At Amazon and AWS, we are always finding innovative ways to build inclusive technology. With voice assistants like Amazon Alexa, we are enabling more people to ask questions and get answers on the spot without having to type. Whether you’re a person with a motor disability, juggling multiple tasks, or simply away from your computer, getting search results without typing is a valuable feature. With modern voice assistants, you can now ask your questions conversationally and get verbal answers instantly.
In this post, we discuss voice-guided applications. Specifically, we focus on chatbots. Chatbots are no longer a niche technology. They are now ubiquitous on customer service websites, providing around-the-clock automated assistance. Although AI chatbots have been around for years, recent advances of large language models (LLMs) like generative AI have enabled more natural conversations. Chatbots are proving useful across industries, handling both general and industry-specific questions. Voice-based assistants like Alexa demonstrate how we are entering an era of conversational interfaces. Typing questions already feels cumbersome to many who prefer the simplicity and ease of speaking with their devices.
We explore how to build a fully serverless, voice-based contextual chatbot tailored for individuals who need it. We also provide a sample chatbot application. The application is available in the accompanying GitHub repository. We create an intelligent conversational assistant that can understand and respond to voice inputs in a contextually relevant manner. The AI assistant is powered by Amazon Bedrock. This chatbot is designed to assist users with various tasks, provide information, and offer personalized support based on their unique requirements. For our LLM, we use Anthropic Claude on Amazon Bedrock.
We demonstrate the process of integrating Anthropic Claude’s advanced natural language processing capabilities with the serverless architecture of Amazon Bedrock, enabling the deployment of a highly scalable and cost-effective solution. Additionally, we discuss techniques for enhancing the chatbot’s accessibility and usability for people with motor disabilities. The aim of this post is to provide a comprehensive understanding of how to build a voice-based, contextual chatbot that uses the latest advancements in AI and serverless computing.
We hope that this solution can help people with certain mobility disabilities. A limited level of interaction is still required, and specific identification of start and stop talking operations is required. In our sample application, we address this by having a dedicated Talk button that performs the transcription process while being pressed.
For people with significant motor disabilities, the same operation can be implemented with a dedicated physical button that can be pressed by a single finger or another body part. Alternatively, a special keyword can be said to indicate the beginning of the command. This approach is used when you communicate with Alexa. The user always starts the conversation with “Alexa.”
Solution overview
The following diagram illustrates the architecture of the solution.
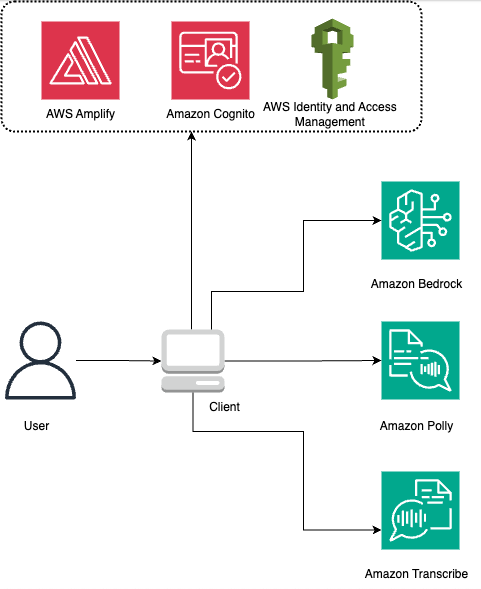
To deploy this architecture, we need managed compute that can host the web application, authentication mechanisms, and relevant permissions. We discuss this later in the post.
All the services that we use are serverless and fully managed by AWS. You don’t need to provision the compute resources. You only consume the services through their API. All the calls to the services are made directly from the client application.
The application is a simple React application that we create using the Vite build tool. We use the AWS SDK for JavaScript to call the services. The solution uses the following major services:
- Amazon Polly is a service that turns text into lifelike speech.
- Amazon Transcribe is an AWS AI service that makes it straightforward to convert speech to text.
- Amazon Bedrock is a fully managed service that offers a choice of high-performing foundation models (FMs) along with a broad set of capabilities that you need to build generative AI applications.
- Amazon Cognito is an identity service for web and mobile apps. It’s a user directory, an authentication server, and an authorization service for OAuth 2.0 access tokens and AWS credentials.
To consume AWS services, the user needs to obtain temporary credentials from AWS Identity and Access Management (IAM). This is possible due to the Amazon Cognito identity pool, which acts as a mediator between your application user and IAM services. The identity pool holds the information about the IAM roles with all permissions necessary to run the solution.
Amazon Polly and Amazon Transcribe don’t require additional setup from the client aside from what we have described. However, Amazon Bedrock requires named user authentication. This means that having an Amazon Cognito identity pool is not enough—you also need to use the Amazon Cognito user pool, which allows you to define users and bind them to the Amazon Cognito identity pool. To understand better how Amazon Cognito allows external applications to invoke AWS services, refer to refer to Secure API Access with Amazon Cognito Federated Identities, Amazon Cognito User Pools, and Amazon API Gateway.
The heavy lifting of provisioning the Amazon Cognito user pool and identity pool, including generating the sign-in UI for the React application, is done by AWS Amplify. Amplify consists of a set of tools (open source framework, visual development environment, console) and services (web application and static website hosting) to accelerate the development of mobile and web applications on AWS. We cover the steps of setting Amplify in the next sections.
Prerequisites
Before you begin, complete the following prerequisites:
- Make sure you have the following installed:
- Create an IAM role to use in the Amazon Cognito identity pool. Use the least privilege principal to provide only the minimum set of permissions needed to run the application.
- To invoke Amazon Bedrock, use the following code:
{
"Version": "2012-10-17",
"Statement": [
{
"Sid": "VisualEditor1",
"Effect": "Allow",
"Action": "bedrock:InvokeModel",
"Resource": "*"
}
]
}
- To invoke Amazon Polly, use the following code:
{
"Version": "2012-10-17",
"Statement": [
{
"Sid": "VisualEditor2",
"Effect": "Allow",
"Action": "polly:SynthesizeSpeech",
"Resource": "*"
}
]
}
- To invoke Amazon Transcribe, use the following code:
{
"Version": "2012-10-17",
"Statement": [
{
"Sid": "VisualEditor3",
"Effect": "Allow",
"Action": "transcribe:StartStreamTranscriptionWebSocket",
"Resource": "*"
}
]
}
The full policy JSON should look as follows:
{
"Version": "2012-10-17",
"Statement": [
{
"Sid": "VisualEditor1",
"Effect": "Allow",
"Action": "bedrock:InvokeModel",
"Resource": "*"
},
{
"Sid": "VisualEditor2",
"Effect": "Allow",
"Action": "polly:SynthesizeSpeech",
"Resource": "*"
},
{
"Sid": "VisualEditor3",
"Effect": "Allow",
"Action": "transcribe:StartStreamTranscriptionWebSocket",
"Resource": "*"
}
]
}
- Run the following command to clone the GitHub repository:
git clone https://github.com/aws-samples/serverless-conversational-chatbot.git
- To use Amplify, refer to Set up Amplify CLI to complete the initial setup.
- To be consistent with the values that you use later in the instructions, call your AWS profile
amplify
when you see the following prompt.

- Create the role
amplifyconsole-backend-role
with the AdministratorAccess-Amplify
managed policy, which allows Amplify to create the necessary resources.
