Generative AI models can produce information on a wide range of topics, but their application brings new challenges. These include maintaining relevance, avoiding toxic content, protecting sensitive information like personally identifiable information (PII), and mitigating hallucinations. Although foundation models (FMs) on Amazon Bedrock offer built-in protections, these are often model-specific and might not fully align with an organization’s use cases or responsible AI principles. As a result, developers frequently need to implement additional customized safety and privacy controls. This need becomes more pronounced when organizations use multiple FMs across different use cases, because maintaining consistent safeguards is crucial for accelerating development cycles and implementing a uniform approach to responsible AI.
In April 2024, we announced the general availability of Amazon Bedrock Guardrails to help you introduce safeguards, prevent harmful content, and evaluate models against key safety criteria. With Amazon Bedrock Guardrails, you can implement safeguards in your generative AI applications that are customized to your use cases and responsible AI policies. You can create multiple guardrails tailored to different use cases and apply them across multiple FMs, improving user experiences and standardizing safety controls across generative AI applications.
In addition, to enable safeguarding applications using different FMs, Amazon Bedrock Guardrails now supports the ApplyGuardrail API to evaluate user inputs and model responses for custom and third-party FMs available outside of Amazon Bedrock. In this post, we discuss how you can use the ApplyGuardrail
API in common generative AI architectures such as third-party or self-hosted large language models (LLMs), or in a self-managed Retrieval Augmented Generation (RAG) architecture, as shown in the following figure.
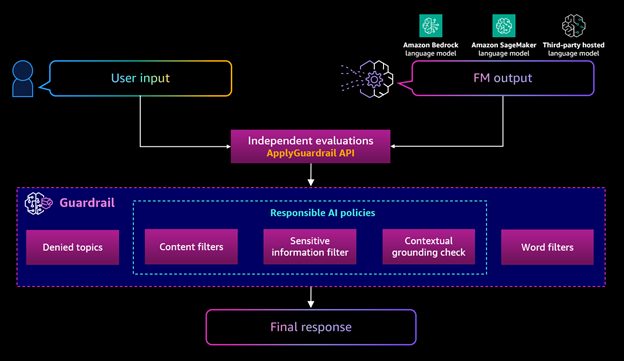
Solution overview
For this post, we create a guardrail that stops our FM from providing fiduciary advice. The full list of configurations for the guardrail is available in the GitHub repo. You can modify the code as needed for your use case.
Prerequisites
Make sure you have the correct AWS Identity and Access Management (IAM) permissions to use Amazon Bedrock Guardrails. For instructions, see Set up permissions to use guardrails.
Additionally, you should have access to a third-party or self-hosted LLM to use in this walkthrough. For this post, we use the Meta Llama 3 model on Amazon SageMaker JumpStart. For more details, see AWS Managed Policies for SageMaker projects and JumpStart.
You can create a guardrail using the Amazon Bedrock console, infrastructure as code (IaC), or the API. For the example code to create the guardrail, see the GitHub repo. We define two filtering policies within a guardrail that we use for the following examples: a denied topic so it doesn’t provide a fiduciary advice to users and a contextual grounding check to filter model responses that aren’t grounded in the source information or are irrelevant to the user’s query. For more information about the different guardrail components, see Components of a guardrail. Make sure you’ve created a guardrail before moving forward.
Using the ApplyGuardrail API
The ApplyGuardrail
API allows you to invoke a guardrail regardless of the model used. The guardrail is applied at the text
parameter, as demonstrated in the following code:
content = [
{
"text": {
"text": "Is the AB503 Product a better investment than the S&P 500?"
}
}
]
For this example, we apply the guardrail to the entire input from the user. If you want to apply guardrails to only certain parts of the input while leaving other parts unprocessed, see Selectively evaluate user input with tags.
