We recently announced the general availability of cross-account sharing of Amazon SageMaker Model Registry using AWS Resource Access Manager (AWS RAM), making it easier to securely share and discover machine learning (ML) models across your AWS accounts.
Customers find it challenging to share and access ML models across AWS accounts because they have to set up complex AWS Identity and Access Management (IAM) policies and create custom integrations. With this launch, customers can now seamlessly share and access ML models registered in SageMaker Model Registry between different AWS accounts.
Customers can use the SageMaker Studio UI or APIs to specify the SageMaker Model Registry model to be shared and grant access to specific AWS accounts or to everyone in the organization. Authorized users can then quickly discover and use those shared models in their own AWS accounts. This streamlines the ML workflows, enables better visibility and governance, and accelerates the adoption of ML models across the organization.
In this post, we will show you how to use this new cross-account model sharing feature to build your own centralized model governance capability, which is often needed for centralized model approval, deployment, auditing, and monitoring workflows. Before we dive into the details of the architecture for sharing models, let’s review what use case and model governance is and why it’s needed.
Use case governance is essential to help ensure that AI systems are developed and used in ways that respect values, rights, and regulations. According to the EU AI Act, use case governance refers to the process of overseeing and managing the development, deployment, and use of AI systems in specific contexts or applications. This includes:
- Risk assessment: Identifying and evaluating potential risks associated with AI systems.
- Mitigation strategies: Implementing measures to minimize or eliminate risks.
- Transparency and explainability: Making sure that AI systems are transparent, explainable, and accountable.
- Human oversight: Including human involvement in AI decision-making processes.
- Monitoring and evaluation: Continuously monitoring and evaluating AI systems to help ensure compliance with regulations and ethical standards.
Model governance involves overseeing the development, deployment, and maintenance of ML models to help ensure that they meet business objectives and are accurate, fair, and compliant with regulations. It includes processes for monitoring model performance, managing risks, ensuring data quality, and maintaining transparency and accountability throughout the model’s lifecycle. In AWS, these model lifecycle activities can be performed over multiple AWS accounts (for example, development, test, and production accounts) at the use case or business unit level. However, model governance functions in an organization are centralized and to perform those functions, teams need access to metadata about model lifecycle activities across those accounts for validation, approval, auditing, and monitoring to manage risk and compliance.
Use case and model governance plays a crucial role in implementing responsible AI and helps with the reliability, fairness, compliance, and risk management of ML models across use cases in the organization. It helps prevent biases, manage risks, protect against misuse, and maintain transparency. By establishing robust oversight, organizations can build trust, meet regulatory requirements, and help ensure ethical use of AI technologies.
Use case and model lifecycle governance overview
In the context of regulations such as the European Union’s Artificial Intelligence Act (EU AI Act), a use case refers to a specific application or scenario where AI is used to achieve a particular goal or solve a problem. The EU AI Act proposes to regulate AI systems based on their intended use cases, which are categorized into four levels of risk:
- Unacceptable risk: Significant threat to safety, livelihoods, or rights
- High risk: Significant impacts on lives (for example, use of AI in healthcare and transportation)
- Limited risk: Minimal impacts (for example, chatbots and virtual assistants)
- Minimal risk: Negligible risks (for example, entertainment and gaming)
An AI system is built to satisfy a use case such as credit risk, which can be comprised of workflows orchestrated with one or more ML models—such as credit risk and fraud detection models. You can build a use case (or AI system) using existing models, newly built models, or combination of both. Regardless of how the AI system is built, governance will be applied at the AI system level where use case decisions (for example, denying a loan application) are being made. However, explaining why that decision was made requires next-level detailed reports from each affected model component of that AI system. Therefore, governance applies both at the use case and model level and is driven by each of their lifecycle stages.
Use case lifecycle stages
A use case has its own set of lifecycle stages from development through deployment to production, shown in the following figure. A use case typically starts with an experimentation or proof-of-concept (POC) stage where the idea is explored for feasibility. When the use case is determined to be feasible, it’s approved and moves to the next stage for development. The use case is then developed using various components including ML models and unit testing, and then moved to the next stage—quality assurance (QA)—after approval. Next, the use case is tested, validated, and approved to be moved to the pre-production stage where it’s A/B tested with production-like settings and approved for the next stage. Now, the use case is deployed and operational in production. When the use case is no longer needed for business, it’s retired and decommissioned. Even though these stages are depicted as linear in the diagram, they are frequently iterative.
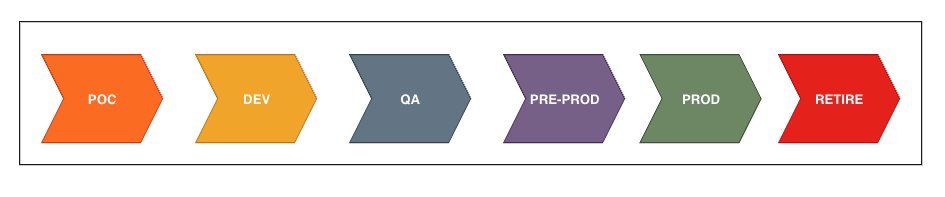
Model lifecycle stages
When an ML model is developed it goes through a similar set of lifecycle stages as a use case. In the case of an ML model, shown in the following figure, the lifecycle starts with the development or candidate model. Prior to that stage, there would be several experiments performed to build the candidate model. From a governance perspective, tracking starts from the candidate or dev model stage. After approval in dev, the model moves into the QA stage where it’s validated and integration tested to make sure that it meets the use case requirements and then is approved for promotion to the next stage. The model is then A/B tested along with the use case in pre-production with production-like data settings and approved for deployment to the next stage. The model is finally deployed to production. When the model is no longer needed, it’s retired and removed from deployed endpoints.
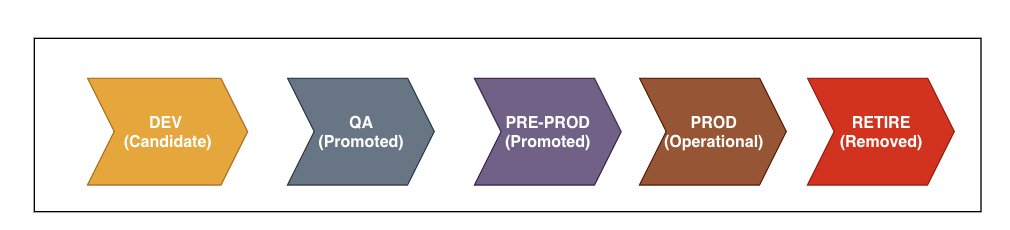
Stage status types
In the preceding use case and model stages discussion, we mentioned approving the model to go to the next stage. However, there are two other possible states—pending and rejected, as depicted in the following figure. These stages are applicable to both use case and model stages. For example, a use case that’s been moved from the QA stage to pre-production could be rejected and sent back to the development stage for rework because of missing documentation related to meeting certain regulatory controls.
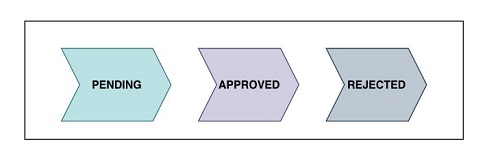
Multi-account architecture for sharing models
A multi-account strategy improves security, scalability, and reliability of your systems. It also helps achieve data, project, and team isolation while supporting software development lifecycle best practices. Cross-account model sharing supports a multi-account strategy, removing the overhead of assuming roles into multiple accounts. Furthermore, sharing model resources directly across multiple accounts helps improve ML model approval, deployment, and auditing.
The following diagram depicts an architecture for centralizing model governance using AWS RAM for sharing models using a SageMaker Model Group, a core construct within SageMaker Model Registry where you register your model version.
Figure 1: Centralizing Model Governance using AWS RAM Share
In the architecture presented in the preceding figure, the use case stakeholder, data scientist (DS) and ML engineer (MLE) perform the following steps:
- The use case stakeholder, that is the DS team lead, receives the request to build an AI use case such as credit risk from their line of business lead.
- The DS team lead records the credit risk use case in the POC stage in the stage governance table.
- The MLE is notified to set up a model group for new model development. The MLE creates the necessary infrastructure pipeline to set up a new model group.
- The MLE sets up the pipeline to share the model group with the necessary permissions (create and update the model version) to the ML project team’s development account. Optionally, this model group can also be shared with their test and production accounts if local account access to model versions is needed.
