A number of organizations use Gmail for their business email needs. Gmail for business is part of Google Workspace, which provides a set of productivity and collaboration tools like Google Drive, Gmail, and Google Calendar. Google Drive supports storing documents such as Emails contain a wealth of information found in different places, such as within the subject of an email, the message content, or even attachments. Performing an intelligent search on emails with co-workers can help you find answers to questions, improving productivity and enhancing the overall customer experience for the organization.
Amazon Q Business is a fully managed, generative AI-powered assistant designed to enhance enterprise operations. It can be tailored to specific business needs by connecting to company data, information, and systems through over 40 built-in connectors.
Amazon Q Business enables users in various roles, such as marketers, project managers, and sales representatives, to have tailored conversations, solve problems, generate content, take action, and more, all through a web-based interface. This tool aims to make employees work smarter, move faster, and drive more significant impact by providing immediate and relevant information and streamlining tasks.
With the Gmail connector for Amazon Q Business, you can enhance productivity and streamline communication processes within your organization. This integration empowers you to use advanced search capabilities and intelligent email management using natural language.
In this post, we guide you through the process of setting up the Gmail connector, enabling seamless interaction between Gmail and Amazon Q Business. Whether you’re a small startup or a large enterprise, this solution can help you maximize the potential of your Gmail data and empower your team with actionable insights.
Finding accurate answers from content in Gmail mailbox using Amazon Q Business
After you integrate Amazon Q Business with Gmail, you can ask a question and Amazon Q Business can index through your mailbox and find relevant answers. For example, you can make the following queries:
- Natural language search – You can search for emails and attachments within your mailbox using natural language, making it effortless to find your desired information without having to remember specific keywords or filters
- Summarization – You can request a concise summary of the conversations and attachments matching your search query, allowing you to quickly grasp the key points without having to manually sift through individual items
- Query clarification – If your query is ambiguous or lacks sufficient context, Amazon Q Business can engage in a dialogue to clarify the intent, so you receive the most relevant and accurate results
Overview of the Gmail connector for Amazon Q Business
To crawl and index contents in Gmail, you can configure the Gmail connector for Amazon Q Business as a data source in your Amazon Q Business application. When you connect Amazon Q Business to a data source and initiate the sync process, Amazon Q Business crawls and indexes documents from the data source into its index.
A data source connector is a mechanism for integrating and synchronizing data from multiple repositories into one container index. A data source is a data repository or location that Amazon Q Business connects to in order to retrieve your email data. After you set up the connector, you can create one or multiple data sources within Amazon Q Business and configure them to start indexing emails from your Gmail account.
Types of documents
Gmail messages can be sorted and stored inside your email inbox using folders and labels.
Let’s looks at what are considered as documents in the context of the Gmail connector for Amazon Q Business. The connector supports the crawling of the following entities in Gmail:
- Email – Each email is considered a single document
- Attachment – Each email attachment is considered a single document
Additionally, supported custom metadata and custom objects are also crawled during the sync process.
The Gmail connector for Amazon Q Business also supports the indexing of a rich set of metadata from the various entities in Gmail. It further provides the ability to map these source metadata fields to Amazon Q index fields for indexing. These field mappings allow you to map Gmail field names to Amazon Q index field names. There are three types of metadata fields that Amazon Q connectors support:
- Default fields – These are required with each document, such as the title, creation date, or author
- Optional fields – These are provided by the data source, and the administrator can optionally choose one or more of these fields if they contain important and relevant information to produce accurate answers
- Custom metadata fields – These are fields created in the data source in addition to what the data source already provides
Refer to Gmail data source connector field mappings for more information.
Authentication
Before we index the content from Gmail, we need to first establish a secure connection between the Gmail connector for Amazon Q Business with your Google service account. To establish a secure connection, we need to authenticate with the data source.
The connector supports authentication using a Google service account. We describe the process of creating an account later in this post. For more information about authentication, see Gmail connector overview.
Secure querying with ACL crawling and identity crawling
Secure querying is when a user runs a query and is returned answers only from documents that the user has access to. To enable users to do secure querying, Amazon Q Business honors the access control lists (ACLs) of the documents. Amazon Q Business does this by first supporting the indexing of ACLs. Indexing documents with ACLs is crucial for maintaining data security, because documents without ACLs are considered public. Additionally, the user’s credentials (email address) are passed along with the query so that answers from documents that are relevant and which user is authorized to access are displayed.
When connecting a Gmail data source, Amazon Q Business crawls the ACL information attached to a document (user and group information) from your Gmail instance. In Gmail, user IDs are mapped to _user_id
. User IDs exist in Gmail on files with set access permissions. They’re mapped from the user emails as the IDs in Gmail.
When a user logs in to a web application to conduct a search, the user’s credentials, such as an email address, need to match what is in the ACL of the document to return results from that document. The web application that the user uses to retrieve answers is connected to an identity provider (IdP) or AWS IAM Identity Center. The user’s credentials from the IdP or IAM Identity Center are referred to here as the federated user credentials. The federated user credentials are passed along with the query so that Amazon Q can return the answers from the documents that this user has access to.
Refer to How Amazon Q Business connector crawls Gmail ACLs for more information.
Solution overview
In the following sections, we demonstrate how to set up the Gmail connector for Amazon Q Business. Then we provide examples of how to use the AI-powered chat interface to gain insights from the connected data source.
In our solution, we index emails from Gmail by configuring the Gmail data source connector. This connector allows you to query your Gmail data using Amazon Q Business as your query engine.
After the configuration is complete, you can configure how often Amazon Q Business should synchronize with your Gmail account to keep up to date with the email content. This process makes sure that your email interactions are systematically updated within Amazon Q Business, enabling you to query and uncover valuable insights from your Gmail data.
The following diagram illustrates the solution architecture. Google Workspace is the data source. Emails and attachments along with the ACL information are passed to Amazon Q Business from the Google workspace. The user submits a query to the Amazon Q Business application. Amazon Q Business retrieves the ACL of the user and provides answers based on the emails and attachments that the user has access to.
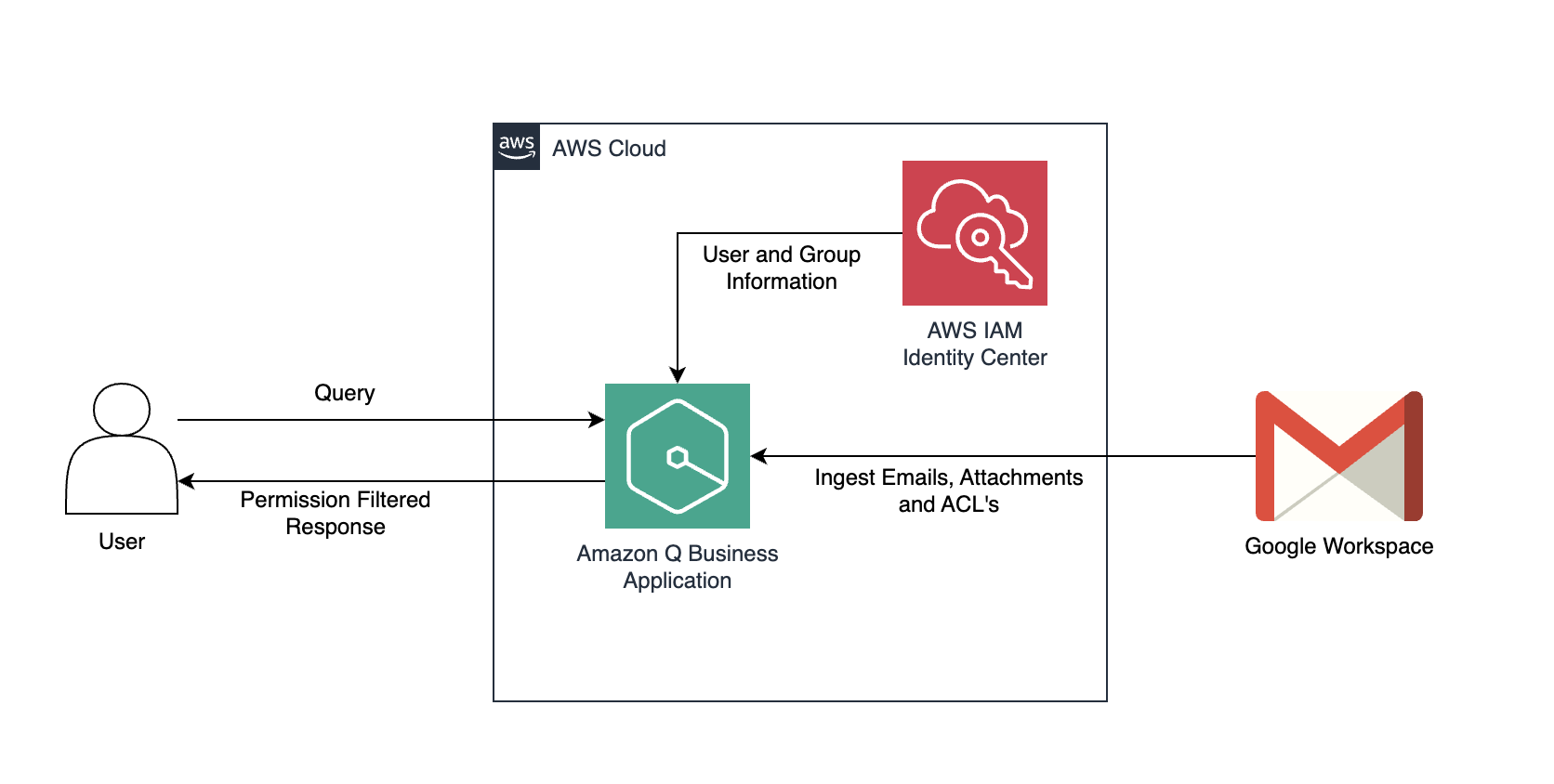
Prerequisites
You should have the following:
Configure the Gmail connector for an Amazon Q Business application
To enable Amazon Q Business to access and index emails from Gmail accounts within the organization, it’s essential to configure the organization’s Google workspace. In the steps that follow, we create a service account that will be used by the Gmail connector for Amazon Q Business to index emails.
We provide the service account with authorization scopes to allow access to the required Gmail APIs. The authorization scopes express the permissions you request users to authorize for your application and are applicable to emails within your organization’s Google workspace.
Complete the following steps:
- Log in to your organization’s Google Cloud account.
- Create a new project with an appropriate name and assign it to your organization. In our example, we name the project
GmailConnector
.
- Choose Create.
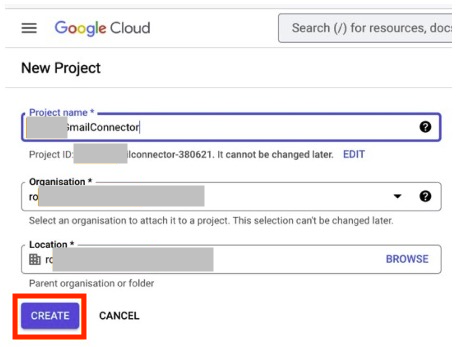
- After you create the project, on the navigation menu, choose APIs and Services and Library to view the API Library.
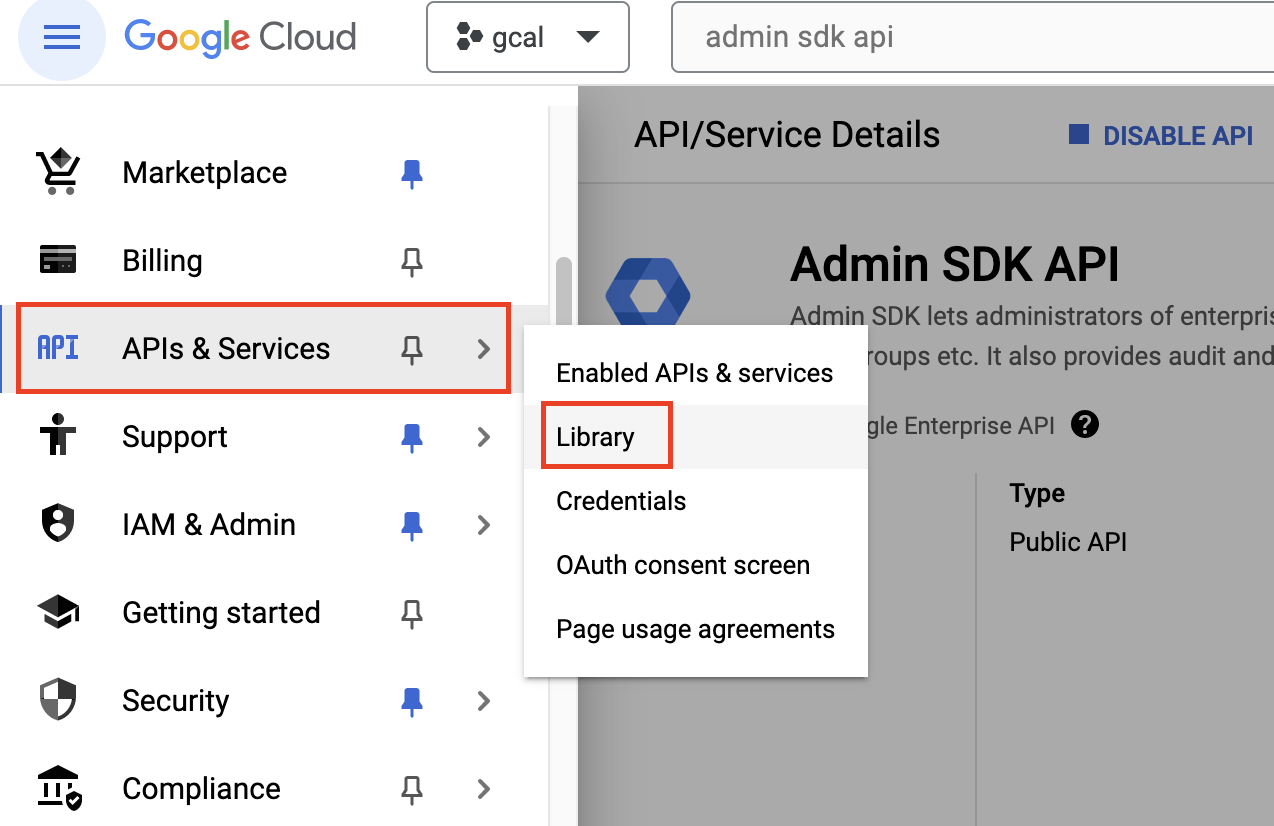
- On the API Library page, search for and choose Admin SDK API.
The Admin SDK API enables managing the Google workspace account resources and audit usage.
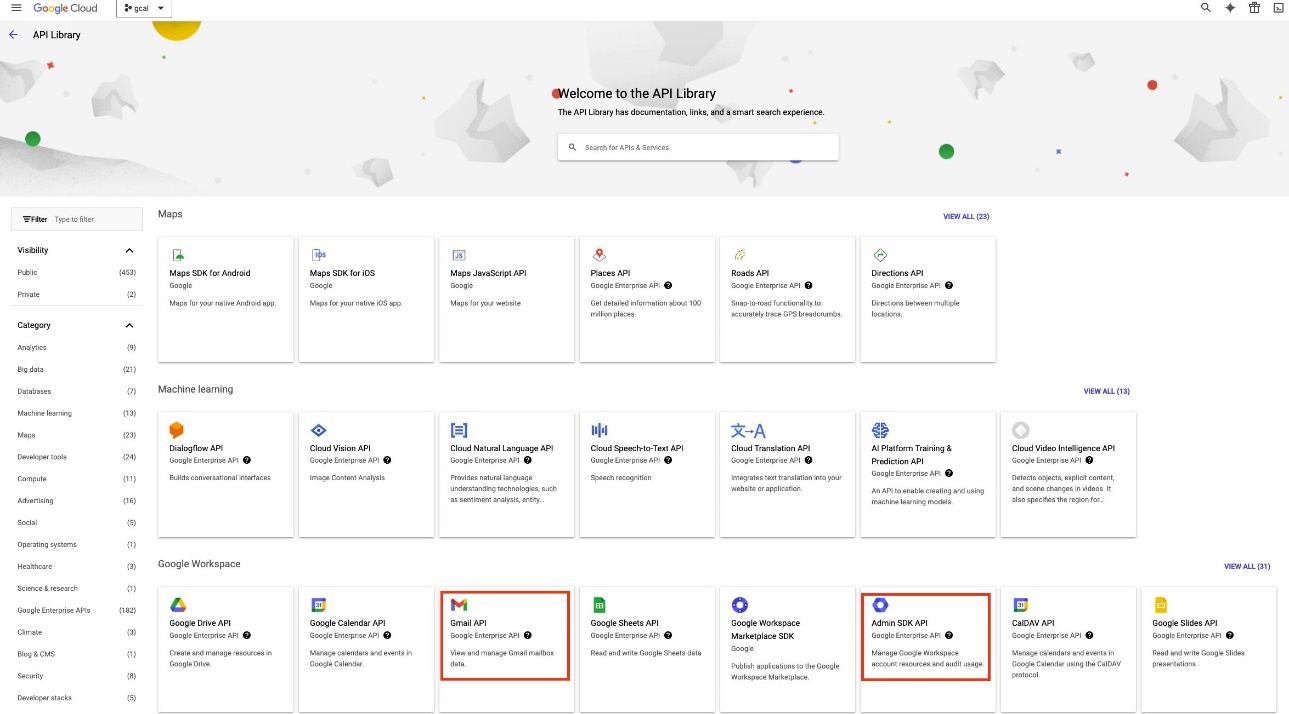
- Choose Enable.