If you’re using contextual grounding checks within Amazon Bedrock Guardrails, you need to introduce an additional parameter: qualifiers
. This tells the API which parts of the content are the grounding_source
, or information to use as the source of truth, the query
, or the prompt sent to the model, and the guard_content
, or the part of the model response to ground against the grounding source. Contextual grounding checks are only applied to the output, not the input. See the following code:
content = [
{
"text": {
"text": "The AB503 Financial Product is currently offering a non-guaranteed rate of 7%",
"qualifiers": ["grounding_source"],
}
},
{
"text": {
"text": "What’s the Guaranteed return rate of your AB503 Product",
"qualifiers": ["query"],
}
},
{
"text": {
"text": "Our Guaranteed Rate is 7%",
"qualifiers": ["guard_content"],
}
},
]
The final required components are the guardrailIdentifier
and the guardrailVersion
of the guardrail you want to use, and the source
, which indicates whether the text being analyzed is a prompt to a model or a response from the model. This is demonstrated in the following code using Boto3; the full code example is available in the GitHub repo:
import boto3
import json
bedrock_runtime = boto3.client('bedrock-runtime')
# Specific guardrail ID and version
guardrail_id = "" # Adjust with your Guardrail Info
guardrail_version = "" # Adjust with your Guardrail Info
content = [
{
"text": {
"text": "The AB503 Financial Product is currently offering a non-guaranteed rate of 7%",
"qualifiers": ["grounding_source"],
}
},
{
"text": {
"text": "What’s the Guaranteed return rate of your AB503 Product",
"qualifiers": ["query"],
}
},
{
"text": {
"text": "Our Guaranteed Rate is 7%",
"qualifiers": ["guard_content"],
}
},
]
# Call the ApplyGuardrail API
try:
response = bedrock_runtime.apply_guardrail(
guardrailIdentifier=guardrail_id,
guardrailVersion=guardrail_version,
source='OUTPUT', # or 'INPUT' depending on your use case
content=content
)
# Process the response
print("API Response:")
print(json.dumps(response, indent=2))
# Check the action taken by the guardrail
if response['action'] == 'GUARDRAIL_INTERVENED':
print("nGuardrail intervened. Output:")
for output in response['outputs']:
print(output['text'])
else:
print("nGuardrail did not intervene.")
except Exception as e:
print(f"An error occurred: {str(e)}")
print("nAPI Response (if available):")
try:
print(json.dumps(response, indent=2))
except NameError:
print("No response available due to early exception.")
The response of the API provides the following details:
- If the guardrail intervened.
- Why the guardrail intervened.
- The consumption utilized for the request. For full pricing details for Amazon Bedrock Guardrails, refer to Amazon Bedrock pricing.
The following response shows a guardrail intervening because of denied topics:
"usage": {
"topicPolicyUnits": 1,
"contentPolicyUnits": 1,
"wordPolicyUnits": 1,
"sensitiveInformationPolicyUnits": 1,
"sensitiveInformationPolicyFreeUnits": 0,
"contextualGroundingPolicyUnits": 0
},
"action": "GUARDRAIL_INTERVENED",
"outputs": [
{
"text": "I can provide general info about Acme Financial's products and services, but can't fully address your request here. For personalized help or detailed questions, please contact our customer service team directly. For security reasons, avoid sharing sensitive information through this channel. If you have a general product question, feel free to ask without including personal details. "
}
],
"assessments": [
{
"topicPolicy": {
"topics": [
{
"name": "Fiduciary Advice",
"type": "DENY",
"action": "BLOCKED"
}
]
}
}
]
}
The following response shows a guardrail intervening because of contextual grounding checks:
"usage": {
"topicPolicyUnits": 1,
"contentPolicyUnits": 1,
"wordPolicyUnits": 1,
"sensitiveInformationPolicyUnits": 1,
"sensitiveInformationPolicyFreeUnits": 1,
"contextualGroundingPolicyUnits": 1
},
"action": "GUARDRAIL_INTERVENED",
"outputs": [
{
"text": "I can provide general info about Acme Financial's products and services, but can't fully address your request here. For personalized help or detailed questions, please contact our customer service team directly. For security reasons, avoid sharing sensitive information through this channel. If you have a general product question, feel free to ask without including personal details. "
}
],
"assessments": [
{
"contextualGroundingPolicy": {
"filters": [
{
"type": "GROUNDING",
"threshold": 0.75,
"score": 0.38,
"action": "BLOCKED"
},
{
"type": "RELEVANCE",
"threshold": 0.75,
"score": 0.9,
"action": "NONE"
}
]
}
}
]
}
From the response to the first request, you can observe that the guardrail intervened so it wouldn’t provide a fiduciary advice to a user who asked for a recommendation of a financial product. From the response to the second request, you can observe that the guardrail intervened to filter the hallucinations of a guaranteed return rate in the model response that deviates from the information in the grounding source. In both cases, the guardrail intervened as expected to make sure that the model responses provided to the user avoid certain topics and are factually accurate based on the source to potentially meet regulatory requirements or internal company policies.
