Artificial intelligence will be the driving force behind India’s digital transformation, fueling innovation, economic growth, and global leadership, NVIDIA founder and CEO Jensen Huang said Thursday at NVIDIA’s AI Summit in Mumbai.
Addressing a crowd of entrepreneurs, developers, academics and business leaders, Huang positioned AI as the cornerstone of the country’s future.
India has an “amazing natural resource” in its IT and computer science expertise,” Huang said, noting the vast potential waiting to be unlocked.
To capitalize on this country’s talent and India’s immense data resources, the country’s leading cloud infrastructure providers are rapidly accelerating their data center capacity. NVIDIA is playing a key role, with NVIDIA GPU deployments expected to grow nearly 10x by year’s end, creating the backbone for an AI-driven economy.
Together with NVIDIA, these companies are at the cutting edge of a shift Huang compared to the seismic change in computing introduced by IBM’s System 360 in 1964, calling it the most profound platform shift since then.
“This industry, the computing industry, is going to become the intelligence industry,” Huang said, pointing to India’s unique strengths to lead this industry, thanks to its enormous amounts of data and large population.
With this rapid expansion in infrastructure, AI factories will play a critical role in India’s future, serving as the backbone of the nation’s AI-driven growth.
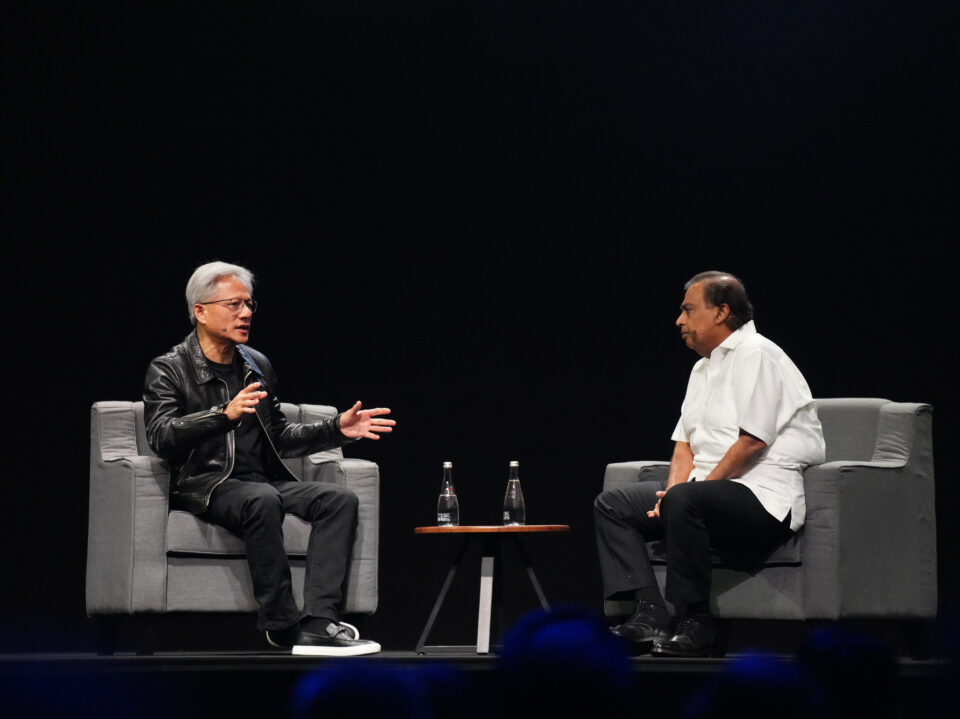
“It makes complete sense that India should manufacture its own AI,” Huang said. “You should not export data to import intelligence,” he added, noting the importance of India building its own AI infrastructure.
Huang identified three areas where AI will transform industries: sovereign AI, where nations use their own data to drive innovation; agentic AI, which automates knowledge-based work; and physical AI, which applies AI to industrial tasks through robotics and autonomous systems. India, Huang noted, is uniquely positioned to lead in all three areas.
India’s startups are already harnessing NVIDIA technology to drive innovation across industries and are positioning themselves as global players, bringing the country’s AI solutions to the world.
Meanwhile, India’s robotics ecosystem is adopting NVIDIA Isaac and Omniverse to power the next generation of physical AI, revolutionizing industries like manufacturing and logistics with advanced automation.
Huang’s also keynote featured a surprise appearance by actor and producer Akshay Kumar.
Following Huang’s remarks, the focus shifted to a fireside chat between Huang and Reliance Industries Chairman Mukesh Ambani, where the two leaders explored how AI will shape the future of Indian industries, particularly in sectors like energy, telecommunications and manufacturing.
Ambani emphasized that AI is central to this continued growth. Reliance, in partnership with NVIDIA, is building AI factories to automate industrial tasks and transform processes in sectors like energy and manufacturing.
Both men discussed their companies’ joint efforts to pioneer AI infrastructure in India.
Ambani underscored the role of AI in public sector services, explaining how India’s data combined with AI is already transforming governance and service delivery.
Huang added that AI promises to democratize technology.
“The ability to program AI is something that everyone can do … if AI could be put into the hands of every citizen, it would elevate and put into the hands of everyone this incredible capability,” he said.
Huang emphasized NVIDIA’s role in preparing India’s workforce for an AI-driven future.
NVIDIA is partnering with India’s IT giants such as Infosys, TCS, Tech Mahindra and Wipro to upskill nearly half a million developers, ensuring India leads the AI revolution with a highly trained workforce.
“India’s technical talent is unmatched,” Huang said.
Ambani echoed these sentiments, stressing that “India will be one of the biggest intelligence markets,” pointing to the nation’s youthful, technically talented population.
A Vision for India’s AI-Driven Future
As the session drew to a close, Huang and Ambani reflected on their vision for India’s AI-driven future.
With its vast talent pool, burgeoning tech ecosystem and immense data resources, the country, they agreed, has the potential to contribute globally in sectors such as energy, healthcare, finance and manufacturing.
“This cannot be done by any one company, any one individual, but we all have to work together to bring this intelligence age safely to the world so that we can create a more equal world, a more prosperous world,” Ambani said.
Huang echoed the sentiment, adding: “Let’s make it a promise today that we will work together so that India can take advantage of the intelligence revolution that’s ahead of us.”