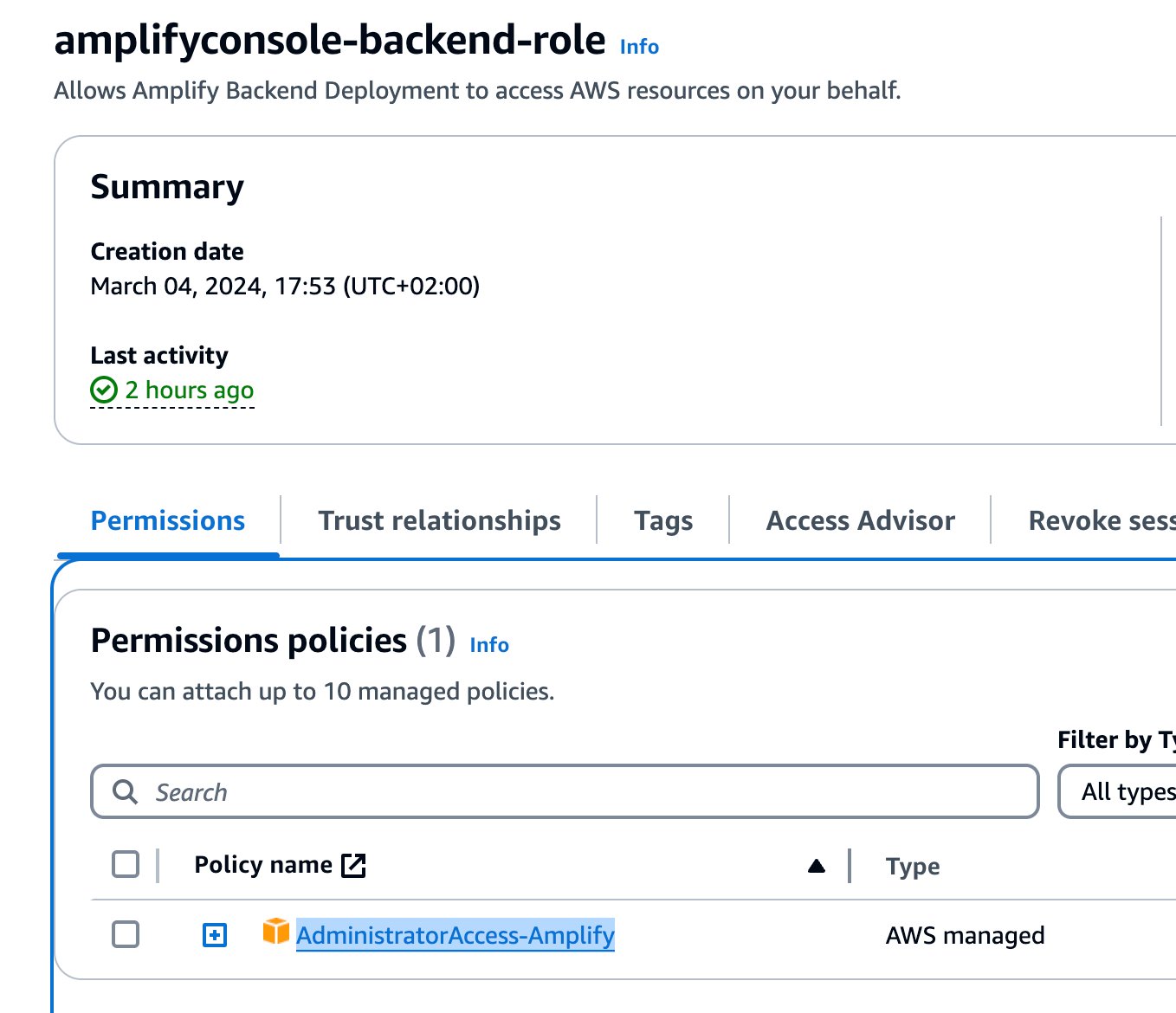
- For this post, we use the Anthropic Claude 3 Haiku LLM. To enable the LLM in Amazon Bedrock, refer to Access Amazon Bedrock foundation models.
Deploy the solution
There are two options to deploy the solution:
- Use Amplify to deploy the application automatically
- Deploy the application manually
We provide the steps for both options in this section.
Deploy the application automatically using Amplify
Amplify can deploy the application automatically if it’s stored in GitHub, Bitbucket, GitLab, or AWS CodeCommit. Upload the application that you downloaded earlier to your preferred repository (from the aforementioned options). For instructions, see Getting started with deploying an app to Amplify Hosting.
You can now continue to the next section of this post to set up IAM permissions.
Deploy the application manually
If you don’t have access to one of the storage options that we mentioned, you can deploy the application manually. This can also be useful if you want to modify the application to better fit your use case.
We tested the deployment on AWS Cloud9, a cloud integrated development environment (IDE) for writing, running, and debugging code, with Ubuntu Server 22.04 and Amazon Linux 2023.
We use the Visual Studio Code IDE and run all the following commands directly in the terminal window inside the IDE, but you can also run the commands in the terminal of your choice.
- From the directory where you checked out the application on GitHub, run the following command:
cd serverless-conversational-chatbot
- Run the following commands:
- Follow the prompts as shown in the following screenshot.
- For authentication, choose the AWS profile
amplify
that you created as part of the prerequisite steps.
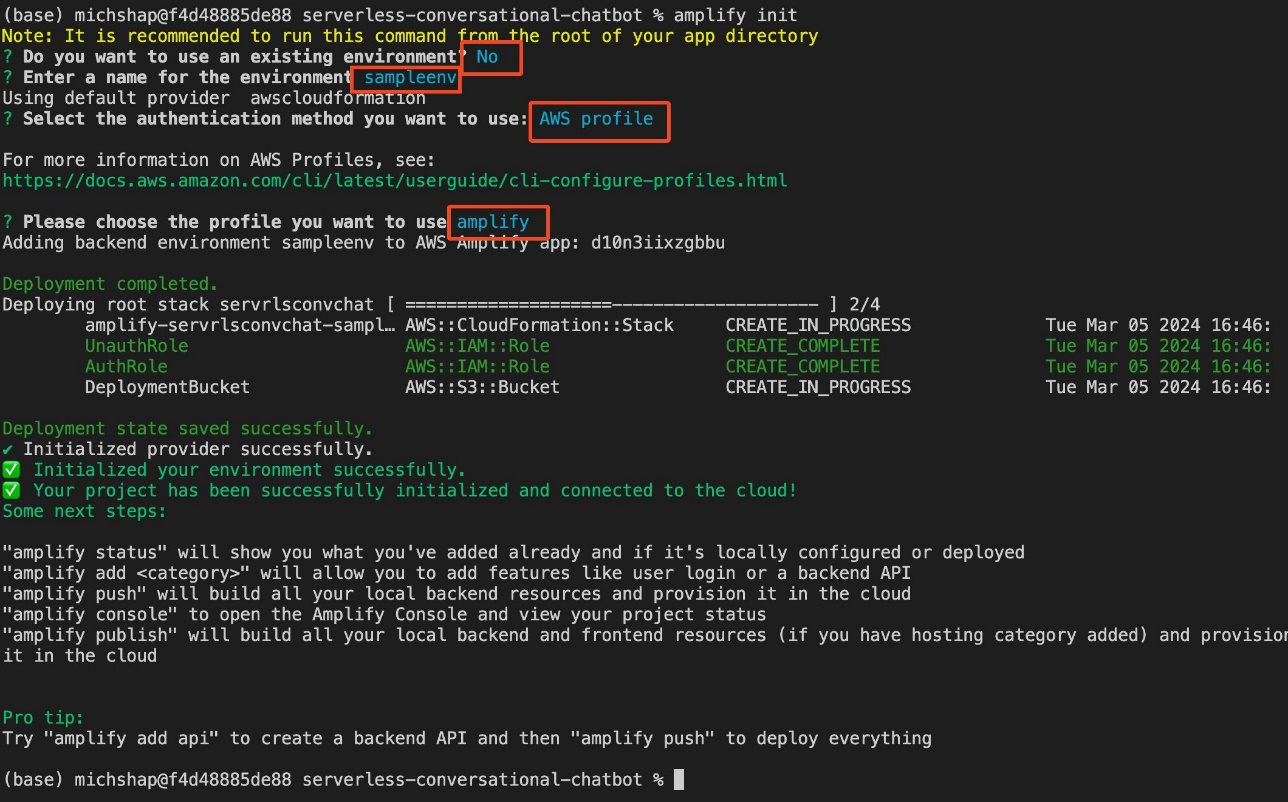
- Two new files will appear in the project under the
src
folder:
amplifyconfiguration.json
aws-exports.js
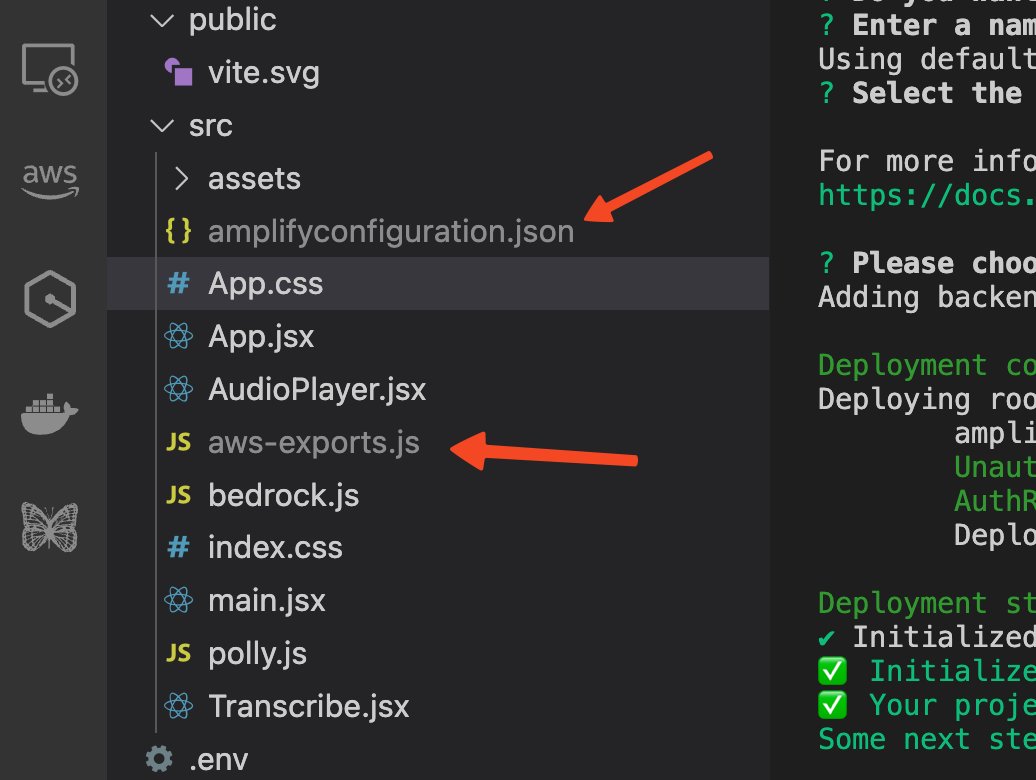
- Next run the following command:
amplify configure project
Then select “Project Information”

- Enter the following information:
Which setting do you want to configure? Project information