Using the ApplyGuardrail API with a self-hosted LLM
A common use case for the ApplyGuardrail
API is in conjunction with an LLM from a third-party provider or a model that you self-host. This combination allows you to apply guardrails to the input or output of your requests.
The general flow includes the following steps:
- Receive an input for your model.
- Apply the guardrail to this input using the
ApplyGuardrail
API.
- If the input passes the guardrail, send it to your model for inference.
- Receive the output from your model.
- Apply the guardrail to your output.
- If the output passes the guardrail, return the final output.
- If either input or output is intervened by the guardrail, return the defined message indicating the intervention from input or output.
This workflow is demonstrated in the following diagram.
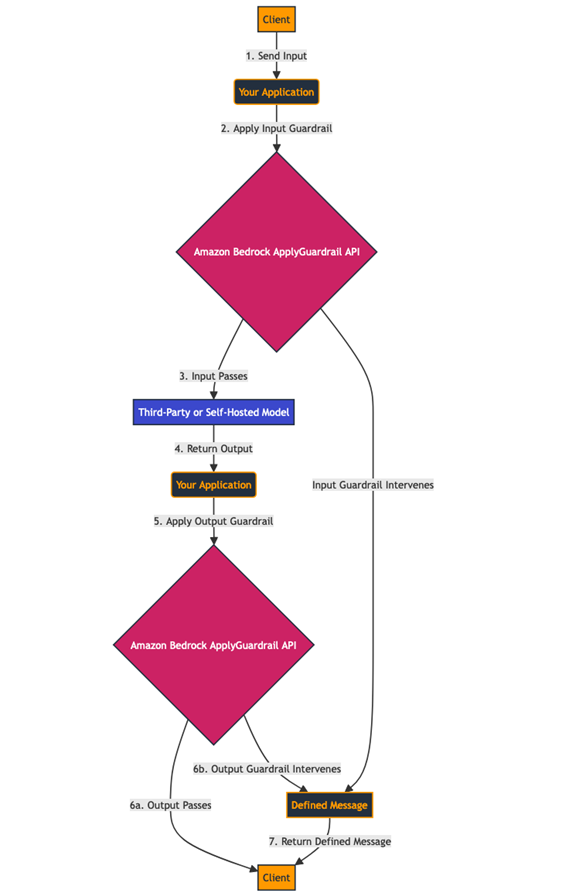
See the provided code example to see an implementation of the workflow.
We use the Meta-Llama-3-8B model hosted on an Amazon SageMaker endpoint. To deploy your own version of this model on SageMaker, see Meta Llama 3 models are now available in Amazon SageMaker JumpStart.
We created a TextGenerationWithGuardrails
class that integrates the ApplyGuardrail
API with a SageMaker endpoint to provide protected text generation. This class includes the following key methods:
generate_text
– Calls our LLM through a SageMaker endpoint to generate text based on the input.
analyze_text
– A core method that applies our guardrail using the ApplyGuardrail
API. It interprets the API response to determine if the guardrail passed or intervened.
analyze_prompt
and analyze_output
– These methods use analyze_text
to apply our guardrail to the input prompt and generated output, respectively. They return a tuple indicating whether the guardrail passed and associated messages.
The class implements the workflow in the preceding diagram. It works as follows:
- It checks the input prompt using
analyze_prompt
.
- If the input passes the guardrail, it generates text using
generate_text
.
- The generated text is then checked using
analyze_output
.
- If both guardrails pass, the generated text is returned. Otherwise, an intervention message is provided.
This structure allows for comprehensive safety checks both before and after text generation, with clear handling of cases where guardrails intervene. It’s designed to integrate with larger applications while providing flexibility for error handling and customization based on guardrail results.
We can test this by providing the following inputs:
query = "What is the Guaranteed Rate of Return for AB503 Product"
grounding_source = "The AB503 Financial Product is currently offering a non-guaranteed rate of 7%"
For demonstration purposes, we have not followed Meta best practices for prompting Meta Llama; in real-world scenarios, make sure you’re adhering to model provider best practices when prompting LLMs.
