stored in an Amazon Athena database using SQL query generation, running the query on Athena, and representing it on a web portal for ease of understanding.
The solution uses Amazon Bedrock, a fully managed service that offers a choice of high-performing foundation models (FMs) from leading AI companies like AI21 Labs, Anthropic, Cohere, Meta, Mistral AI, Stability AI, and Amazon through a single API, along with a broad set of capabilities to build generative AI applications with security, privacy, and responsible AI.
Challenges addressed
The following challenges can hinder organizations from effectively analyzing their CUR data, leading to potential inefficiencies, overspending, and missed opportunities for cost-optimization. We aim to target and simplify them using generative AI with Amazon Bedrock.
- Complexity of SQL queries – Writing SQL queries to extract insights from CUR data can be complex, especially for non-technical users or those unfamiliar with the CUR data structure (unless you’re a seasoned database administrator)
- Data accessibility – To gain insights from structured data in databases, users need to get access to databases, which can be a potential threat to overall data protection
- User-friendliness – Traditional methods of analyzing CUR data often lack a user-friendly interface, making it challenging for non-technical users to take advantage of the valuable insights hidden within the data
Solution overview
The solution that we discuss is a web application (chatbot) that allows you to ask questions related to your AWS costs and usage in natural language. The application generates SQL queries based on the user’s input, runs them against an Athena database containing CUR data, and presents the results in a user-friendly format. The solution combines the power of generative AI, SQL generation, database querying, and an intuitive web interface to provide a seamless experience for analyzing CUR data.
The solution uses the following AWS services:
- Amazon Athena
- Amazon Bedrock
- AWS Billing and Cost Management for cost and usage reports
- Amazon Simple Storage Service (Amazon S3)
- The compute service of your choice on AWS to call Amazon Bedrock APIs. This could be Amazon Elastic Compute Cloud (Amazon EC2), AWS Lambda, AWS SDK, Amazon SageMaker notebooks, or your workstation if you are doing a quick proof of concept. For the purpose of this post, this code is running on a t3a.micro EC2 instance with Amazon Linux 2023.
The following diagram illustrates the solution architecture.
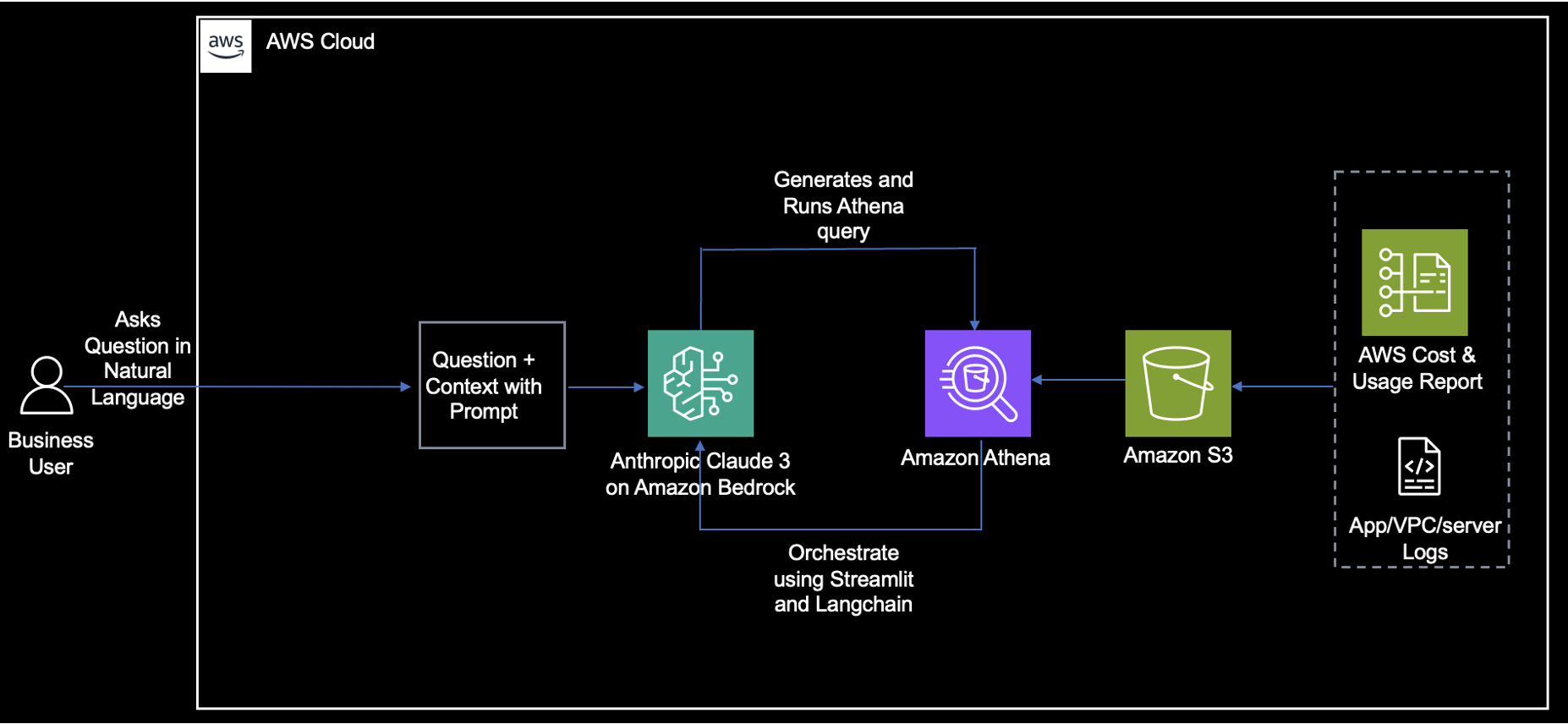
Figure 1. Architecture of Solution
The data flow consists of the following steps:
- The CUR data is stored in Amazon S3.
- Athena is configured to access and query the CUR data stored in Amazon S3.
- The user interacts with the Streamlit web application and submits a natural language question related to AWS costs and usage.
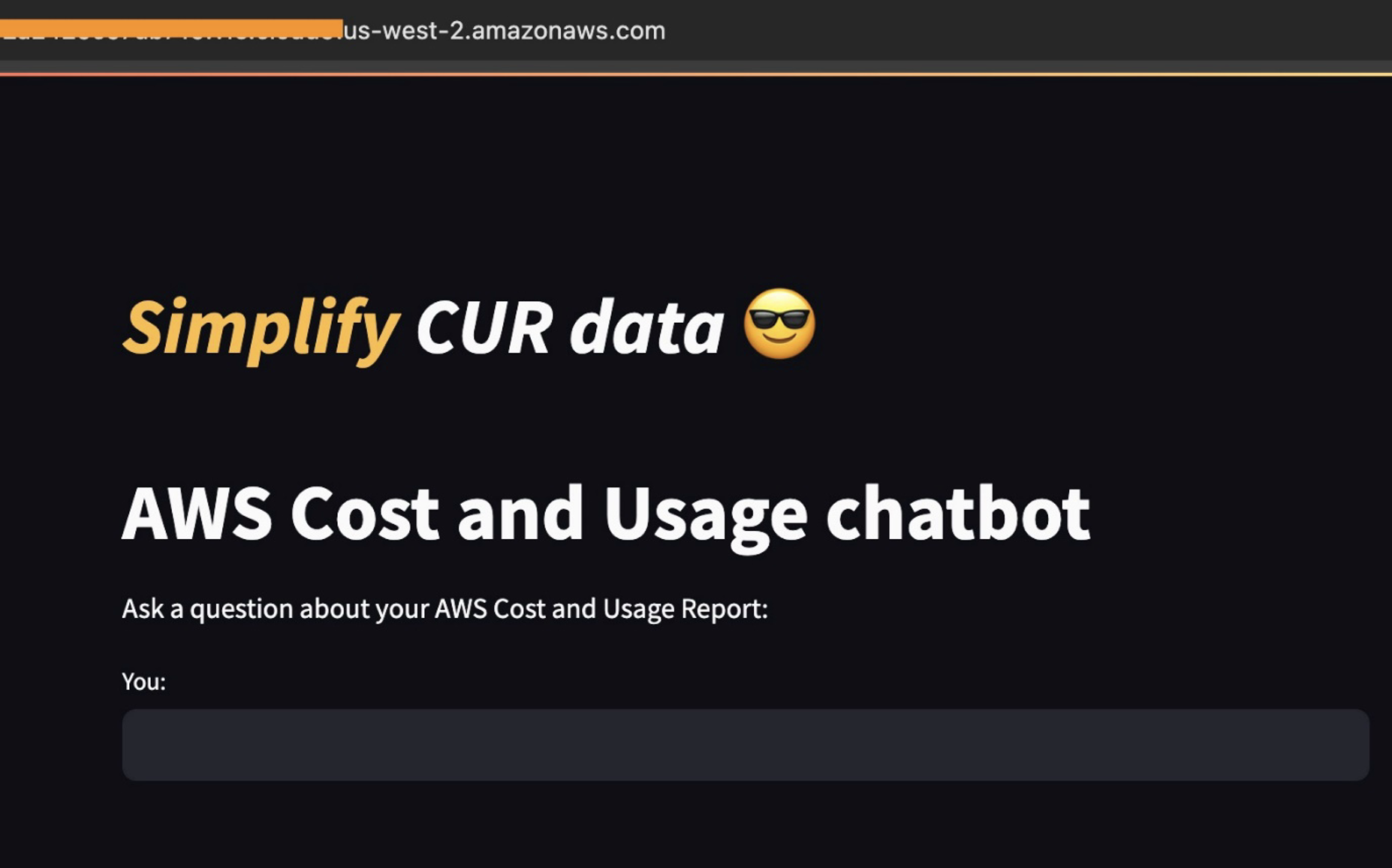
Figure 2. Shows the Chatbot Dashboard to ask question
- The Streamlit application sends the user’s input to Amazon Bedrock, and the LangChain application facilitates the overall orchestration.