Enter a name for the project: servrlsconvchat
Choose your default editor: Visual Studio Code
Choose the type of app that you're building: javascript
What javascript framework are you using: react
Source Directory Path: src
Distribution Directory Path: dist
Build Command: npm run-script build
Start Command: npm run-script start
You can use an existing Amazon Cognito identity pool and user pool or create new objects.
- For our application, run the following command:
If you get the following message, you can ignore it:
Auth has already been added to this project. To update run amplify update auth
- Choose Default configuration.

- Accept all options proposed by the prompt.
- Run the following command:
- Choose your hosting option.
You have two options to host the application. The application can be hosted to the Amplify console or to Amazon Simple Storage Service (Amazon S3) and then exposed through Amazon CloudFront.
Hosting with the Amplify console differs from CloudFront and Amazon S3. The Amplify console is a managed service providing continuous integration and delivery (CI/CD) and SSL certificates, prioritizing swift deployment of serverless web applications and backend APIs. In contrast, CloudFront and Amazon S3 offer greater flexibility and customization options, particularly for hosting static websites and assets with features like caching and distribution. CloudFront and Amazon S3 are preferable for intricate, high-traffic web applications with specific performance and security needs.
For this post, we use the Amplify console. To learn more about the deployment with Amazon S3 and Amazon CloudFront, refer to documentation.

Now you’re ready to publish the application. There is an option to publish the application to GitHub to support CI/CD pipelines. Amplify has built-in integration with GitHub and can redeploy the application automatically when you push the changes. For simplicity, we use manual deployment.
- Choose Manual deployment.
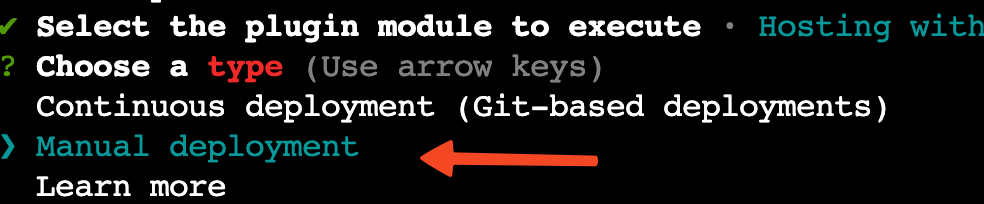
- Run the following command:
After the application is published, you will see the following output. Note down this URL to use in a later step.