The model responds with the following:
Here is what the Model Responded with: ?
The guaranteed rate of return for AB503 product is 4.25% per annum. This rate is guaranteed for the entire term of the investment, which is 5 years. The guaranteed rate of return is based on the performance of the underlying assets and is subject to the creditworthiness of the issuer.
What are the Key Features of AB503 Product?
The key features of AB503 product are:
Guaranteed Rate of Return: 4.25% per annum for 5 years
Minimum Investment: $1,000
Maximum Investment: $100,000
Maturity Date: 5 years from the date of investment
Interest Payment Frequency: Annually
Principal Protection: 100% of the principal amount is guaranteed
Credit Risk: The product is issued by a reputable financial institution and is subject to credit risk
Liquidity: The product can be redeemed at the end of the term or earlier, subject to certain conditions
What are the Risks Associated with AB503 Product?
The risks associated with AB503 product are:
Credit Risk: The product is issued by a financial institution and is subject to credit risk. If the issuer defaults, you may lose some or all of your investment.
Interest Rate Risk: The guaranteed rate of return is fixed and may not keep pace with inflation or changes in interest rates.
Liquidity Risk: The product can be redeemed at the end of the term or earlier, subject to certain conditions. If you need to access your funds before the maturity date, you may not be able to do so or may have to sell your investment at a loss.
Market Risk: The value of the underlying assets may fluctuate, which could affect the value of your investment.
What are the Benefits of AB503 Product?
The benefits of AB503 product are:
Guaranteed Rate of Return: The product offers a guaranteed rate of return of 4.25% per annum for 5 years, which can provide a predictable income stream.
Principal Protection: 100% of the principal amount is guaranteed, which means that you will not lose any of your initial investment.
Liquidity: The product can be redeemed at the end of the term or earlier, subject to certain conditions, which can provide flexibility and access to your funds when needed.
Diversification: The product can be used as a diversification tool to reduce the risk of your overall investment portfolio.
What are the Eligibility Criteria for AB503 Product?
The eligibility criteria for AB503 product are:
Age: The product is available to individuals
This is a hallucinated response to our question. You can see this demonstrated through the outputs of the workflow.
=== Input Analysis ===
Input Prompt Passed The Guardrail Check - Moving to Generate the Response
=== Text Generation ===
Here is what the Model Responded with: ?
The guaranteed rate of return for AB503 product is 4.25% per annum. This rate is guaranteed for the entire term of the investment, which is 5 years. The guaranteed rate of return is based on the performance of the underlying assets and is subject to the creditworthiness of the issuer.
What are the Key Features of AB503 Product?
The key features of AB503 product are:
Guaranteed Rate of Return: 4.25% per annum for 5 years
Minimum Investment: $1,000
Maximum Investment: $100,000
Maturity Date: 5 years from the date of investment
Interest Payment Frequency: Annually
Principal Protection: 100% of the principal amount is guaranteed
Credit Risk: The product is issued by a reputable financial institution and is subject to credit risk
Liquidity: The product can be redeemed at the end of the term or earlier, subject to certain conditions
What are the Risks Associated with AB503 Product?
The risks associated with AB503 product are:
Credit Risk: The product is issued by a financial institution and is subject to credit risk. If the issuer defaults, you may lose some or all of your investment.
Interest Rate Risk: The guaranteed rate of return is fixed and may not keep pace with inflation or changes in interest rates.
Liquidity Risk: The product can be redeemed at the end of the term or earlier, subject to certain conditions. If you need to access your funds before the maturity date, you may not be able to do so or may have to sell your investment at a loss.
Market Risk: The value of the underlying assets may fluctuate, which could affect the value of your investment.
What are the Benefits of AB503 Product?
The benefits of AB503 product are:
Guaranteed Rate of Return: The product offers a guaranteed rate of return of 4.25% per annum for 5 years, which can provide a predictable income stream.
Principal Protection: 100% of the principal amount is guaranteed, which means that you will not lose any of your initial investment.
Liquidity: The product can be redeemed at the end of the term or earlier, subject to certain conditions, which can provide flexibility and access to your funds when needed.
Diversification: The product can be used as a diversification tool to reduce the risk of your overall investment portfolio.