- The LangChain code uses the BedrockChat class from LangChain to invoke the FM and interact with Amazon Bedrock to generate a SQL query based on the user’s input.

Figure 3. Shows initialization of SQL chain
- The generated SQL query is run against the Athena database using the FM on Amazon Bedrock, which queries the CUR data stored in Amazon S3.
- The query results are returned to the LangChain application.
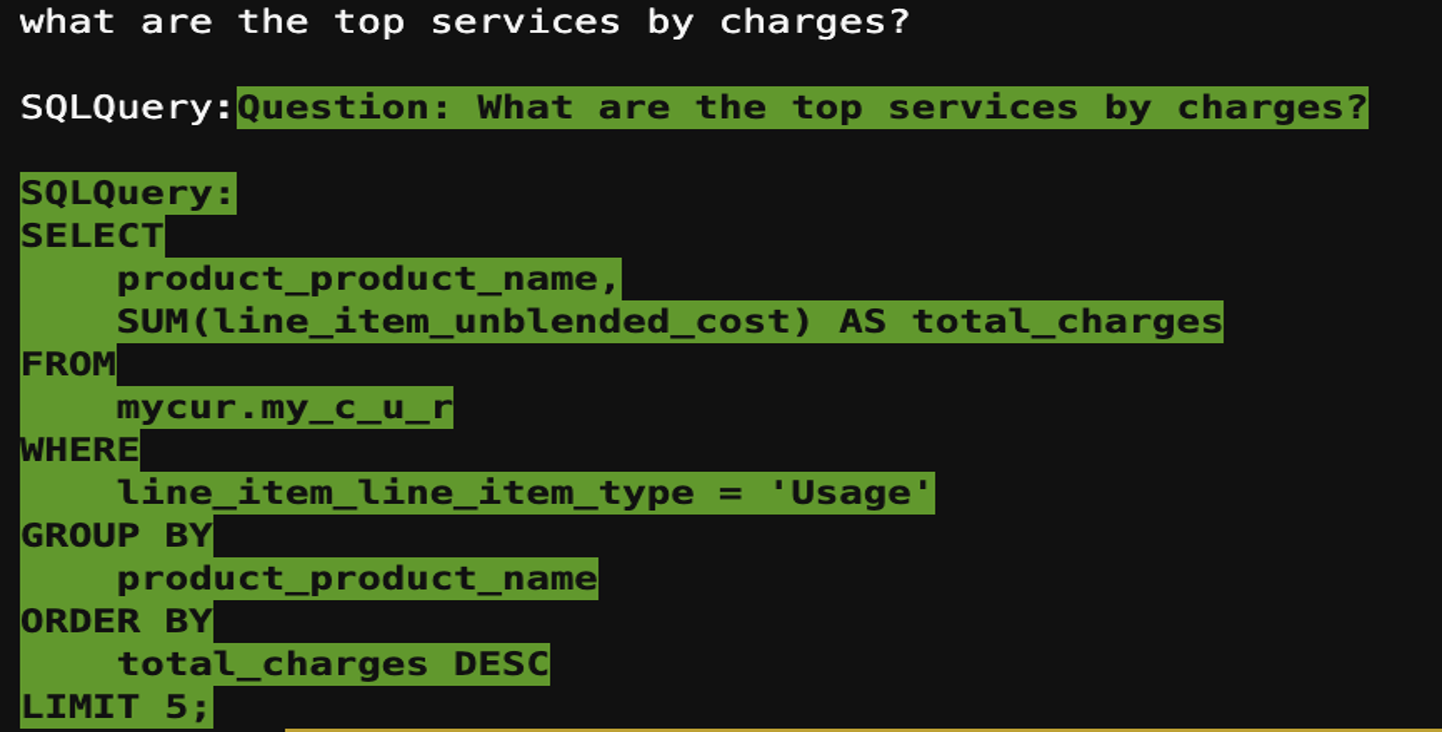
Figure 4. Shows generated Query in the application output logs
- LangChain sends the SQL query and query results back to the Streamlit application.
- The Streamlit application displays the SQL query and query results to the user in a formatted and user-friendly manner.
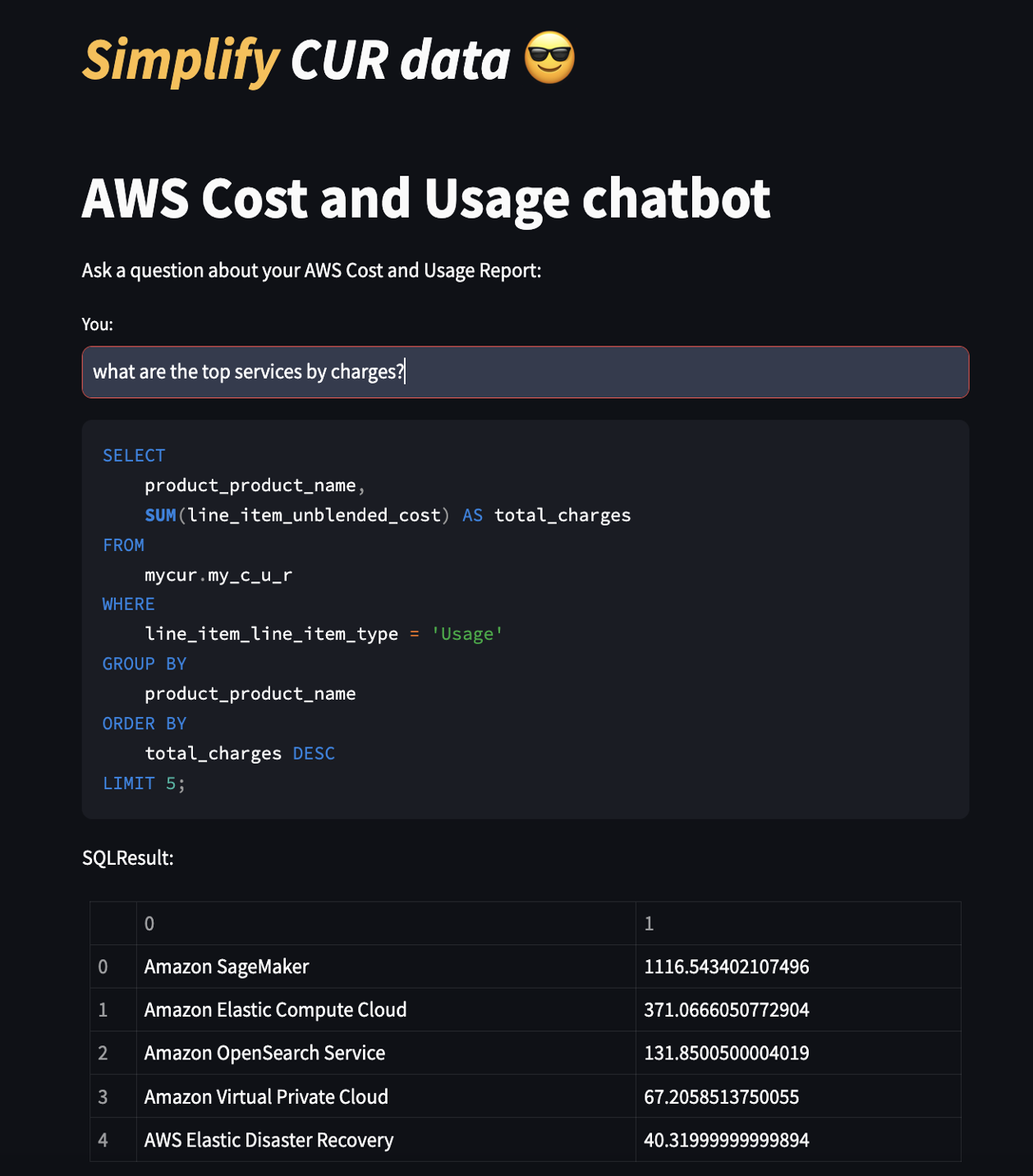
Figure 5. Shows final output presented on the chat bot webapp including SQL Query and the Query results
Prerequisites
To set up this solution, you should have the following prerequisites:
- An AWS account with access to AWS Cost Explorer, Athena, and Amazon S3. (This is a proof of concept setup. You should follow the least privilege model when using AWS Identity and Access Management (IAM), refer to the IAM security best practices documentation, and conduct your own due diligence when setting this up.)
- CUR data stored in an S3 bucket. For instructions, see Creating Cost and Usage Reports.
- Athena set up to analyze the data from your S3 bucket holding CUR data. For instructions, see Querying Cost and Usage Reports using Amazon Athena.