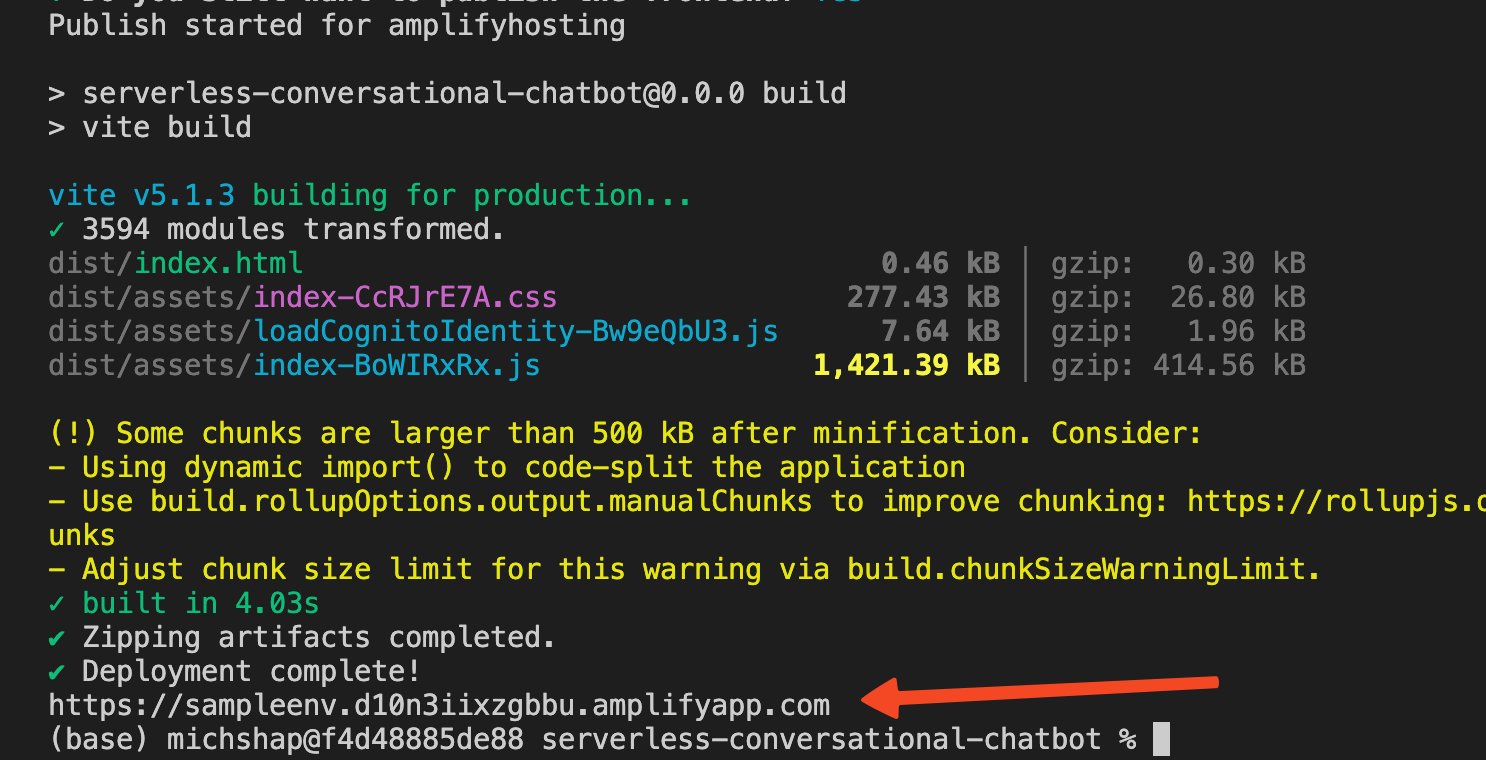
- Log in to the Amplify console, navigate to the
servrlsconvchat
application, and choose General under App settings in the navigation pane.
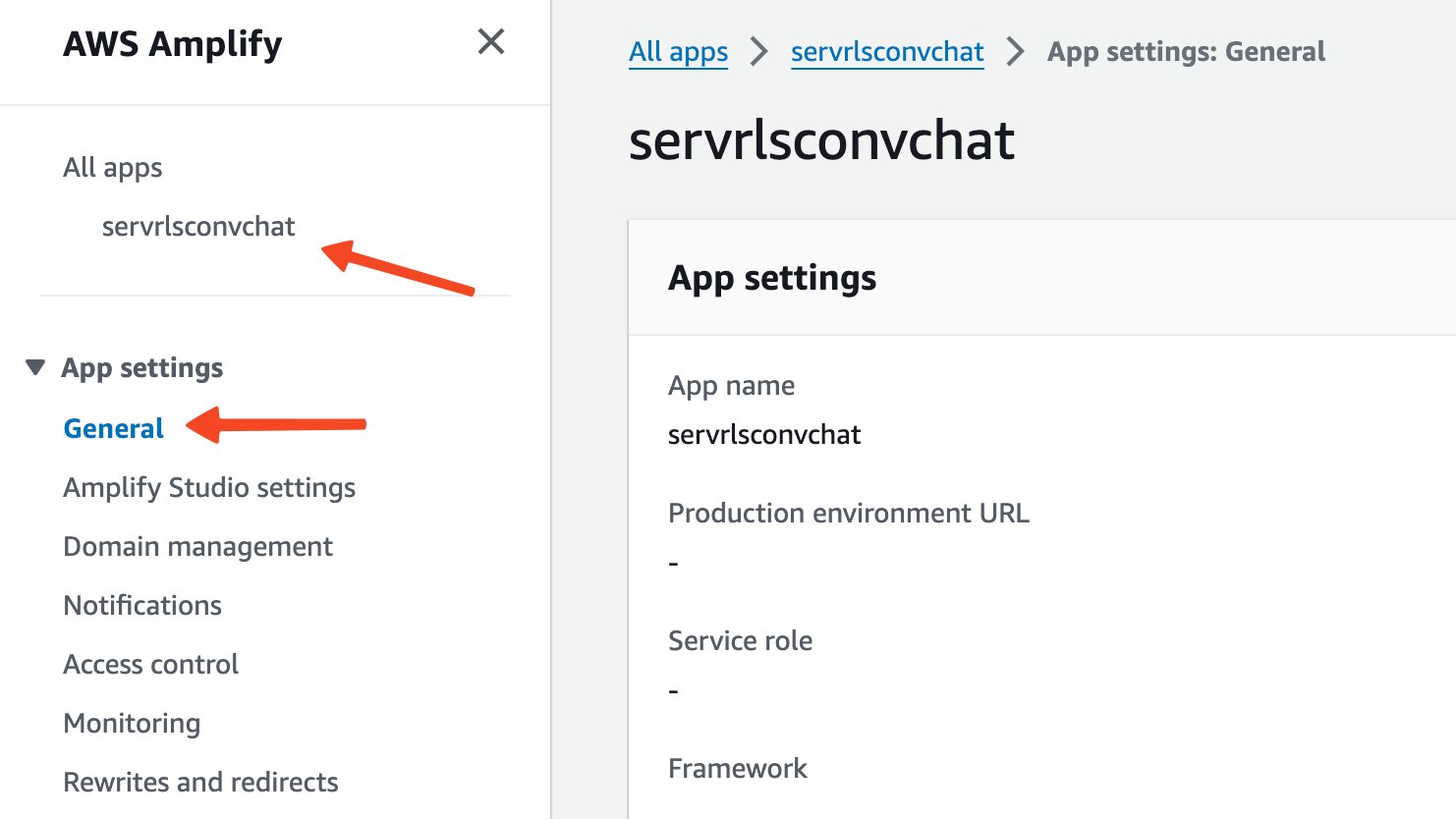
- Edit the app settings and enter
amplifyconsole-backend-role
for Service role (you created this role in the prerequisites section).