- The DS uses SageMaker Training jobs to generate metrics captured by , selects a candidate model, and registers the model version inside the shared model group in their local model registry.
- Because this is a shared model group, the actual model version will be recorded in the shared services account model registry and a link will be maintained in the development account. The Amazon S3 model artifacts associated to the model will be copied to the shared services account when the model is registered in the shared services model registry.
- The model group and associated model version will be synced into the model stage governance Amazon DynamoDB table with attributes such as model group, model version, model stage (development, test, production, and so on), model status (pending, approved, or rejected), and model metrics (in JSON format). The ML admin sets up this table with the necessary attributes based on their central governance requirements.
- The model version is approved for deployment into the test stage and is deployed into the test account along with necessary infrastructure for invoking the model, such as an Amazon API gateway and AWS Lambda
- Model is integration tested in the test environment and model test metrics are updated in the model stage governance table
- Model test results are validated, and the model version is approved for deployment into the production stage and is deployed into the production account along with the necessary infrastructure for invoking the model such as an API gateway and Lambda functions.
- The model is A/B tested or optionally shadow tested in the production environment and model production metrics are updated in the model stage governance table. When satisfactory production results are attained, the model version is rolled out in the production environment.
- The model governance (compliance) officer uses the governance dashboard to act on model governance functions such as reviewing the model to validate compliance and monitoring for risk mitigation.
Building a central model registry using model group resource sharing
Model group resource sharing makes it possible to build a central model registry with few clicks or API calls without needing to write complex IAM policies. We will demonstrate how to set up a central model registry based on the architecture we described in the previous sections. We will start by using the SageMaker Studio UI and then by using APIs. In both cases, we will demonstrate how to create a model package group in the ML Shared Services account (Account A) and share it with the ML Dev account (Account B) so that any updates to model versions in Account B automatically update the corresponding model versions in Account A.
Prerequisites
You need to have the following prerequisites in place to implement the solution in this post.
After you have the prerequisites set up, start by creating and sharing a model group across accounts. The basic steps are:
- In Account A, create a model group.
- In Account A, create a resource share for the model group, and then attach permissions and specify the target account to share the resource. Permissions can be standard or custom.
- Account B should accept the resource sharing invitation to start using the shared resource from Account A.
- Optionally, if Account A and Account B are part of the same AWS Organizations, and the resource sharing is enabled within AWS Organizations, then the resource sharing invitation are auto accepted without any manual intervention.
Create and share a model group across accounts using SageMaker Studio
The following section shows how to use SageMaker Studio to share models in a multi-account environment to build a central model registry. The following are instructions for using the AWS Management Console for SageMaker Studio to create a model package group in the shared services account, adding the necessary permissions, with the ML Dev account.
To use the console to create and share a model package:
- In the SageMaker Studio console, sign in to Account A and navigate to the model registry, select the model package group (in this example, the
credit-risk-package-group-1724904598
), and choose Share.
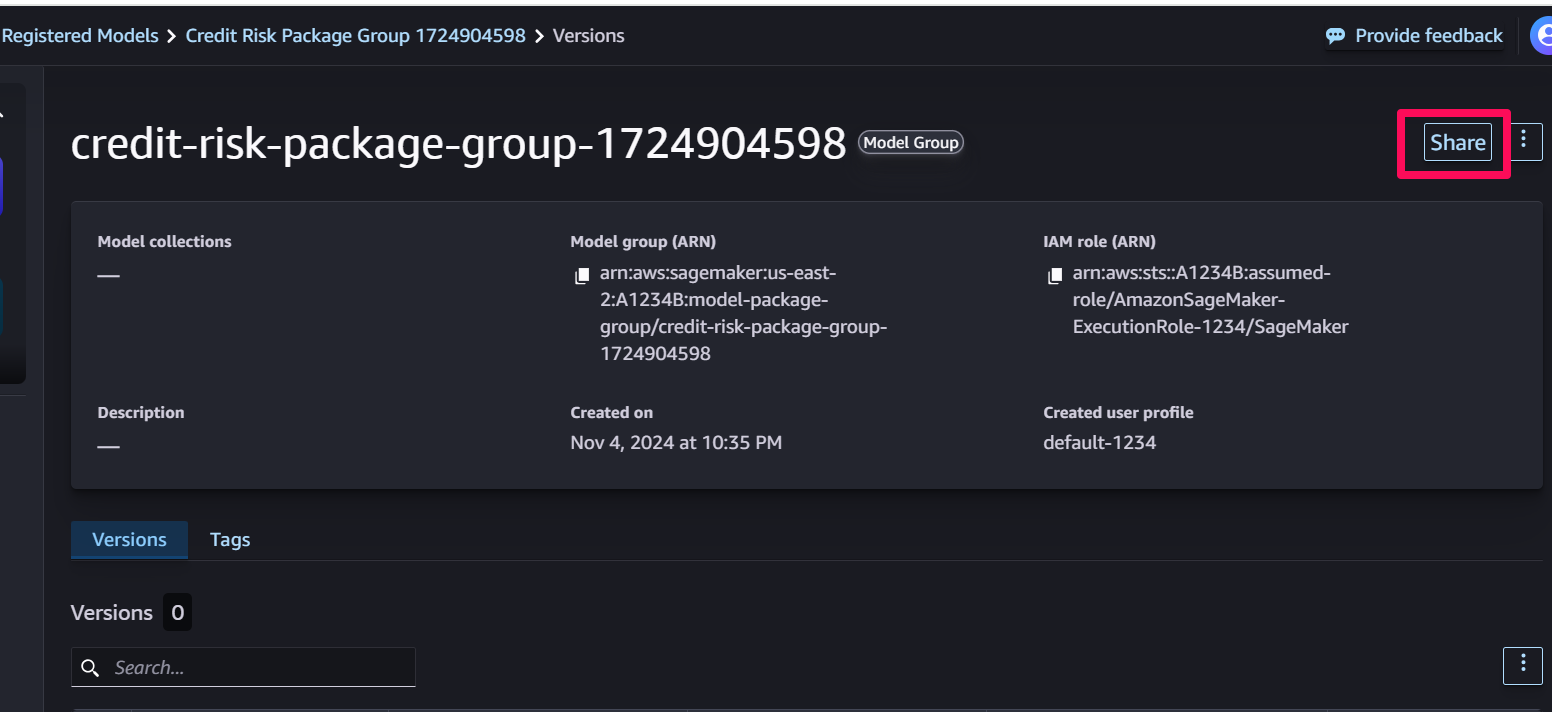
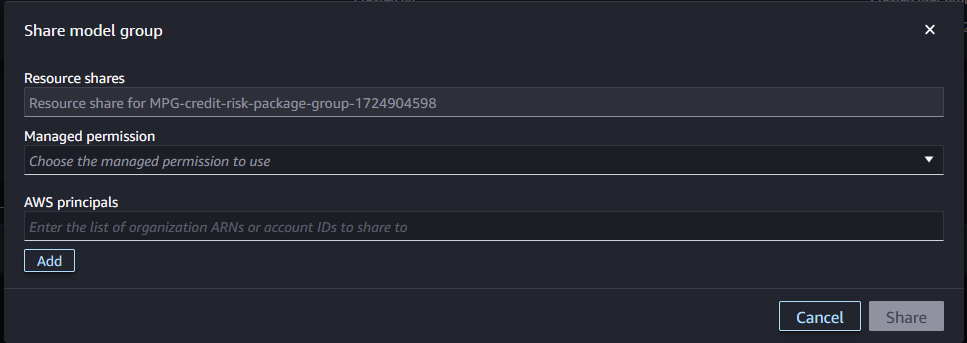
- In Account A, select the appropriate permissions to share the model package group with Account B. If you need to allow custom policy, navigate to the AWS RAM console and create the policy.
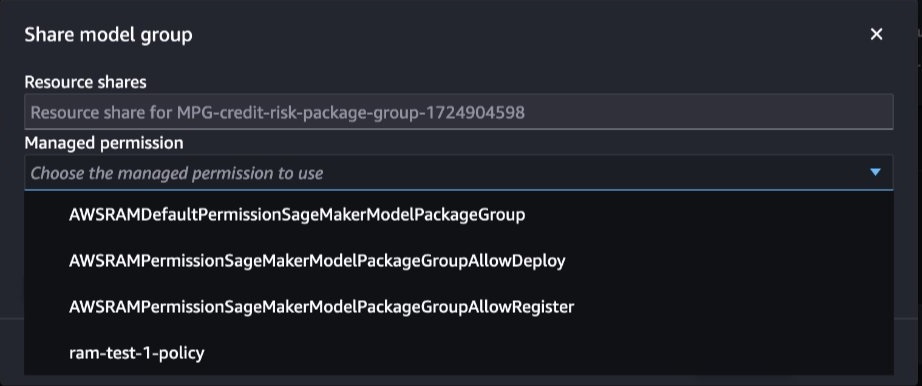
- After selecting the permission policy, specify Account B (and any other accounts) to share the resource, then choose Share.
