This post is co-written with Etzik Bega from Agmatix. Agmatix is an Agtech company pioneering data-driven solutions for the agriculture industry that harnesses advanced AI technologies, including generative AI, to expedite R&D processes, enhance crop yields, and advance sustainable agriculture. Focused on addressing the challenge of agricultural data standardization, Agmatix has developed proprietary patented technology to harmonize and standardize data, facilitating informed decision-making in agriculture. Its suite of data-driven tools enables the management of agronomic field trials, the creation of digital crop nutrient prescriptions, and the promotion of sustainable agricultural practices. Widely embraced by agronomists, scientists, and R&D teams in crop input manufacturing and contract-based research organizations, Agmatix’s field trial and analysis solutions are at the forefront of agricultural innovation.
This post describes how Agmatix uses Amazon Bedrock and AWS fully featured services to enhance the research process and development of higher-yielding seeds and sustainable molecules for global agriculture.
Amazon Bedrock is a fully managed service that offers a choice of high-performing foundation models (FMs) from leading AI companies like AI21 Labs, Anthropic, Cohere, Meta, Mistral AI, Stability AI, and Amazon through a single API, along with a broad set of capabilities to build generative AI applications with security, privacy, and responsible AI. With Amazon Bedrock, you can experiment with and evaluate top FMs for your use case, privately customize them with your data using techniques such as fine-tuning and Retrieval Augmented Generation (RAG), and build agents that run tasks using your enterprise systems and data sources.
Through this innovative approach, Agmatix streamlines operations, accelerates the introduction of higher-yielding seeds, and fosters the development of new and sustainable molecules used in crop protection, including pesticides, herbicides, fungicides, and biologicals.
Innovation in field trial R&D is complex
Innovation continues to be a major driver for increasing yields and the security of our global food supply. Discoveries and improvements across seed genetics, site-specific fertilizers, and molecule development for crop protection products have coincided with innovations in generative AI, Internet of Things (IoT) and integrated research and development trial data, and high-performance computing analytical services.
Holistically, these systems have enabled dramatic reductions in time to market for new genetics and molecules, enabling growers with new and more effective products. Historical and current R&D on crop varieties and agricultural chemicals is essential to improving agricultural yields, but the process of bringing a new crop input to farms is expensive and complex. A key stage in this process is field trials. After new inputs are developed in labs, field trials are conducted to test the effectiveness of new crop varieties and agricultural chemicals in real-world conditions.
There are various technologies that help operationalize and optimize the process of field trials, including data management and analytics, IoT, remote sensing, robotics, machine learning (ML), and now generative AI.
Led by agricultural technology innovators, generative AI is the latest AI technology that helps agronomists and researchers have open-ended human-like interactions with computing applications to assist with a variety of tasks and automate historically manual processes. Applications of generative AI in agriculture include yield prediction, improving precision agriculture recommendations, educating and training agronomy staff, and enabling users to query vast datasets using natural language.
Current challenges in analyzing field trial data
Agronomic field trials are complex and create vast amounts of data. Most companies are unable to use their field trial data based on manual processes and disparate systems. Agmatix’s trial management and agronomic data analysis infrastructure can collect, manage, and analyze agricultural field trials data. Agronomists use this service to accelerate innovation and turn research and experimentation data into meaningful, actionable intelligence.
Agronomists upload or enter field trial data, create and manage tasks for monitoring field trials, and analyze and visualize trial data to generate insights. The time-consuming, undifferentiated task of cleaning, standardizing, harmonizing, and processing the data is automated and handled by Agmatix’s intelligent service.
Without the use of generative AI, the ability to build an analytical dashboard to analyze trial data and gain meaningful insights from field trials is complex and time-consuming. The following are two common challenges:
- Each trial may contain hundreds of different parameters, and it’s challenging for an agronomist to understand which parameters and data points are meaningful to the specific problems they want to investigate.
- There is a wide range of analytical visualization tools and charts (such as ANOVA One-Way, Regression, Boxplots, and Maps) available to choose from. However, selecting the most appropriate visualization technique that facilitates understanding of patterns and identification of anomalies within the data can be a challenging task.
Moreover, after the analytical dashboard is created, it can be complex to draw conclusions and establish connections between the different data points. For example, do the results of the trial support the hypothesis of the trial? Is there a connection between the fertilizer applied and the weight of the grain produced? Which external factors have the biggest impact on the efficacy of the product trial?