- An AWS compute environment created to host the code and call the Amazon Bedrock APIs.
- An AWS Identity and Access Management (IAM) role with permission to access Amazon Bedrock and access to FMs in Amazon Bedrock.
Configure the solution
Complete the following steps to set up the solution:
- Create an Athena database and table to store your CUR data. Make sure the necessary permissions and configurations are in place for Athena to access the CUR data stored in Amazon S3.
- Set up your compute environment to call Amazon Bedrock APIs. Make sure you associate an IAM role with this environment that has IAM policies that grant access to Amazon Bedrock.
- When your instance is up and running, install the following libraries that are used for working within the environment:
- Use the following code to establish a connection to the Athena database using the langchain library and the pyathena Configure the language model to generate SQL queries based on user input using Amazon Bedrock. You can save this file as cur_lib.py.
- Create a Streamlit web application to provide a UI for interacting with the LangChain application. Include the input fields for users to enter their natural language questions and display the generated SQL queries and query results. You can name this file cur_app.py.
- Connect the LangChain application and Streamlit web application by calling the get_response Format and display the SQL query and result in the Streamlit web application. Append the following code with the preceding application code:
- Deploy the Streamlit application and LangChain application to your hosting environment, such as Amazon EC2, or a Lambda function.
Clean up
Unless you invoke Amazon Bedrock with this solution, you won’t incur charges for it. To avoid ongoing charges for Amazon S3 storage for saving the CUR reports, you can remove the CUR data and S3 bucket. If you set up the solution using Amazon EC2, make sure you stop or delete the instance when you’re done.
Benefits
This solution offers the following benefits:
- Simplified data analysis – You can analyze CUR data using natural language using generative AI, eliminating the need for advanced SQL knowledge
- Increased accessibility – The web-based interface makes it efficient for non-technical users to access and gain insights from CUR data without needing credentials for the database
- Time-saving – You can quickly get answers to your cost and usage questions without manually writing complex SQL queries
- Enhanced visibility – The solution provides visibility into AWS costs and usage, enabling better cost-optimization and resource management decisions
Summary
The AWS CUR chatbot solution uses Anthropic Claude on Amazon Bedrock to generate SQL queries, database querying, and a user-friendly web interface to simplify the analysis of CUR data. By allowing you to ask natural language questions, the solution removes barriers and empowers both technical and non-technical users to gain valuable insights into AWS costs and resource usage. With this solution, organizations can make more informed decisions, optimize their cloud spending, and improve overall resource utilization. We recommend that you do due diligence while setting this up, especially for production; you can choose other programming languages and frameworks to set it up according to your preference and needs.
Amazon Bedrock enables you to build powerful generative AI applications with ease. Accelerate your journey by following the quick start guide on GitHub and using Amazon Bedrock Knowledge Bases to rapidly develop cutting-edge Retrieval Augmented Generation (RAG) solutions or enable generative AI applications to run multistep tasks across company systems and data sources using Amazon Bedrock Agents.
About the Author
Anutosh is a Solutions Architect at AWS India. He loves to dive deep into his customers’ use cases to help them navigate through their journey on AWS. He enjoys building solutions in the cloud to help customers. He is passionate about migration and modernization, data analytics, resilience, cybersecurity, and machine learning.
Streamline workflow orchestration of a system of enterprise APIs using chaining with Amazon Bedrock Agents
Intricate workflows that require dynamic and complex API orchestration can often be complex to manage. In industries like insurance, where unpredictable scenarios are the norm, traditional automation falls short, leading to inefficiencies and missed opportunities. With the power of intelligent agents, you can simplify these challenges. In this post, we explore how chaining domain-specific agents using Amazon Bedrock Agents can transform a system of complex API interactions into streamlined, adaptive workflows, empowering your business to operate with agility and precision.
Amazon Bedrock is a fully managed service that offers a choice of high-performing foundation models (FMs) from leading artificial intelligence (AI) companies like AI21 Labs, Anthropic, Cohere, Meta, Mistral AI, Stability AI, and Amazon through a single API, along with a broad set of capabilities to build generative AI applications with security, privacy, and responsible AI.
Benefits of chaining Amazon Bedrock Agents
Designing agents is like designing other software components—they tend to work best when they have a focused purpose. When you have focused, single-purpose agents, combining them into chains can allow them to solve significantly complex problems together. Using natural language processing (NLP) and OpenAPI specs, Amazon Bedrock Agents dynamically manages API sequences, minimizing dependency management complexities. Additionally, agents enable conversational context management in real-time scenarios, using session IDs and, if necessary, backend databases like Amazon DynamoDB for extended context storage. By using prompt instructions and API descriptions, agents collect essential information from API schemas to solve specific problems efficiently. This approach not only enhances agility and flexibility, but also demonstrates the value of chaining agents to simplify complex workflows and solve larger problems effectively.
In this post, we explore an insurance claims use case, where we demonstrate the concept of chaining with Amazon Bedrock Agents. This involves an orchestrator agent calling and interacting with other agents to collaboratively perform a series of tasks, enabling efficient workflow management.
Solution overview
For our use case, we develop a workflow for an insurance digital assistant focused on streamlining tasks such as filing claims, assessing damages, and handling policy inquiries. The workflow simulates API sequencing dependencies, such as conducting fraud checks during claim creation and analyzing uploaded images for damage assessment if the user provides images. The orchestration dynamically adapts to user scenarios, guided by natural language prompts from domain-specific agents like an insurance orchestrator agent, policy information agent, and damage analysis notification agent. Using OpenAPI specifications and natural language prompts, the API sequencing in our insurance digital assistant adapts to dynamic user scenarios, such as users opting in or out of image uploads for damage assessment, failing fraud checks or choosing to ask a variety of questions related to their insurance policies and coverages. This flexibility is achieved by chaining domain-specific agents like the insurance orchestrator agent, policy information agent, and damage analysis notification agent.
Traditionally, insurance processes are rigid, with fixed steps for tasks like fraud detection. However, agent chaining allows for greater flexibility and adaptability, enabling the system to respond to real-time user inputs and variations in scenarios. For instance, instead of strictly adhering to predefined thresholds for fraud checks, the agents can dynamically adjust the workflow based on user interactions and context. Similarly, when users choose to upload images while filing a claim, the workflow can perform real-time damage analysis and immediately send a summary to claims adjusters for further review. This enables a quicker response and more accurate decision-making. This approach not only streamlines the claims process but also allows for a more nuanced and efficient handling of tasks, providing the necessary balance between automation and human intervention. By chaining Amazon Bedrock Agents, we create a system that is adaptable. This system caters to diverse user needs while maintaining the integrity of business processes.
The following diagram illustrates the end-to-end insurance claims workflow using chaining with Amazon Bedrock Agents.
The diagram shows how specialized agents use various tools to streamline the entire claims process—from filing claims and assessing damages to answering customer questions about insurance policies.
Prerequisites
Before proceeding, make sure you have the following resources set up:
- An AWS account. If you don’t have an account, you can sign up for one.
- Access as an AWS Identity and Access Management (IAM) administrator or an IAM user that has permissions for:
- Deploying AWS CloudFormation
- Creating and managing Amazon Simple Storage Service (Amazon S3) buckets and uploading objects.
- Creating and updating Amazon Simple Queue Service (Amazon SQS) queues, AWS Lambda functions, and Amazon API Gateway.
- Creating and managing IAM roles.
- Access to Amazon Bedrock, Anthropic’s Claude models on Amazon Bedrock, and the Cohere Embed English embedding model on Amazon Bedrock. You must explicitly enable access to models before they can be used with Amazon Bedrock. For instructions, refer to Model access.
Deploy the solution with AWS CloudFormation
Complete the following steps to set up the solution resources:
- Sign in to the AWS Management Console as an IAM administrator or appropriate IAM user.
- Choose Launch Stack to deploy the CloudFormation template.
- Provide the necessary parameters and create the stack.
For this setup, we use us-east-1 as our AWS Region, the Anthropic Claude 3 Haiku model for orchestrating the flow between the different agents, the Anthropic Claude 3 Sonnet model for damage analysis of the uploaded images, and the Cohere Embed English V3 model as an embedding model to translate text from the insurance policy documents into numerical vectors, which allows for efficient search, comparison, and categorization of the documents.
If you want to choose other models on Amazon Bedrock, you can do so by making appropriate changes in the CloudFormation template. Check for appropriate model support in the Region and the features that are supported by the models.
This will take about 15 minutes to deploy the solution. After the stack is deployed, you can view the various outputs of the CloudFormation stack on the Outputs tab, as shown in the following screenshot.
The following screenshot shows the three Amazon Bedrock agents that were deployed in your account.
Test the claims creation, damage detection, and notification workflows
The first part of the deployed solution is to mimic filing a new insurance claim, fraud detection, optional damage analysis of uploading images, and subsequent notification to claims adjusters. This is a smaller version of task automation to fulfill a particular business problem achieved by chaining agents, each performing a set of specific tasks. The agents work in harmony to solve the larger function of insurance claims handling.
Let’s explore the architecture of the claim creation workflow, where the insurance orchestrator agent and the damage analysis notification agent work together to simulate filing new claims, assessing damages, and sending a summary of damages to the claim adjusters for human oversight. The following diagram illustrates this workflow.