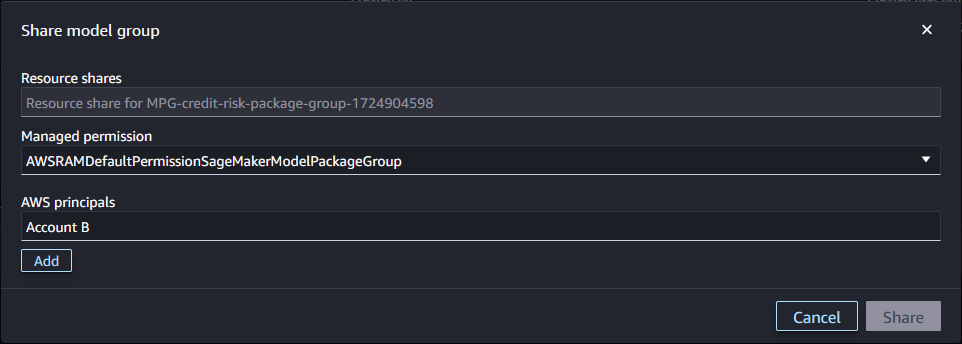
- In Account B, navigate to the model registry, choose Shared with me, and then choose View pending approvals to see the model shared from Account A.
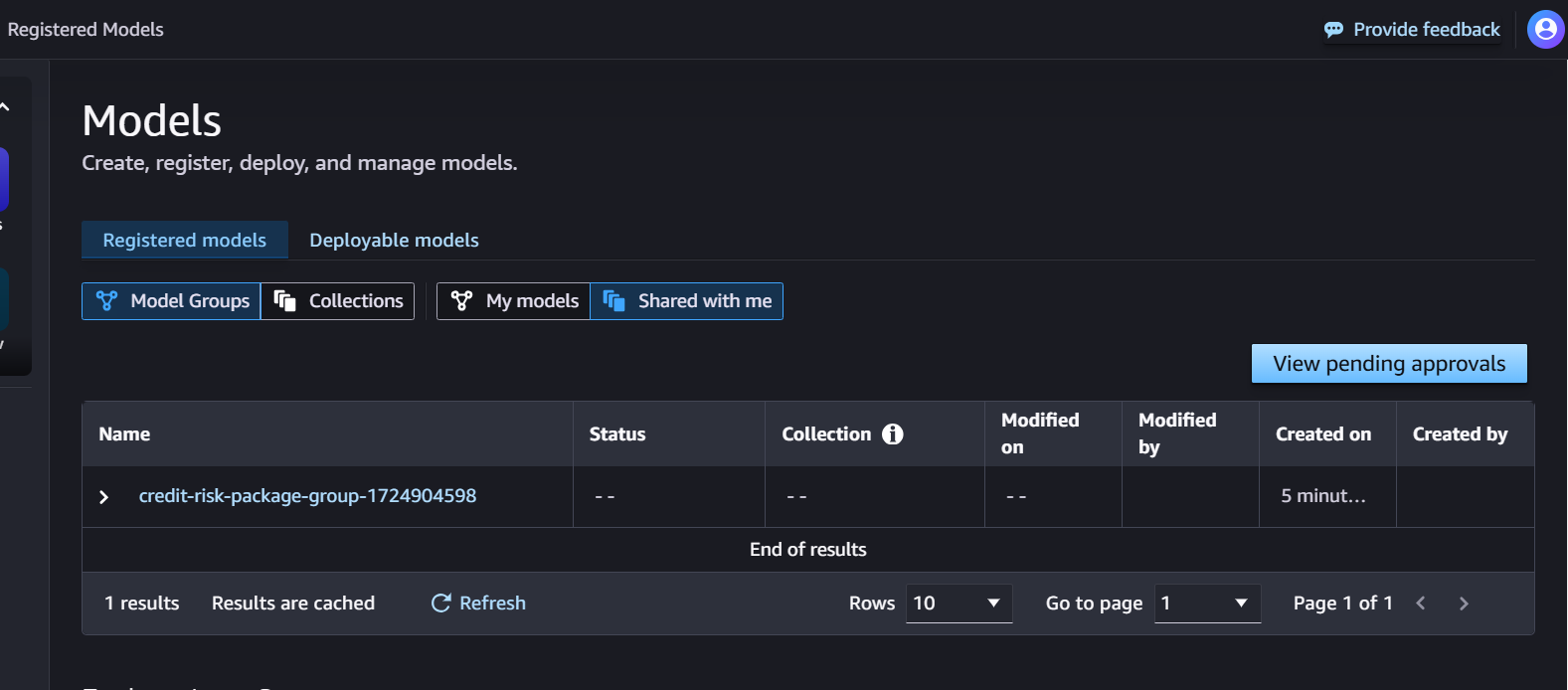
- Accept the model invitation from Account A to access the shared model package group and its versions. When accounts are set up in the same organization, invitations will be accepted without requiring user intervention.
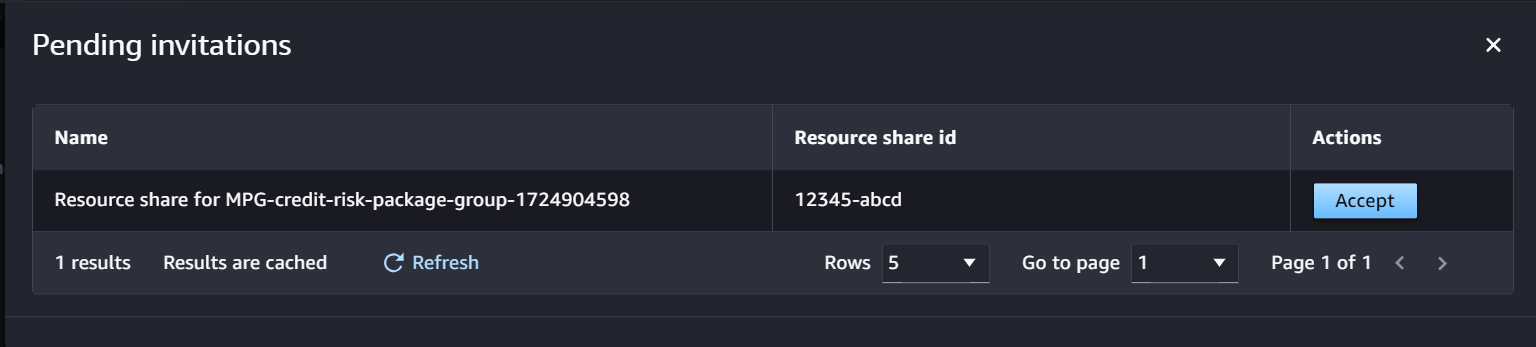
Create and share the model group across accounts using APIs
The following section shows how to use APIs to share models in a multi-account environment to build a central model registry. Create a model package group in the ML Shared Services account (Account A) and share it with the ML Dev account (Account B).
Following are the steps completed by using APIs to create and share a model package group across accounts.
- In Account A, create a model package group.
- In Account A, if needed, create custom sharing permissions; otherwise use standard sharing permissions.
- In Account A, create a resource share for the model package group, attach permissions, and specify the target account to share the resource.
- In Account B, accept the resource sharing invitation to start using the resource.
- If Account A and B are part of the same organization, then the resource sharing invitation can be accepted without any manual intervention.