AWS generative AI services provide a solution
In addition to other AWS services, Agmatix uses Amazon Bedrock to solve these challenges. Amazon Bedrock is a fully managed, serverless generative AI offering from AWS that provides a range of high-performance FMs to support generative AI use cases.
Through the integration of Agmatix’s landscape with Amazon Bedrock, Agmatix has developed a specialized generative AI assistant called Leafy, which gives agronomists and R&D staff a significantly improved user experience.
Instead of spending hours evaluating data points for investigation, selecting the right visualization tools, and creating multiple dashboards for analyzing R&D and trial information, agronomists can write their questions in natural language and get Leafy to provide the relevant dashboards and insights immediately (see the following screenshot for an example of Leafy in action). This helps improve productivity and user experience.
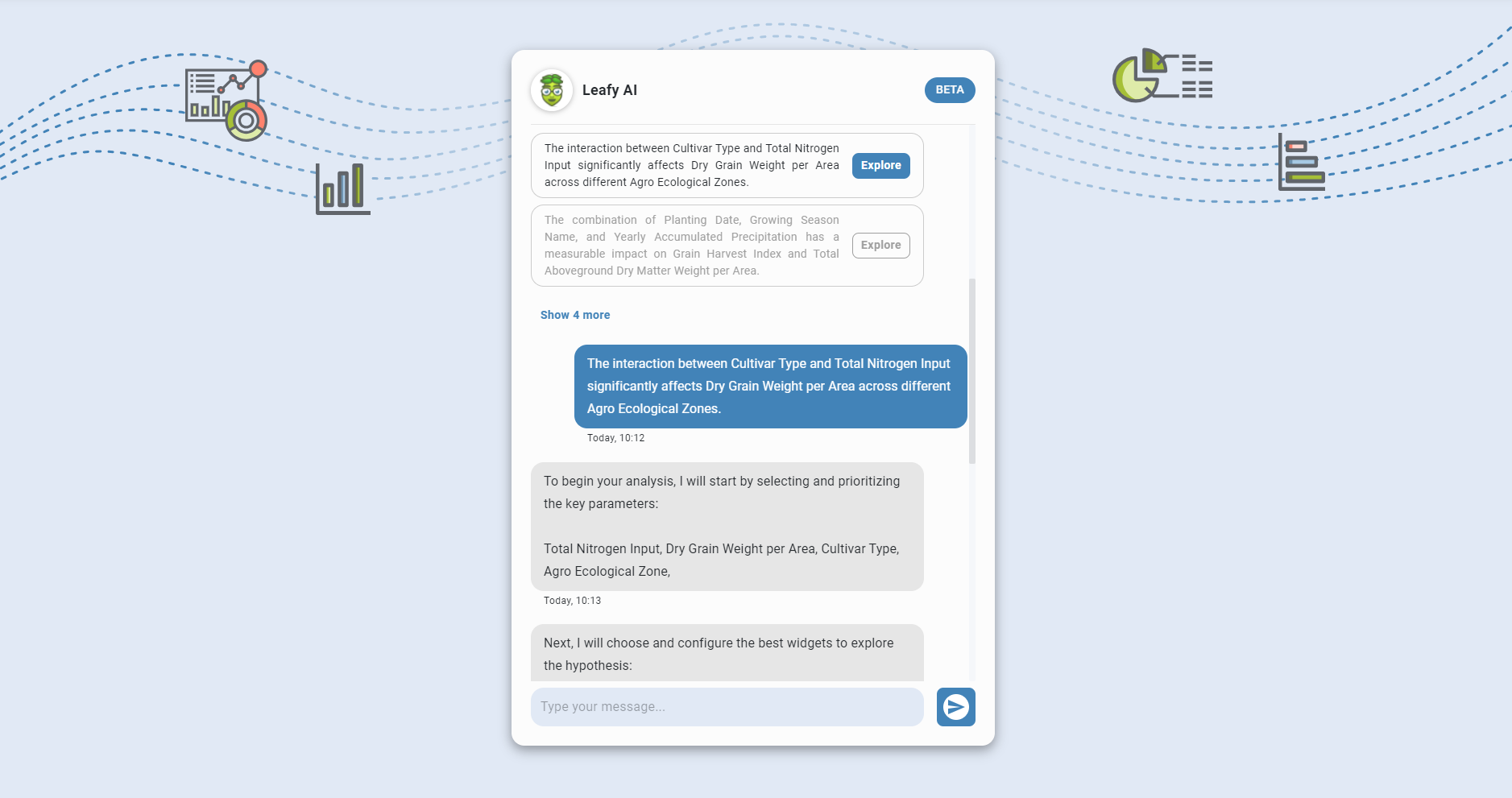
The first step in developing and deploying generative AI use cases is having a well-defined data strategy. Agmatix’s technology architecture is built on AWS. Their data pipeline (as shown in the following architecture diagram) consists of ingestion, storage, ETL (extract, transform, and load), and a data governance layer. Multi-source data is initially received and stored in an Amazon Simple Storage Service (Amazon S3) data lake. AWS Glue accesses data from Amazon S3 to perform data quality checks and important transformations. AWS Lambda is then used to further enrich the data. The transformed data acts as the input to AI/ML services. The generated insights are accessed by users through Agmatix’s interface.