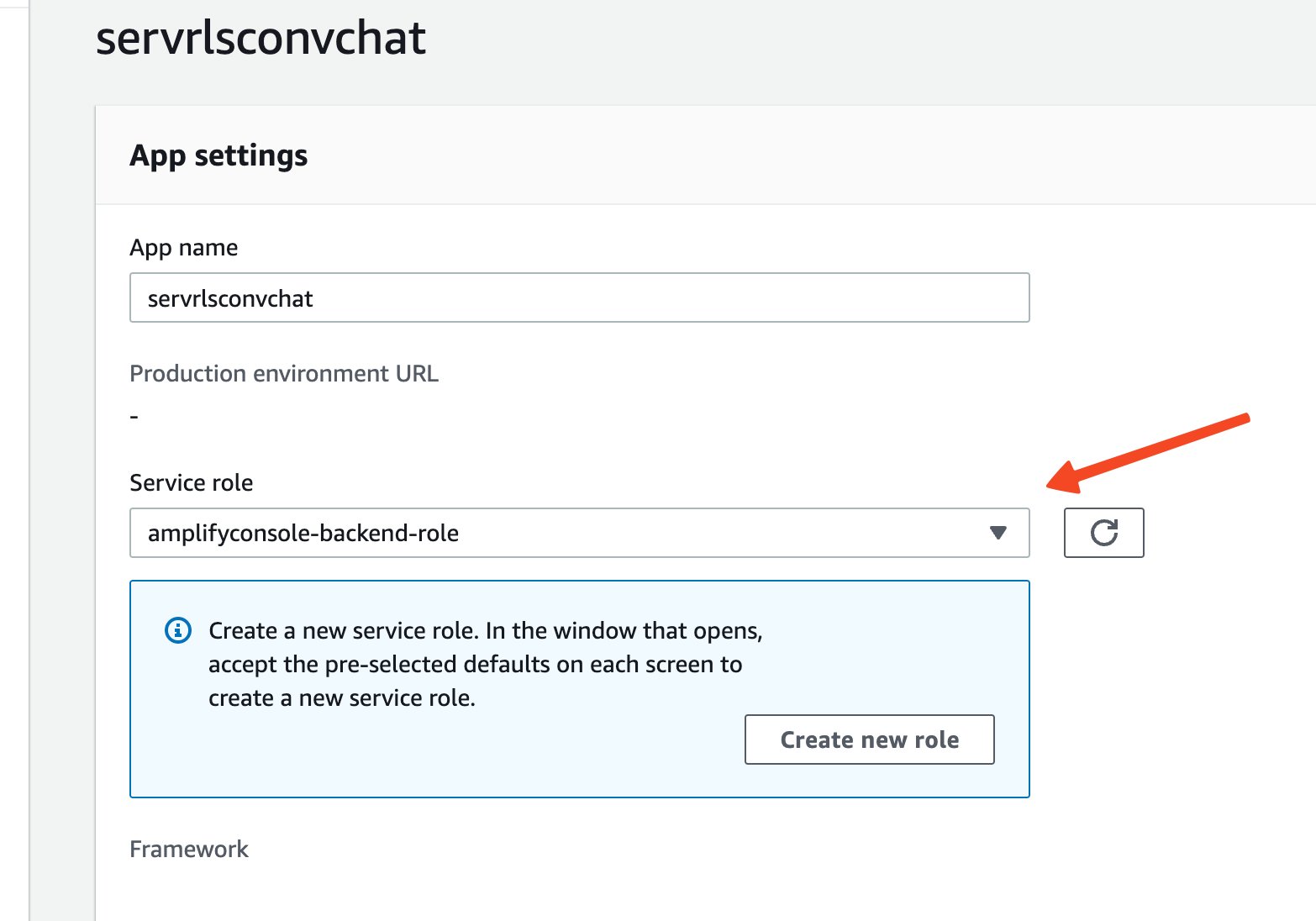
Now you can proceed to the next section to set up IAM permissions.
Configure IAM permissions
As part of the publishing method you completed, you provisioned a new identity pool. You can view this on the Amazon Cognito console, along with a new user pool. The names will be different from those presented in this post.
As we explained earlier, you need to attach policies to this role to allow interaction with Amazon Bedrock, Amazon Polly, and Amazon Transcribe. To set up IAM permissions, complete the following steps:
- On the Amazon Cognito console, choose Identity pools in the navigation pane.
- Navigate to your identity pool.
- On the User access tab, choose the link for the authenticated role.
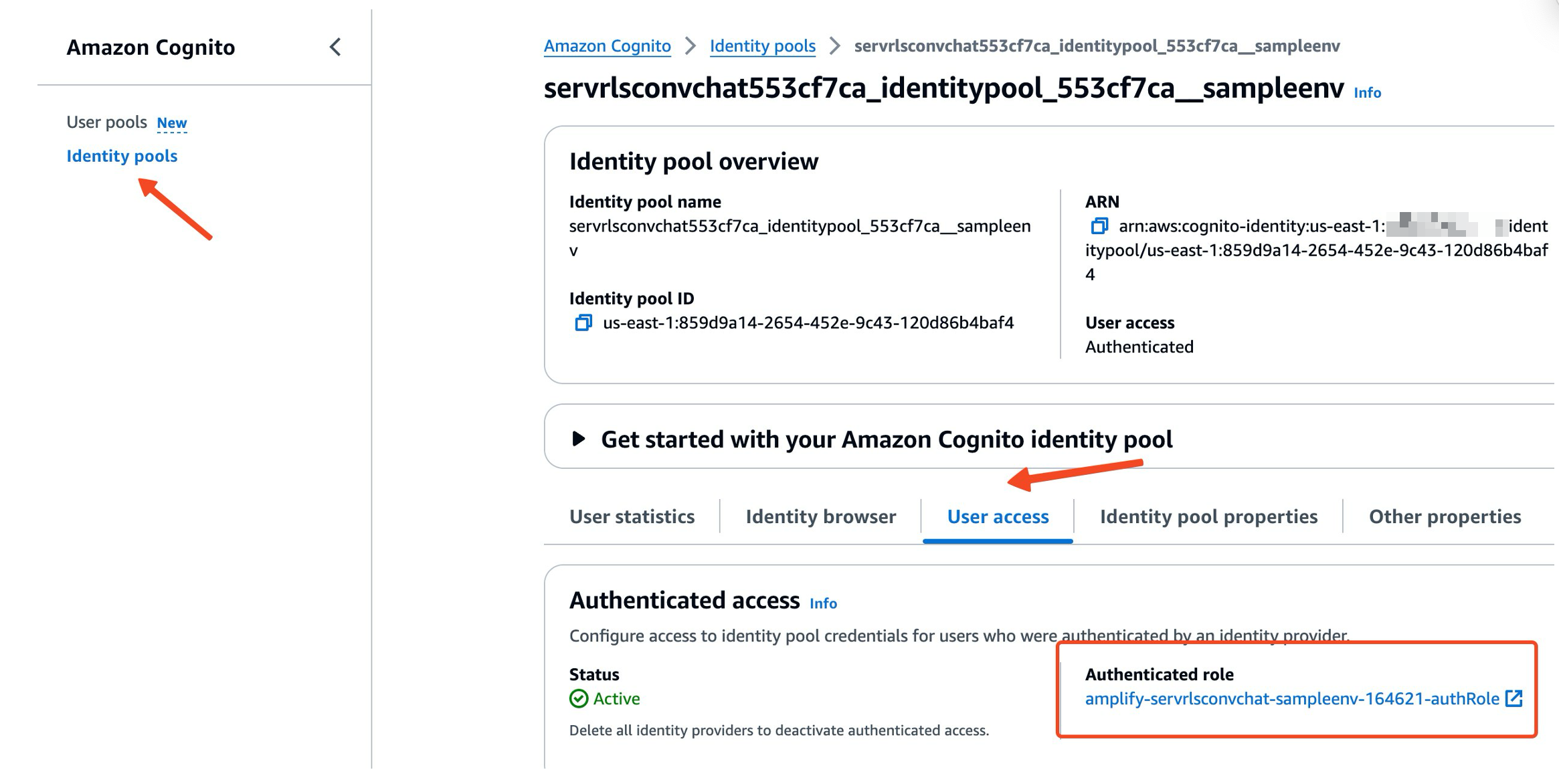
- Attach the policies that you defined in the prerequisites section.