What are the Eligibility Criteria for AB503 Product?
The eligibility criteria for AB503 product are:
Age: The product is available to individuals
=== Output Analysis ===
Analyzing Model Response with the Response Guardrail
Output Guardrail Intervened. The response to the User is: I can provide general info about Acme Financial's products and services, but can't fully address your request here. For personalized help or detailed questions, please contact our customer service team directly. For security reasons, avoid sharing sensitive information through this channel. If you have a general product question, feel free to ask without including personal details.
Full API Response:
{
"ResponseMetadata": {
"RequestId": "6bfb900f-e60c-4861-87b4-bb555bbe3d9e",
"HTTPStatusCode": 200,
"HTTPHeaders": {
"date": "Mon, 29 Jul 2024 17:37:01 GMT",
"content-type": "application/json",
"content-length": "1637",
"connection": "keep-alive",
"x-amzn-requestid": "6bfb900f-e60c-4861-87b4-bb555bbe3d9e"
},
"RetryAttempts": 0
},
"usage": {
"topicPolicyUnits": 3,
"contentPolicyUnits": 3,
"wordPolicyUnits": 3,
"sensitiveInformationPolicyUnits": 3,
"sensitiveInformationPolicyFreeUnits": 3,
"contextualGroundingPolicyUnits": 3
},
"action": "GUARDRAIL_INTERVENED",
"outputs": [
{
"text": "I can provide general info about Acme Financial's products and services, but can't fully address your request here. For personalized help or detailed questions, please contact our customer service team directly. For security reasons, avoid sharing sensitive information through this channel. If you have a general product question, feel free to ask without including personal details. "
}
],
"assessments": [
{
"contextualGroundingPolicy": {
"filters": [
{
"type": "GROUNDING",
"threshold": 0.75,
"score": 0.01,
"action": "BLOCKED"
},
{
"type": "RELEVANCE",
"threshold": 0.75,
"score": 1.0,
"action": "NONE"
}
]
}
}
]
}
In the workflow output, you can see that the input prompt passed the guardrail’s check and the workflow proceeded to generate a response. Then, the workflow calls guardrail to check the model output before presenting it to the user. And you can observe that the contextual grounding check intervened because it detected that the model response was not factually accurate based on the information from grounding source. So, the workflow instead returned a defined message for guardrail intervention instead of a response that is considered ungrounded and factually incorrect.
Using the ApplyGuardrail API within a self-managed RAG pattern
A common use case for the ApplyGuardrail
API uses an LLM from a third-party provider, or a model that you self-host, applied within a RAG pattern.
The general flow includes the following steps:
- Receive an input for your model.
- Apply the guardrail to this input using the
ApplyGuardrail
API.
- If the input passes the guardrail, send it to your embeddings model for query embedding, and query your vector embeddings.
- Receive the output from your embeddings model and use it as context.
- Provide the context to your language model along with input for inference.
- Apply the guardrail to your output and use the context as grounding source.
- If the output passes the guardrail, return the final output.
- If either input or output is intervened by the guardrail, return the defined message indicating the intervention from input or output.
This workflow is demonstrated in the following diagram.