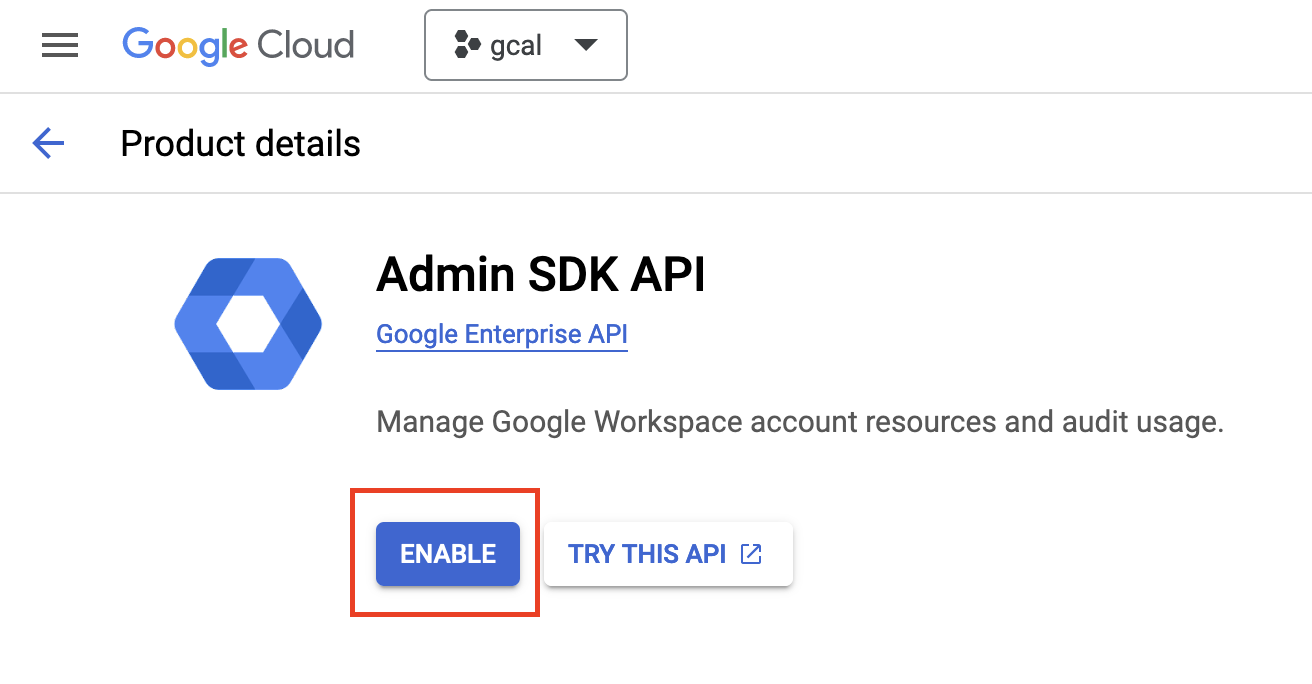
- Similarly, search for the Gmail API on the API Library
The Gmail API can help in viewing and managing the Gmail mailbox data like threads, messages, and labels.
- Choose Enable to enable this API.
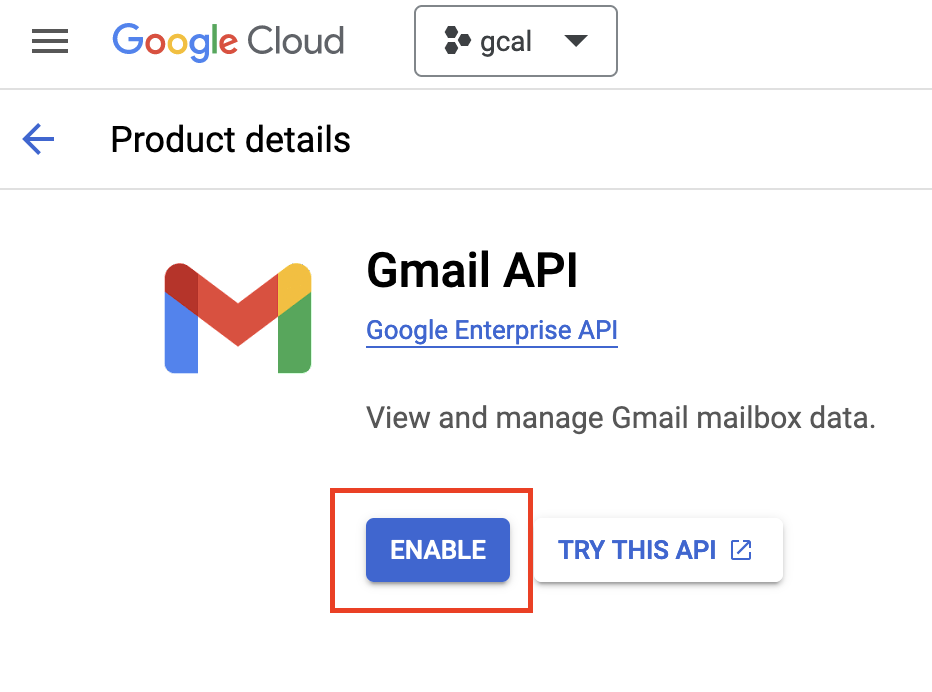
We now create a service account. The service account will be used by the Amazon Q Business Gmail data source connector to access the organization’s emails based on the allowed API scope.
- On the navigation menu, choose IAM and Admin and Service accounts.
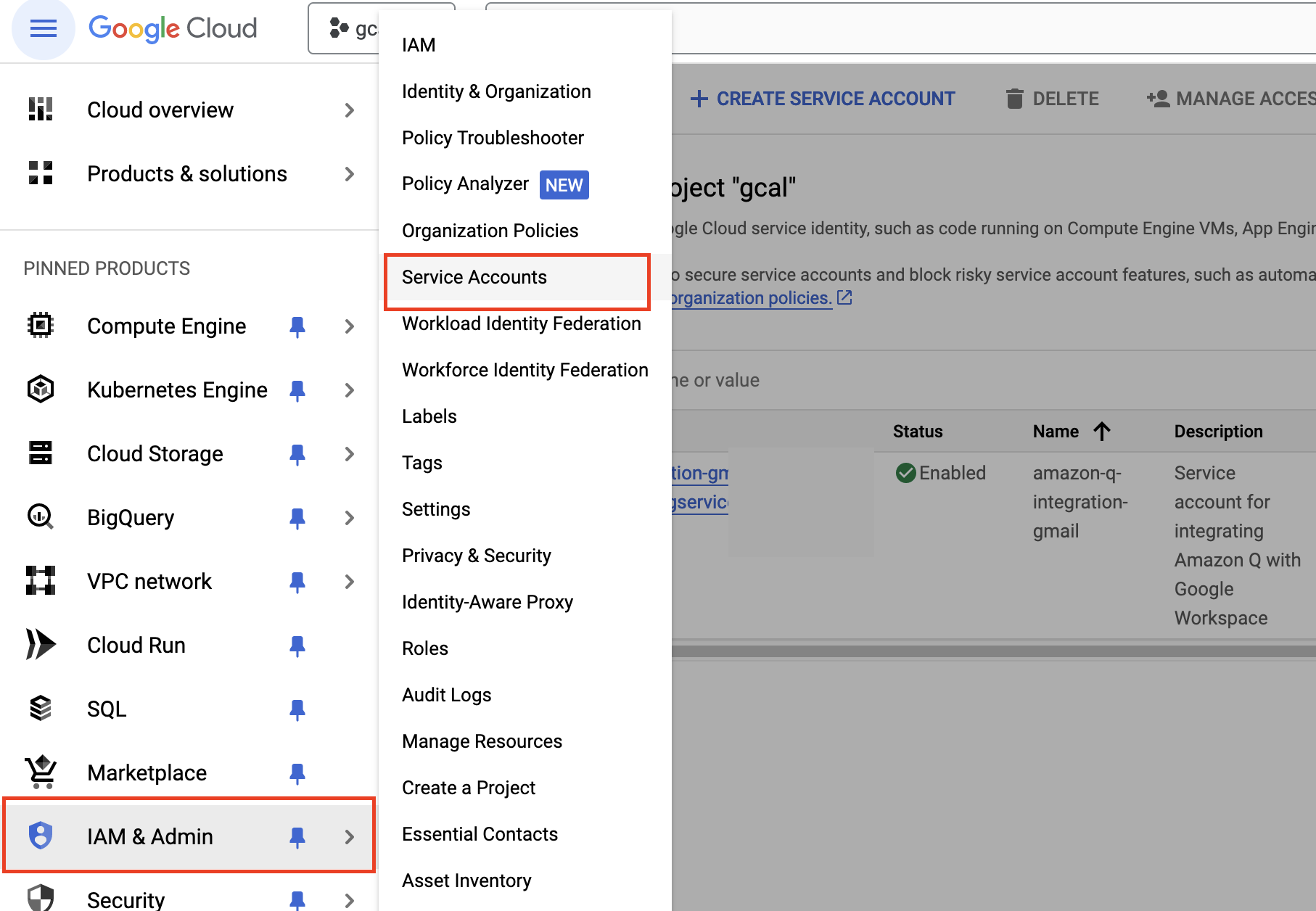
- Choose Create service account.
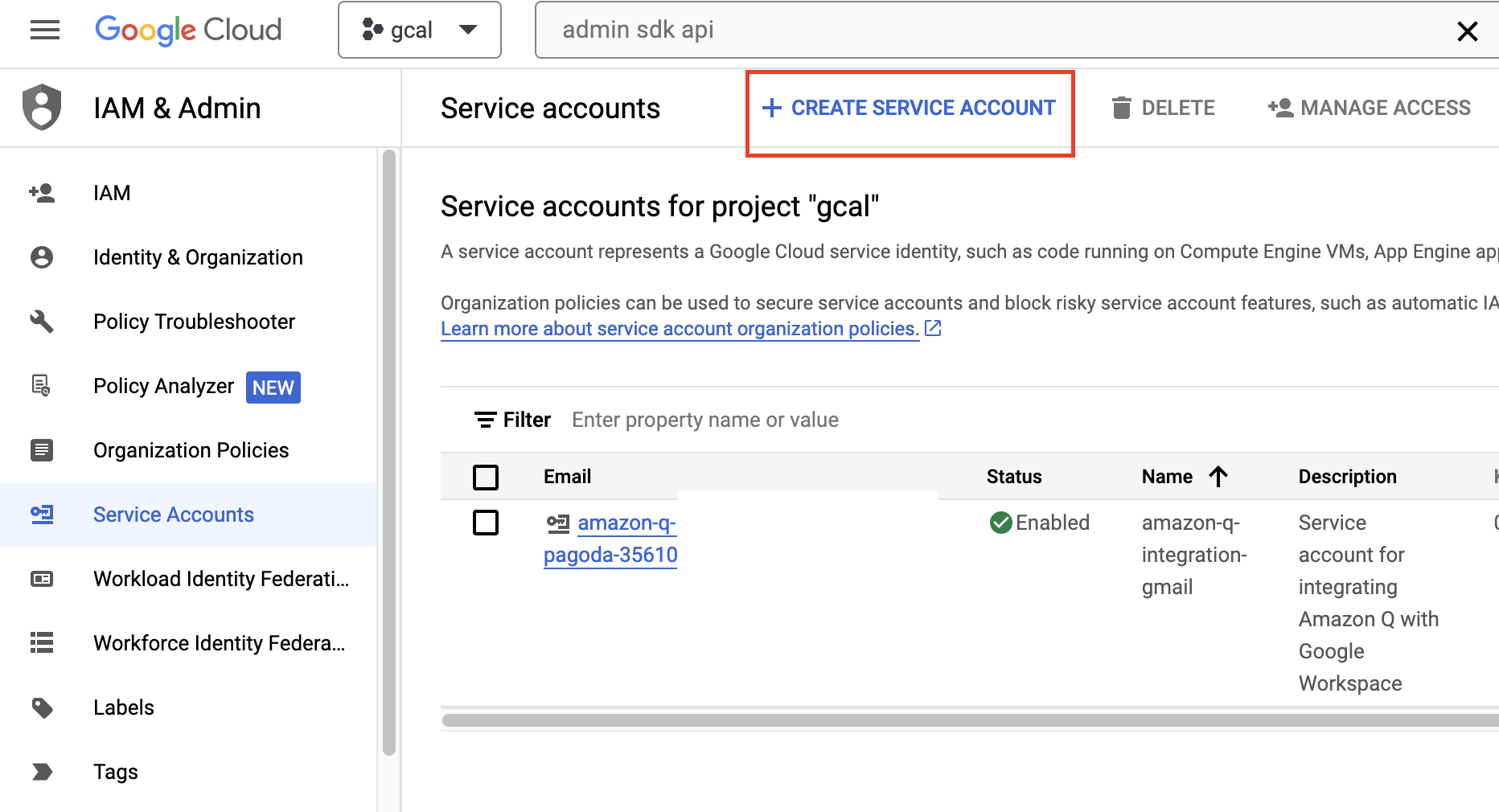
- Name the service account
Amazon-q-integration-gmail
, enter a description, and choose Create and continue.
- Skip the optional sections Grant this service account access to project and Grant users access to this service account.
- Choose Done.
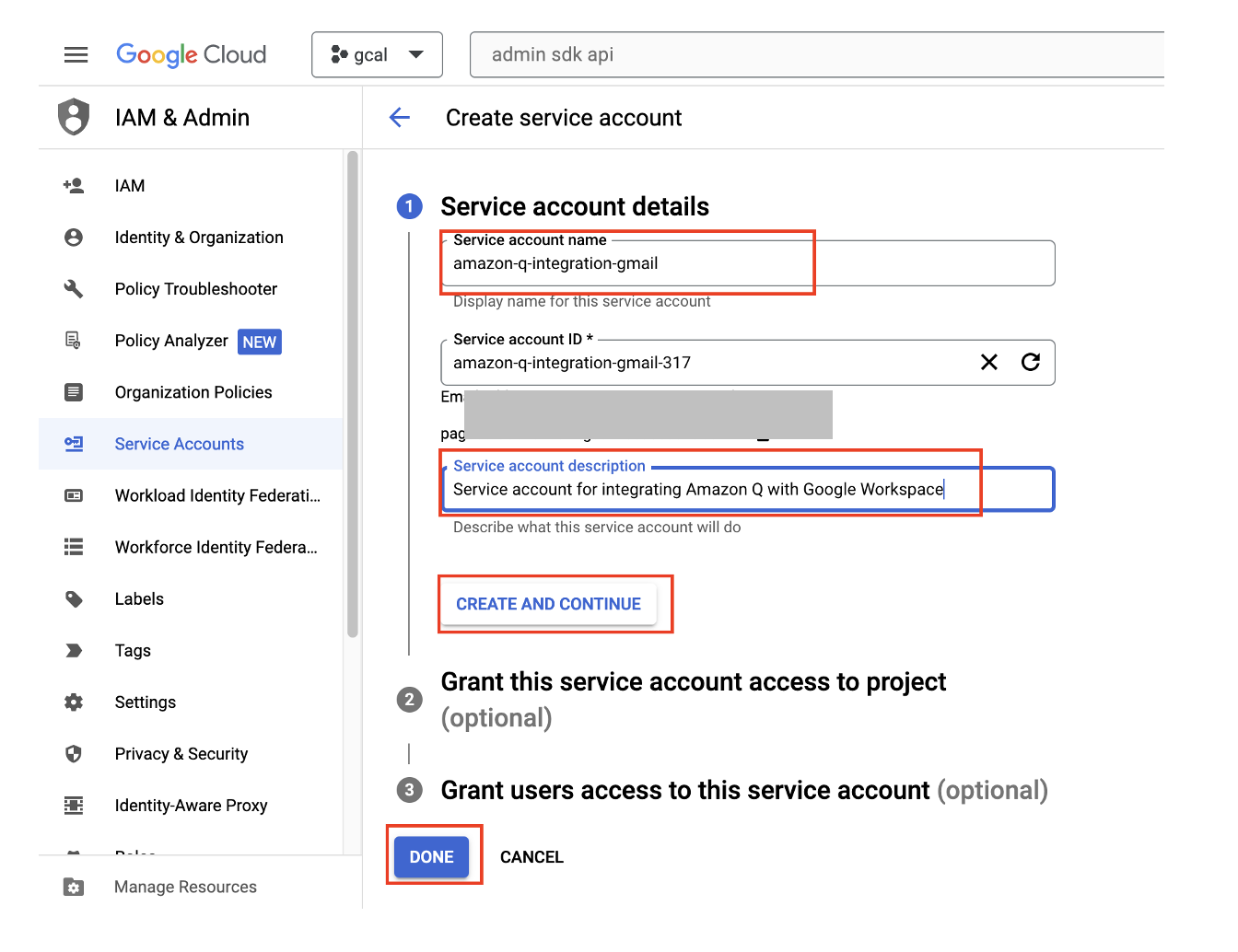
- Choose the service account you created to navigate to the service account details page.
- Note the unique ID for the service account—the unique ID is also known as the client ID, and will be used in later steps.
