Amazon Rufus is a shopping assistant experience powered by generative AI. It generates answers using relevant information from across Amazon and the web to help Amazon customers make better, more informed shopping decisions. With Rufus, customers can shop alongside a generative AI-powered expert that knows Amazon’s selection inside and out, and can bring it all together with information from across the web to help shoppers make more informed purchase decisions.
To meet the needs of Amazon customers at scale, Rufus required a low-cost, performant, and highly available infrastructure for inference. The solution needed the capability to serve multi-billion parameter large language models (LLMs) with low latency across the world to service its expansive customer base. Low latency makes sure users have a positive experience chatting with Rufus and can start getting responses in less than a second. To achieve this, the Rufus team is using multiple AWS services and AWS AI chips, AWS Trainium and AWS Inferentia.
Inferentia and Trainium are purpose-built chips developed by AWS that accelerate deep learning workloads with high performance and lower overall costs. With these chips, Rufus reduced its costs by 4.5 times lower than other evaluated solutions while maintaining low latency for its customers. In this post, we dive into the Rufus inference deployment using AWS chips and how this enabled one of the most demanding events of the year—Amazon Prime Day.
Solution overview
At its core, Rufus is powered by an LLM trained on Amazon’s product catalog and information from across the web. LLM deployment can be challenging, requiring you to balance factors such as model size, model accuracy, and inference performance. Larger models generally have better knowledge and reasoning capabilities but come at a higher cost due to more demanding compute requirements and increasing latency. Rufus would need to be deployed and scale to meet the tremendous demand of peak events like Amazon Prime Day. Considerations for this scale include how well it needs to perform, its environmental impact, and the cost of hosting the solution. To meet these challenges, Rufus used a combination of AWS solutions: Inferentia2 and Trainium, Amazon Elastic Container Service (Amazon ECS), and Application Load Balancer (ALB). In addition, the Rufus team partnered with NVIDIA to power the solution using NVIDIA’s Triton Inference Server, providing capabilities to host the model using AWS chips.
Rufus inference is a Retrieval Augmented Generation (RAG) system with responses enhanced by retrieving additional information such as product information from Amazon search results. These results are based on the customer query, making sure the LLM generates reliable, high-quality, and precise responses.
To make sure Rufus was best positioned for Prime Day, the Rufus team built a heterogeneous inference system using multiple AWS Regions powered by Inferentia2 and Trainium. Building a system across multiple Regions allowed Rufus to benefit in two key areas. First, it provided additional capacity that could be used during times of high demand, and second, it improved the overall resiliency of the system.
The Rufus team was also able to use both Inf2 and Trn1 instance types. Because Inf2 and Trn1 instance types use the same AWS Neuron SDK, the Rufus team was able to use both instances to serve the same Rufus model. The only configuration setting to adjust was the tensor parallelism degree (24 for Inf2, 32 for Trn1). Using Trn1 instances also led to an additional 20% latency reduction and throughput improvement compared to Inf2.
The following diagram illustrates the solution architecture.
To support real-time traffic routing across multiple Regions, Rufus built a novel traffic orchestrator. Amazon CloudWatch supported the underlying monitoring, helping the team adjust the traffic ratio across the different Regions in less than 15 minutes based on the traffic pattern changes. By using this type of orchestration, the Rufus team had the ability to direct requests to other Regions when needed, with a small trade-off of latency to the first token. Due to Rufus’s streaming architecture and the performant AWS network between Regions, the perceived latency was minimal for end-users.
These choices allowed Rufus to scale up over 80,000 Trainium and Inferentia chips across three Regions serving an average of 3 million tokens a minute while maintaining P99 less than 1 second latency to the first response for Prime Day customers. In addition, by using these purpose-built chips, Rufus achieved 54% better performance per watt than other evaluated solutions, which helped the Rufus team meet energy efficiency goals.
Optimizing inference performance and host utilization
Within each Region, the Rufus inference system used Amazon ECS, which managed the underlying Inferentia and Trainium powered instances. By managing the underlying infrastructure, the Rufus team only needed to bring their container and configuration by defining an ECS task. Within each container, an NVIDIA Triton Inference Server with a Python backend is used running vLLM with the Neuron SDK. vLLM is a memory-efficient inference and serving engine that is optimized for high throughput. The Neuron SDK makes it straightforward for teams to adopt AWS chips and supports many different libraries and frameworks such as PyTorch Lightning.
The Neuron SDK provides a straightforward LLM inference solution on Trainium and Inferentia hardware with optimized performance supporting a wide range of transformer-based LLM architectures. To reduce latency, Rufus has collaborated with the AWS Annapurna team to develop various optimizations such as INT8 (weight only) quantization, continuous batching with vLLM, resource, compute, and memory bandwidth in the Neuron compiler and runtime. These optimizations are currently deployed in Rufus production and are available to use in the Neuron SDK 2.18 and onward.