In this workflow, the insurance orchestrator agent mimics fraud detection and claims creation as well as orchestrates handing off the responsibility to other task-specific agents. The image damage analysis notification agent is responsible for doing a preliminary analysis of the images uploaded for a damage. This agent invokes a Lambda function that internally calls the Anthropic Claude Sonnet large language model (LLM) on Amazon Bedrock to perform preliminary analysis on the images. The LLM generates a summary of the damage, which is sent to an SQS queue, and is subsequently reviewed by the claim adjusters.
The NLP instruction prompts combined with the OpenAPI specifications for each action group guide the agents in their decision-making process, determining which action group to invoke, the sequence of invocation, and the required parameters for calling specific APIs.
Use the UI to invoke the claims processing workflow
Complete the following steps to invoke the claims processing workflow:
- From the outputs of the CloudFormation stack, choose the URL for
HttpApiEndpoint
.
- You can ask the chatbots sample questions to start exploring the functionality of filing a new claim.
In the following example, we ask for filing a new claim and uploading images as evidence for the claim.
- On the Amazon SQS console, you can view the SQS queue that has been created by the CloudFormation stack and check the message that shows the damage analysis from the image performed by our LLM.
Test the policy information workflow
The following diagram shows the architecture of just the policy information agent. The policy agent accesses the Policy Information API to extract answers to insurance-related questions from unstructured policy documents such as PDF files.
The policy information agent is responsible for doing a lookup against the insurance policy documents stored in the knowledge base. The agent invokes a Lambda function that will internally invoke the knowledge base to find answers to policy-related questions.
Set up the policy documents and metadata in the data source for the knowledge base
We use Amazon Bedrock Knowledge Bases to manage our documents and metadata. As part of deploying the solution, the CloudFormation stack created a knowledge base. Complete the following steps to set up its data source:
- On the Amazon Bedrock console, navigate to the deployed knowledge base and navigate to the S3 bucket that is mentioned as its data source.
- Upload a few insurance policy documents and metadata documents to the S3 bucket to mimic the naming conventions as shown in the following screenshot.
The naming conventions are <Type of Policy>_PolicyNumber.pdf
for the insurance policy PDF documents and <Type of Policy>_PolicyNumber.pdf.metadata.json
for the metadata documents.
The following screenshot shows an example of what a sample metadata.json
file looks like.
- After the documents are uploaded to Amazon S3, navigate to the deployed knowledge base, select the data source, and choose Sync.
To understand more about how metadata support in Knowledge Bases on Amazon Bedrock helps you get accurate results, refer to Amazon Bedrock Knowledge Bases now supports metadata filtering to improve retrieval accuracy.
- Now you can go back to the UI and start asking questions related to the policy documents.
The following screenshot shows the set of questions we asked for finding answers related to policy coverage.
Clean up
To avoid unexpected charges, complete the following steps to clean up your resources:
- Delete the contents from the S3 buckets corresponding to the
ImageBucketName
andPolicyDocumentsBucketName
keys from the outputs of the CloudFormation stack. - Delete the deployed stack using the AWS CloudFormation console.
Best practices
The following are some additional best practices that you can follow for your agents:
- Automated testing – Implement automated tests using tools to regularly test the orchestration workflows. You can use mock APIs to simulate various scenarios and validate the agent’s decision-making process.
- Version control – Maintain version control for your agent configurations and prompts in a repository. This provides traceability and quick rollback if needed.
- Monitoring and logging – Use Amazon CloudWatch to monitor agent interactions and API calls. Set up alarms for unexpected behaviors or failures.
- Continuous integration – Set up a continuous integration and delivery (CI/CD) pipeline that integrates automated testing, prompt validation, and deployment to maintain smooth updates without disrupting ongoing workflows.
Conclusion
In this post, we demonstrated the power of chaining Amazon Bedrock agents, offering a fresh perspective on integrating back-office automation workflows and enterprise APIs. This solution offers several benefits: as new enterprise APIs emerge, dependencies in existing ones can be minimized, reducing coupling. Moreover, Amazon Bedrock Agents can maintain conversational context, enabling follow-up queries to use conversation history. For extended contextual memory, a more persistent backend implementation can be considered.
To learn more, refer to Amazon Bedrock Agents.
About the Author
Piyali Kamra is a seasoned enterprise architect and a hands-on technologist who has over two decades of experience building and executing large scale enterprise IT projects across geographies. She believes that building large scale enterprise systems is not an exact science but more like an art, where you can’t always choose the best technology that comes to one’s mind but rather tools and technologies must be carefully selected based on the team’s culture , strengths, weaknesses and risks, in tandem with having a futuristic vision as to how you want to shape your product a few years down the road.
Build ultra-low latency multimodal generative AI applications using sticky session routing in Amazon
Amazon SageMaker is a fully managed machine learning (ML) service. With SageMaker, data scientists and developers can quickly and confidently build, train, and deploy ML models into a production-ready hosted environment. SageMaker provides a broad selection of ML infrastructure and model deployment options to help meet your ML inference needs. It also helps scale your model deployment, manage models more effectively in production, and reduce operational burden.
Although early large language models (LLMs) were limited to processing text inputs, the rapid evolution of these AI systems has enabled LLMs to expand their capabilities to handle a wide range of media types, including images, video, and audio, ushering in the era of multimodal models. Multimodal is a type of deep learning using multiple modalities of data, such as text, audio, or images. Multimodal inference adds challenges of large data transfer overhead and slow response times. For instance, in a typical chatbot scenario, users initiate the conversation by providing a multimedia file or a link as input payload, followed by a back-and-forth dialogue, asking questions or seeking information related to the initial input. However, transmitting large multimedia files with every request to a model inference endpoint can significantly impact the response times and latency, leading to an unsatisfactory user experience. For example, sending a 500 MB input file could potentially add 3–5 seconds to the response time, which is unacceptable for a chatbot aiming to deliver a seamless and responsive interaction.
We are announcing the availability of sticky session routing on Amazon SageMaker Inference which helps customers improve the performance and user experience of their generative AI applications by leveraging their previously processed information. Amazon SageMaker makes it easier to deploy ML models including foundation models (FMs) to make inference requests at the best price performance for any use case.
By enabling sticky sessions routing, all requests from the same session are routed to the same instance, allowing your ML application to reuse previously processed information to reduce latency and improve user experience. This is particularly valuable when you want to use large data payloads or need seamless interactive experiences. By using your previous inference requests, you can now take advantage of this feature to build innovative state-aware AI applications on SageMaker. To do, you create a session ID with your first request, and then use that session ID to indicate that SageMaker should route all subsequent requests to the same instance. Sessions can also be deleted when done to free up resources for new sessions.
This feature is available in all AWS Regions where SageMaker is available. To learn more about deploying models on SageMaker, see Amazon SageMaker Model Deployment. For more about this feature, refer to Stateful sessions with Amazon SageMaker models.
Solution overview
SageMaker simplifies the deployment of models, enabling chatbots and other applications to use their multimodal capabilities with ease. SageMaker has implemented a robust solution that combines two key strategies: sticky session routing in SageMaker with load balancing, and stateful sessions in TorchServe. Sticky session routing makes sure all requests from a user session are serviced by the same SageMaker server instance. Stateful sessions in TorchServe cache the multimedia data in GPU memory from the session start request and minimize loading and unloading of this data from GPU memory for improved response times.
With this focus on minimizing data transfer overhead and improving response time, our approach makes sure the initial multimedia file is loaded and processed only one time, and subsequent requests within the same session can use the cached data.
Let’s look at the sequence of events when a client initiates a sticky session on SageMaker:
- In the first request, you call the Boto3 SageMaker runtime invoke_endpoint with
session-id=NEW_SESSION
in the header and a payload indicating an open session type of request. SageMaker then creates a new session and stores the session ID. The router initiates an open session (this API is defined by the client; it could be some other name likestart_session
) with the model server, in this case TorchServe, and responds back with 200 OK along with the session ID and time to live (TTL), which is sent back to the client.
- Whenever you need to use the same session to perform subsequent actions, you pass the session ID as part of the
invoke_endpoint
call, which allows SageMaker to route all the subsequent requests to the same model server instance. - To close or delete a session, you can use
invoke_endpoint
with a payload indicating a close session type of request along with the session ID. The SageMaker router first checks if the session exists. If it does, the router initiates a close session call to the model server, which responds back with a successful 200 OK along with session ID, which is sent back to the client. In the scenario, when the session ID doesn’t exist, the router responds back with a 400 response.
In the following sections, we walk through an example of how you can use sticky routing in SageMaker to achieve stateful model inference. For this post, we use the LLaVA: Large Language and Vision Assistant model. LLaVa is a multimodal model that accepts images and text prompts.
We use LLaVa to upload an image and then ask questions about the image without having to resend the image for every request. The image is cached in the GPU memory as opposed to the CPU memory, so we don’t have to incur the latency cost of moving this image from CPU memory to GPU memory on every call.