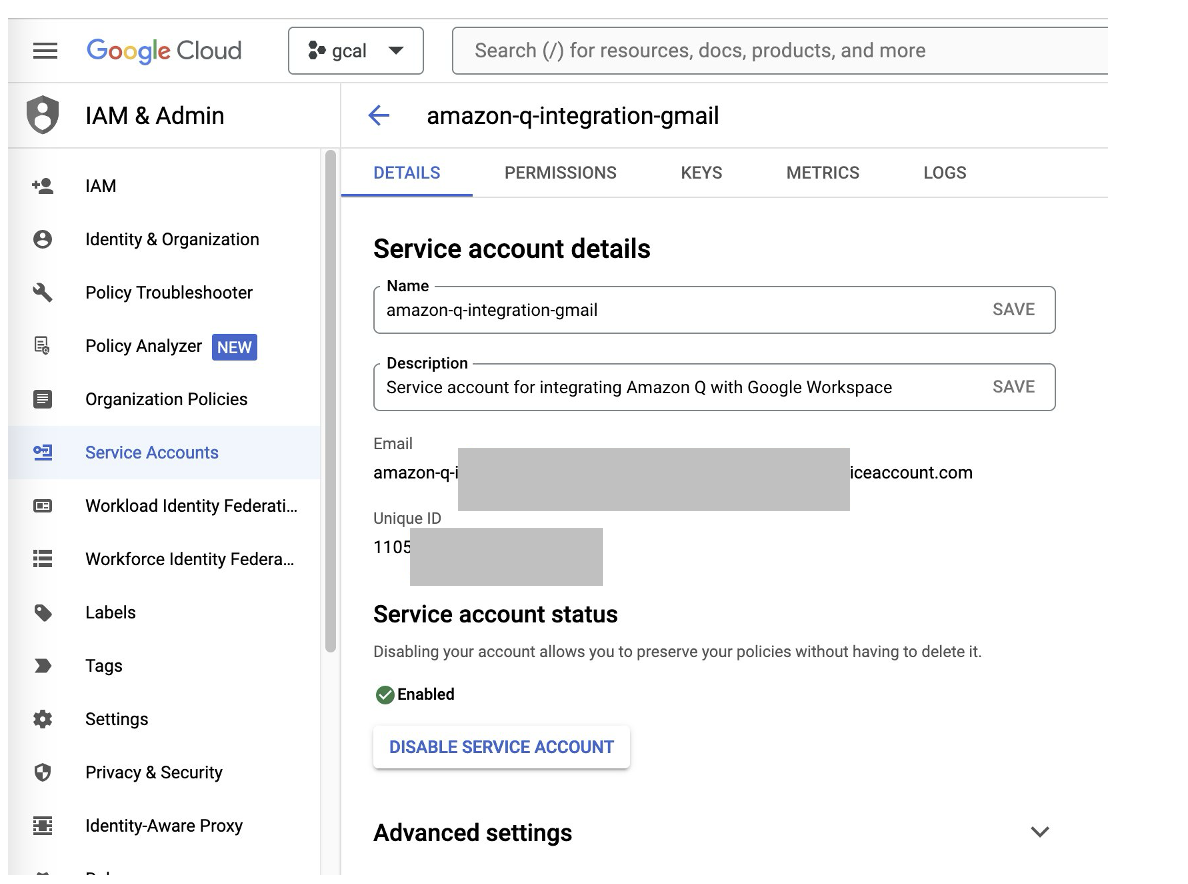
Next, we create the keys for the service account, which will allow it to be used by the Gmail connector for Amazon Q Business.
- On the Keys tab, choose Add key and Create new key.
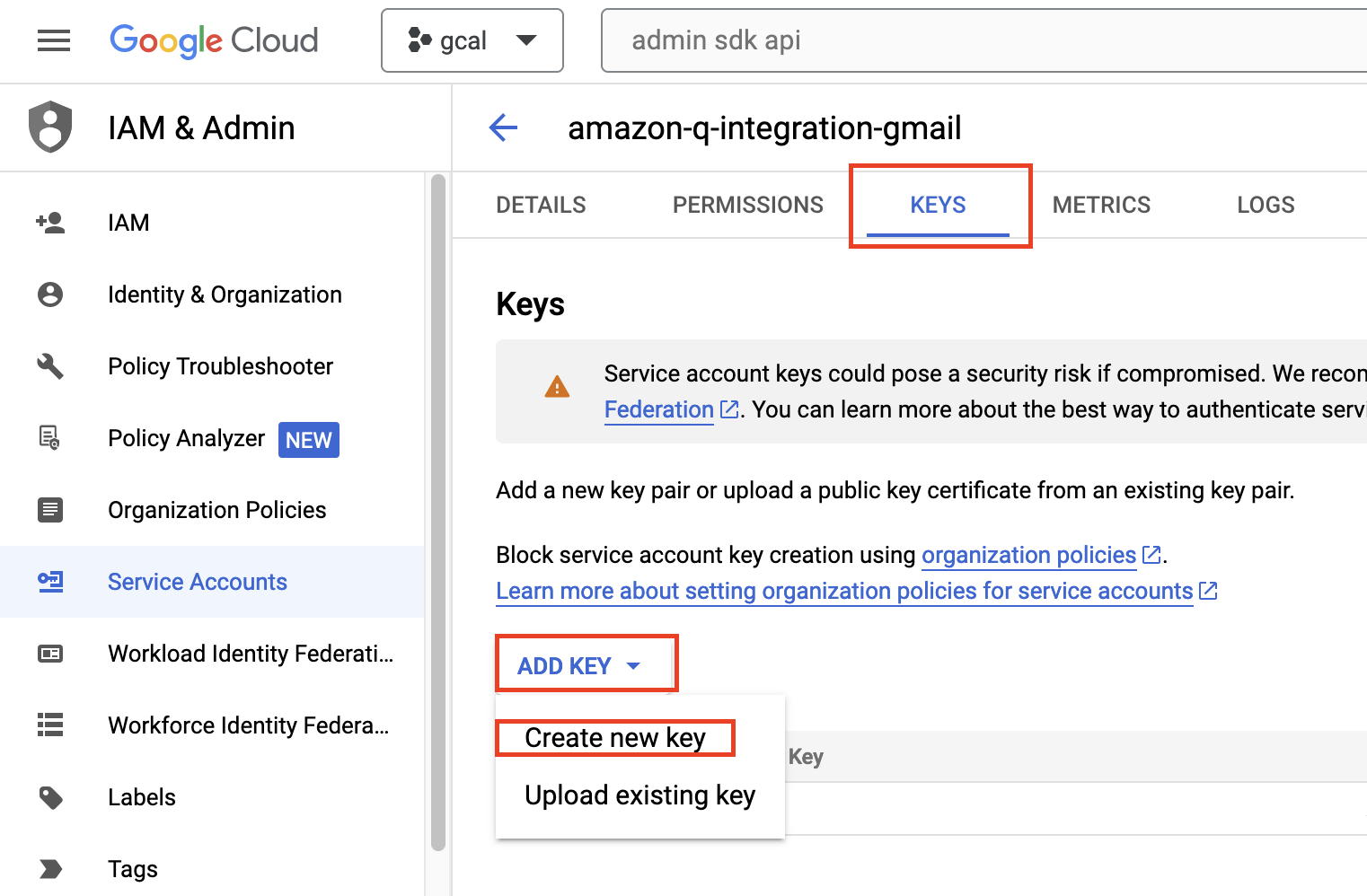
- When prompted for the key type, select the recommended option JSON and choose Create.
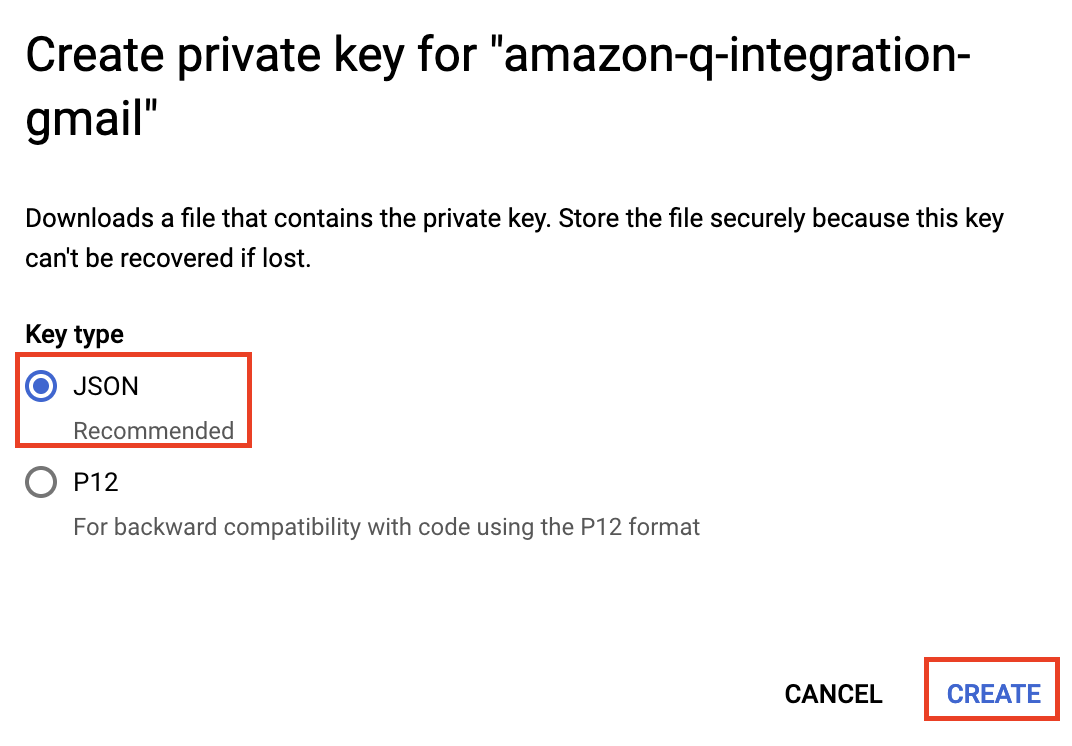
This will download the private key to your computer, which must be kept safe to allow configuration within the Amazon Q console. The following screenshot shows an example of the credentials JSON file.
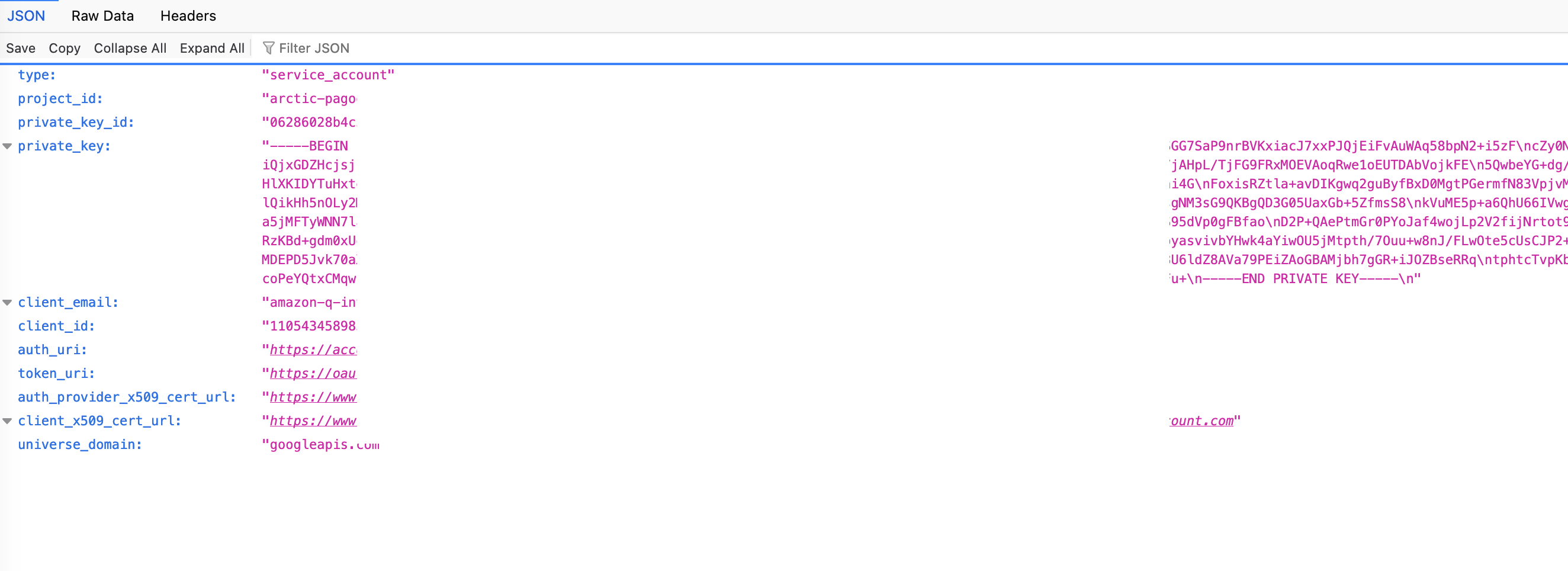
- On the Details tab, expand the Advanced settings section and choose View Google Workspace Admin console in the Domain-wide Delegation
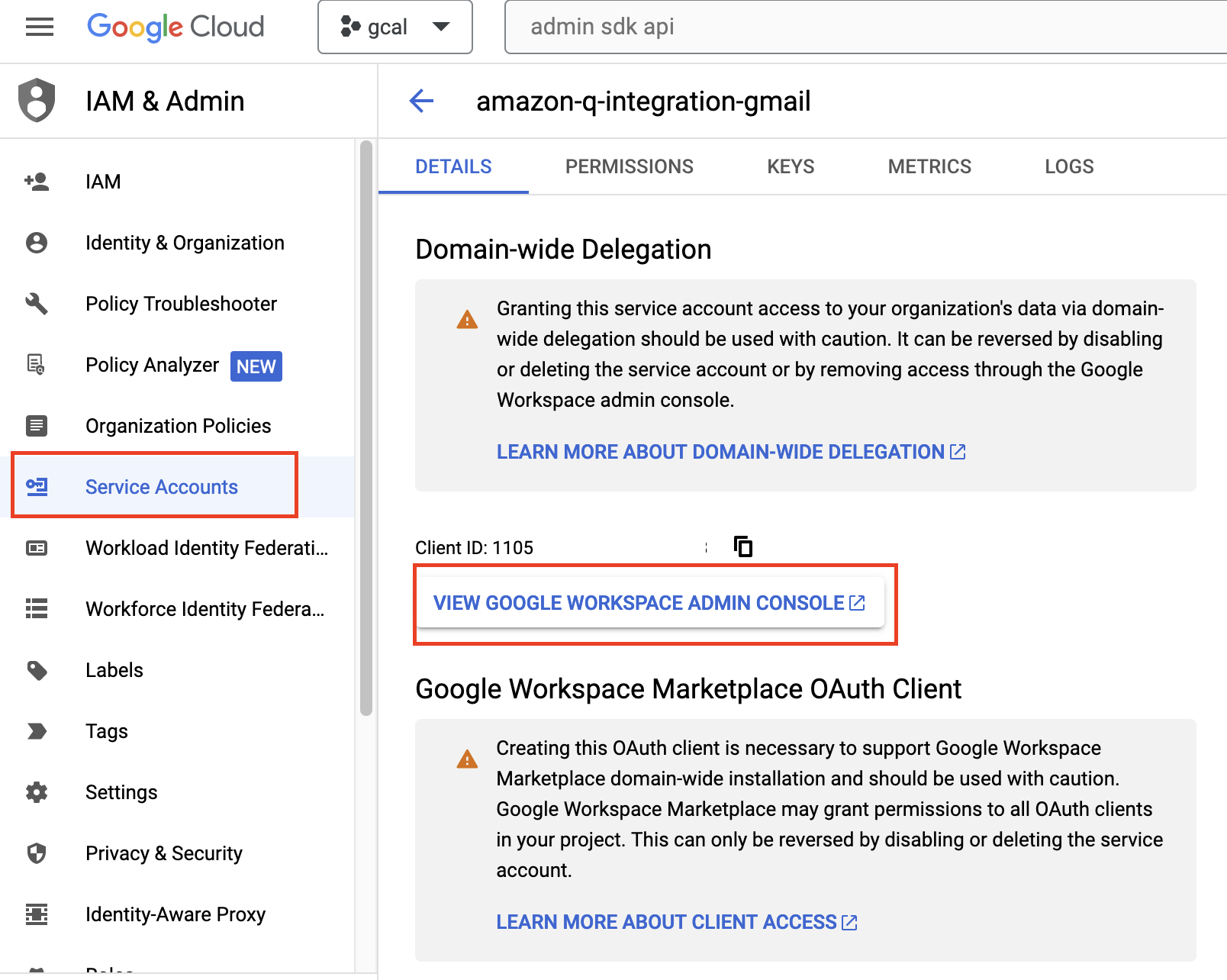
Granting access to the service account using a domain-wide delegation to your organization’s data must be treated as a privileged operation and done with caution. You can reverse the access grant by disabling or deleting the service account or removing access through the Google Workspace Admin console.
- Use the Google Workspace Admin credentials to log in to the Google Workspace Admin console.
- Under Security on the navigation menu, under Access and data control, choose API controls.
- In the Domain-wide delegation section, choose Manage domain-wide delegation.