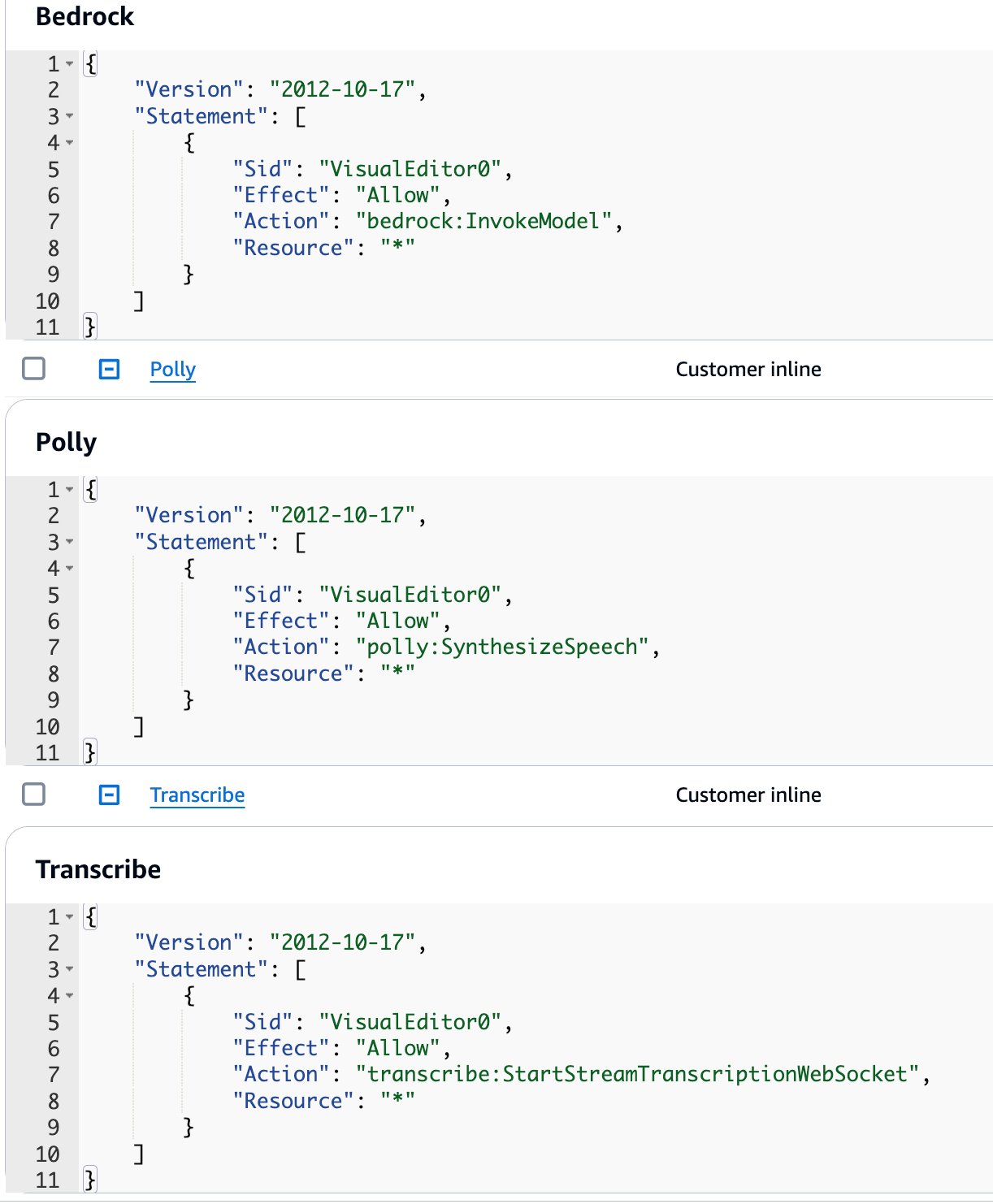
Amazon Bedrock can only be used with a named user, so we create a sample user in the Amazon Cognito user pool that was provisioned as part of the application publishing process.
- On the user pool details page, on the Users tab, choose Create user.
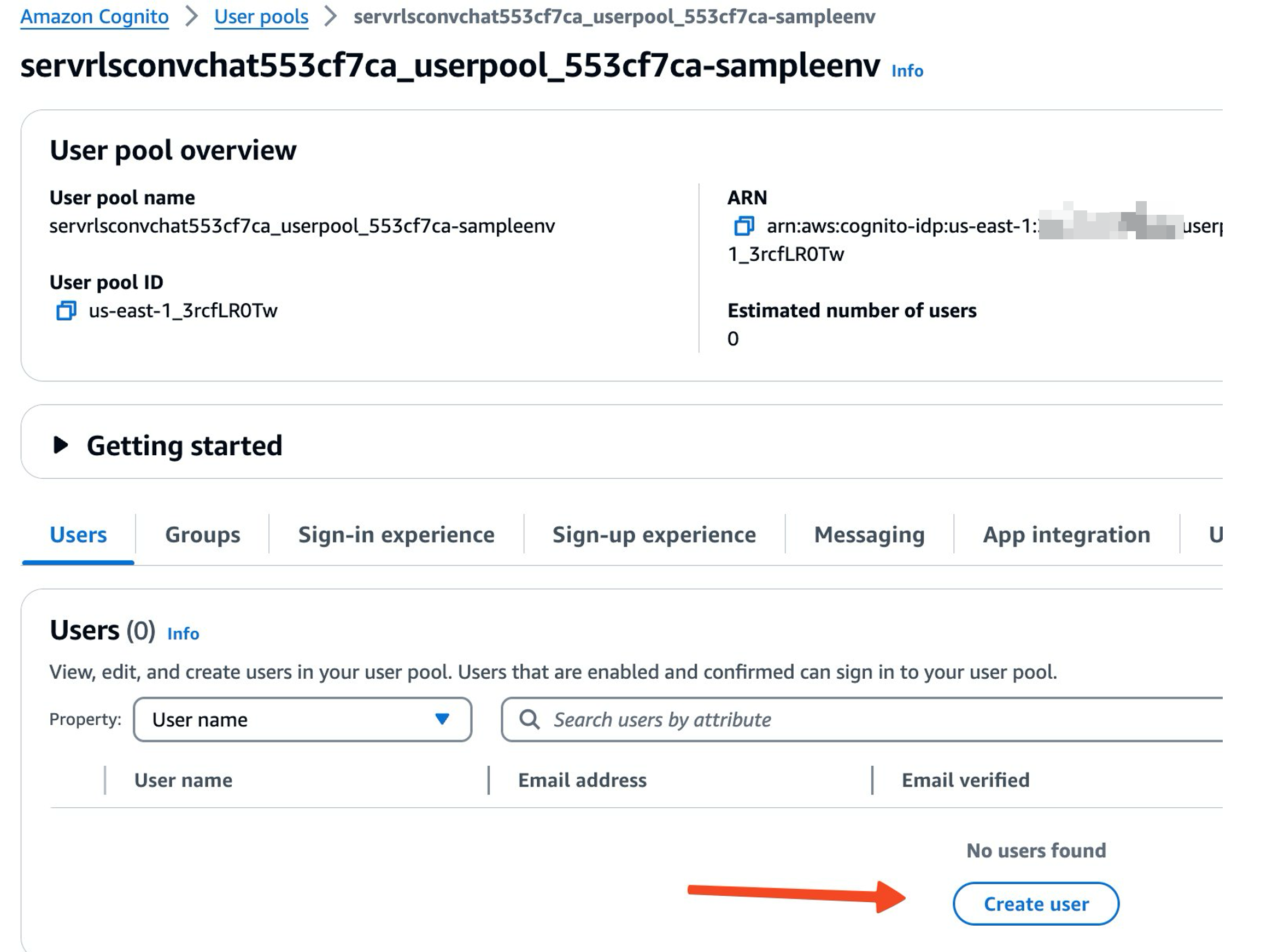
- Provide your user information.