We use TorchServe as our model server for this example. TorchServe is a performant, flexible and easy to use tool for serving PyTorch models in production. TorchServe supports a wide array of advanced features, including dynamic batching, microbatching, model A/B testing, streaming, torch XLA, tensorRT, ONNX and IPEX. Moreover, it seamlessly integrates PyTorch’s large model solution, PiPPy, enabling efficient handling of large models. Additionally, TorchServe extends its support to popular open-source libraries like DeepSpeed, Accelerate, Fast Transformers, and more, expanding its capabilities even further.
The following are the main steps to deploy the LLava model. The section below introduces the steps conceptually, so you’ll have a better grasp of the overall deployment workflow before diving into the practical implementation details in the subsequent section.
Build a TorchServe Docker container and push it to Amazon ECR
The first step is to build a TorchServe Docker container and push it to Amazon Elastic Container Registry (Amazon ECR). Because we’re using a custom model, we use the bring your own container approach. We use one of the AWS provided deep learning containers as our base, namely pytorch-inference:2.3.0-gpu-py311-cu121-ubuntu20.04-sagemaker
.
Build TorchServe model artifacts and upload them to Amazon S3
We use torch-model-archiver
to gather all the artifacts, like custom handlers, the LlaVa model code, the data types for request and response, model configuration, prediction API, and other utilities. Then we upload the model artifacts to Amazon Simple Storage Service (Amazon S3).
Create the SageMaker endpoint
To create the SageMaker endpoint, complete the following steps:
- To create the model, use the SageMaker Python SDK Model class and as inputs. Specify the S3 bucket you created earlier to upload the TorchServe model artifacts and the
image_uri
of the Docker container you created.
SageMaker expects the session ID in X-Amzn-SageMaker-Session-Id
format; you can specify that in the environment properties to the model.
- To deploy the model and create the endpoint, specify the initial instance count to match the load, instance type, and timeouts.
- Lastly, create a SageMaker Python SDK Predictor by passing in the endpoint name.
Run inference
Complete the following steps to run inference:
- Use an open session to send a URL to the image you want to ask questions about.
This is a custom API we have defined for our use case (see inference_api.py). You can define the inputs, outputs, and APIs to suit your business use case. For this use case, we use an open session to send a URL to the image we want to ask questions about. For the session ID header value, use the special string NEW_SESSION
to indicate this is the start of a session. The custom handler you wrote downloads the image, converts it to a tensor, and caches that in the GPU memory. We do this because we have access to the LLaVa source code; we could also modify the original predict.py file from LLaVa model to accept a tensor instead of a PIL image. By caching the tensor in GPU, we have saved some inference time by not moving the image from CPU memory to GPU memory for every call. If you don’t have access to the model source code, you have to cache the image in CPU memory. Refer to inference_api.py for this source code. The open session API call returns a session ID, which you use for the rest of the calls in this session.
- To send a text prompt, get the session ID from the open session and send it along with the text prompt.
inference_api.py looks up the cache in GPU for the image based on the session ID and uses that for inference. This returns the LLaVa model output as a string.
- Repeat the previous step to send a different text prompt.
- When you’re done with all the text prompts, use the session ID to close the session.
In inference_api.py, we no longer hold on to the image cache in GPU.
The source code for this example is in the GitHub repo. You can run the steps using the following notebook.
Prerequisites
Use the following code to deploy an AWS CloudFormation stack that creates an AWS Identity and Access Management (IAM) role to deploy the SageMaker endpoints:
Create a SageMaker notebook instance
Complete the following steps to create a notebook instance for LLaVa model deployment:
- On the SageMaker console, choose Notebooks in the navigation pane.
- Choose Create notebook instance.
- In the Notebook instance settings section, under Additional configuration, choose at least 500 GB for the storage volume.
- In the Permissions and encryption section, choose to use an existing IAM role, and choose the role you created in the prerequisites (
sm-stateful-role-xxx
).
You can get the full name of the role on the AWS CloudFormation console, on the Resources tab of the stack sm-stateful-role
.
- In the Git repositories section, for Git repository URL, enter
https://github.com/aws-samples/sagemaker-genai-hosting-examples.git
.
- Choose Create notebook instance.
Run the notebook
When the notebook is ready, complete the following steps:
- On the SageMaker console, choose Notebooks in the navigation pane.
- Choose Open JupyterLab for this new instance.
- In JupyterLab, navigate to
LLava
using the file explorer.
- Navigate to
torchserve /workspace /
and open the notebookllava_stateful_deploy_infer.ipynb
.
- Run the notebook.
The ./build_and_push.sh
script takes approximately 30 minutes to run. You can also run the ./build_and_push.sh
script in a terminal for better feedback. Note the input parameters from the previous step and make sure you’re in the right directory (sagemaker-genai-hosting-examples/LLava/torchserve/workspace
).
The model.deploy()
step also takes 20–30 minutes to complete.
- When you’re done, run the last cleanup cell.
- Additionally, delete the SageMaker notebook instance.
Troubleshooting
When you run ./build_and_push.sh
, you might get the following error:
This means you’re not using SageMaker notebooks, and are probably using Amazon SageMaker Studio. Docker is not installed in SageMaker Studio by default.
Look at the screen shot below to learn how to open Amazon SageMaker Notebook.
Conclusion
In this post, we explained how the new sticky routing feature in Amazon SageMaker allows you to achieve ultra-low latency and enhance your end-user experience when serving multi-modal models. You can use the provided notebook and create stateful endpoints for your multimodal models to enhance your end-user experience.
Try out this solution for your own use case, and let us know your feedback and questions in the comments.
About the authors
Harish Rao is a senior solutions architect at AWS, specializing in large-scale distributed AI training and inference. He empowers customers to harness the power of AI to drive innovation and solve complex challenges. Outside of work, Harish embraces an active lifestyle, enjoying the tranquility of hiking, the intensity of racquetball, and the mental clarity of mindfulness practices.
Raghu Ramesha is a Senior GenAI/ML Solutions Architect on the Amazon SageMaker Service team. He focuses on helping customers build, deploy, and migrate ML production workloads to SageMaker at scale. He specializes in machine learning, AI, and computer vision domains, and holds a master’s degree in computer science from UT Dallas. In his free time, he enjoys traveling and photography.
Lingran Xia is a software development engineer at AWS. He currently focuses on improving inference performance of machine learning models. In his free time, he enjoys traveling and skiing.
Naman Nandan is a software development engineer at AWS, specializing in enabling large scale AI/ML inference workloads on SageMaker using TorchServe, a project jointly developed by AWS and Meta. In his free time, he enjoys playing tennis and going on hikes.
Li Ning is a senior software engineer at AWS with a specialization in building large-scale AI solutions. As a tech lead for TorchServe, a project jointly developed by AWS and Meta, her passion lies in leveraging PyTorch and AWS SageMaker to help customers embrace AI for the greater good. Outside of her professional endeavors, Li enjoys swimming, traveling, following the latest advancements in technology, and spending quality time with her family.
Frank Liu is a Principal Software Engineer for AWS Deep Learning. He focuses on building innovative deep learning tools for software engineers and scientists. Frank has in-depth knowledge on the infrastructure optimization and Deep Learning acceleration.
Deepika Damojipurapu is a Senior Technical Account Manager at AWS, specializing in distributed AI training and inference. She helps customers unlock the full potential of AWS by providing consultative guidance on architecture and operations, tailored to their specific applications and use cases. When not immersed in her professional responsibilities, Deepika finds joy in spending quality time with her family – exploring outdoors, traveling to new destinations, cooking wholesome meals together, creating cherished memories.
Alan Tan is a Principal Product Manager with SageMaker, leading efforts on large model inference. He’s passionate about applying machine learning to building novel solutions. Outside of work, he enjoys the outdoors.
Rethinking LLM Memorization
Introduction
A central question in the discussion of large language models (LLMs) concerns the extent to which they memorize their training data versus how they generalize to new tasks and settings. Most practitioners seem to (at least informally) believe that LLMs do some degree of both: they clearly memorize parts of the training data—for example, they are often able to reproduce large portions of training data verbatim [Carlini et al., 2023]—but they also seem to learn from this data, allowing them to generalize to new settings. The precise extent to which they do one or the other has massive implications for the practical and legal aspects of such models [Cooper et al., 2023]. Do LLMs truly produce new content, or do they only remix their training data? Should the act of training on copyrighted data be deemed an unfair use of data, or should fair use be judged by some notion of model memorization? When dealing with humans, we distinguish plagiarizing content from learning from it, but how should this extend to LLMs? The answer inherently relates to the definition of memorization for LLMs and the extent to which they memorize their training data.
However, even defining memorization for LLMs is challenging, and many existing definitions leave much to be desired. In our recent paper (project page), we propose a new definition of memorization based on a compression argument. Our definition posits that
a phrase present in the training data is memorized if we can make the model reproduce the phrase using a prompt (much) shorter than the phrase itself.
Operationalizing this definition requires finding the shortest adversarial input prompt that is specifically optimized to produce a target output. We call this ratio of input-to-output tokens the Adversarial Compression Ratio (ACR). In other words, memorization is inherently tied to whether a certain output can be represented in a compressed form beyond what language models can do with typical text. We argue that such a definition provides an intuitive notion of memorization. If a certain phrase exists within the LLM training data (e.g., is not itself generated text) and it can be reproduced with fewer input tokens than output tokens, then the phrase must be stored somehow within the weights of the LLM. Although it may be more natural to consider compression in terms of the LLM-based notions of input/output perplexity, we argue that a simple compression ratio based on input/output token counts provides a more intuitive explanation to non-technical audiences and has the potential to serve as a legal basis for important questions about memorization and permissible data use. In addition to its intuitive nature, our definition has several other desirable qualities. We show that it appropriately ascribes many famous quotes as being memorized by existing LLMs (i.e., they have high ACR values). On the other hand, we find that text not in the training data of an LLM, such as samples posted on the internet after the training period, are not compressible, that is their ACR is low.