Run the following code in the ML Shared Services account (Account A).
import json
import time
import os
import boto3
region = boto3.Session().region_name
sm_client = boto3.client('sagemaker', region_name=region)
# Replace model package group name as per use case
model_package_group_name = "model-group-" + str(round(time.time()))
model_package_group_input_dict = {
"ModelPackageGroupName" : model_package_group_name,
"ModelPackageGroupDescription" : "Sample model package group"
}
# Create Model package group with Sagemaker client
create_model_package_group_response = sm_client.create_model_package_group(**model_package_group_input_dict)
model_package_group_arn = create_model_package_group_response['ModelPackageGroupArn']
print('ModelPackageGroup Arn : {}'.format(model_package_group_arn))
ram_client = boto3.client('ram')
# # Use this code path to create custom permission
# # Custom permission template resource policy string
# policy_template = '{nt"Effect": "Allow",nt"Action": [ntt"sagemaker:DescribeModelPackageGroup"nt]n}'
# permission = ram_client.create_permission(
# name = "custom-permission" + str(round(time.time())),
# resourceType = "sagemaker:ModelPackageGroup",
# policyTemplate = policy_template
# )
# print('Created Permission: {}'.format(permission['permission']['arn']))
# permission = permission['permission']['arn']
# Use this code path to use managed Permission
# It can be one of:
# 1. arn:aws:ram::aws:permission/AWSRAMDefaultPermissionSageMakerModelPackageGroup
# 2. arn:aws:ram::aws:permission/AWSRAMPermissionSageMakerModelPackageGroupAllowDeploy
# 3. arn:aws:ram::aws:permission/AWSRAMPermissionSageMakerModelPackageGroupAllowRegister
# More details :
permission = 'arn:aws:ram::aws:permission/AWSRAMDefaultPermissionSageMakerModelPackageGroup'
# Principals can be IAM User, Role, Account or Organization ID. Ref: https://boto3.amazonaws.com/v1/documentation/api/latest/reference/services/ram/client/create_resource_share.html
response = ram_client.create_resource_share(
name="model-group-resource-share",
resourceArns=[create_model_package_group_response['ModelPackageGroupArn']],
principals=['12345'],
permissionArns = [permission]
)
resource_share_arn = response['resourceShare']['resourceShareArn']
print('Resource Share Arn : {}'.format(resource_share_arn))
Run the following code in the ML Dev account (Account B).
import json
import os
import boto3
from time import gmtime, strftime
region = boto3.Session().region_name
ram_client = boto3.client('ram')
response = ram_client.get_resource_share_invitations()
pending_invitations = []
# Review all pending invitations
for i in response['resourceShareInvitations']:
if i['status'] == "PENDING":
pending_invitations.append(i)
print(pending_invitations,sep='n')
# Accept the resource share invitation from central account
# Replace with the intended invitation arn for acceptance from the central account
if pending_invitations:
response = ram_client.accept_resource_share_invitation(resourceShareInvitationArn=pending_invitations[0]['resourceShareInvitationArn'])
print(response)
sm_client = boto3.client('sagemaker', region_name=region)
response = sm_client.list_model_package_groups(CrossAccountFilterOption="CrossAccount")
MLflow experimentation with the shared model group
The following section shows you how to use Amazon SageMaker with MLflow to track your experiments in the development account and save candidate models in the shared model group while developing a credit risk model. It’s a binary classification problem where the goal is to predict whether a customer is a credit risk. If you want to run the code in your own environment, check out the notebook in this GitHub repository.
SageMaker with MLflow is a capability of SageMaker that you can use to create, manage, analyze, and compare your ML experiments. To get started with MLflow, you need to set up an MLflow tracking server to monitor your experiments and runs. You can set up the server programmatically or by using the SageMaker Studio UI. It can take up to 20 minutes for the setup to complete. The following code snippet shows how to create a tracking server.
sagemaker_client = boto3.client("sagemaker")
timestamp = strftime('%d-%H-%M-%S', gmtime())
server_name = f"mlflow-{domain_id}-{timestamp}"
response = sagemaker_client.create_mlflow_tracking_server(
TrackingServerName=server_name,
ArtifactStoreUri=f"s3://{bucket_name}/mlflow/{timestamp}",
RoleArn=sm_role,
AutomaticModelRegistration=True,
)
mlflow_arn = response['TrackingServerArn']
To set up an MLflow tracking server in SageMaker Studio, choose the MLflow application icon. When your server is running, click on the ellipses button and then click on Open MLflow button to open the MLflow UI.
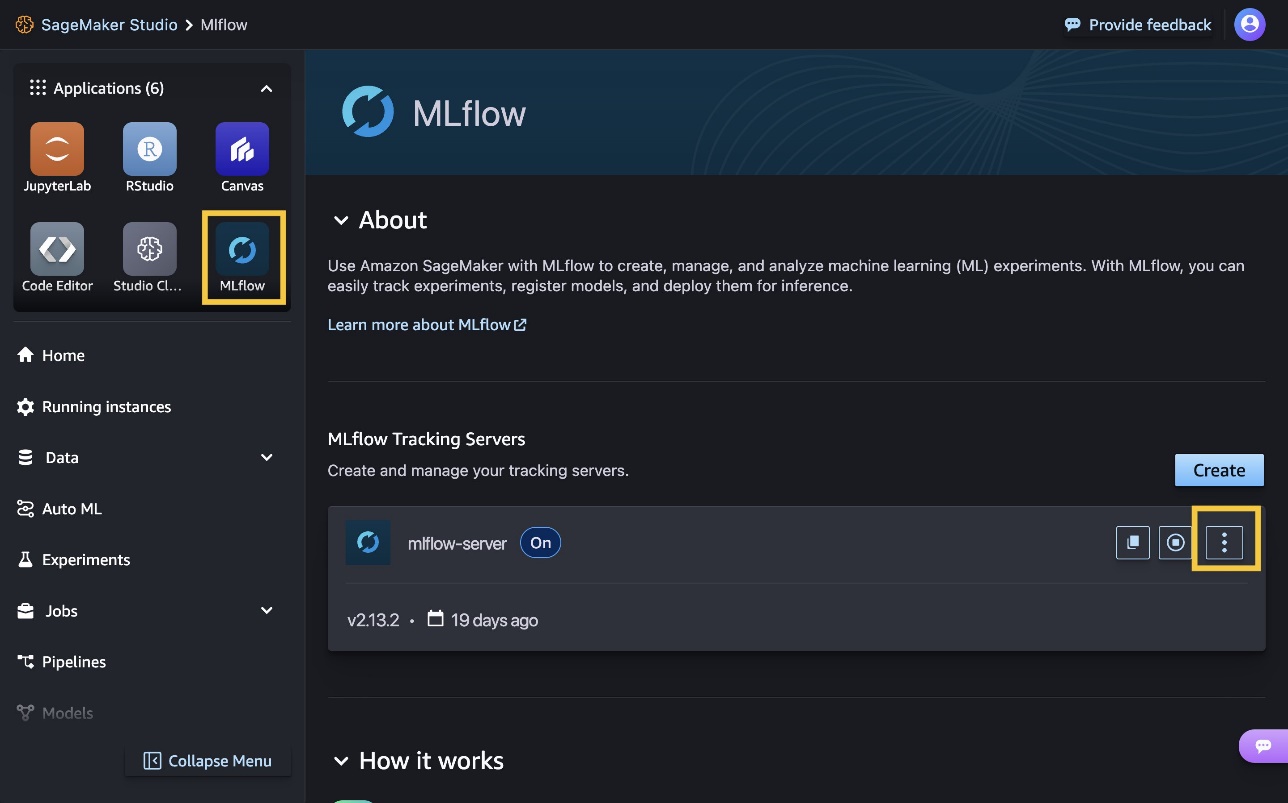
Now that your MLflow tracking server is running, you can start tracking your experiments. MLflow tracking allows you to programmatically track the inputs, parameters, configurations, and models of your iterations as experiments
and runs
.
- Runs are executions of some piece of data science code and record metadata and generated artifacts.
- An experiment collects multiple runs with the same objective.
The following code shows you how to set up an experiment and track your executions while developing the credit risk model.
Data preparation
For this example, you will use the South German Credit dataset open source dataset. To use the dataset to train the model, you need to first do some pre-processing, You can run the pre-processing code in your JupyterLab application or on a SageMaker ephemeral cluster as a SageMaker Training job using the @remote
decorator. In both cases, you can track your experiments using MLflow.
The following code demonstrates how to track your experiments when executing your code on a SageMaker ephemeral cluster using the @remote
decorator. To get started, set-up a name for your experiment.
from time import gmtime, strftime
experiment_suffix = strftime('%d-%H-%M-%S', gmtime())
experiment_name = f"credit-risk-model-experiment-{experiment_suffix}"
The processing script creates a new MLflow active experiment by calling the mlflow.set_experiment()
method with the experiment name above. After that, it invokes mlflow.start_run()
to launch an MLflow run
under that experiment.