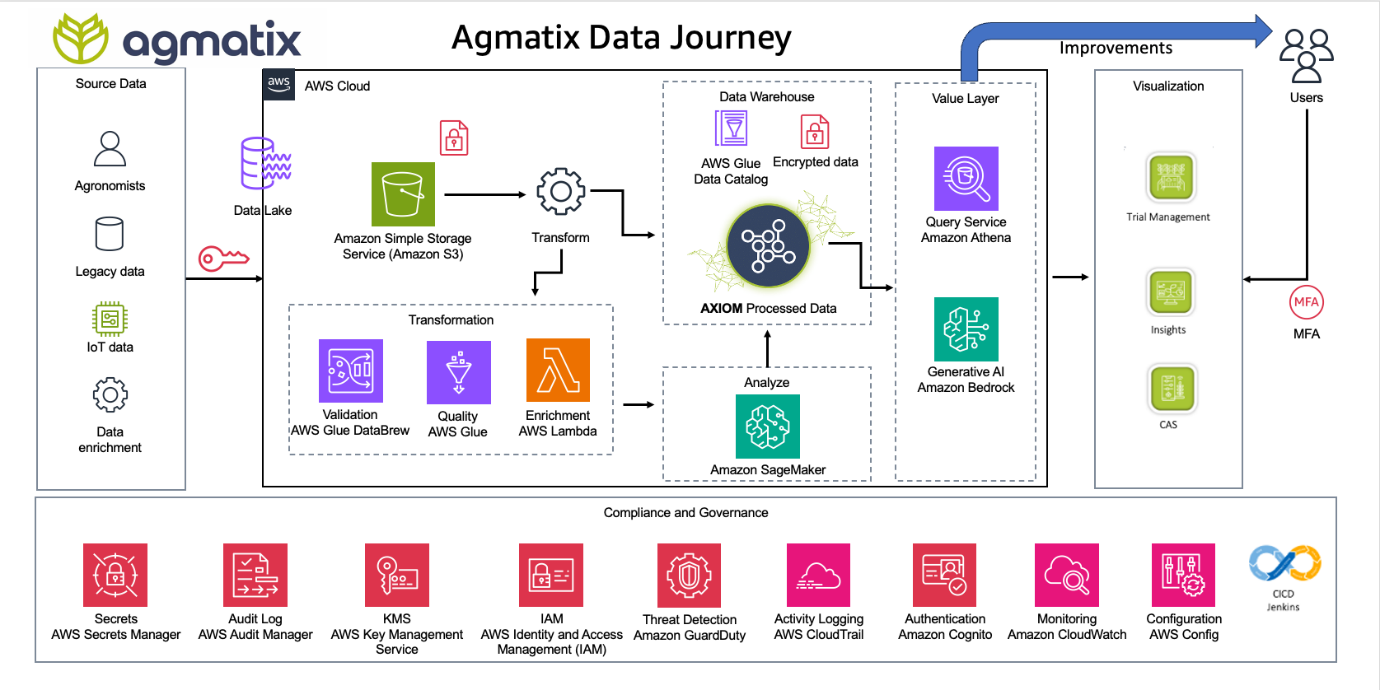
Focusing on generative AI, let’s first understand the fundamentals of the generative AI chatbot application:
- Prompt – The input question or task including contextual information provided by the user
- Data – The data required to answer the question in the prompt
- Agent – The agent that performs the orchestration of tasks
In the case of Agmatix, when the agronomist asks Leafy a question, Agmatix’s Insights solution sends a request to Anthropic Claude on Amazon Bedrock through an API:
- Prompt – The prompt sent to Anthropic Claude consists of tasks and data. The task is the question submitted by the user.