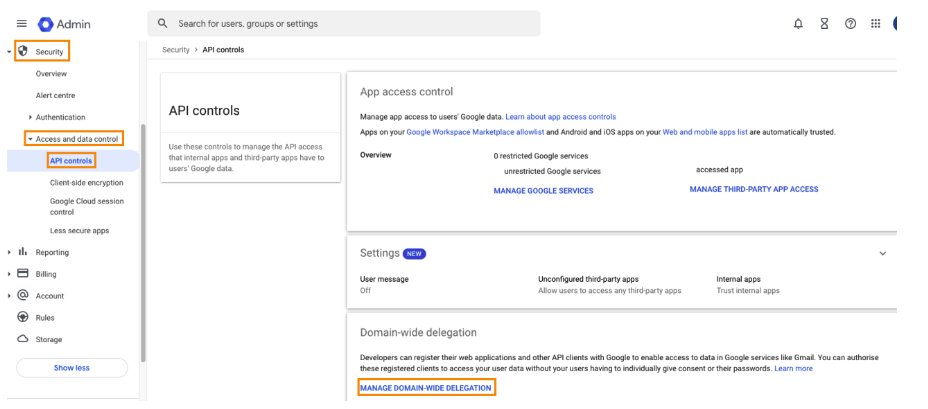
- Choose Add new.
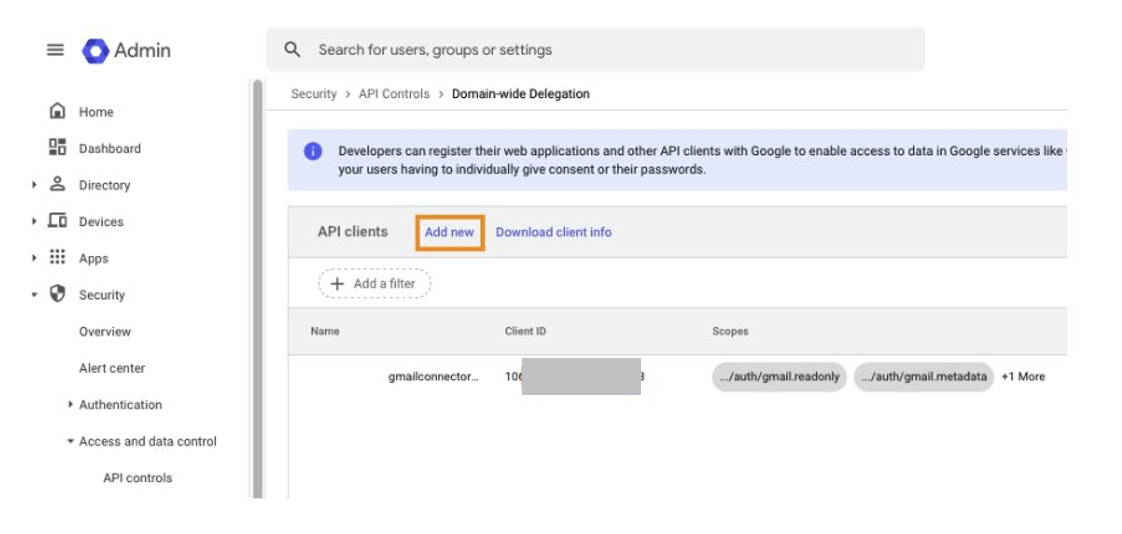
- In the Add a new client ID dialog, enter the unique ID for the service account you created.
- Enter the following scopes to allow the service account to access the emails from Gmail:
- https://www.googleapis.com/auth/gmail.readonly – This scope allows to you to view your email messages and settings.
- https://www.googleapis.com/auth/admin.directory.user.readonly – This scope allows to see and download your organization’s Google Workspace directory.
For more details about all the scopes available, refer to OAuth 2.0 Scopes for Google APIs.
- Choose Authorize.
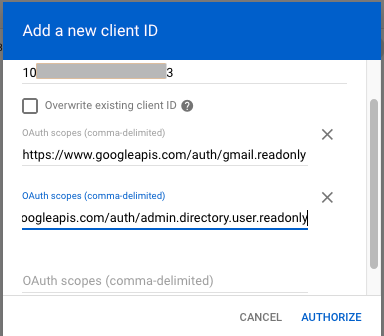
This concludes the configuration within the Google Cloud console and Google Workspace Admin console.
Create the Gmail connector for an Amazon Q Business application
This post assumes that an Amazon Q Business application has already been created beforehand. If you haven’t created one yet, refer to Build private and secure enterprise generative AI apps with Amazon Q Business and AWS IAM Identity Center for instructions.
Complete the following steps to configure the connector:
- On the Amazon Q Business console, choose Applications in the navigation pane.
- Select the application that you want to add the Gmail connector to.
- On the Actions menu, choose Edit.

- On the Update application page, leave all values unchanged and choose Update.
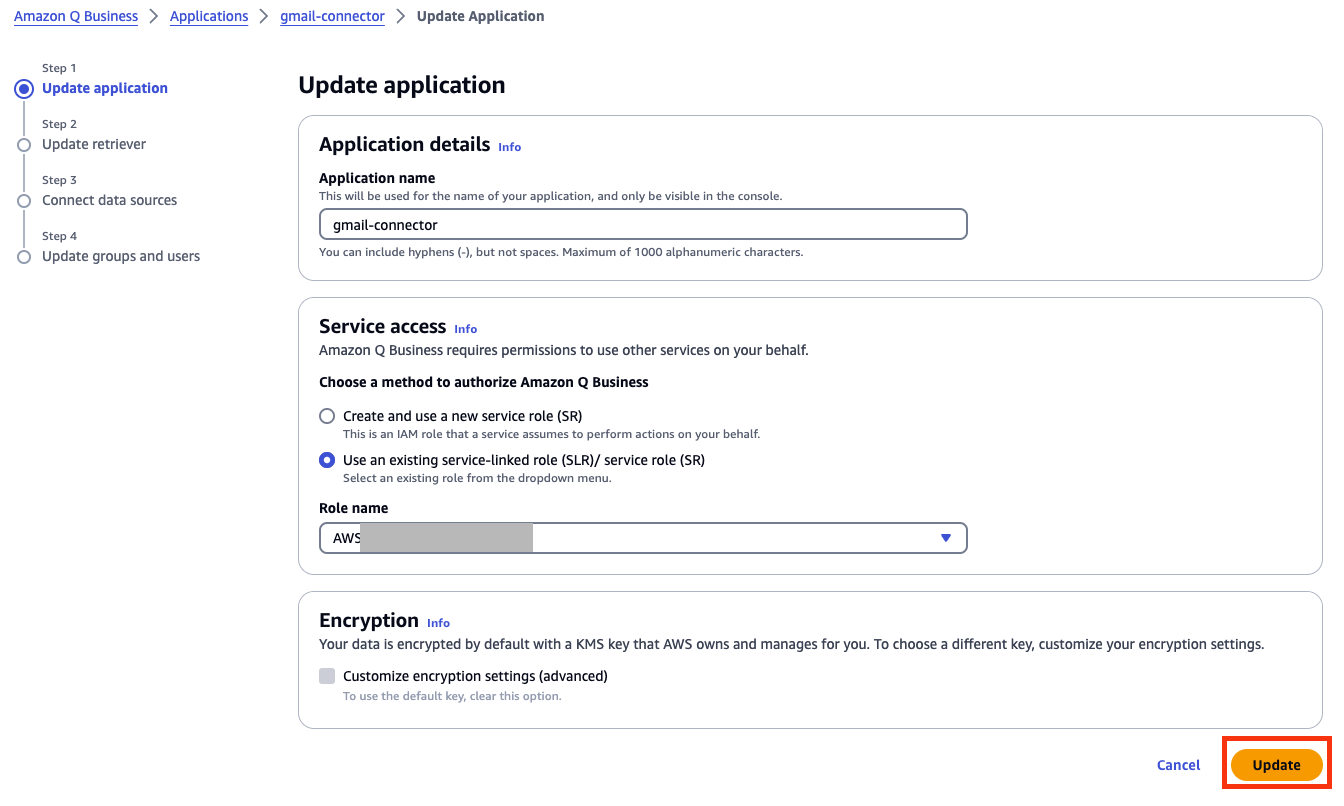
- On the Update retriever page, leave all values as default and choose Next.
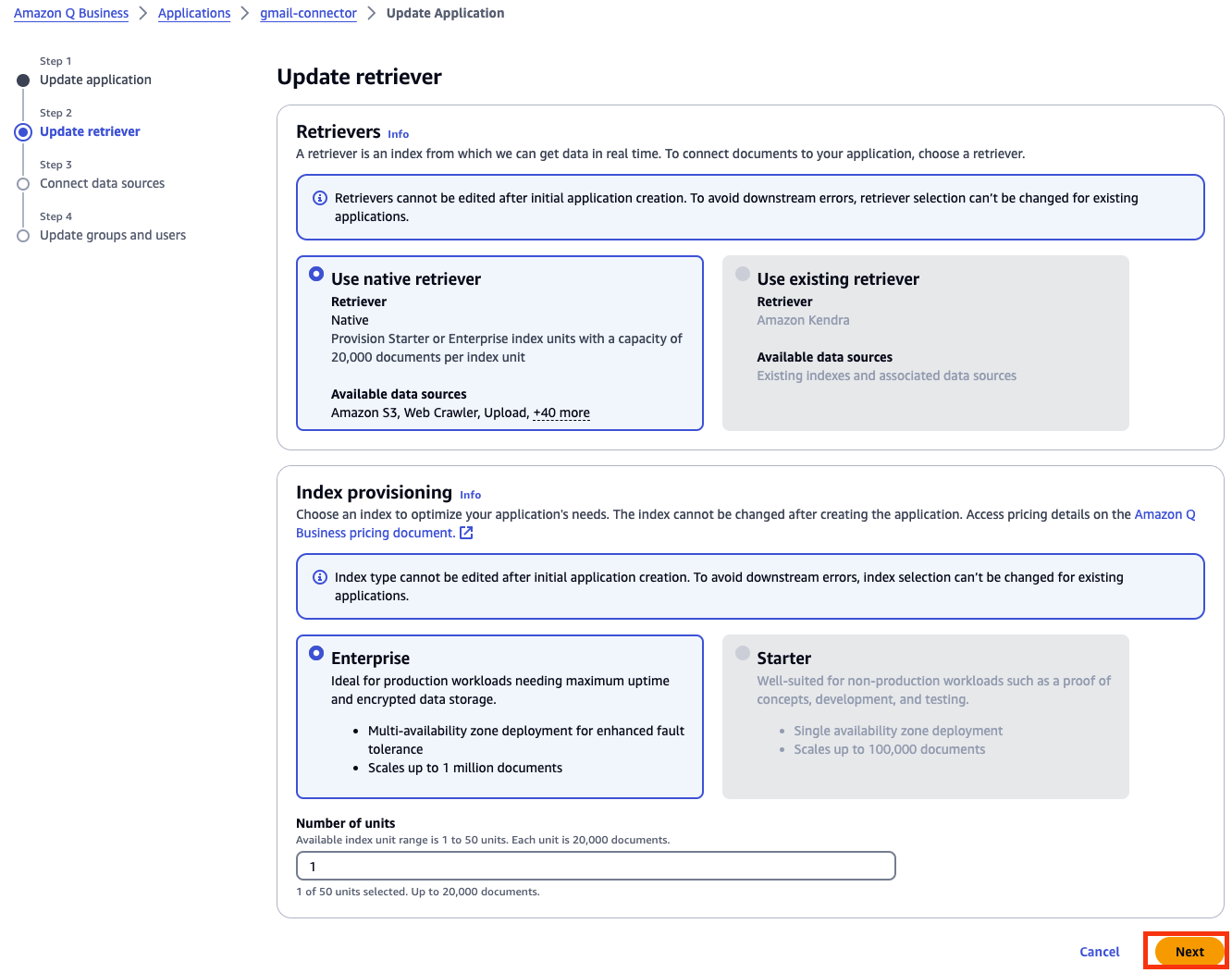
- On the Connect data sources page, on the All tab, search for Gmail in the search field.
- Choose the plus sign next to Gmail, which will open up a page to set up the data source.
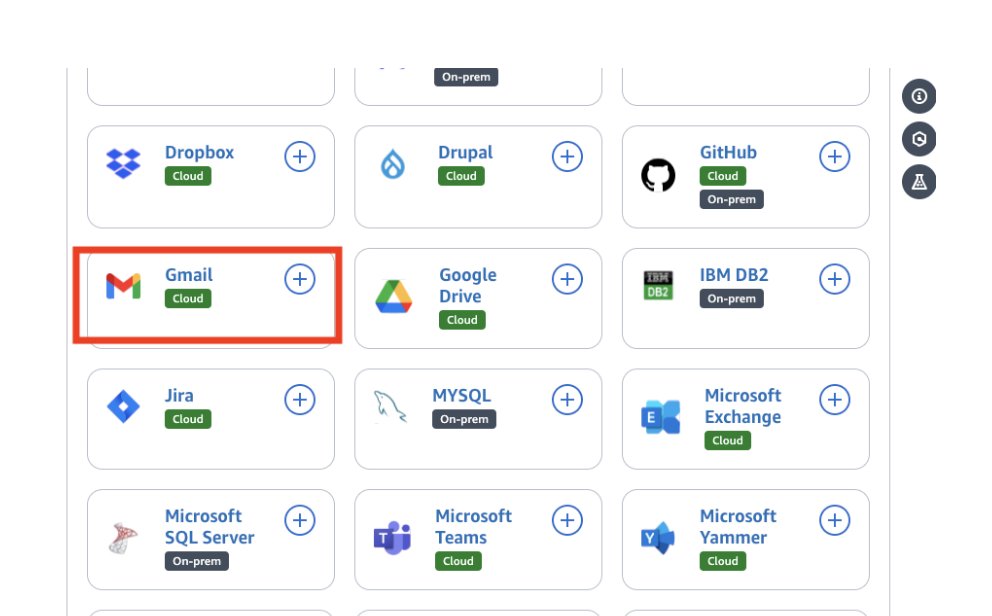
- In the Name and description section, enter a name and description.
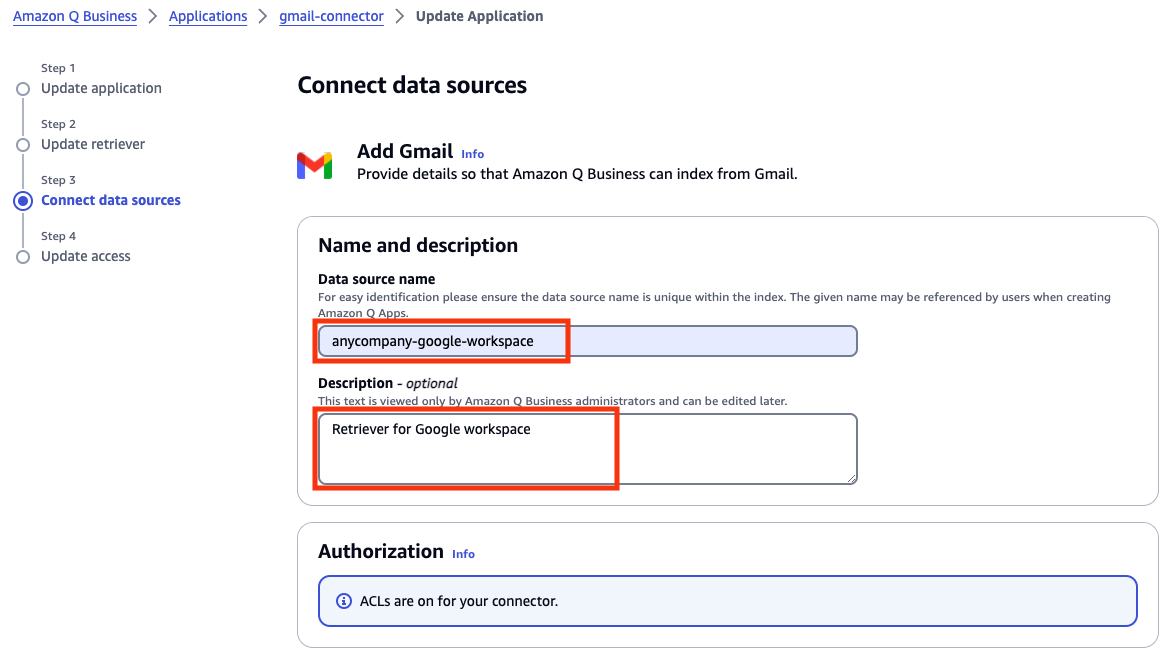
- In the Authentication section, choose Create and add new secret.