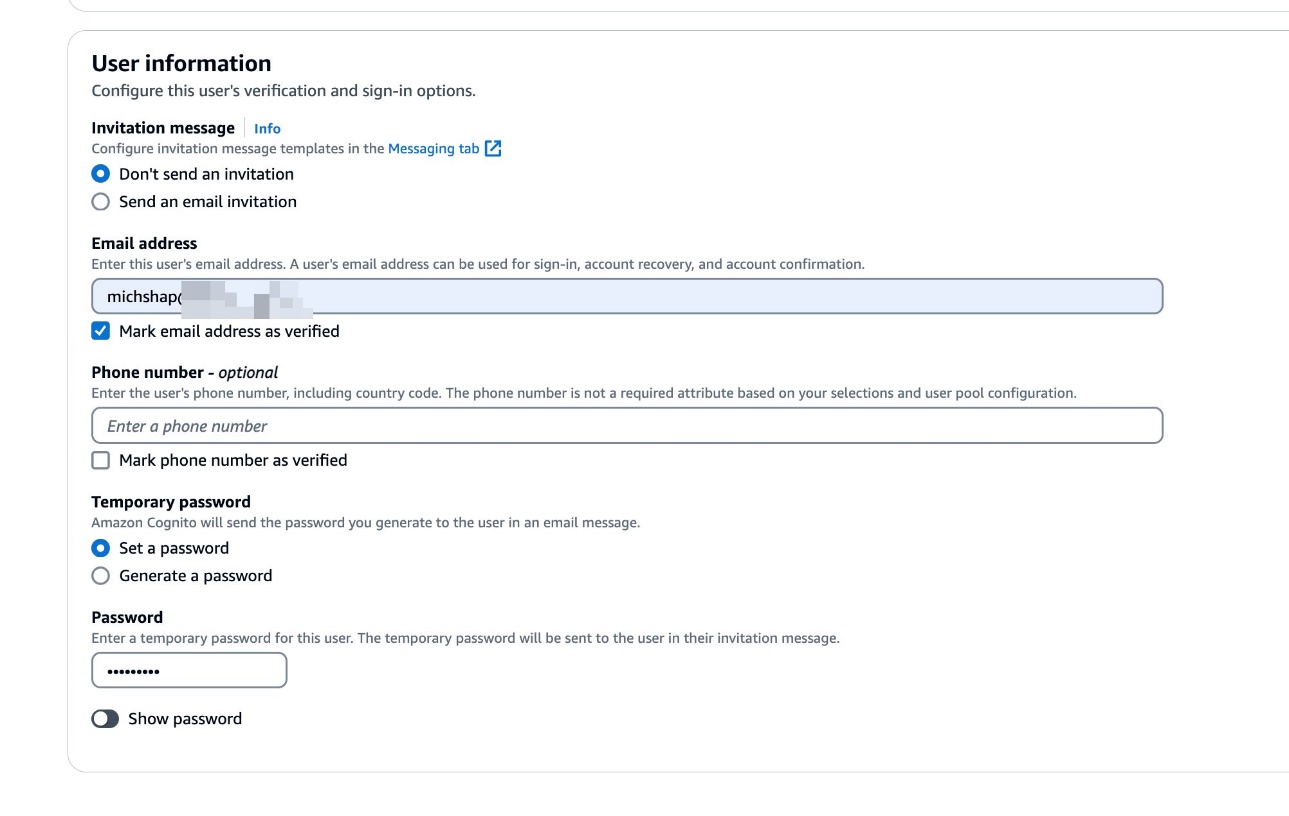
You’re now ready to run the application.
Use the sample serverless application
To access the application, navigate to the URL you saved from the output at the end of the application publishing process. Sign in to the application with the user you created in the previous step. You might be asked to change the password the first time you sign in.
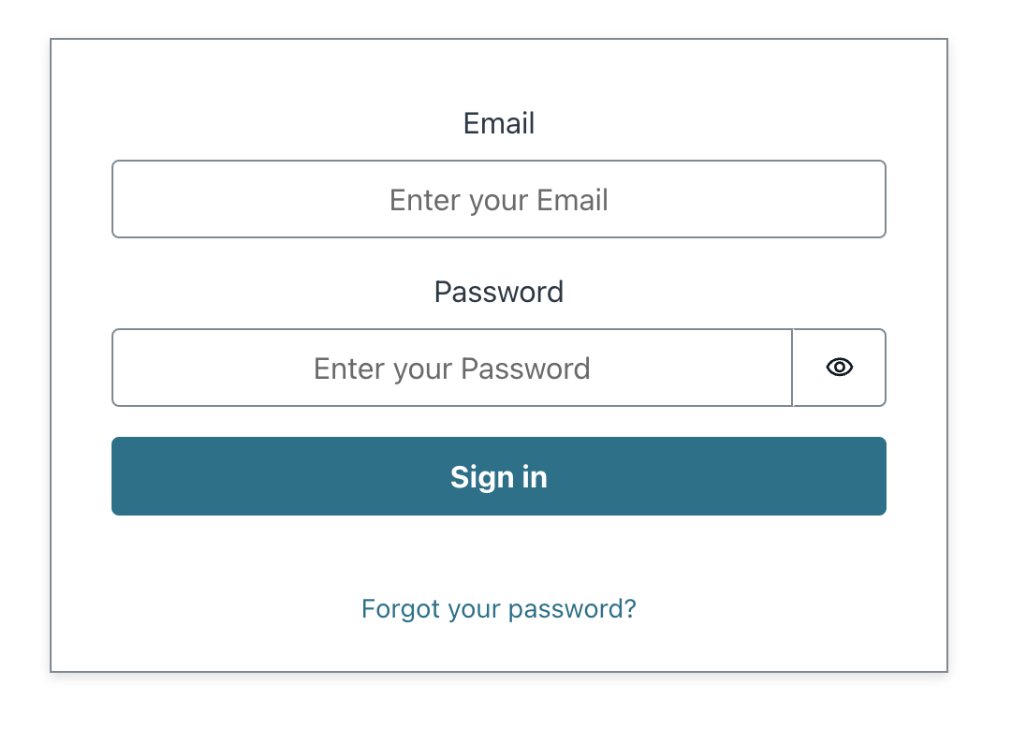
Use the Talk button and hold it while you’re asking the question. (We use this approach for the simplicity of demonstrating the abilities of the tool. For people with motor disabilities, we propose using a dedicated button that can be operated with different body parts, or a special keyword to initiate the conversation.)
When you release the button, the application sends your voice to Amazon Transcribe and returns the transcription text. This text is used as an input for an Amazon Bedrock LLM. For this example, we use Anthropic Claude 3 Haiku, but you can modify the code and use another model.
The response from Amazon Bedrock is displayed as text and is also spoken by Amazon Polly.