To reduce overall waiting time for customers to start seeing a response from Rufus, the team also developed an inference streaming architecture. With the high compute and memory load needed for LLM inference, the total time it takes to finish generating the full response for a customer query can take multiple seconds. With a streaming architecture, Rufus is able to return the tokens right after they’re generated. This optimization allows the customer to start consuming the response in less than 1 second. In addition, multiple services work together using gRPC connections to intelligently aggregate and enhance the streaming response in real time for customers.
As shown in the following figure, images and links are embedded in the response, which allow customers to engage and continue exploring with Rufus.
Scaling up
Although we have to maintain low latency for the best customer experience, it’s also crucial to scale the service throughput by achieving high hardware resource utilization. High hardware utilization makes sure accelerators don’t sit idle and needlessly increase costs. To optimize the inference system throughput, the team improved both single-host throughput as well as load balancing efficiency.
Load balancing for LLM inference is tricky due to following challenges. First, a single host can only handle a limited number of concurrent requests. Second, the end-to-end latency to complete one request can vary, spanning many seconds depending on the LLM response length.
To address the challenges, the team optimized throughput by considering both single-host throughput and throughput across many hosts using load balancing.
The team used the least outstanding requests (LOR) routing algorithm from ALB, increasing throughput by five times faster in comparison to an earlier baseline measurement. This allows each host to have enough time to process in-flight requests and stream back responses using a gRPC connection, without getting overwhelmed by multiple requests received at the same time. Rufus also collaborated with AWS and vLLM teams to improve single-host concurrency using vLLM integration with the Neuron SDK and NVIDIA Triton Inference Server.
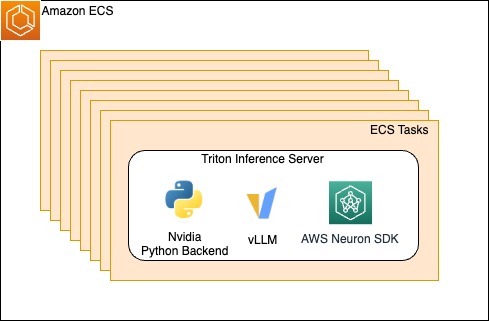
Figure 1. ECS tasks scale horizontally hosting the Triton Inference Server and dependencies
With this integration, Rufus was able to benefit from a critical optimization: continuous batching. Continuous batching allows a single host to greatly increase throughput. In addition, continuous batching provides unique capabilities in comparison to other batch techniques, such as static batching. For example, when using static batching, the time to first token (TTFT) increases linearly with the number of requests in one batch. Continuous batching prioritizes the prefill stage for LLM inference, keeping TTFT under control even with more requests running at the same time. This helped Rufus provide a pleasant experience with low latency when generating the first response, and improve the single-host throughput to keep serving costs under control.
Conclusion
In this post, we discussed how Rufus is able to reliably deploy and serve its multi-billion-parameter LLM using the Neuron SDK with Inferentia2 and Trainium chips and AWS services. Rufus continues to evolve with advancements in generative AI and customer feedback and we encourage you to use Inferentia and Trainium.
Learn more about how we are innovating with generative AI across Amazon.
About the author
James Park is a Solutions Architect at Amazon Web Services. He works with Amazon.com to design, build, and deploy technology solutions on AWS, and has a particular interest in AI and machine learning. In his spare time, he enjoys seeking out new cultures, new experiences, and staying up to date with the latest technology trends.
RJ is an Engineer within Amazon. He builds and optimizes systems for distributed systems for training and works on optimizing adopting systems to reduce latency for ML Inference. Outside work, he is exploring using Generative AI for building food recipes.
Yang Zhou is a software engineer working on building and optimizing machine learning systems. His recent focus is enhancing the performance and cost efficiency of generative AI inference. Beyond work, he enjoys traveling and has recently discovered a passion for running long distances.
Adam (Hongshen) Zhao is a Software Development Manager at Amazon Stores Foundational AI. In his current role, Adam is leading Rufus Inference team to build GenAI inference optimization solutions and inference system at scale for fast inference at low cost. Outside work, he enjoys traveling with his wife and art creations.
Faqin Zhong is a software engineer at Amazon Stores Foundational AI, working on Large Language Model (LLM) inference infrastructure and optimizations. Passionate about Generative AI technology, Faqin collaborates with leading teams to drive innovations, making LLMs more accessible and impactful, ultimately enhancing customer experiences across diverse applications. Outside of work she enjoys cardio exercise and baking with her son.
Nicolas Trown is an engineer in Amazon Stores Foundational AI. His recent focus is lending his systems expertise across Rufus to aid Rufus Inference team and efficient utilization across the Rufus experience. Outside of work he enjoys spending time with his wife and day trips to nearby coast, Napa, and Sonoma areas.
Bing Yin is a director of science at Amazon Stores Foundational AI. He leads the effort to build LLMs that are specialized for shopping use cases and optimized for inference at Amazon scale. Outside of work, he enjoys running marathon races.