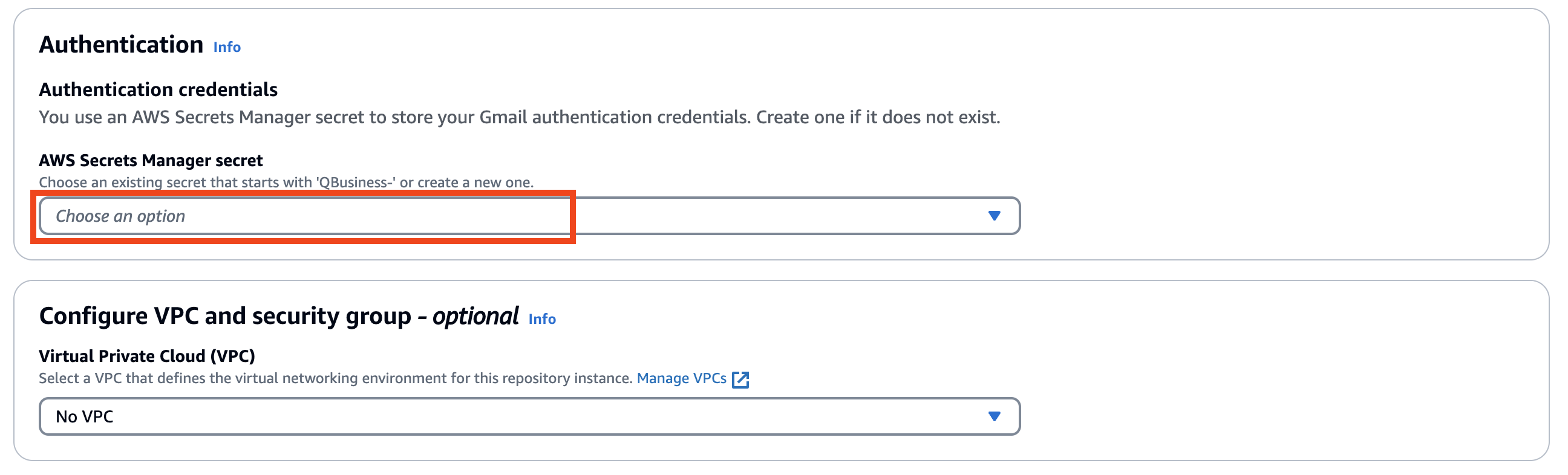
- In the Create an AWS Secrets Manager secret pop-up, provide the following information:
- Enter a name for your Secrets Manager secret.
- For Client email and Private key, refer to the JSON file that you downloaded to your local machine earlier.
- For Admin account email, enter the admin account for your Google
- For Private key, enter the private key details.
- Choose Save.
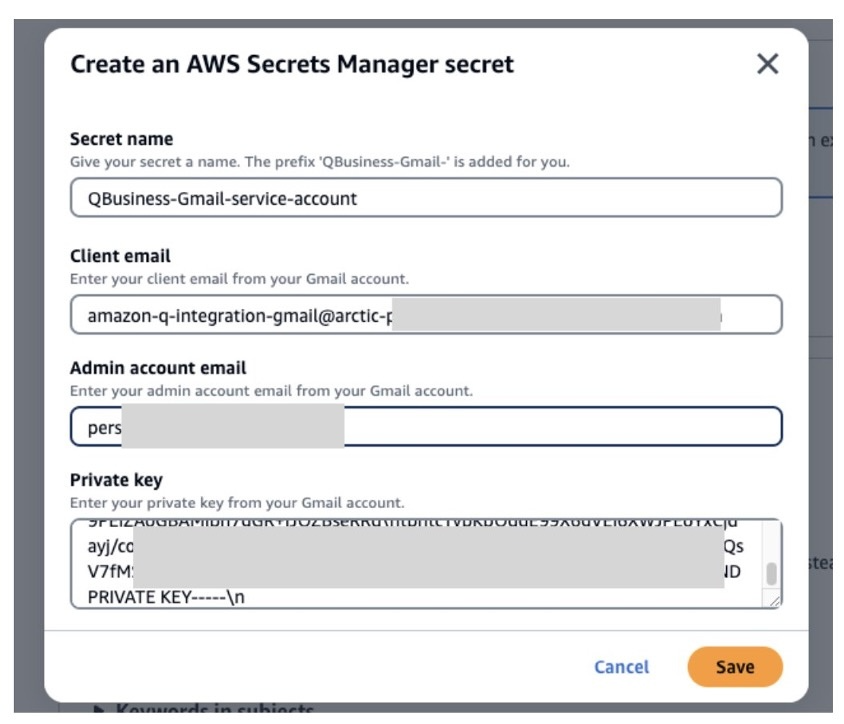
- In the IAM role section, for IAM role, choose Create a new service role (recommended).
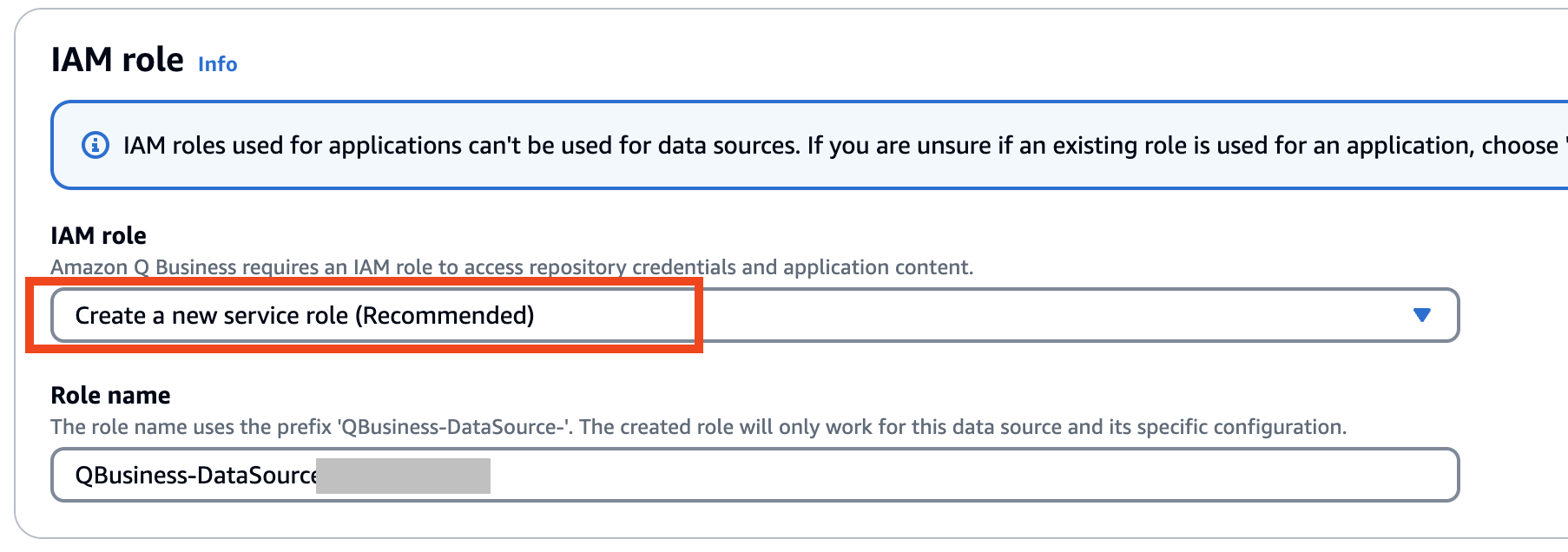
- In the Sync scope section, select Message attachments and enter a value for Maximum file size.
- Optionally, configure the following under Additional configuration (we leave everything as default for this post):
- For Date range, enter the start and end dates for emails to be crawled. Emails received on or after the start date and before the end date are included in the sync scope.
- For Email domains, enter the email from domains, email to domains, subject, CC emails, and BCC emails you want to include or exclude in your index.
- For Keywords in subjects, include or exclude any documents with at least one keyword mentioned in their subjects
- For Labels, add regular expression patterns to include or exclude certain labels or attachment types. You can add up to 100 patterns.
- For Attachments, add regular expression patterns to include or exclude certain attachments. You can add up to 100 patterns.
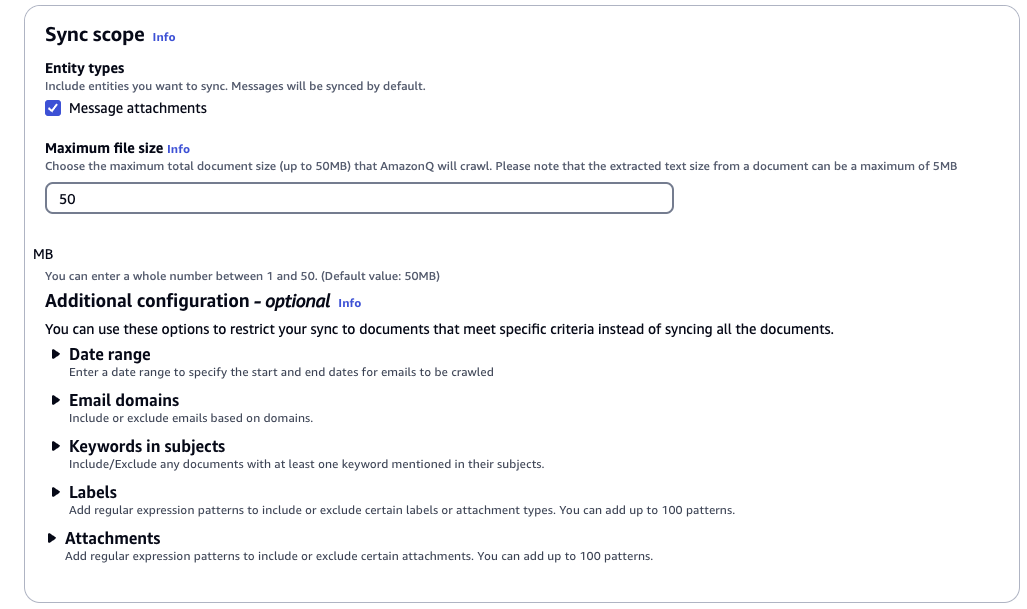
- In the Sync mode section, select New, modified, or deleted content sync.
- In the Sync run schedule section, choose the frequency that works best for your use case. For this post, we choose Run on demand.
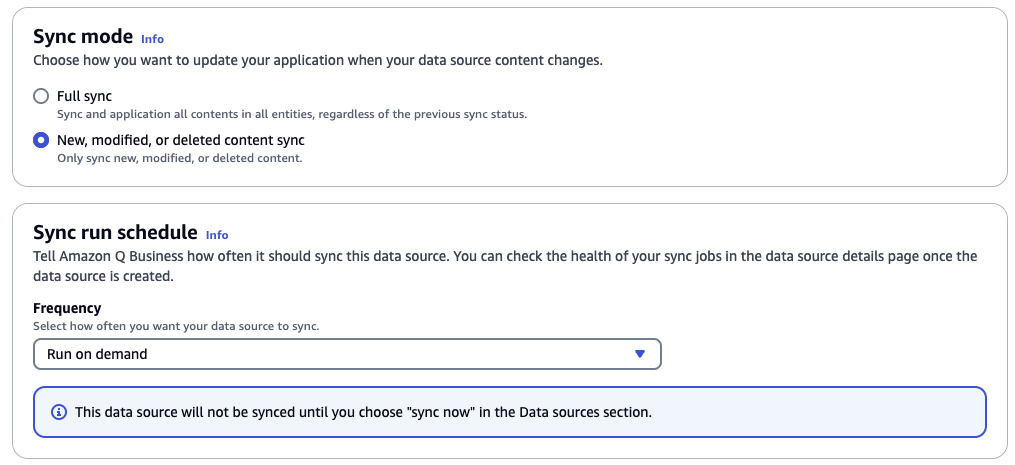
- Choose Add data source and wait for the retriever to be created.
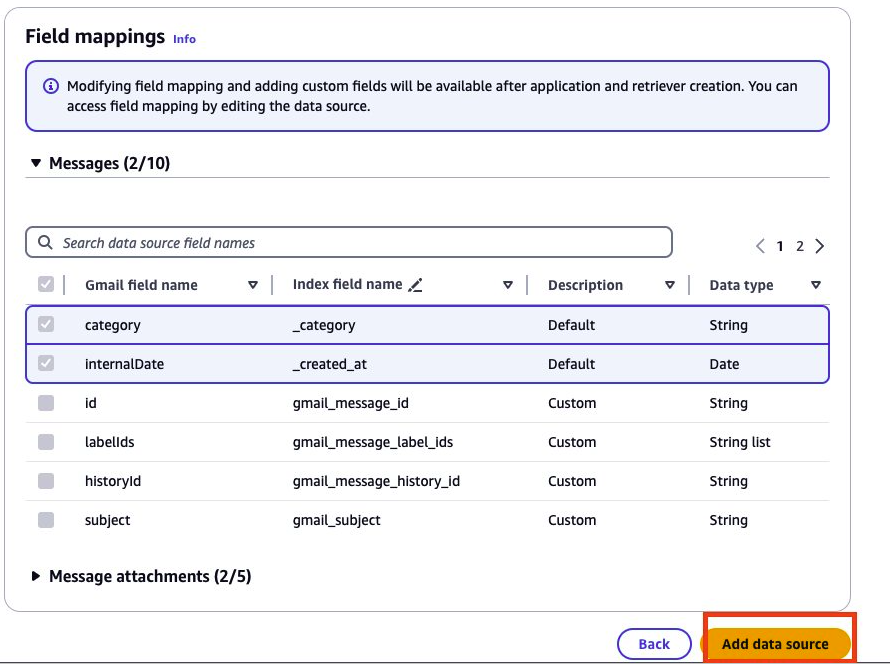
After the data source is created, you’re redirected to the Connect data sources page to add more data sources as needed.
- Verify your data source is added and choose Next.
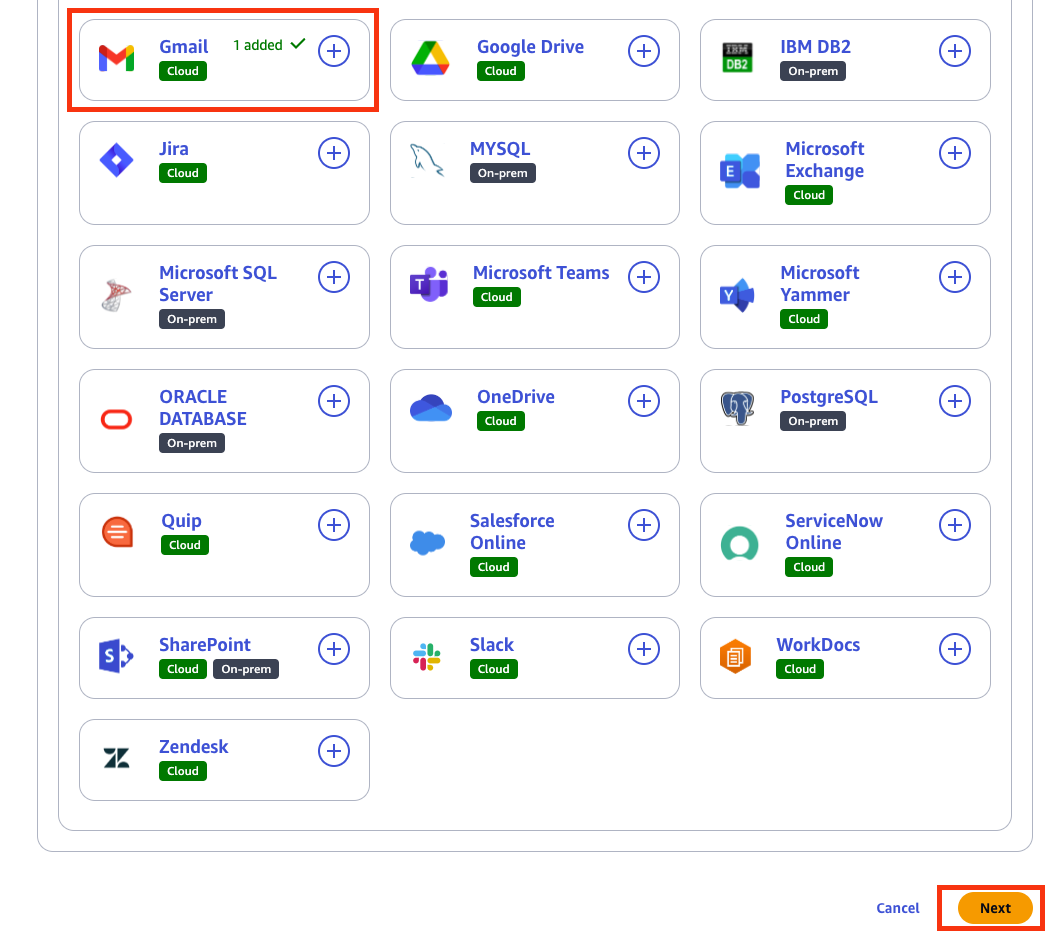
- On the Update groups and users page, choose Add groups and users.
The users and groups that you add in this section are from the IAM Identity Center users and groups set up by your administrator.