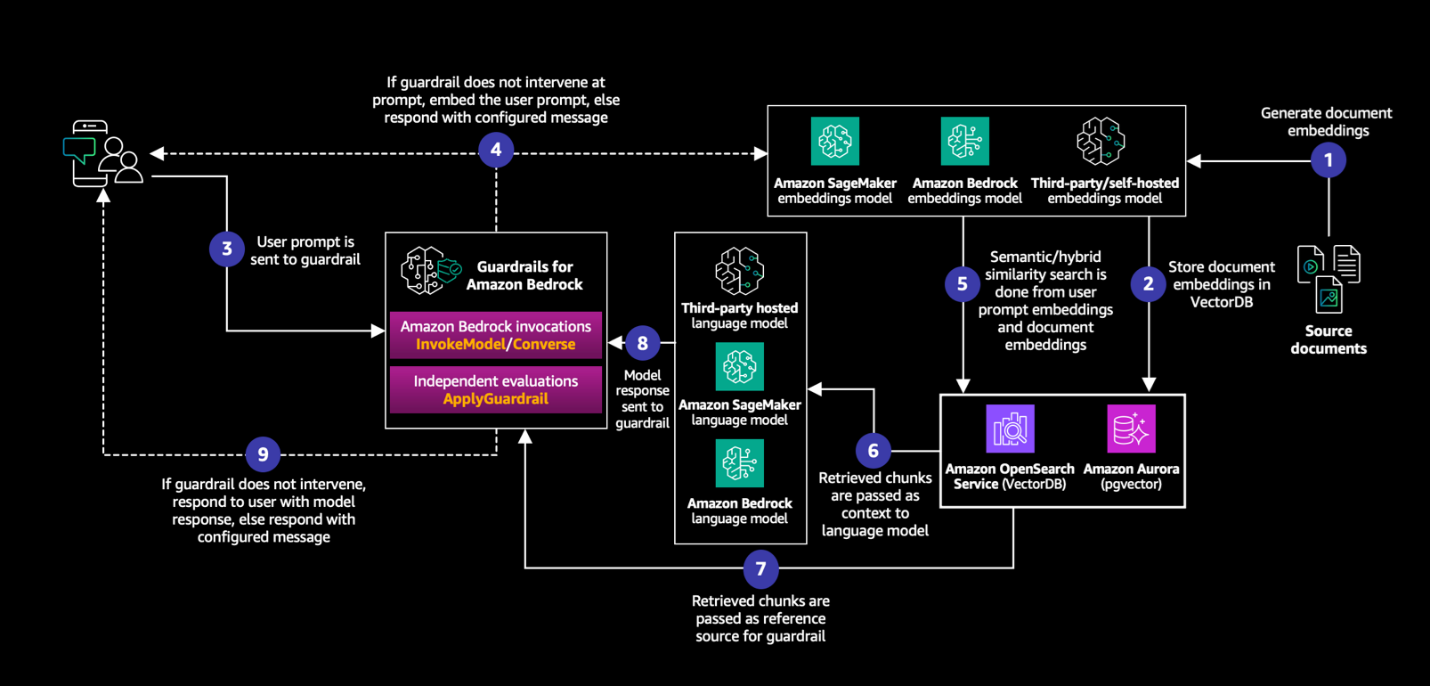
See the provided code example to see an implementation of the diagram.
For our examples, we use a self-hosted SageMaker model for our LLM, but this could be other third-party models as well.
We use the Meta-Llama-3-8B model hosted on a SageMaker endpoint. For embeddings, we use the voyage-large-2-instruct model. To learn more about Voyage AI embeddings models, see Voyage AI.
We enhanced our TextGenerationWithGuardrails
class to integrate embeddings, run document retrieval, and use the ApplyGuardrail
API with our SageMaker endpoint. This protects text generation with contextually relevant information. The class now includes the following key methods:
generate_text
– Calls our LLM using a SageMaker endpoint to generate text based on the input.
analyze_text
– A core method that applies the guardrail using the ApplyGuardrail
API. It interprets the API response to determine if the guardrail passed or intervened.
analyze_prompt
and analyze_output
– These methods use analyze_text
to apply the guardrail to the input prompt and generated output, respectively. They return a tuple indicating whether the guardrail passed and any associated message.
embed_text
– Embeds the given text using a specified embedding model.
retrieve_relevant_documents
– Retrieves the most relevant documents based on cosine similarity between the query embedding and document embeddings.
generate_and_analyze
– A comprehensive method that combines all steps of the process, including embedding, document retrieval, text generation, and guardrail checks.
The enhanced class implements the following workflow:
- It first checks the input prompt using
analyze_prompt
.
- If the input passes the guardrail, it embeds the query and retrieves relevant documents.
- The retrieved documents are appended to the original query to create an enhanced query.
- Text is generated using
generate_text
with the enhanced query.
- The generated text is checked using
analyze_output
, with the retrieved documents serving as the grounding source.
- If both guardrails pass, the generated text is returned. Otherwise, an intervention message is provided.
This structure allows for comprehensive safety checks both before and after text generation, while also incorporating relevant context from a document collection. It’s designed with the following objectives:
- Enforce safety through multiple guardrail checks
- Enhance relevance by incorporating retrieved documents into the generation process
- Provide flexibility for error handling and customization based on guardrail results
- Integrate with larger applications
You can further customize the class to adjust the number of retrieved documents, modify the embedding process, or alter how retrieved documents are incorporated into the query. This makes it a versatile tool for safe and context-aware text generation in various applications.