- Data – The data in the prompt includes two types of data:
- Context data instructions to the model; for example, a list of the types of widgets available for visualization.
- The data from the specific field trial.
The following diagram illustrates the generative AI workflow.
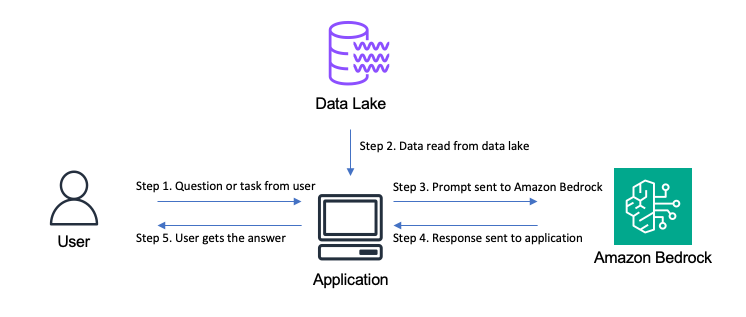
The workflow consists of the following steps:
- The user submits the question to Agmatix’s AI assistant, Leafy.
- The application reads the field trial data, business rules, and other required data from the data lake.
- The agent inside the Insights application collects questions and tasks and the relevant data, and sends it as a prompt to the FM through Amazon Bedrock.
- The generative AI model’s response is sent back to the Insights application.
- The response is displayed to the user through the widgets visualizing the trial data and the answer to the user’s specific question, as shown in the following screenshot.
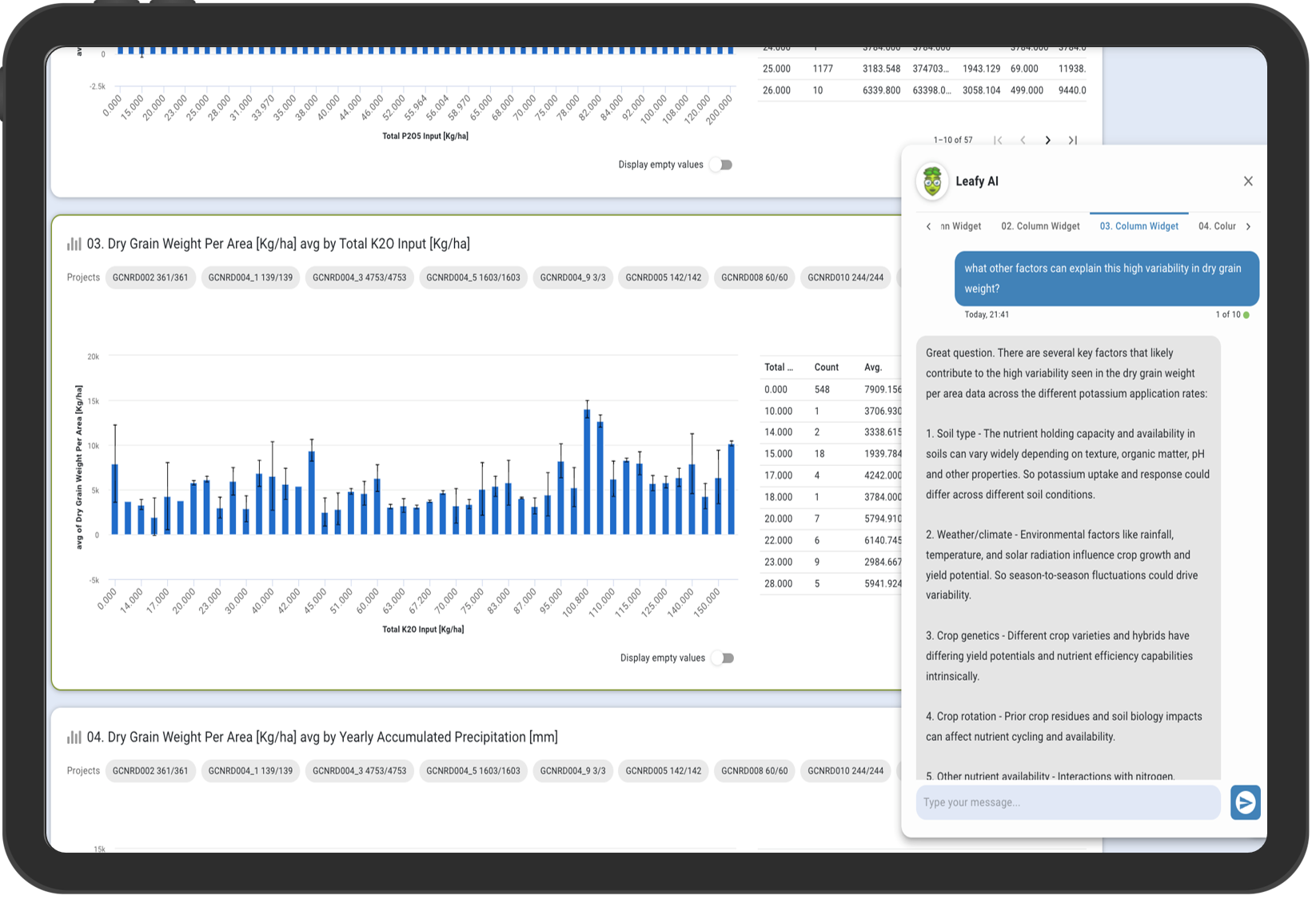
The data used in the prompt engineering (trial result and rules) is stored in plain text and sent to the model as is. Prompt engineering plays a central part in this generative AI solution. For more information, refer to the Anthropic Claude prompt engineering guide.
Overall, by using Amazon Bedrock on AWS, Agmatix’s data-driven field trials service observed over 20% improved efficiency, more than 25% improvement in data integrity, and a three-fold increase in analysis potential throughput.
This is how generative AI technology is helping improve the overall experience and productivity of agronomists so they can focus on solving complex challenges and tasks that require human knowledge and intervention.
A real-life example of this solution can be seen within the largest open nutrient database for crop nutrition, powered by the Agmatix infrastructure, where researchers can tap into insights gleaned from thousands of field trials. In this practical scenario, users benefit from guided question prompts and responses facilitated by generative AI. This advanced data processing enhances users’ grasp of evolving trends in crop nutrient uptake and removal, simplifying the creation of decision support systems.
Conclusion