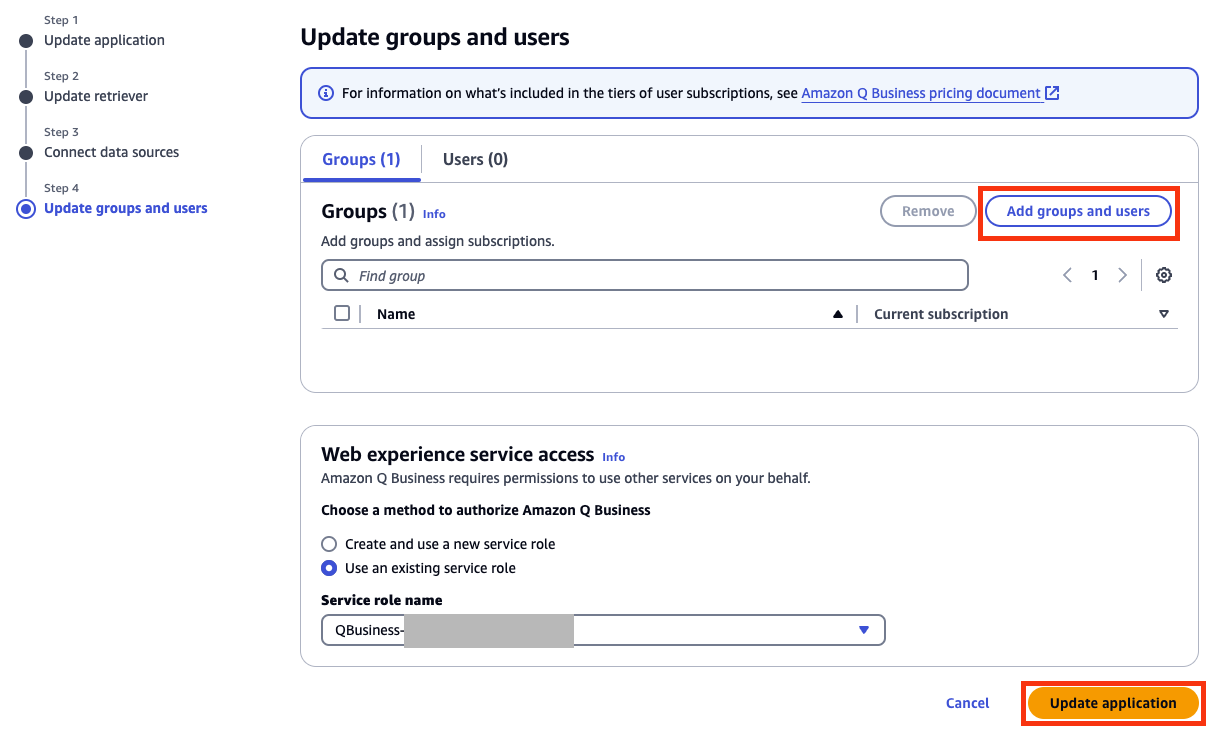
- In the Add or assign users and groups pop-up window, select Assign existing users and groups to add existing users configured in your connected IAM Identity Center, then choose Next.
Optionally, if you have permissions to add users to connected IAM Identity Center, you can select Add new users.
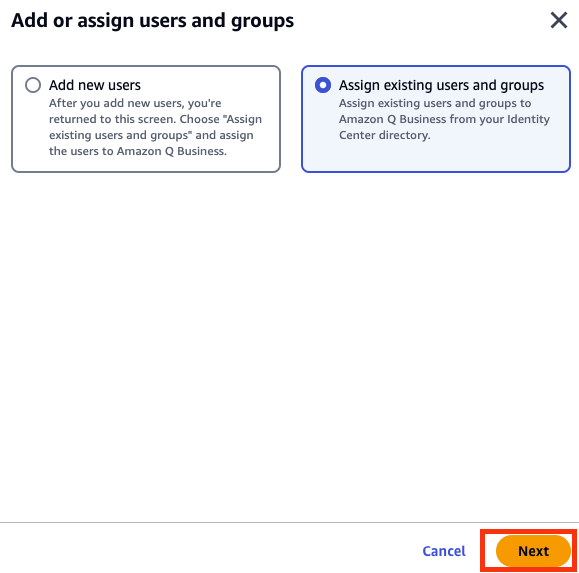
- Choose Get started.
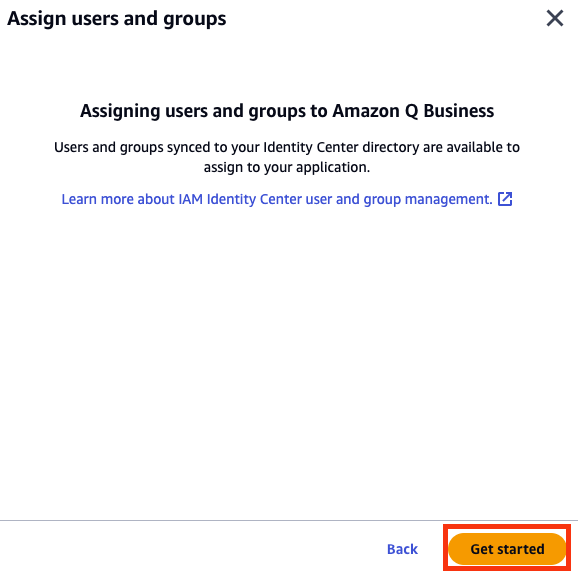
- Search for users by user display name or groups by group name.
- Choose the users or groups you want you add and choose Assign.
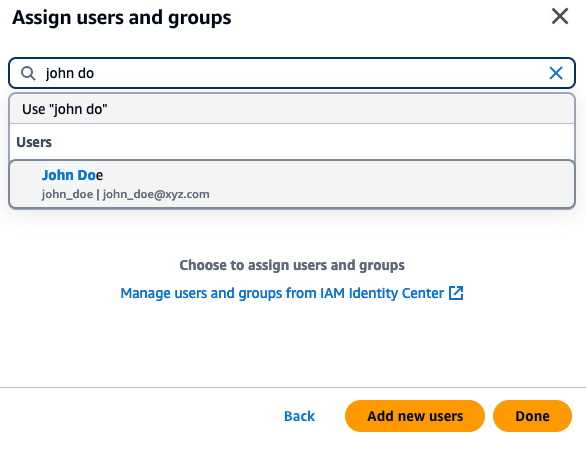
The groups and users that you added should now be available on the Groups or Users tabs.
- Choose Assign.
For each group or user entry, an Amazon Q Business subscription tier needs to be assigned.
- To enable a subscription for a group, on the Update groups and users page, choose the Groups tab (if individual users need to be assigned a subscription, choose the Users tab).
- Under the Subscription column, select Choose subscription and choose a subscription (Q Business Lite or Q Business Pro).
- Choose Update application to complete adding and setting up the Gmail connector for Amazon Q Business.
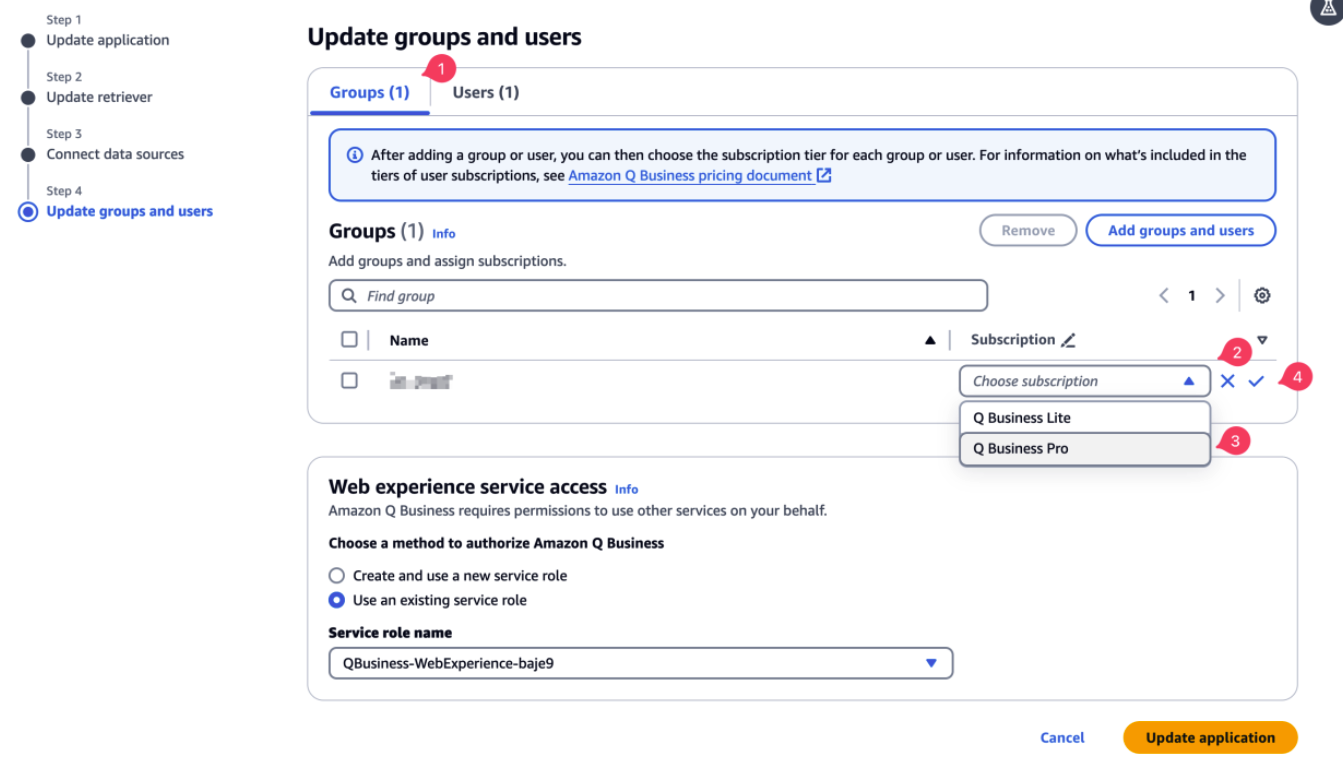
Configure Gmail field mappings
To help you structure data for retrieval and chat filtering, Amazon Q Business crawls data source document attributes or metadata and maps them to fields in your Amazon Q index. Amazon Q has reserved fields that it uses when querying your application. When possible, Amazon Q automatically maps these built-in fields to attributes in your data source.
If a built-in field doesn’t have a default mapping, or if you want to map additional index fields, use the custom field mappings to specify how a data source attribute maps to your Amazon Q application.
- On the Amazon Q Business console, choose your application.
- Under Data sources, select your data source.
- On the Actions menu, choose Edit.

- In the Field mappings section, select the required fields to crawl under Messages and Message attachments and any types that are available.
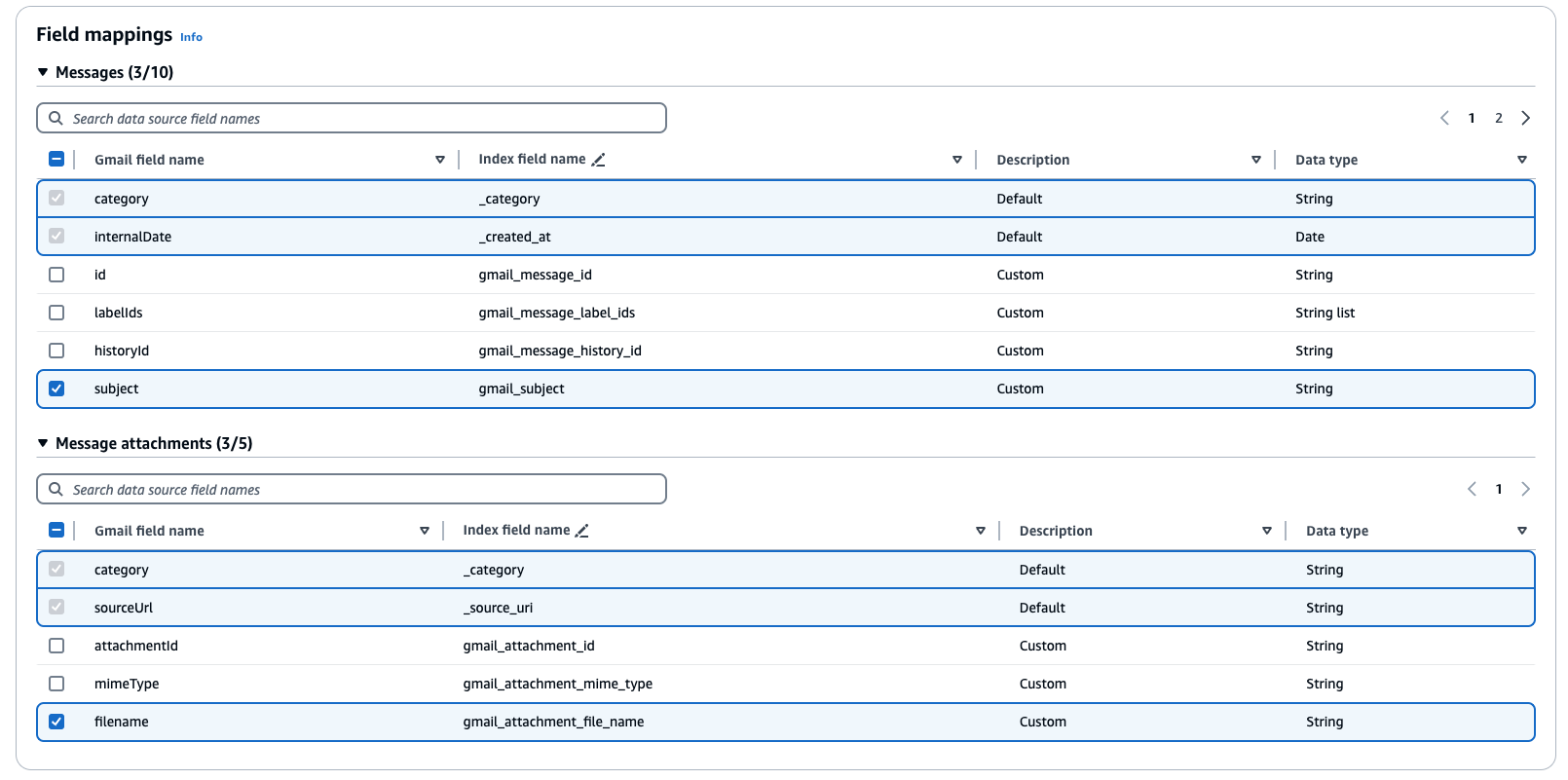
The Gmail connector setup for Amazon Q Business is now complete.
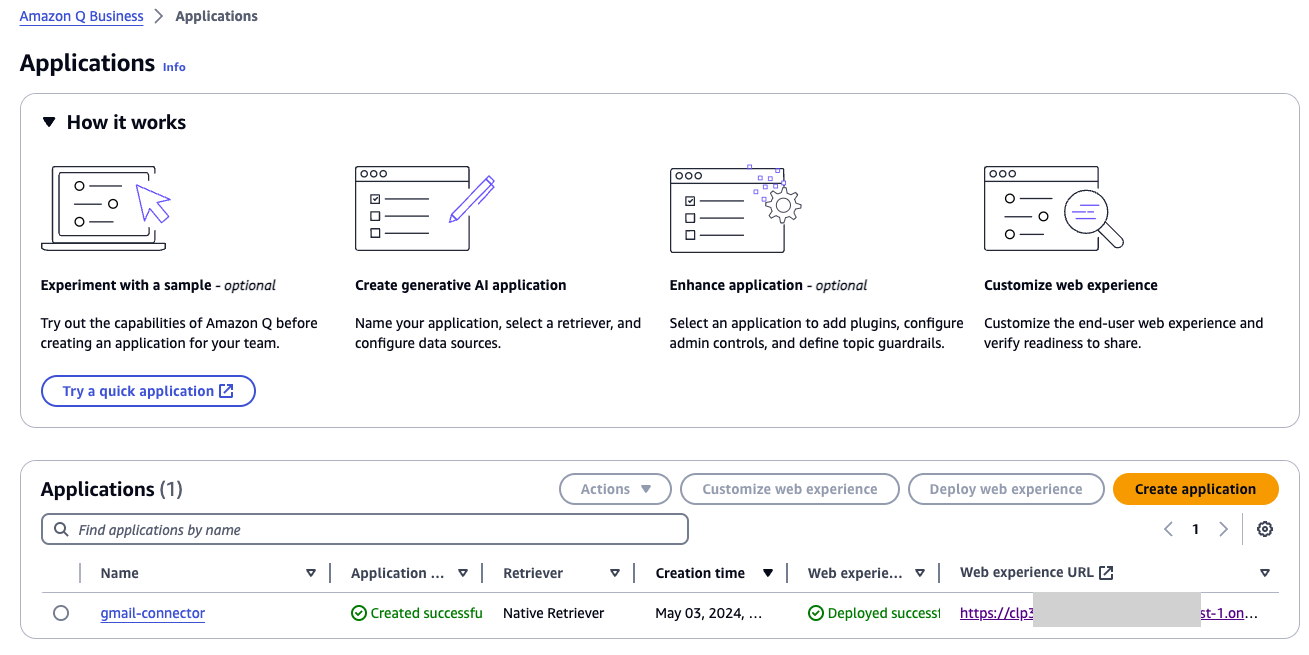
To test the connectivity to Gmail and initiate the data synchronization, choose Sync now. The initial sync process may take several minutes to complete.