@remote(s3_root_uri=f"s3://{bucket_name}/{prefix}", dependencies=f"requirements.txt", instance_type="ml.m5.large")
def preprocess(df, experiment_name, mlflow_arn, bucket_name, prefix, run_id=None):
try:
suffix = strftime('%d-%H-%M-%S', gmtime())
mlflow.set_tracking_uri(mlflow_arn)
mlflow.set_experiment(experiment_name=experiment_name if experiment_name else f"credit-risk-model-experiment-{suffix}")
run = mlflow.start_run(run_id=run_id) if run_id else mlflow.start_run(run_name=f"remote-processing-{suffix}", nested=True)
.....
except Exception as e:
print(f"Exception in processing script: {e}")
raise e
finally:
mlflow.end_run()
You can also log the input dataset and the sklearn model used to fit the training set during pre-processing as part of the same script.
model_dataset = mlflow.data.from_pandas(df)
mlflow.log_input(model_dataset, context="model_dataset")
.....
featurizer_model = transformer.fit(X)
features = featurizer_model.transform(X)
labels = LabelEncoder().fit_transform(y)
.....
mlflow.sklearn.log_model(
sk_model=featurizer_model,
artifact_path=f"processing/model",
registered_model_name="sk-learn-model",
)
In the MLflow UI, use the Experiments to locate your experiment. Its name should start with “credit-risk-model-experiment”.
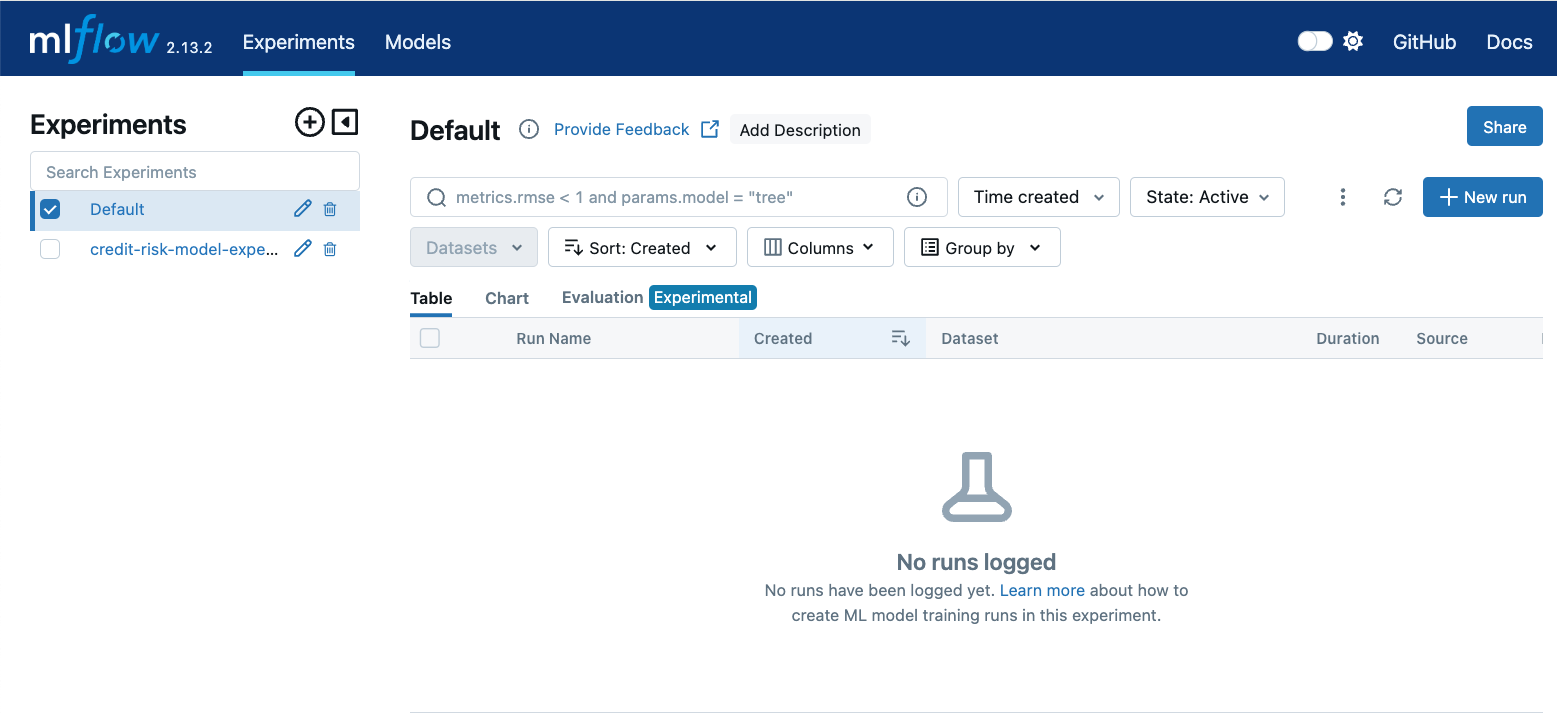
Click on the experiment name to reveal the table with the associated Runs and then click on the Run whose name starts with “remote-processing”. You will see its details as sin the following figure.
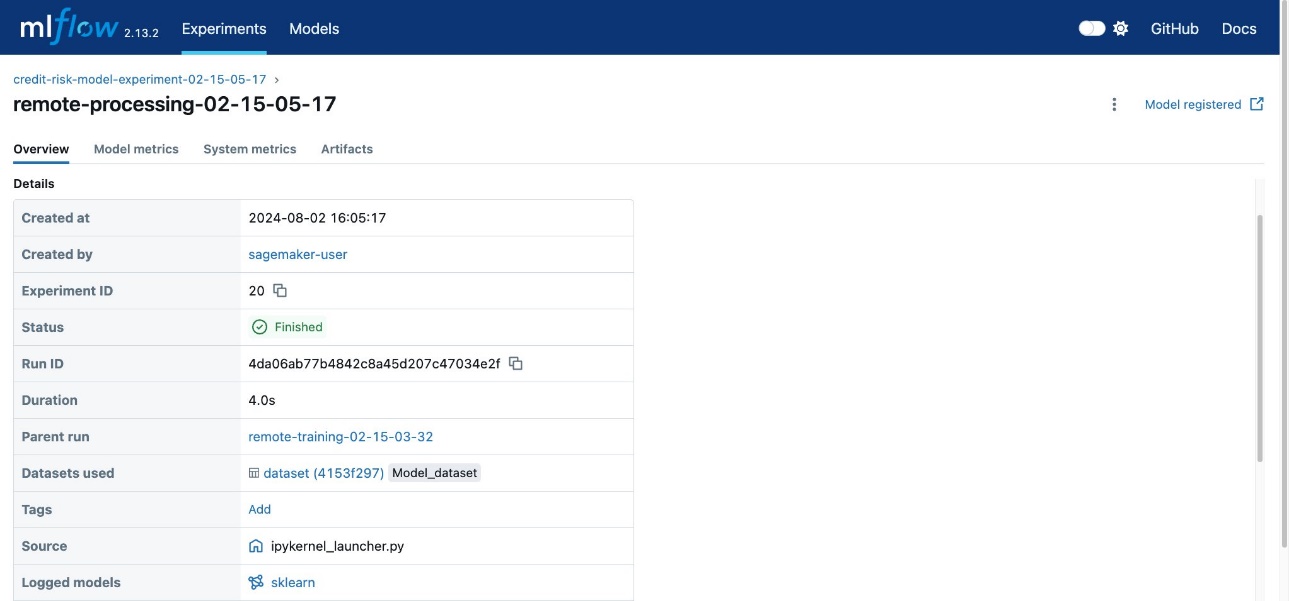
Click on the Artifacts tab to see the MLFlow model generated.