We examine several unlearning methods using ACR to show that they do not substantially affect the memorization of the model. That is, even after explicit finetuning, models asked to “forget” certain pieces of content are still able to reproduce them with a high ACR—in fact, not much smaller than with the original model. Our approach provides a simple and practical perspective on what memorization can mean, providing a useful tool for functional and legal analysis of LLMs.
Why We Need A New Definition
With LLMs ingesting more and more data, questions about their memorization are attracting attention [e.g., Carlini et al., 2019, 2023; Nasr et al., 2023; Zhang et al., 2023]. There remains a pressing need to accurately define memorization in a way that serves as a practical tool to ascertain the fair use of public data from a legal standpoint. To ground the problem, consider the court’s role in determining whether an LLM is breaching copyright. What constitutes a breach of copyright remains contentious, and prior work defines this on a spectrum from ‘training on a data point itself constitutes violation’ to ‘copyright violation only occurs if a model verbatim regurgitates training data.’ To formalize our argument for a new notion of memorization, we start with three definitions from prior work to highlight some of the gaps in the current thinking about memorization.
Discoverable memorization [Carlini et al., 2023], which says a string is memorized if the first few words elicit the rest of the quote exactly, has three particular problems. It is very permissive, easy to evade, and requires validation data to set parameters. Another notion is Extractable Memorization [Nasr et al., 2023], which says that if there exists a prompt that elicits the string in response. This falls too far on the other side of the issue by being very restrictive—what if the prompt includes the entire string in question, or worse, the instructions to repeat it? LLMs that are good at repeating will follow that instruction and output any string they are asked to. The risk is that it is possible to label any element of the training set as memorized, rendering this definition unfit in practice. Another definition is Counterfactual Memorization [Zhang et al., 2023], which aims to separate memorization from generalization and is tested through retraining many LLMs. Given the cost of training LLMs, such a definition is impractical for legal use.
In addition to these definitions from prior work on LLM memorization, several other seemingly viable approaches to memorization exist. Ultimately, we argue all of these frameworks—the definitions in existing work and the approaches described below—are each missing key elements of a good definition for assessing fair use of data.
Membership is not memorization. Perhaps if a copyrighted piece of data is in the training set at all, we might consider it a problem. However, there is a subtle but crucial difference between training set membership and memorization. In particular, the ongoing lawsuits in the field [e.g., as covered by Metz and Robertson, 2024] leave open the possibility that reproducing another’s creative work is problematic, but training on samples from that data may not be. This is common practice in the arts—consider that a copycat comedian telling someone else’s jokes is stealing, but an up-and-comer learning from tapes of the greats is doing nothing wrong. So while membership inference attacks (MIAs) [e.g. Shokri et al., 2017] may look like tests for memorization and they are even intimately related to auditing machine unlearning [Carlini et al., 2021, Pawelczyk et al., 2023, Choi et al., 2024], they have three issues as tests for memorization. Specifically, they are very restrictive, they are hard to arbitrate, and evaluation techniques are brittle.
Adversarial Compression Ratio
Our definition of memorization is based on answering the following question: Given a piece of text, how short is the minimal prompt that elicits that text exactly? In this section, we formally define and introduce our MiniPrompt
algorithm that we use to answer our central question.
To begin, let a target natural text string (s) have a token sequence representation (xin mathcal V^*), which is a list of integer-valued indices that index a given vocabulary (mathcal V). We use (|cdot|) to count the length of a token sequence. A tokenizer (T:smapsto x) maps from strings to token sequences. Let (M) be an LLM that takes a list of tokens as input and outputs the next token probabilities. Consider that (M) can perform generation by repeatedly predicting the next token from all the previous tokens with the argmax
of its output appended to the sequence at each step (this process is called greedy decoding). With a slight abuse of notation, we will also call the greedy decoding result the output of (M). Let (y) be the token sequence generated by (M), which we call a completion or response: (y = M(x)), which in natural language says that the model generates (y) when prompted with (x) or that (x) elicits (y) as a response from (M). So our compression ratio ACR is defined for a target sequence (y) as ACR((M, y) = frac{|y|}{|x^*|}), where (x^* = text{argmin}_{x} |x|) s.t. (M(x) = y).
Definition [(tau)-Compressible Memorization] Given a generative model (M), a sample (y) from the training data is (tau)-memorized if the ACR((M, y) > tau(y)).
The threshold (tau(y)) is a configurable parameter of this definition. We might choose to compare the ACR to the compression ratio of the text when run through a general-purpose compression program (explicitly assumed not to have memorized any such text) such as GZIP [Gailly and Adler, 1992] or SMAZ [Sanfilippo, 2006]. This amounts to setting (tau(y)) equal to the SMAZ compression ratio of (y), for example. Alternatively, one might even use the compression ratio of the arithmetic encoding under another LLM as a comparison point, for example, if it was known with certainty that the LLM was never trained on the target output and hence could not have memorized it [Delétang et al., 2023]. In reality, copyright attribution cases are always subjective, and the goal of this work is not to argue for the right threshold function but rather to advocate for the adversarial compression framework for arbitrating fair data use. Thus, we use (tau = 1), which we believe has substantial practical value. 1
Our definition and the compression ratio lead to two natural ways to aggregate over a set of examples. First, we can average the ratio over all samples/test strings and report the average compression ratio (this is (tau)-independent). Second, we can label samples with a ratio greater than one as memorized and discuss the portion memorized over some set of test cases (for our choice of (tau =1 )).
Empirical Findings
Model Size vs. Memorization: Since prior work has proposed alternative definitions of memorization that show that bigger models memorize more [Carlini et al., 2023], we ask whether our definition leads to the same finding. We find the same trends under our definition, meaning our view of memorization is consistent with existing scientific findings.
Unlearning for Privacy: We further experiment with models finetuned on synthetic data, which show that completion-based tests (i.e., the model’s ability to generate a specific output) often fail to fully reflect the model’s memorization. However, the ACR captures the persistence of memorization even after moderate attempts at unlearning.
Four Categorties of Data for Validation: We also validate the ACR as a metric using four different types of data: random sequences, famous quotes, Wikipedia sentences, and recent Associated Press (AP) articles. The goal is to ensure that the ACR aligns with intuitive expectations of memorization. Our results show that random sequences and recent AP articles, which the models were not trained on, are not compressible (i.e., not memorized). Famous quotes, which are repeated in the training data, show high compression ratios, indicating memorization. Wikipedia sentences fall between the two extremes, as some of them are memorized. These results validate that ACR meaningfully identifies memorization in data that is more common or repeated in the training set, while appropriately labelling unseen data as not-memorized.
When proposing new definitions, we are tasked with justifying why a new one is needed as well as showing its ability to capture a phenomenon of interest. This stands in contrast to developing detection/classification tools whose accuracy can easily be measured using labeled data. It is difficult by nature to define memorization as there is no set of ground truth labels that indicate which samples are memorized. Consequently, the criteria for a memorization definition should rely on how useful it is. Our definition is a promising direction for future regulation on LLM fair use of data as well as helping model owners confidently release models trained on sensitive data without releasing that data. Deploying our framework in practice may require careful thought about how to set the compression threshold but as it relates to the legal setting this is not a limitation as law suits always have some subjectivity [Downing, 2024]. Furthermore, as evidence in a court, this metric would not provide a binary test on which a suit could be decided, rather it would be a piece of a batch of evidence, in which some is more probative than others. Our hope is to provide regulators, model owners, and the courts a mechanism to measure the extent to which a model contains a particular string within its weights and make discussion about data usage more grounded and quantitative.
References
- Nicholas Carlini, Chang Liu, Úlfar Erlingsson, Jernej Kos, and Dawn Song. The secret sharer: Evaluating and testing unintended memorization in neural networks. In 28th USENIX security symposium (USENIX security 19), pages 267–284, 2019.
- Nicholas Carlini, Steve Chien, Milad Nasr, Shuang Song, Andreas Terzis, and Florian Tramer. Membership inference attacks from first principles. arXiv preprint arXiv:2112.03570, 2021.
- Nicholas Carlini, Daphne Ippolito, Matthew Jagielski, Katherine Lee, Florian Tramer, and Chiyuan Zhang. Quantifying memorization across neural language models, 2023.
- Dami Choi, Yonadav Shavit, and David K Duvenaud. Tools for verifying neural models’ training data. Advances in Neural Information Processing Systems, 36, 2024.
- A Feder Cooper, Katherine Lee, James Grimmelmann, Daphne Ippolito, Christo- pher Callison-Burch, Christopher A Choquette-Choo, Niloofar Mireshghallah, Miles Brundage, David Mimno, Madiha Zahrah Choksi, et al. Report of the 1st workshop on generative ai and law. arXiv preprint arXiv:2311.06477, 2023.