Seed, chemical, and fertilizer manufacturers need innovative, smart agricultural solutions to advance the next generation of genetics and molecules. Ron Baruchi, President and CEO of Agmatix, highlights the beneficial synergy between humans and technology:
“AI complements, rather than replaces, human expertise. By integrating Amazon Bedrock’s generative AI into our infrastructure, we provide our customers with self-service analytical tools that simplify complex and time-consuming tasks.”
This integration equips agronomists and researchers with advanced AI capabilities for data processing and analysis, enabling them to concentrate on strategic decision-making and creative problem-solving.
Field trial management has long needed a fresh dose of technology infusion. With Agmatix’s AI-enabled agriculture service, powered by AWS, input manufacturers can reduce the time and cost associated with field trials, while improving the overall productivity and experience of agronomists and growers. By delivering growers the most successful seeds, crop protection products, and fertilizers, their farming operations can thrive. This approach not only maximizes the efficiency of these essential crop inputs but also minimizes natural resource usage, resulting in a more sustainable and healthier planet for all.
Contact us to learn more about Agmatix.
Resources
Check out the following resources to learn more about AWS and Amazon Bedrock:
About the Authors
Etzik Bega is the Chief Architect of Agmatix, where he has revolutionized the company’s data lake architecture using cutting-edge GenAI technology. With over 25 years of experience in cybersecurity, system architecture, and communications, Etzik has recently focused on helping organizations move to the public cloud securely and efficiently.
Menachem Melamed is a Senior Solutions Architect at AWS, specializing in Big Data analytics and AI. With a deep background in software development and cloud architecture, he empowers organizations to build innovative solutions using modern cloud technologies.
Prerana Sharma is Manager of Solutions Architects at AWS, specializing in Manufacturing. With a wide experience of working in the Digital Farming space, Prerana helps customers solve business problems by experimenting and innovating with emerging technologies on AWS.
Read More