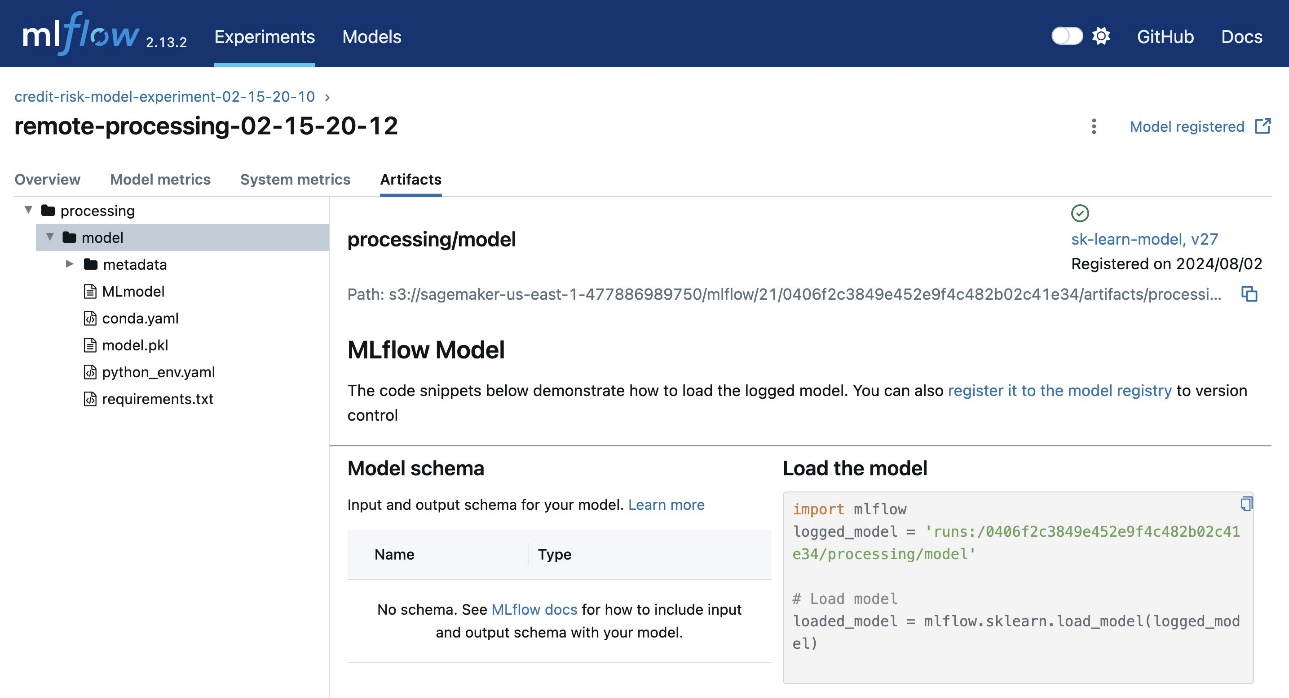
Model training
You can continue experimenting with different feature engineering techniques in your JupyterLab environment and track your experiments in MLflow. After you have completed the data preparation step, it’s time to train the classification model. You can use the xgboost algorithm for this purpose and run your code either in your JupyterLab environment or as a SageMaker Training job. Again, you can track your experiments using MLflow in both cases. The following example shows how to use MLflow with a SageMaker Training job in your code. You can use the method mlflow.autolog()
to log metrics, parameters, and models without the need for explicit log statements.
import xgboost
import pickle as pkl
import os
import mlflow
import tarfile
@remote(s3_root_uri=f"s3://{bucket_name}/{prefix}", dependencies=f"requirements.txt", instance_type="ml.m5.large")
def train(X, val_X, y, val_y, num_round, params, mlflow_arn, experiment_name,run_id=None):
output_path = "/opt/ml/model"
mlflow.set_tracking_uri(mlflow_arn)
mlflow.autolog()
suffix = strftime('%d-%H-%M-%S', gmtime())
mlflow.set_experiment(experiment_name=experiment_name if experiment_name else f"credit-risk-model-experiment-{suffix}")
run = mlflow.start_run(run_id=run_id) if run_id else mlflow.start_run(run_name=f"remote-training-{suffix}", nested=True)
try:
os.makedirs(output_path, exist_ok=True)
print(f"Directory '{output_path}' created successfully.")
except OSError as e:
print(f"Error creating directory '{output_path}': {e}")
dtrain = xgboost.DMatrix(X, label=y)
dval = xgboost.DMatrix(val_X, label=val_y)
dtrain = xgboost.DMatrix(X, label=y)
dval = xgboost.DMatrix(val_X, label=val_y)
watchlist = [(dtrain, "train"), (dval, "validation")]
mlflow.log_params(params)
print("Training the model")
evaluation__results = {}
bst = xgboost.train(
params=params, dtrain=dtrain, evals=watchlist, num_boost_round=num_round
)
pkl.dump(bst, open(output_path + "/model.bin", "wb"))
# Compress the model.bin artifact to a tar file
tar_filename = f"{output_path}/model.tar.gz"
with tarfile.open(tar_filename, "w:gz") as tar:
tar.add(f"{output_path}/model.bin", arcname="model.bin")
mlflow.log_artifact(local_path=tar_filename)
In addition, you can use the mlflow.log_artifact()
method to save the model.tar.gz
file in MLflow so that you can directly use it later when you register the model to the model registry.
Navigate back to the MLflow UI. Click on the name of your experiment at the top of your screen starting with “credit-risk-model-experiment” to see the updated Runs table. Click on the name of your remote-training Run to see the overview of the training run including the associated hyperparameters, model metrics, and generated model artifacts.
The following figure shows the overview of a training run.
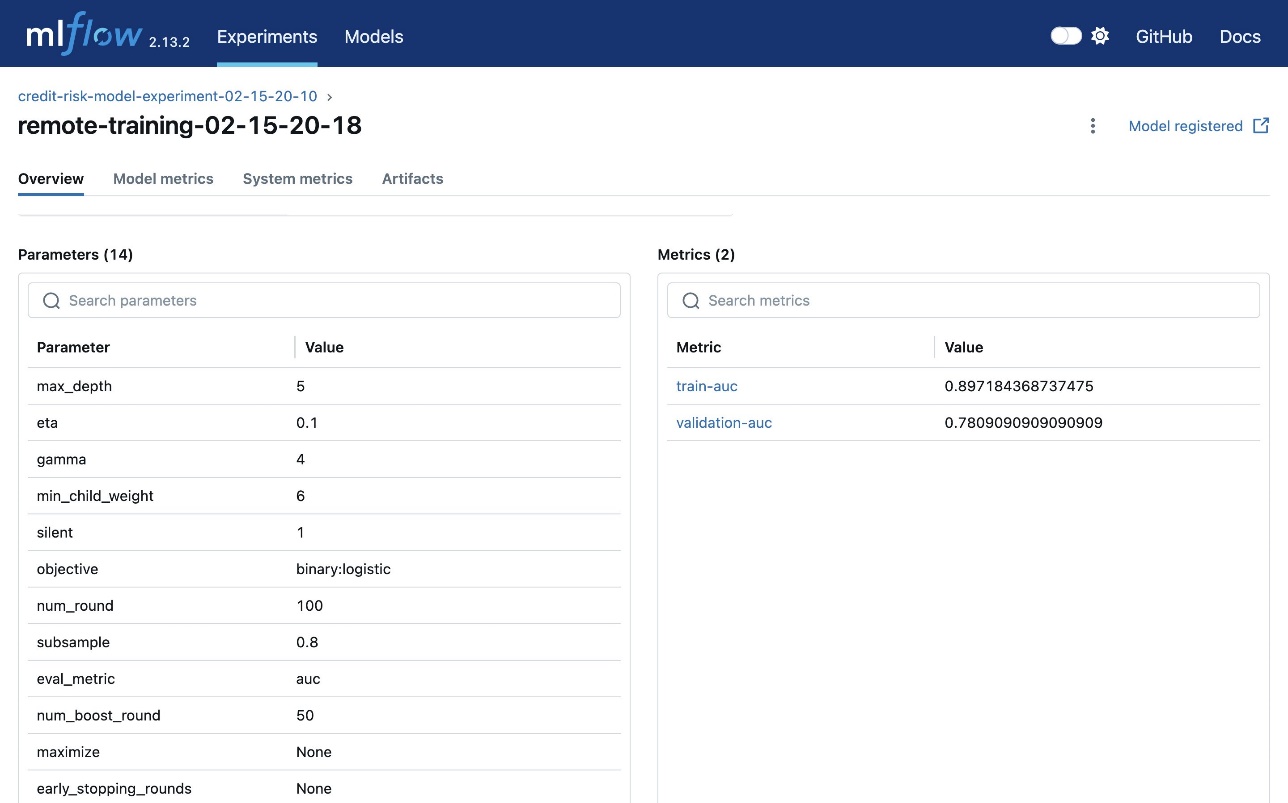
Click on the Model metrics tab to view the metrics tracked during the training run. The figure below shows the metrics of a training run.