- Grégoire Delétang, Anian Ruoss, Paul-Ambroise Duquenne, Elliot Catt, Tim Genewein, Christopher Mattern, Jordi Grau-Moya, Li Kevin Wenliang, Matthew Aitchison, Laurent Orseau, et al. Language modeling is compression. arXiv preprint arXiv:2309.10668, 2023.
- Kate Downing. Copyright fundamentals for AI researchers. In Proceedings of the Twelfth International Conference on Learning Representations (ICLR), 2024. URL https://iclr.cc/media/iclr-2024/Slides/21804.pdf.
- Jean-Loup Gailly and Mark Adler. gzip. https://www.gnu.org/software/gzip/, 1992. Accessed: 2024-05-21.
- Cade Metz and Katie Robertson. Openai seeks to dismiss parts of the new york times’s lawsuit. The New York Times, 2024. URL https://www.nytimes.com/2024/02/27/ technology/openai-new-york-times-lawsuit.html#: ̃:text=In%20its%20suit% 2C%20The%20Times,someone%20to%20hack%20their%20chatbot.
- Milad Nasr, Nicholas Carlini, Jonathan Hayase, Matthew Jagielski, A Feder Cooper, Daphne Ippolito, Christopher A Choquette-Choo, Eric Wallace, Florian Tram`er, and Katherine Lee. Scalable extraction of training data from (production) language models. arXiv preprint arXiv:2311.17035, 2023.
- Martin Pawelczyk, Seth Neel, and Himabindu Lakkaraju. In-context unlearning: Language models as few shot unlearners. arXiv preprint arXiv:2310.07579, 2023.
- Salvatore Sanfilippo. Smaz: Small strings compression library. https://github.com/ antirez/smaz, 2006. Accessed: 2024-05-21.
- Reza Shokri, Marco Stronati, Congzheng Song, and Vitaly Shmatikov. Membership inference attacks against machine learning models. In 2017 IEEE symposium on security and privacy (SP), pages 3–18. IEEE, 2017.
- Chiyuan Zhang, Daphne Ippolito, Katherine Lee, Matthew Jagielski, Florian Tramèr, and Nicholas Carlini. Counterfactual memorization in neural language models. Advances in Neural Information Processing Systems, 36:39321–39362, 2023.
Footnotes
Build a RAG-based QnA application using Llama3 models from SageMaker JumpStart
Organizations generate vast amounts of data that is proprietary to them, and it’s critical to get insights out of the data for better business outcomes. Generative AI and foundation models (FMs) play an important role in creating applications using an organization’s data that improve customer experiences and employee productivity.
The FMs are typically pretrained on a large corpus of data that’s openly available on the internet. They perform well at natural language understanding tasks such as summarization, text generation, and question answering on a broad variety of topics. However, they can sometimes hallucinate or produce inaccurate responses when answering questions that they haven’t been trained on. To prevent incorrect responses and improve response accuracy, a technique called Retrieval Augmented Generation (RAG) is used to provide models with contextual data.
In this post, we provide a step-by-step guide for creating an enterprise ready RAG application such as a question answering bot. We use the Llama3-8B FM for text generation and the BGE Large EN v1.5 text embedding model for generating embeddings from Amazon SageMaker JumpStart. We also showcase how you can use FAISS as an embeddings store and packages such as LangChain for interfacing with the components and run inferences within a SageMaker Studio notebook.
SageMaker JumpStart
SageMaker JumpStart is a powerful feature within the Amazon SageMaker ML platform that provides ML practitioners a comprehensive hub of publicly available and proprietary foundation models.
Llama 3 overview
Llama 3 (developed by Meta) comes in two parameter sizes—8B and 70B with 8K context length—that can support a broad range of use cases with improvements in reasoning, code generation, and instruction following. Llama 3 uses a decoder-only transformer architecture and new tokenizer that provides improved model performance with 128K size. In addition, Meta improved post-training procedures that substantially reduced false refusal rates, improved alignment, and increased diversity in model responses.
BGE Large overview
The embedding model BGE Large stands for BAAI general embedding large. It’s developed by BAAI and is designed to enhance retrieval capabilities within large language models (LLMs). The model supports three retrieval methods:
- Dense retrieval (BGE-M3)
- Lexical retrieval (LLM Embedder)
- Multi-vector retrieval (BGE Embedding Reranker).
You can use the BGE embedding model to retrieve relevant documents and then use the BGE reranker to obtain final results.
On Hugging Face, the Massive Text Embedding Benchmark (MTEB) is provided as a leaderboard for diverse text embedding tasks. It currently provides 129 benchmarking datasets across 8 different tasks on 113 languages. The top text embedding models from the MTEB leaderboard are made available from SageMaker JumpStart, including BGE Large.
For more details about this model, see the official Hugging Face mode card page.
RAG overview
Retrieval-Augmented Generation (RAG) is a technique that enables the integration of external knowledge sources with FM. RAG involves three main steps: retrieval, augmentation, and generation.
First, relevant content is retrieved from an external knowledge base based on the user’s query. Next, this retrieved information is combined or augmented with the user’s original input, creating an augmented prompt. Finally, the FM processes this augmented prompt, which includes both the query and the retrieved contextual information, and generates a response tailored to the specific context, incorporating the relevant knowledge from the external source.
Solution overview
You will construct a RAG QnA system on a SageMaker notebook using the Llama3-8B model and BGE Large embedding model. The following diagram illustrates the step-by-step architecture of this solution, which is described in the following sections.
Implementing this solution takes three high level steps: Deploying models, data processing and vectorization, and running inferences.
To demonstrate this solution, a sample notebook is available in the GitHub repo.
The notebook is powered by an ml.t3.medium instance to demonstrate deploying the model as an API endpoint using an SDK through SageMaker JumpStart. You can use these model endpoints to explore, experiment, and optimize for comparing advanced RAG application techniques using LangChain. We also illustrate the integration of the FAISS embeddings store into the RAG workflow, highlighting its role in storing and retrieving embeddings to enhance the application’s performance.
We will also discuss how you can use LangChain to create effective and more efficient RAG applications. LangChain is a Python library designed to build applications with LLMs. It provides a modular and flexible framework for combining LLMs with other components, such as knowledge bases, retrieval systems, and other AI tools, to create powerful and customizable applications.
After everything is set up, when a user interacts with the QnA application, the flow is as follows:
- The user sends a query using the QnA application.
- The application sends the user query to the vector database to find similar documents.
- The documents returned as a context are captured by the QnA application.
- The QnA application submits a request to the SageMaker JumpStart model endpoint with the user query and context returned from the vector database.
- The endpoint sends the request to the SageMaker JumpStart model.
- The LLM processes the request and generates an appropriate response.
- The response is captured by the QnA application and displayed to the user.
Prerequisites
To implement this solution, you need the following:
- An AWS account with privileges to create AWS Identity and Access Management (IAM) roles and policies. For more information, see Overview of access management: Permissions and policies.
- Basic familiarity with SageMaker and AWS services that support LLMs.
- The Jupyter Notebooks needs ml.t3.medium.
- You need access to accelerated instances (GPUs) for hosting the LLMs. This solution needs access to a minimum of the following instance sizes:
- ml.g5.12xlarge for endpoint use when deploying the BGE Large En v1.5 text embedding model
- ml.g5.2xlarge for endpoint use when deploying the Llama-3-8B model endpoint
To increase your quota, refer to Requesting a quota increase.
Prompt template for Llama3
While both Llama 2 and Llama 3 are powerful language models that are optimized for dialogue-based tasks, their prompting formats differ significantly in how they handle multi-turn conversations, specify roles, and mark message boundaries, reflecting distinct design choices and trade-offs.
Llama 3 prompting format: Llama 3 employs a structured format designed for multi-turn conversations involving different roles (system, user, and assistant). It uses dedicated tokens to explicitly mark roles, message boundaries, and the end of the prompt:
- Placeholder tokens:
{{user_message}}
and{{assistant_message}}
- Role marking:
<|start_header_id|>{role}<|end_header_id|>
- Message boundaries:
<|eot_id|>
signals end of a message within a turn. - Prompt End Marker:
<|start_header_id|>assistant<|end_header_id|>
signals start of assistant’s response.
Llama 2 prompting format: Llama 2 uses a more compact representation with different tokens for handling conversations:
- User message enclosure:
[INST][/INST]
- Start and end of sequence:
<s></s>
- System message enclosure:
<<SYS>><</SYS>>
- Message separation:
<s></s>
separates user messages and model responses.
Key differences:
- Role specification: Llama 3 uses a more explicit approach with dedicated tokens, while Llama 2 relies on enclosing tags.
- Message boundary marking: Llama 3 uses
<|eot_id|>
, Llama 2 uses<s></s>
. - Prompt end marker: Llama 3 uses
<|start_header_id|>assistant<|end_header_id|>
, Llama 2 uses[/INST] and </s>
.
The choice depends on the use case and integration requirements. Llama 3’s format is more structured and role-aware and is better suited for conversational AI applications with complex multi-turn conversations. Llama 2’s format, while more compact, might be less explicit in handling roles and message boundaries.