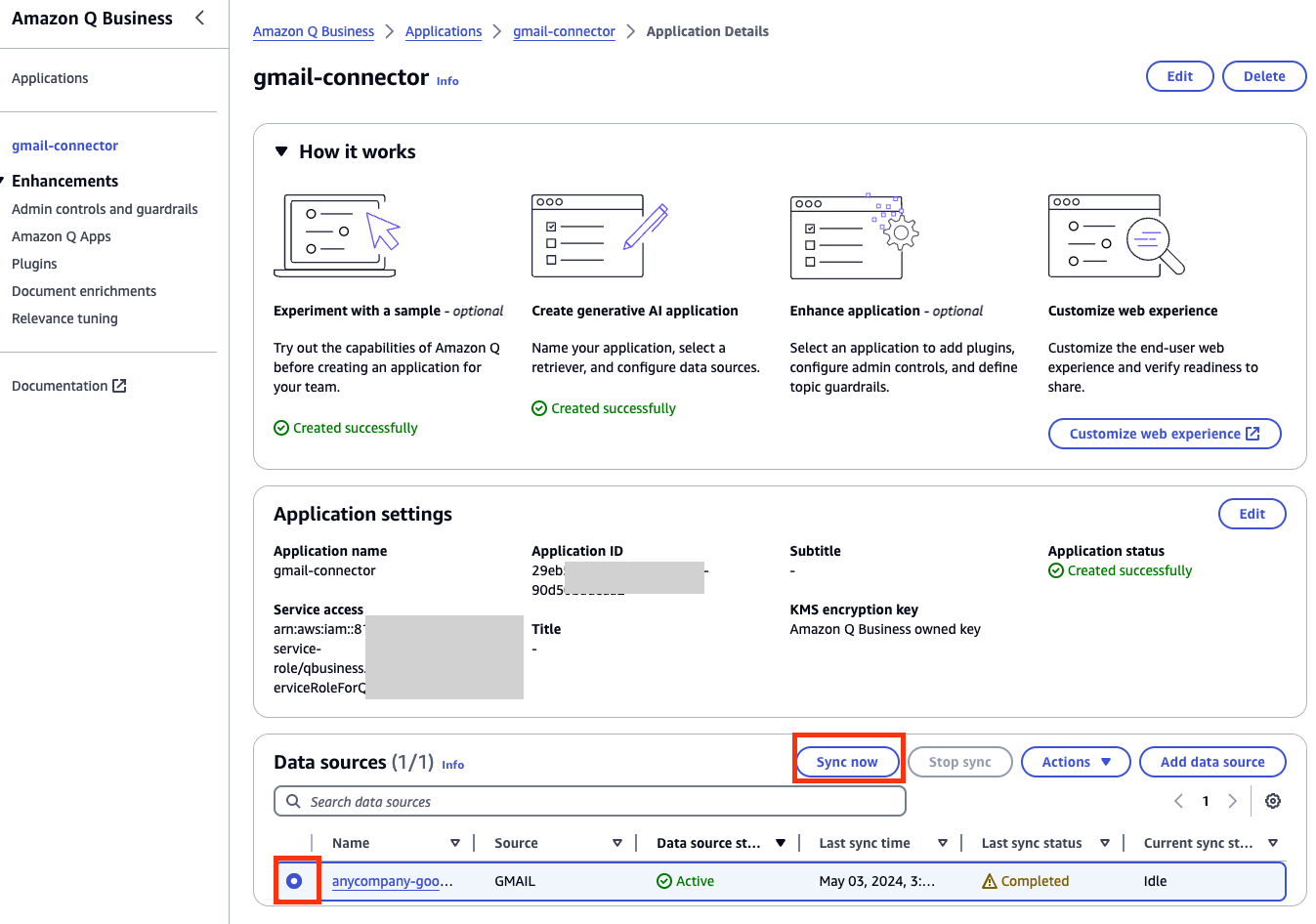
When the sync is complete, in the Sync run history section, you can see the sync status along with a summary of how may total items were added, deleted, modified, and failed during the sync process.
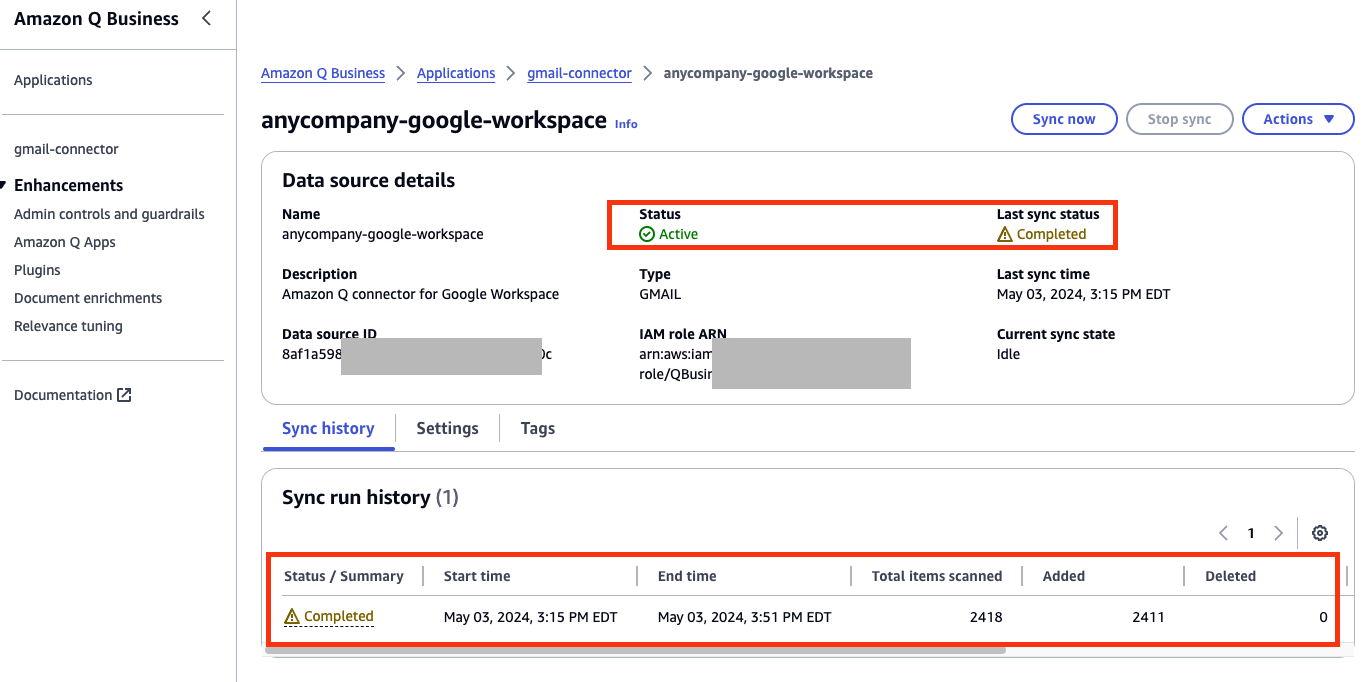
Query Gmail data using the Amazon Q web experience
Now that the data synchronization is complete, you can start exploring insights from Amazon Q. In the newly created Amazon Q application, choose Customize web experience to open a new tab with a preview of the UI and options to customize as per your needs.
You can customize the Title, Subtitle, and Welcome message fields according to your needs, which will be reflected in the UI.
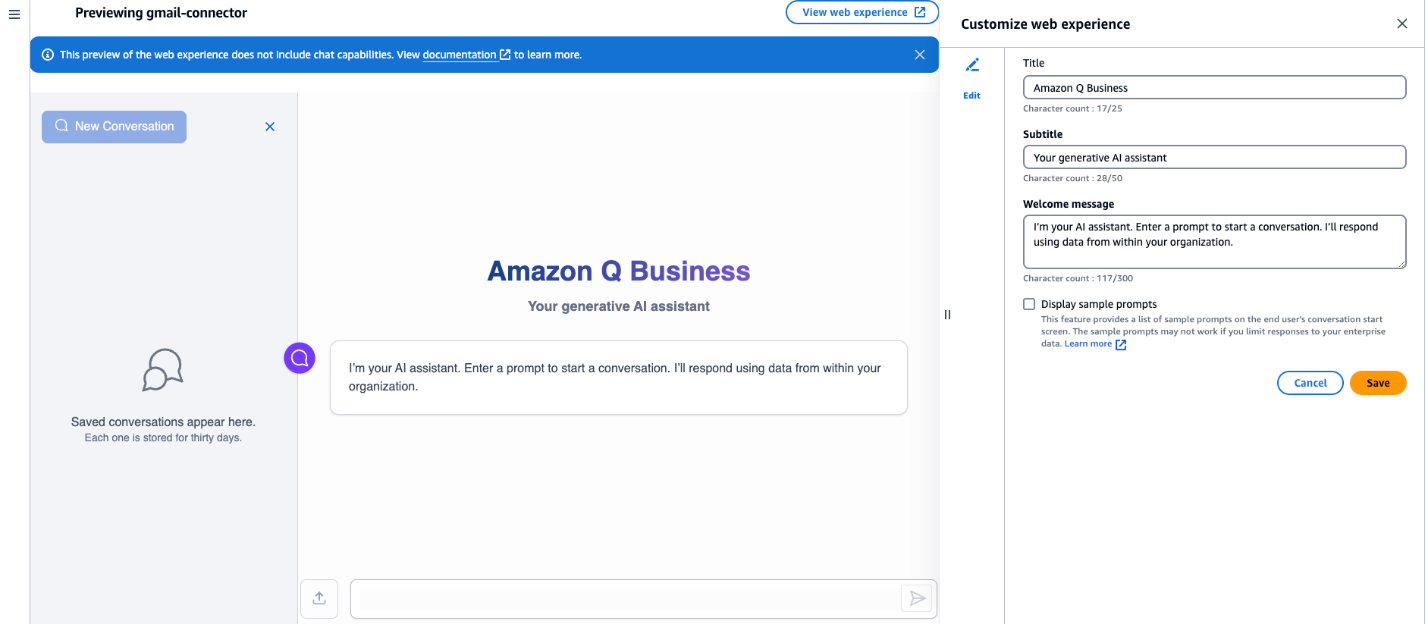
For this walkthrough, we use the defaults and choose View web experience to be redirected to the login page for the Amazon Q application.
Log in to the application using the credentials for the user that were added to the Amazon Q application. After the login is successful, you’re redirected to the Amazon Q assistant UI, where you can ask questions using natural language and get insights from your Gmail index.
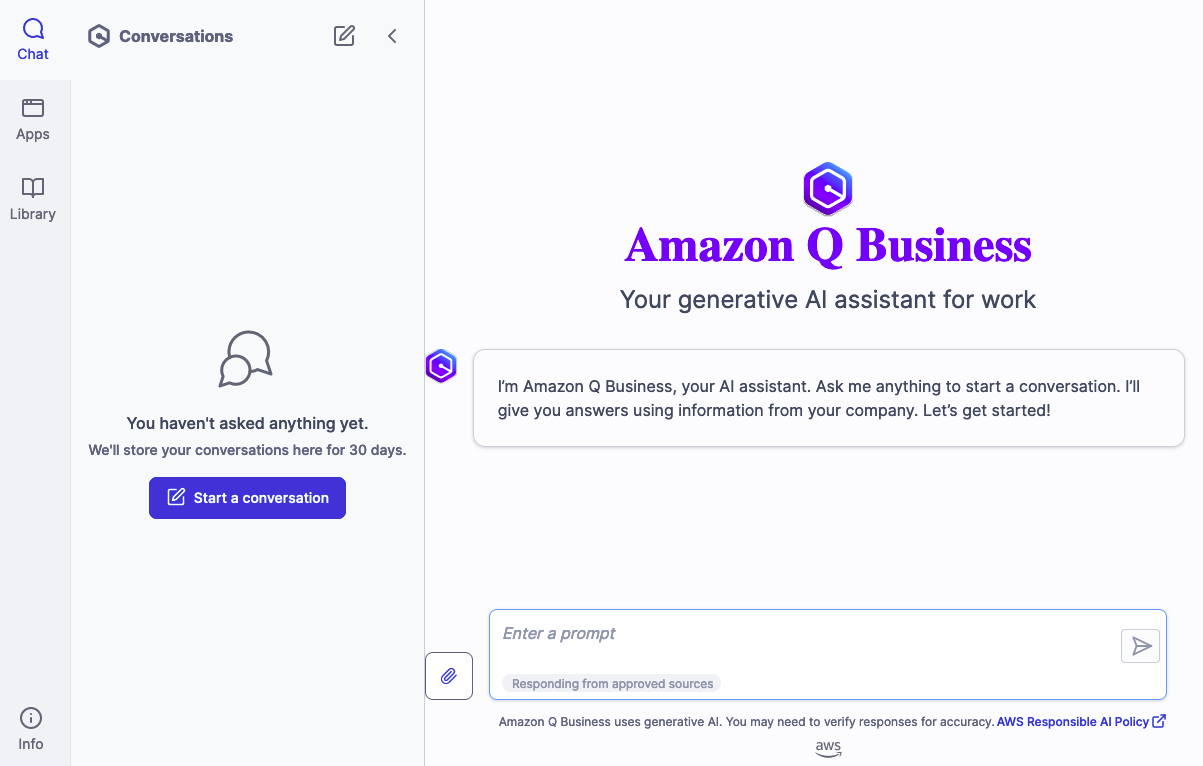
The Gmail data source connected to this Amazon Q Business application has email and Gmail attachments. We demonstrate how the Amazon Q application lets you ask questions on your email using natural language and receive responses and insights for those queries.
Let’s begin by asking Amazon Q to summarize key points from Matt Garma’s (CEO of AWS) email. The following screenshot displays the response and it also includes the email source from where it is generating the response.
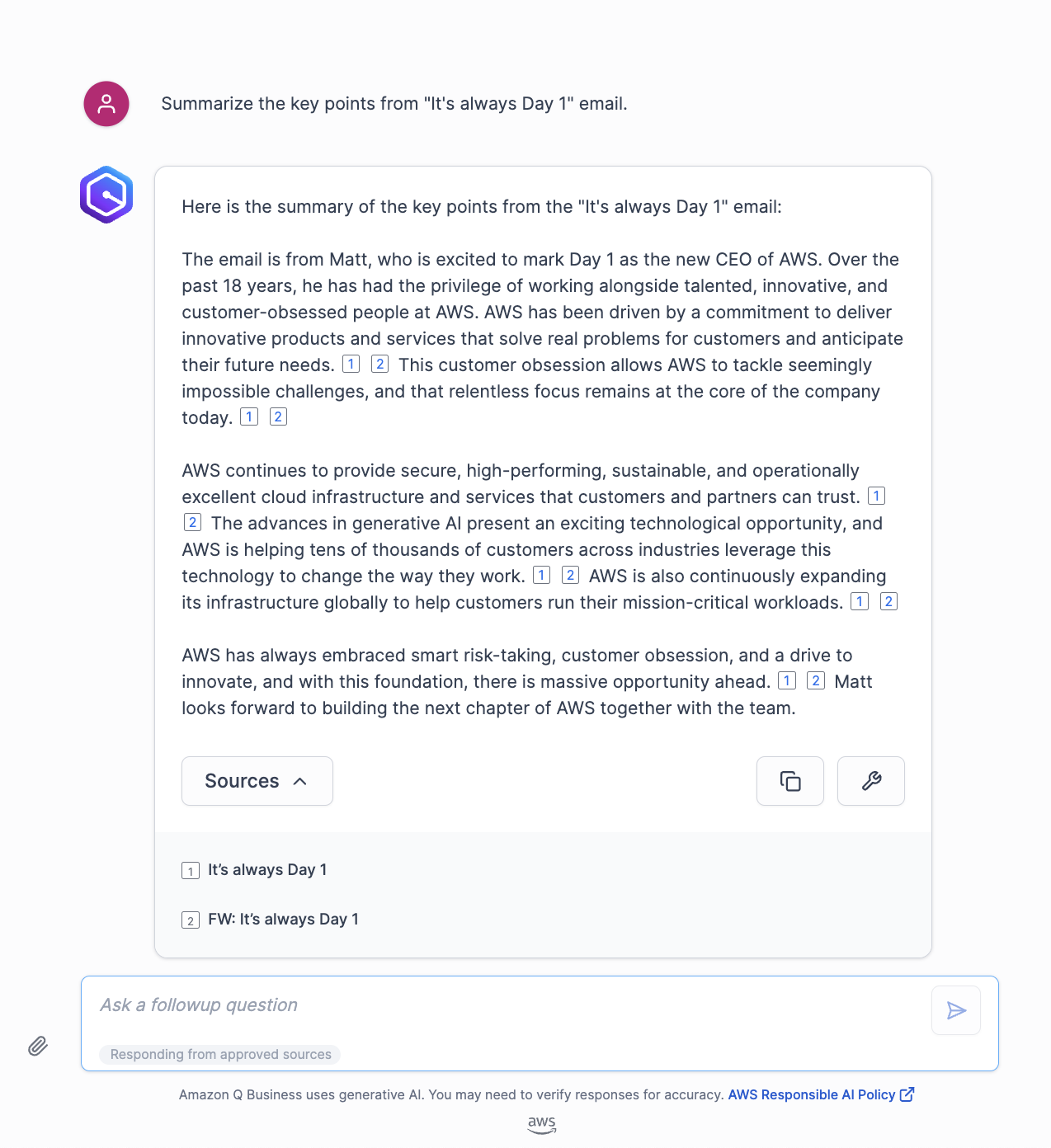
For our next example, let’s ask Amazon Q to provide details about return issue customer is facing for a bicycle order they placed with Amazon. Following screenshot shows the details about the issue being faced by the customer and includes the email source from where Amazon Q is generating the response.