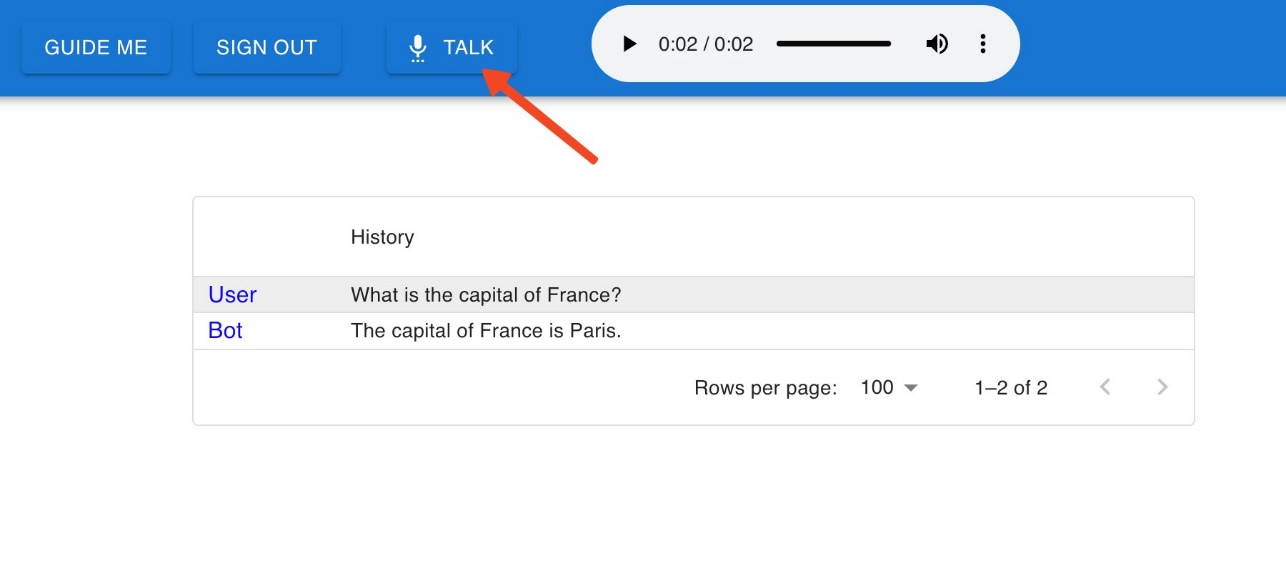
The conversation history is also stored. This means that you can ask follow-up questions, and the context of the conversation is preserved. For example, we asked, “What is the most famous tower there?” without specifying the location, and our chatbot was able to understand that the context of the question is Paris based on our previous question.
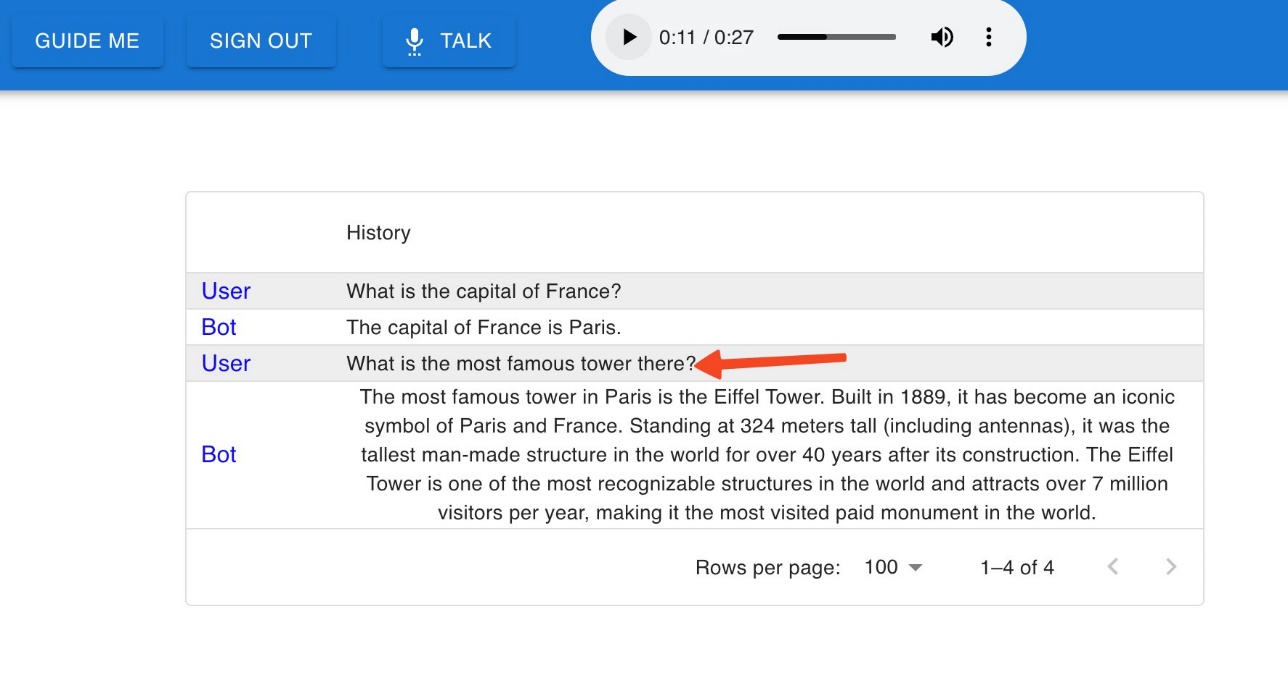
We store the conversation history inside a JavaScript variable, which means that if you refresh the page, the context will be lost. We discuss how to preserve the conversation context in a persistent way later in this post.
To identify that the transcription process is happening, choose and hold the Talk button. The color of the button changes and a microphone icon appears.
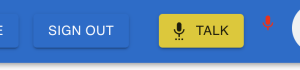
Clean up
To clean up your resources, run the following command from the same directory where you ran the Amplify commands:
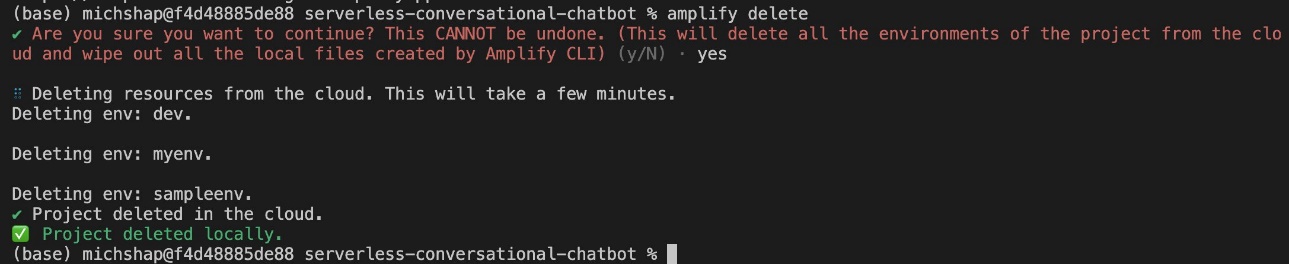
This command removes the Amplify settings from the React application, Amplify resources, and all Amazon Cognito objects, including the IAM role and Amazon Cognito user pool’s user.
Conclusion
In this post, we presented how to create a fully serverless voice-based contextual chatbot using Amazon Bedrock with Anthropic Claude.
This serves a starting point for a serverless and cost-effective solution. For example, you could extend the solution to have persistent conversational memory for your chats, such as Amazon DynamoDB. If you want to use a Retrieval Augmented Generation (RAG) approach, you can use Amazon Bedrock Knowledge Bases to securely connect FMs in Amazon Bedrock to your company data.
Another approach is to customize the model you use in Amazon Bedrock with your own data using fine-tuning or continued pre-training to build applications that are specific to your domain, organization, and use case. With custom models, you can create unique user experiences that reflect your company’s style, voice, and services.
For additional resources, refer to the following:
About the Author
Michael Shapira is a Senior Solution Architect covering general topics in AWS and part of the AWS Machine Learning community. He has 16 years’ experience in Software Development. He finds it fascinating to work with cloud technologies and help others on their cloud journey.
Eitan Sela is a Machine Learning Specialist Solutions Architect with Amazon Web Services. He works with AWS customers to provide guidance and technical assistance, helping them build and operate machine learning solutions on AWS. In his spare time, Eitan enjoys jogging and reading the latest machine learning articles.
Read More