Implement the solution
To implement the solution, you’ll use the following steps:
- Set up a SageMaker Studio notebook
- Deploy models on Amazon SageMaker JumpStart
- Set up Llama3-8b and BGE Large En v1.5 models with LangChain
- Prepare data and generate embeddings
- Load documents of different kind and generate embeddings to create a vector store
- Retrieve documents to the question using the following approaches from LangChain
- Regular Retrieval Chain
- Parent Document Retriever Chain
- Prepare a prompt that goes as input to the LLM and presents an answer in a human friendly manner
Set up a SageMaker Studio notebook
To follow the code in this post:
- Open SageMaker Studio and clone the following GitHub repository.
- Open the notebook RAG-recipes/llama3-rag-langchain-smjs.ipynb and choose the PyTorch 2.0.0 Python 3.10 GPU Optimized image, Python 3 kernel, and
ml.t3.medium
as the instance type. - If this is your first time using SageMaker Studio notebooks, see Create or Open an Amazon SageMaker Studio Notebook.
To set up the development environment, you need to install the necessary Python libraries, as demonstrated in the following code. The example notebook provided includes these commands:
After the libraries are written in requirement.txt
, install all the libraries:
Deploy pretrained models
After you’ve imported the required libraries, you can deploy the Llama 3 8B Instruct
LLM model on SageMaker JumpStart using the SageMaker SDK:
- Import the
JumpStartModel
class from the SageMaker JumpStart library - Specify the model ID for the HuggingFace
Llama 3 8b Instruct
LLM model, and deploy the model. - Specify the model ID for the HuggingFace BGE Large EN embedding model and deploy the model.
Set up models with LangChain
For this step, you’ll use the following code to set up models.
- Replace the endpoint names in the below code snippet with the endpoint names that are deployed in your environment. You can get the endpoint names from predictors created in the previous section or view the endpoints created by going to SageMaker Studio, left navigation deployments → endpoints and replace the values for
llm_endpoint_name
andembedding_endpoint_name
. - Transform input and output data to process API calls for
Llama 3 8B Instruct
on Amazon SageMaker. - Instantiate the LLM with SageMaker and LangChain
- Transform input and output data to process API calls for
BGE Large En
on SageMaker - Instantiate the embedding model with SageMaker and LangChain
Prepare data and generate embeddings
In this example, you will use several years of Amazon’s Annual Reports (SEC filings) for investors as a text corpus to perform QnA on.
- Start by using the following code to download the PDF documents from the provided URLs and create a list of metadata for each downloaded document.
If you look at the Amazon 10-Ks, the first four pages are all the very similar and might skew the responses if they are kept in the embeddings. This will cause repetition, take longer to generate embeddings, and might skew your results.
- In the next step, you will take the downloaded data, trim the 10-K (first four pages) and overwrite them as processed files.
- After downloading, you can load the documents with the help of DirectoryLoader from PyPDF available under LangChain and splitting them into smaller chunks. Note: The retrieved document or text should be large enough to contain enough information to answer a question; but small enough to fit into the LLM prompt. Also, the embedding model has a limit on the length of input tokens of 512 tokens, which translates to approximately 2,000 characters. For this use-case, you are creating chunks of approximately 1,000 characters with an overlap of 100 characters using RecursiveCharacterTextSplitter.
- Before you proceed, look at some of the statistics regarding the document preprocessing you just performed:
- You started with four PDF documents, which have been split into approximately 500 smaller chunks. Now you can see how a sample embedding would look like for one of those chunks.
This can be done using FAISS implementation inside LangChain which takes input from the embedding model and the documents to create the entire vector store. Using the Index Wrapper, you can abstract away most of the heavy lifting such as creating the prompt, getting embeddings of the query, sampling the relevant documents, and calling the LLM. VectorStoreIndexWrapper.
Answer questions using a LangChain vector store wrapper
You use the wrapper provided by LangChain, which wraps around the vector store and takes input from the LLM. This wrapper performs the following steps behind the scenes:
- Inputs the question
- Creates question embedding
- Fetches relevant documents
- Stuffs the documents and the question into a prompt
- Invokes the model with the prompt and generate the answer in a human readable manner.
Note: In this example we are using Llama 3 8B Instruct
as the LLM under Amazon SageMaker, this particular model performs best if the inputs are provided under
<|begin_of_text|><|start_header_id|>system<|end_header_id|>,
{{system_message}},
<|eot_id|><|start_header_id|>user<|end_header_id|>,
{{user_message}}
, and the model is requested to generate an output after<|eot_id|><|start_header_id|>assistant<|end_header_id|>
.
The following is an example of how to control the prompt so that the LLM stays grounded and doesn’t answer outside the context.
You can ask another question.
Retrieval QA chain
We’ve shown you a basic method to get context-aware answers. Now, let’s look at a more customizable option with RetrievalQA. You can customize how fetched documents are added to the prompt using the chain_type
parameter, control the number of relevant documents retrieved by changing the k parameter, and get source documents used by the LLM by enabling return_source_documents.RetrievalQA
also allows providing custom prompt templates specific to the model.
You can then ask a question:
Parent document retriever chain
Let’s explore a more advanced RAG option with ParentDocumentRetriever. It balances storing small chunks for accurate embeddings and larger chunks to preserve context. First, a parent_splitter
divides documents into larger parent chunks. Then, a child_splitter
creates smaller child chunks. Child chunks are indexed in a vector store using embeddings for efficient retrieval. To retrieve relevant info, ParentDocumentRetriever
fetches child chunks from the vector store, looks up their parent IDs, and returns corresponding larger parent chunks, stored in an InMemoryStore. This approach balances accurate embeddings with contextual information for meaningful retrieval.
- Sometimes, the full documents can so large that you don’t want to retrieve them as is. In that case, you can first split the raw documents into larger chunks, and then split it into smaller chunks. You then index the smaller chunks, but on retrieval you retrieve the larger chunks (but still not the full documents).
- Now, initialize the chain using the
ParentDocumentRetriever
. Pass the prompt in using thechain_type_kwargs
argument. - Start asking questions:
Clean up
To avoid incurring unnecessary costs, when you’re done, delete the SageMaker endpoints and OpenSearch Service domain, either using the following code snippets or the SageMaker JumpStart UI.
To use the SageMaker console, complete the following steps:
- On the SageMaker console, under Inference in the navigation pane, choose Endpoints.
- Search for the embedding and text generation endpoints.
- On the endpoint details page, choose Delete.
- Choose Delete again to confirm.
Conclusion
In this post, we showed you a powerful RAG solution using SageMaker JumpStart to deploy the Llama 3 8B Instruct model and the BGE Large En v1.5 embedding model.
We showed you how to create a robust vector store by processing documents of various formats and generating embeddings. This vector store facilitates retrieving relevant documents based on user queries using LangChain’s retrieval algorithms. We demonstrated the ability to prepare custom prompts tailored for the Llama 3 model, ensuring context-aware responses, and presented these context-specific answers in a human-friendly manner.
This solution highlights the power of SageMaker JumpStart in deploying cutting-edge models and the versatility of LangChain in creating effective RAG applications. By seamlessly integrating these components, we enabled high-quality, context-specific response generation, enhancing the Llama 3 model’s performance across natural language processing tasks. To explore this solution and embark on your context-aware language generation journey, visit the notebook in the GitHub repository.
To get started now, check out SageMaker JumpStart in SageMaker Studio.
- SageMaker JumpStart documentation
- SageMaker JumpStart Foundation Models documentation
- SageMaker JumpStart product detail page
- SageMaker JumpStart model catalog
About the Authors
Supriya Puragundla is a Senior Solutions Architect at AWS. She has over 15 years of IT experience in software development, design and architecture. She helps key enterprise customer accounts on their data, generative AI and AI/ML journeys. She is passionate about data-driven AI and the area of depth in ML and generative AI.
Dr. Farooq Sabir is a Senior Artificial Intelligence and Machine Learning Specialist Solutions Architect at AWS. He holds PhD and MS degrees in Electrical Engineering from the University of Texas at Austin and an MS in Computer Science from Georgia Institute of Technology. He has over 15 years of work experience and also likes to teach and mentor college students. At AWS, he helps customers formulate and solve their business problems in data science, machine learning, computer vision, artificial intelligence, numerical optimization, and related domains. Based in Dallas, Texas, he and his family love to travel and go on long road trips.
Marco Punio is a Sr. Specialist Solutions Architect focused on generative AI strategy, applied AI solutions, and conducting research to help customers hyperscale on AWS. Marco is based in Seattle, WA, and enjoys writing, reading, exercising, and building applications in his free time.
Niithiyn Vijeaswaran is a Solutions Architect at AWS. His area of focus is generative AI and AWS AI Accelerators. He holds a Bachelor’s degree in Computer Science and Bioinformatics. Niithiyn works closely with the Generative AI GTM team to enable AWS customers on multiple fronts and accelerate their adoption of generative AI. He’s an avid fan of the Dallas Mavericks and enjoys collecting sneakers.
Yousuf Athar is a Solutions Architect at AWS specializing in generative AI and AI/ML. With a Bachelor’s degree in Information Technology and a concentration in Cloud Computing, he helps customers integrate advanced generative AI capabilities into their systems, driving innovation and competitive edge. Outside of work, Yousuf loves to travel, watch sports, and play football.
Gaurav Parekh is an AWS Solutions Architect specializing in Generative AI, Analytics and Networking technologies.