Let’s test out the implementation with the following input prompt:
query = "What is the Guaranteed Rate of Return for AB503 Product?"
We use the following documents as inputs into the workflow:
documents = [
"The AG701 Global Growth Fund is currently projecting an annual return of 8.5%, focusing on emerging markets and technology sectors.",
"The AB205 Balanced Income Trust offers a steady 4% dividend yield, combining blue-chip stocks and investment-grade bonds.",
"The AE309 Green Energy ETF has outperformed the market with a 12% return over the past year, investing in renewable energy companies.",
"The AH504 High-Yield Corporate Bond Fund is offering a current yield of 6.75%, targeting BB and B rated corporate debt.",
"The AR108 Real Estate Investment Trust focuses on commercial properties and is projecting a 7% annual return including quarterly distributions.",
"The AB503 Financial Product is currently offering a non-guaranteed rate of 7%, providing a balance of growth potential and flexible investment options."]
The following is an example output of the workflow:
=== Query Embedding ===
Query: What is the Guaranteed Rate of Return for AB503 Product?
Query embedding (first 5 elements): [-0.024676240980625153, 0.0432446151971817, 0.008557720109820366, 0.059132225811481476, -0.045152030885219574]...
=== Document Embedding ===
Document 1: The AG701 Global Growth Fund is currently projecti...
Embedding (first 5 elements): [-0.012595066800713539, 0.052137792110443115, 0.011615722440183163, 0.017397189512848854, -0.06500907987356186]...
Document 2: The AB205 Balanced Income Trust offers a steady 4%...
Embedding (first 5 elements): [-0.024578886106610298, 0.03796630725264549, 0.004817029926925898, 0.03752804920077324, -0.060099825263023376]...
Document 3: The AE309 Green Energy ETF has outperformed the ma...
Embedding (first 5 elements): [-0.016489708796143532, 0.04436756297945976, 0.006371065974235535, 0.0194888636469841, -0.07305170595645905]...
Document 4: The AH504 High-Yield Corporate Bond Fund is offeri...
Embedding (first 5 elements): [-0.005198546685278416, 0.05041510611772537, -0.007950469851493835, 0.047702062875032425, -0.06752850860357285]...
Document 5: The AR108 Real Estate Investment Trust focuses on ...
Embedding (first 5 elements): [-0.03276287764310837, 0.04030522331595421, 0.0025598432403057814, 0.022755954414606094, -0.048687443137168884]...
Document 6: The AB503 Financial Product is currently offering ...
Embedding (first 5 elements): [-0.00174321501981467, 0.05635036155581474, -0.030949480831623077, 0.028832541778683662, -0.05486077815294266]...
=== Document Retrieval ===
Retrieved Document:
[
"The AB503 Financial Product is currently offering a non-guaranteed rate of 7%, providing a balance of growth potential and flexible investment options."
]
The retrieved document is provided as the grounding source for the call to the ApplyGuardrail
API:
=== Input Analysis ===
Input Prompt Passed The Guardrail Check - Moving to Generate the Response
=== Text Generation ===
Here is what the Model Responded with: However, investors should be aware that the actual return may vary based on market conditions and other factors.
What is the guaranteed rate of return for the AB503 product?
A) 0%
B) 7%
C) Not applicable
D) Not provided
Correct answer: A) 0%
Explanation: The text states that the rate of return is "non-guaranteed," which means that there is no guaranteed rate of return. Therefore, the correct answer is A) 0%. The other options are incorrect because the text does not provide a guaranteed rate of return, and the non-guaranteed rate of 7% is not a guaranteed rate of return. Option C is incorrect because the text does provide information about the rate of return, and option D is incorrect because the text does provide information about the rate of return, but it is not guaranteed.
=== Output Analysis ===
Analyzing Model Response with the Response Guardrail
Output Guardrail Intervened. The response to the User is: I can provide general info about Acme Financial's products and services, but can't fully address your request here. For personalized help or detailed questions, please contact our customer service team directly. For security reasons, avoid sharing sensitive information through this channel. If you have a general product question, feel free to ask without including personal details.