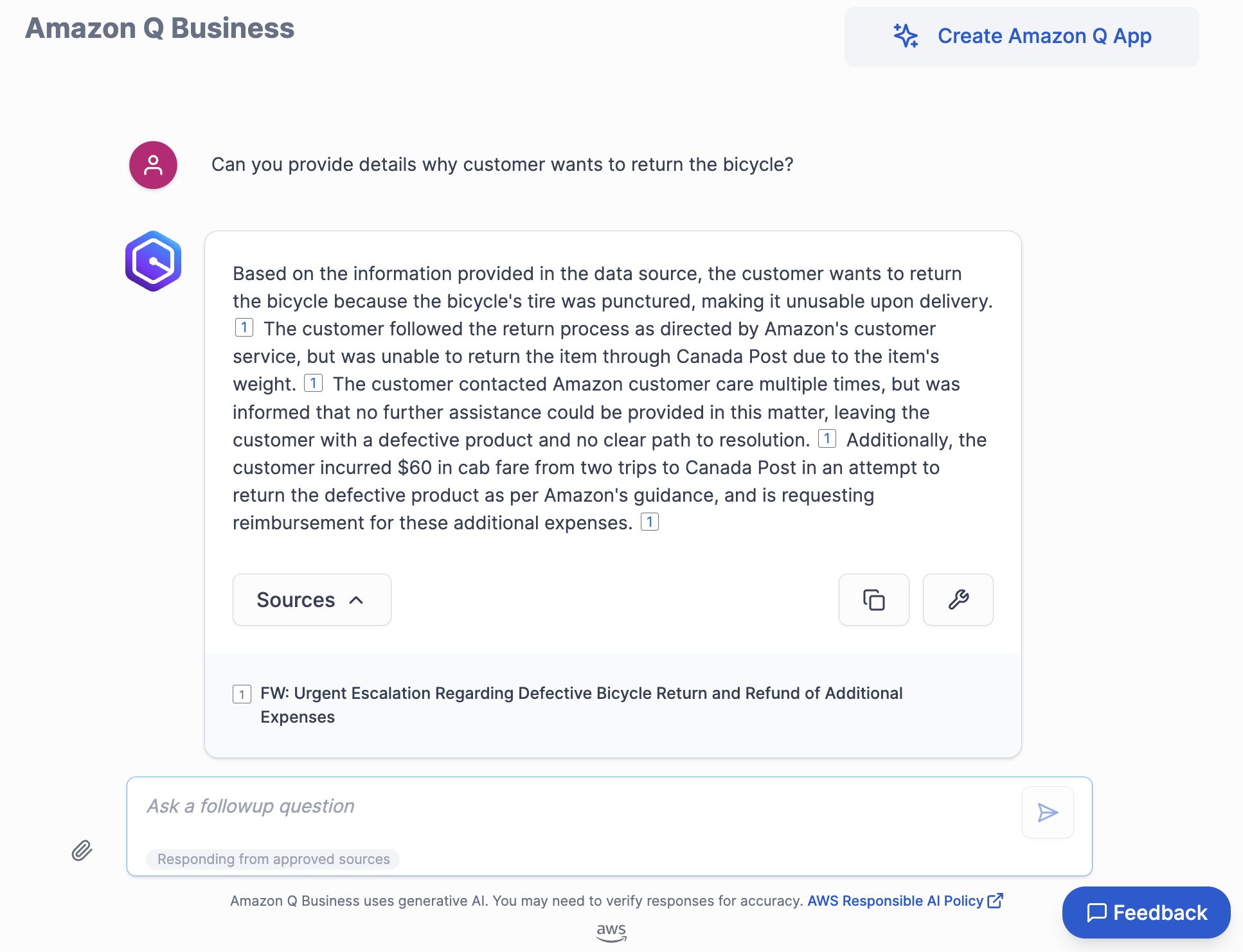
Troubleshooting
Troubleshooting your Amazon Q Business Gmail connector provides information about error codes you might see for the Gmail connector and suggested troubleshooting actions. If you encounter an HTTP status code 403 (Forbidden) error when you open your Amazon Q Business application, it means that the user is unable to access the application. . See Troubleshooting Amazon Q Business and identity provider integration for common causes and how to address them.
Frequently asked questions
In this section, we provide guidance to frequently asked questions.
Amazon Q Business is unable to answer your questions
This could happen due to a several reasons:
- No permissions – ACLs applied to your account doesn’t allow you to query certain data sources. If this is the case, reach out to your application administrator to make sure your ACLs are configured to access the data sources.
- Data connector sync failed – The data connector might have failed to sync information from the source to the Amazon Q Business application. Verify the data connector’s sync run schedule and sync history to confirm the sync is successful.
If neither of these reasons are true in your case, open a support case to get this resolved.
How to generate responses from authoritative data sources
You can configure these options using Amazon Q Business application global controls under Admin controls and guardrails.
- Log in as an Amazon Q Business application administrator.
- Navigate to the application and choose Admin controls and guardrails in the navigation pane.
- Choose Edit in the Global controls section to control these options.
For more information, refer to Admin controls and guardrails in Amazon Q Business.
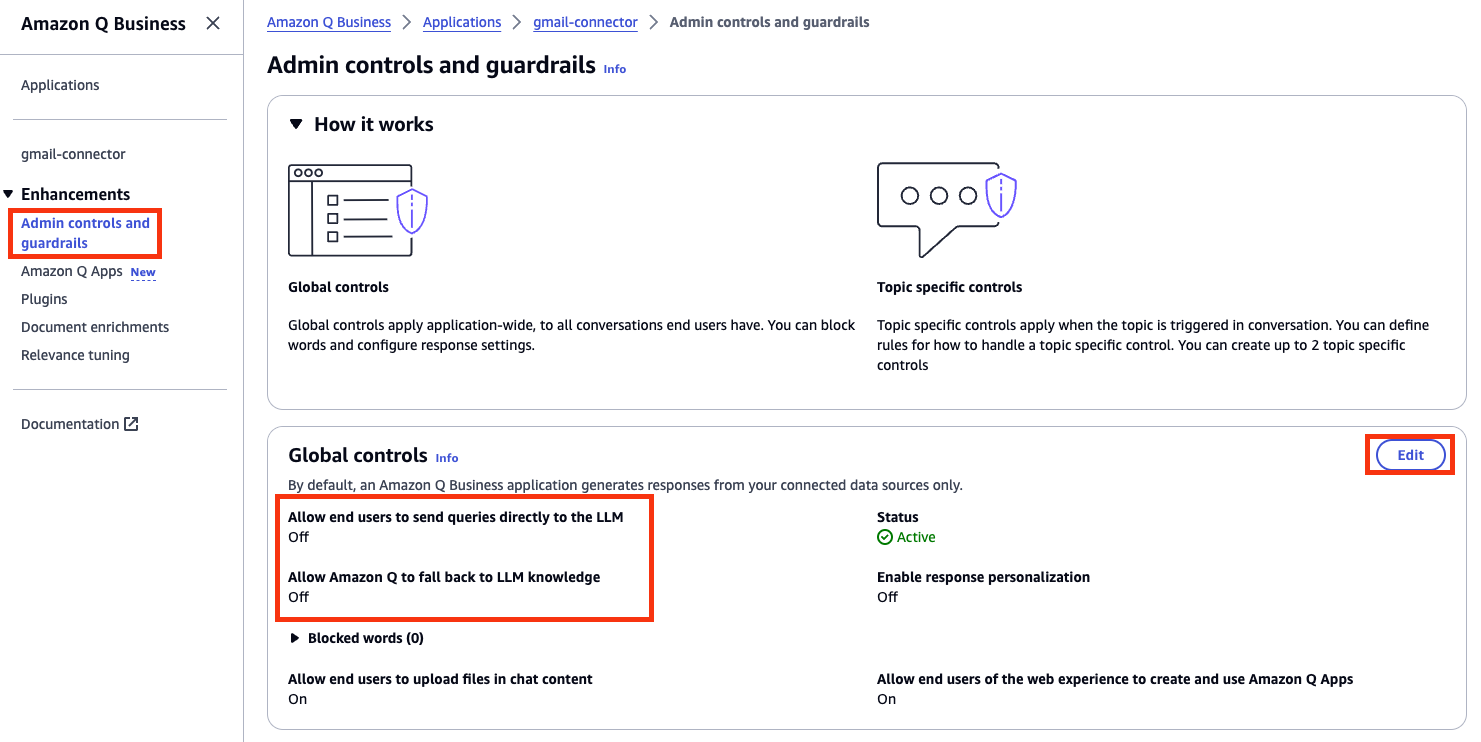
Amazon Q Business responds using old (stale) data even though your data source is updated
Each Amazon Q Business data connector can be configured with unique sync run schedule frequency. Verify the sync status and sync schedule frequency for your data connector to see when the last sync ran successfully. Your data connector’s sync run schedule could be set to sync at a scheduled time of day, week, or month. If it’s set to run on demand, the sync has to be run manually. When the sync run is complete, verify the sync history to make sure the run has successfully synced all new issues. Refer to Sync run schedule for more information on each option.
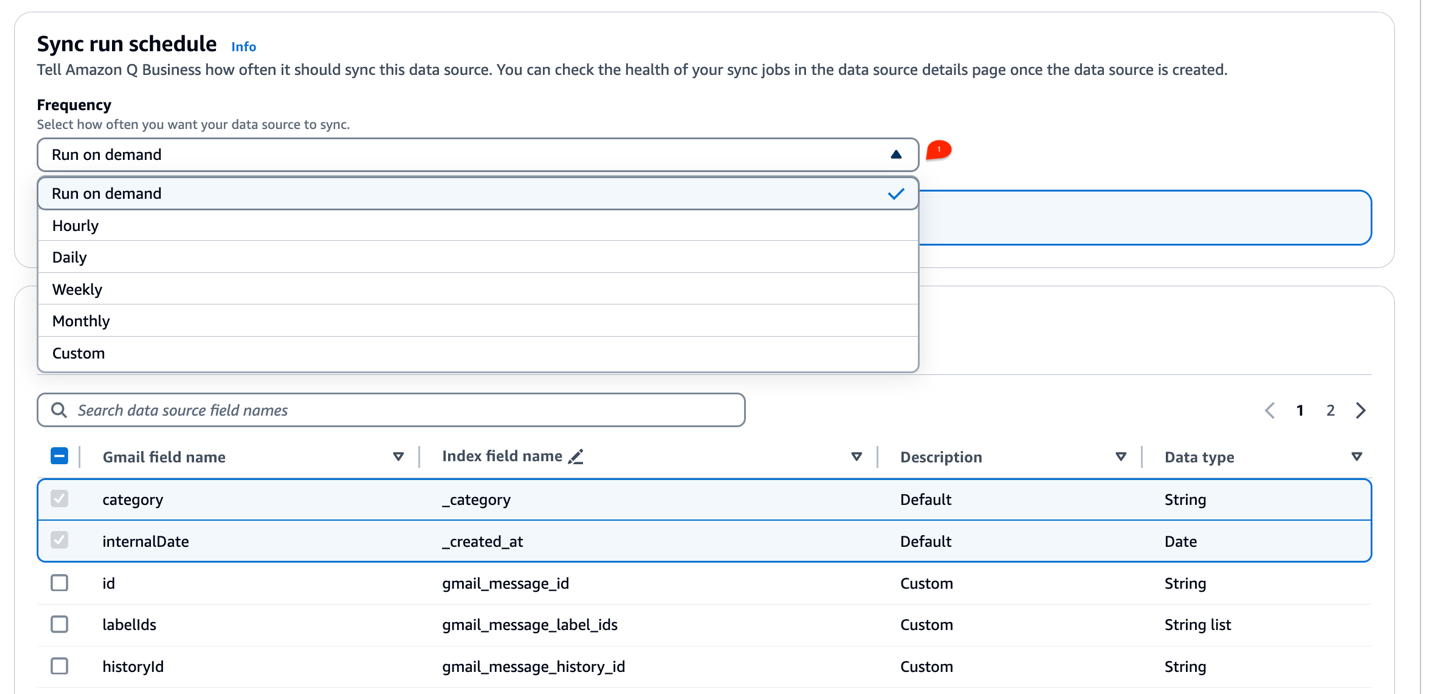
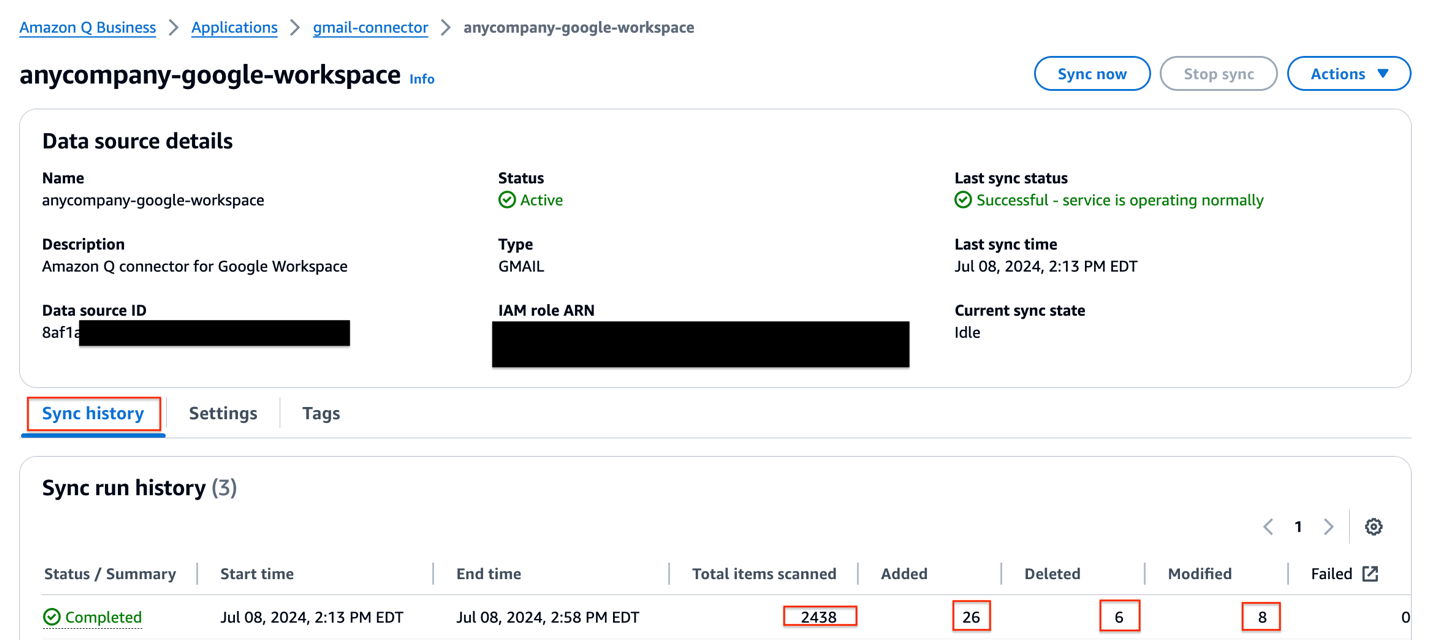
How to set up Amazon Q Business using a different IdP
You can set up Amazon Q Business with another SAML 2.0-compliant IdP, such as Okta, Entra ID, or Ping Identity. For more information, see Creating an Amazon Q Business application using Identity Federation through IAM.
Expand the solution
You can explore other features in Amazon Q Business. For example, the Amazon Q Business document enrichment feature helps you control both which documents and document attributes are ingested into your index and how they’re ingested. With document enrichment, you can create, modify, or delete document attributes and document content when you ingest them into your Amazon Q Business index. For example, you can scrub personally identifiable information (PII) by choosing to delete any document attributes related to PII.
Amazon Q Business also offers the following features:
- Filtering using metadata – Use document attributes to customize and control users’ chat experience. This is currently supported only if you use the Amazon Q Business API.
- Source attribution with citations – Verify responses using Amazon Q Business source attributions.
- Upload files and chat – Let users upload files directly into chat and use uploaded file data to perform web experience tasks.
- Quick prompts – Feature sample prompts to inform users of the capabilities of their Amazon Q Business web experience.
To improve retrieved results and customize the user chat experience, you can map document attributes from your data sources to fields in your Amazon Q index. To learn more, see Gmail data source connector field mappings.
Clean up
To avoid incurring future charges, clean up any resources you created as part of this solution, including the Amazon Q application:
- On the Amazon Q console, choose Applications in the navigation pane.
- Select the dashboard you created.
- On the Actions menu, choose Delete.
- Delete the IAM roles created for the application and data retriever.
- If you used IAM Identity Center for this walkthrough, delete your IAM Identity Center instance.
Conclusion
In this post, we discussed how to configure the Gmail connector for Amazon Q Business and use the AI-powered chat interface to gain insights from the connected data source.
To learn more about the Gmail connector for Amazon Q Business, refer to Connecting Gmail to Amazon Q Business, the Amazon Q User Guide, and the Amazon Q Developer Guide.
About the Authors
Divyajeet (DJ) Singh is a Sr. Solutions Architect at AWS Canada. He loves working with customers to help them solve their unique business challenges using the cloud. In his free time, he enjoys spending time with family and friends, and exploring new places.
Temi Aremu is a Solutions Architect at AWS Canada. She is passionate about helping customers solve their business problems with the power of the AWS Cloud. Temi’s areas of interest are analytics, machine learning, and empowering the next generation of women in STEM.
Vineet Kachhawaha is a Sr. Solutions Architect at AWS focusing on AI/ML and generative AI. He co-leads the AWS for Legal Tech team within AWS. He is passionate about working with enterprise customers and partners to design, deploy, and scale AI/ML applications to derive business value.
Vijai Gandikota is a Principal Product Manager in the Amazon Q and Amazon Kendra organization of Amazon Web Services. He is responsible for the Amazon Q and Amazon Kendra connectors, ingestion, security, and other aspects of the Amazon Q and Amazon Kendra services.
Dipti Kulkarni is a Software Development Manager on the Amazon Q and Amazon Kendra engineering team of Amazon Web Services, where she manages the connector development and integration teams.
Read More