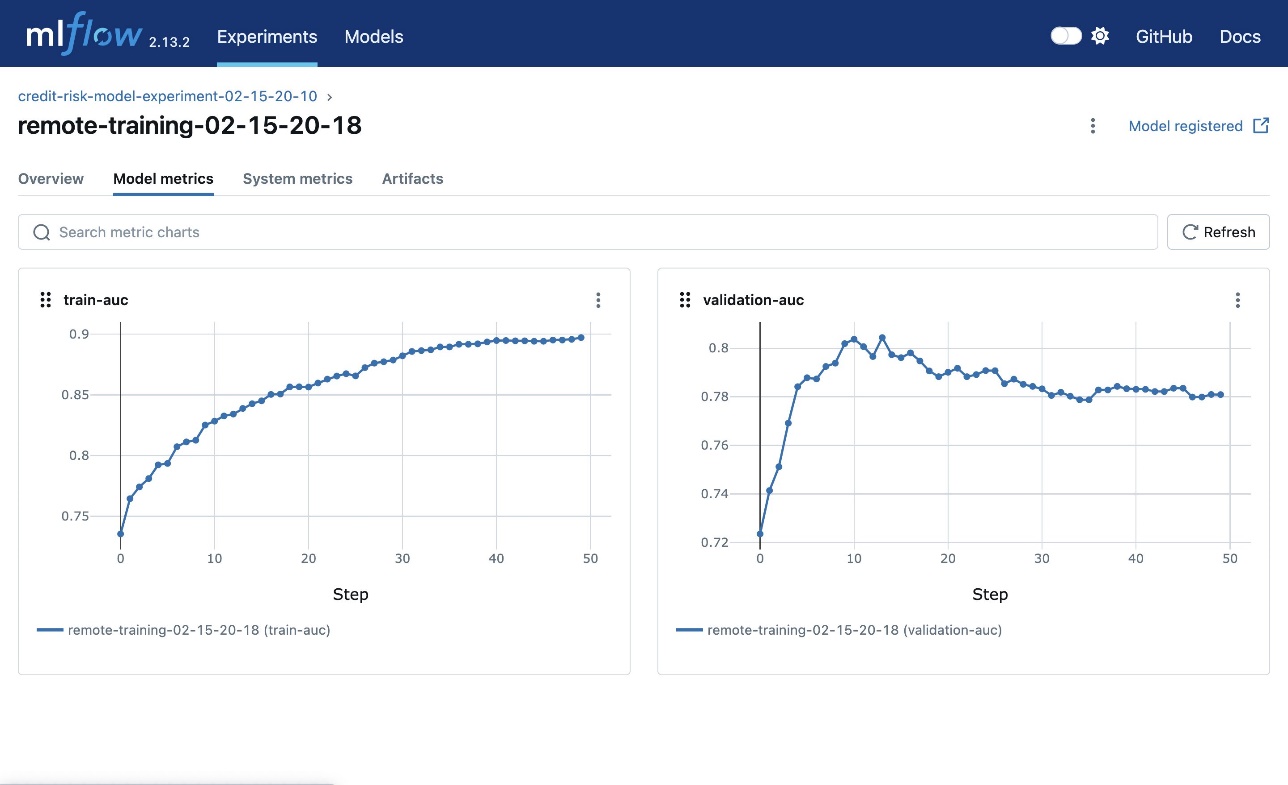
Click on the Artifacts tab to view the artifacts generated during the training run. The following figure shows an example of the generated artifacts.
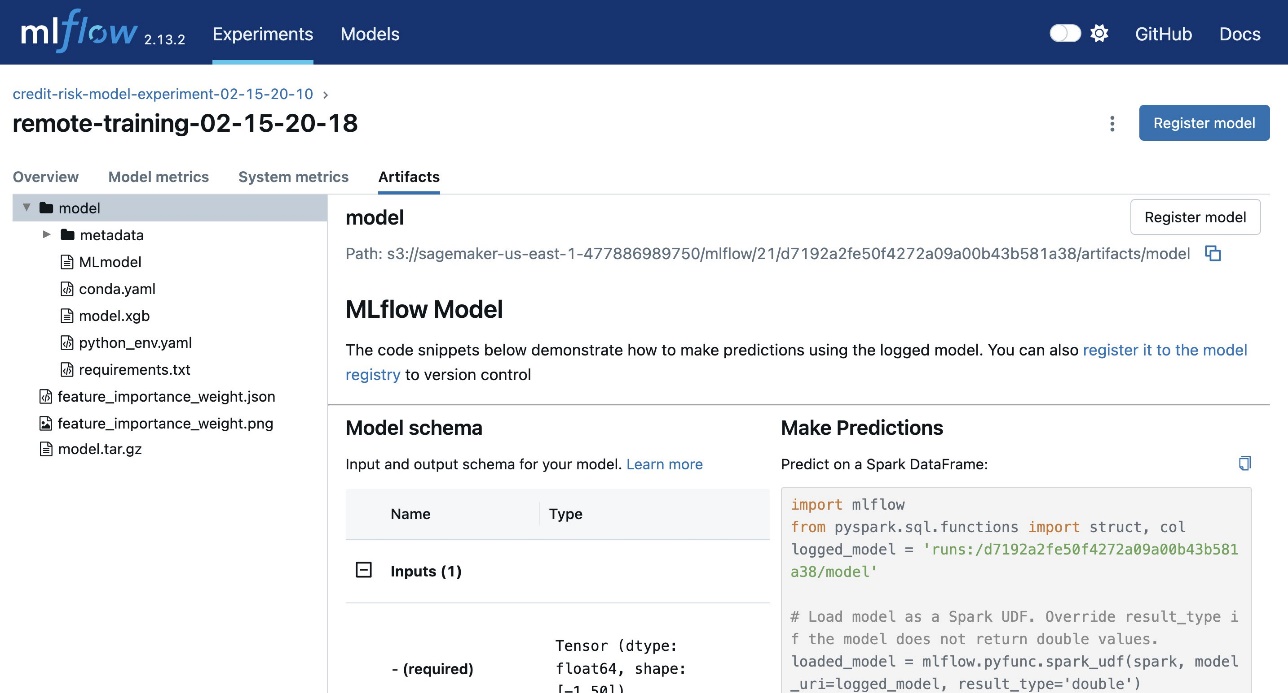
Registering the model to the model registry
ML experimentation is an iterative process and you typically end up with a number of candidate models. With MLflow, you can compare these models to identify the one that you want to move to quality assurance for approval. The following is an example of how to retrieve the best candidate using the MLflow API based on a specific metric.
from mlflow.entities import ViewType
run_filter = f"""
attributes.run_name LIKE "%training%"
attributes.status = 'FINISHED'
"""
runs_with_filter = mlflow.search_runs(
experiment_names=[experiment_name],
run_view_type=ViewType.ACTIVE_ONLY,
filter_string=run_filter,
order_by=["metrics.`validation-auc` DESC"],
)
best_run = runs_with_filter[:1]
artifact_uri = best_run['artifact_uri'][0]
After you have selected a model, you can register it to the shared model group in the shared services account. You can discover the model groups that are available to you either through the SageMaker Studio UI or programmatically.