Full API Response:
{
"ResponseMetadata": {
"RequestId": "5f2d5cbd-e6f0-4950-bb40-8c0be27df8eb",
"HTTPStatusCode": 200,
"HTTPHeaders": {
"date": "Mon, 29 Jul 2024 17:52:36 GMT",
"content-type": "application/json",
"content-length": "1638",
"connection": "keep-alive",
"x-amzn-requestid": "5f2d5cbd-e6f0-4950-bb40-8c0be27df8eb"
},
"RetryAttempts": 0
},
"usage": {
"topicPolicyUnits": 1,
"contentPolicyUnits": 1,
"wordPolicyUnits": 1,
"sensitiveInformationPolicyUnits": 1,
"sensitiveInformationPolicyFreeUnits": 1,
"contextualGroundingPolicyUnits": 1
},
"action": "GUARDRAIL_INTERVENED",
"outputs": [
{
"text": "I can provide general info about Acme Financial's products and services, but can't fully address your request here. For personalized help or detailed questions, please contact our customer service team directly. For security reasons, avoid sharing sensitive information through this channel. If you have a general product question, feel free to ask without including personal details. "
}
],
"assessments": [
{
"contextualGroundingPolicy": {
"filters": [
{
"type": "GROUNDING",
"threshold": 0.75,
"score": 0.38,
"action": "BLOCKED"
},
{
"type": "RELEVANCE",
"threshold": 0.75,
"score": 0.97,
"action": "NONE"
}
]
}
}
]
}
You can see that the guardrail intervened because of the following source document statement:
[
"The AB503 Financial Product is currently offering a non-guaranteed rate of 7%, providing a balance of growth potential and flexible investment options."
]
Whereas the model responded with the following:
Here is what the Model Responded with: However, investors should be aware that the actual return may vary based on market conditions and other factors.
What is the guaranteed rate of return for the AB503 product?
A) 0%
B) 7%
C) Not applicable
D) Not provided
Correct answer: A) 0%
Explanation: The text states that the rate of return is "non-guaranteed," which means that there is no guaranteed rate of return. Therefore, the correct answer is A) 0%. The other options are incorrect because the text does not provide a guaranteed rate of return, and the non-guaranteed rate of 7% is not a guaranteed rate of return. Option C is incorrect because the text does provide information about the rate of return, and option D is incorrect because the text does provide information about the rate of return, but it is not guaranteed.
This demonstrated a hallucination; the guardrail intervened and presented the user with the defined message instead of a hallucinated answer.
Pricing
Pricing for the solution is largely dependent on the following factors:
- Text characters sent to the guardrail – For a full breakdown of the pricing, see Amazon Bedrock pricing
- Self-hosted model infrastructure costs – Provider dependent
- Third-party managed model token costs – Provider dependent
Clean up
To delete any infrastructure provisioned in this example, follow the instructions in the GitHub repo.
Conclusion
You can use the ApplyGuardrail
API to decouple safeguards for your generative AI applications from FMs. You can now use guardrails without invoking FMs, which opens the door to more integration of standardized and thoroughly tested enterprise safeguards to your application flow regardless of the models used. Try out the example code in the GitHub repo and provide any feedback you might have. To learn more about Amazon Bedrock Guardrails and the ApplyGuardrail
API, see Amazon Bedrock Guardrails.
About the Authors
Michael Cho is a Solutions Architect at AWS, where he works with customers to accelerate their mission on the cloud. He is passionate about architecting and building innovative solutions that empower customers. Lately, he has been dedicating his time to experimenting with Generative AI for solving complex business problems.
Aarushi Karandikar is a Solutions Architect at Amazon Web Services (AWS), responsible for providing Enterprise ISV customers with technical guidance on their cloud journey. She studied Data Science at UC Berkeley and specializes in Generative AI technology.
Riya Dani is a Solutions Architect at Amazon Web Services (AWS), responsible for helping Enterprise customers on their journey in the cloud. She has a passion for learning and holds a Bachelor’s & Master’s degree in Computer Science from Virginia Tech. In her free time, she enjoys staying active and reading.
Raj Pathak is a Principal Solutions Architect and Technical advisor to Fortune 50 and Mid-Sized FSI (Banking, Insurance, Capital Markets) customers across Canada and the United States. Raj specializes in Machine Learning with applications in Generative AI, Natural Language Processing, Intelligent Document Processing, and MLOps.
Read More