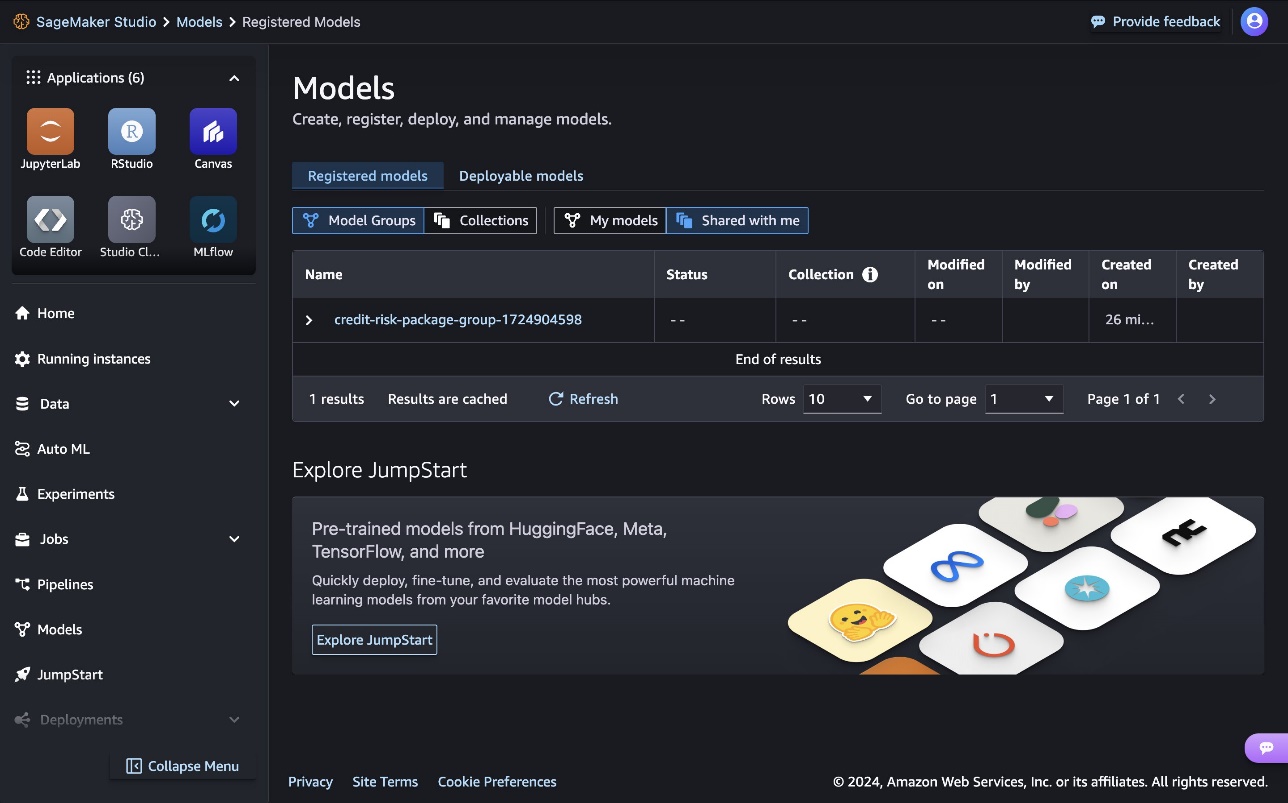
The final step is to register the candidate model to the model group as a new model version.
modelpackage_inference_specification = {
"InferenceSpecification": {
"Containers": [
{
"Image": "885854791233.dkr.ecr.us-east-1.amazonaws.com/sagemaker-distribution-prod@sha256:9e7622bbe2f3ee9dd516797bfe3ed310983b96190eeefbdeeeea69519d3946fe",
"ModelDataUrl": f"{artifact_uri}/model.tar.gz"
}
],
"SupportedContentTypes": [ "text/csv" ],
"SupportedResponseMIMETypes": [ "text/csv" ],
},
"ModelPackageGroupName" : model_package_group_arn,
"ModelPackageDescription" : "Model to detect credit risk",
"ModelApprovalStatus" : "PendingManualApproval"
}
model_package_group_name = "model-group-" + str(round(time.time()))
create_model_package_input_dict = {
"ModelPackageGroupName" : model_package_group_name,
"ModelPackageDescription" : "Model to detect credit risk",
"ModelApprovalStatus" : "PendingManualApproval"
}
create_model_package_input_dict.update(modelpackage_inference_specification)
create_model_package_response = sagemaker_client.create_model_package(**create_model_package_input_dict)
model_package_arn = create_model_package_response["ModelPackageArn"]
print('ModelPackage Version ARN : {}'.format(model_package_arn))
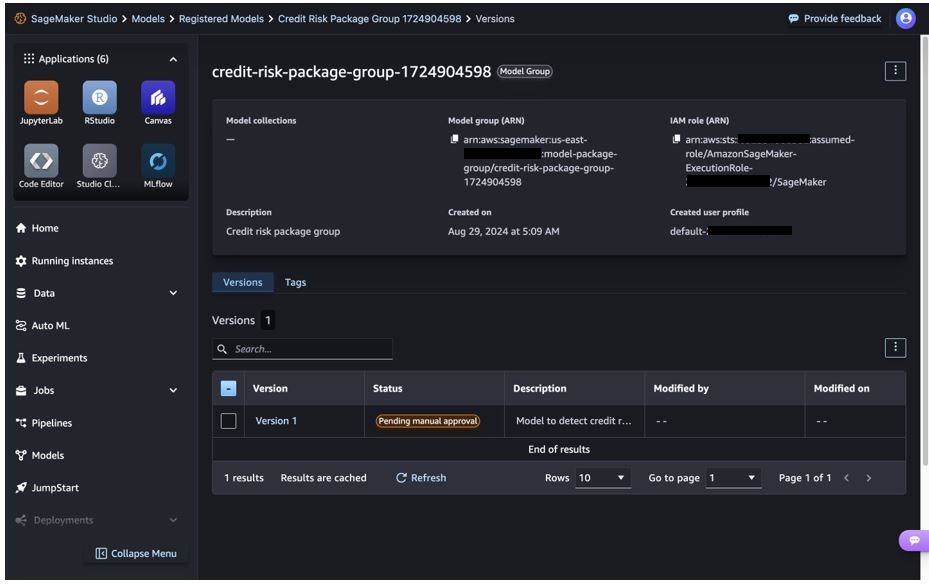
Design considerations for use case and model stage governance
Use case and model stage governance is a construct to track governance information of a use case or model across various stages in its journey to production. Also, periodic tracking of key model performance and drift metrics is used to surface those metrics for governance functions.
There are several use case and model stage governance attributes that need to be tracked, such as the following:
- Use case ID: Unique OD of the use case.
- Use case name: Name of the use case.
- Use case stage: Current stage of the use case. For example, proof of concept, development, QA, and so on.
- Model group: SageMaker model group name.
- Model version: SageMaker model version name.
- Model owner: Person or entity who owns the model.
- Model LoB: Model owner’s line of business.
- Model project: Project or use case that the model is part of.
- Model stage: Stage where the model version is deployed. For example, development, test, or production.
- Model status: Status of the model version in a given stage. For example, pending or approved.
- Model risk: Risk categorization of the model version. For example, high, medium, or low.
- Model validation metrics: Model validation metrics in JSON format.
- Model monitoring metrics: Model monitoring metrics in JSON format. This needs to include the endpoint from which this metrics was captured.
- Model audit timestamp: Timestamp when this record was updated.
- Model audit user: User who updated this record.
Create a use case or model stage governance construct with the preceding set of attributes and drive your deployment and governance workflows using this table. Next, we will describe the design considerations for deployment and governance workflows.
Design considerations for deployment and governance workflows
Following are the design consideration for the deployment and governance workflows:
- The model version is built in the development account and registered with pending status in the central model registry or model group.
- A sync process is triggered to capture the key model attributes, derive additional governance attributes, and create a development stage record in the model governance stage table. Model artifacts from the development account are synced into the central model registry account.
- The model owner approves the model version in the development stage for deployment to the test stage in the central model registry.
- A deployment pipeline is triggered and the model is deployed to the test environment and a new test stage record is created for that model version.
- The model version is tested and validated in the test environment and validation metrics are captured in the test stage record in the model governance stage construct.
- The governance officer verifies the model validation results and approves the model version for deployment to production. The production stage record is created for the model version in the model governance stage table.
- A deployment pipeline is triggered and the model is deployed to the production environment and the production stage record model status is updated to deployed for that model version.
- After the model monitoring jobs are set up, model inference metrics are periodically captured and aggregated and model metrics are updated in model stage governance table.
- The use case stage value is updated to the next stage when all models for that use case are approved in the previous stage.
Conclusion
In this post, we have discussed how to centralize your use case and model governance function in a multi-account environment using the new model group sharing feature of SageMaker Model Registry. We shared an architecture for setting up central use case and model governance and walked through the steps involved in building that architecture. We provided practical guidance for setting up cross-account model group sharing using SageMaker Studio and APIs. Finally, we discussed key design considerations for building the centralized use case and model governance functions to extend the native SageMaker capabilities. We encourage you to try this model-sharing feature along with centralizing your use case and model governance functions. You can leave feedback in the comments section.
About the authors
Ram Vittal is a Principal ML Solutions Architect at AWS. He has over 3 decades of experience architecting and building distributed, hybrid, and cloud applications. He is passionate about building secure and scalable AI/ML and big data solutions to help enterprise customers with their cloud adoption and optimization journey to improve their business outcomes. In his spare time, he rides his motorcycle and walks with his 3-year-old Sheepadoodle.
Anastasia Tzeveleka is a Senior Generative AI/ML Specialist Solutions Architect at AWS. As part of her work, she helps customers across EMEA build foundation models and create scalable generative AI and machine learning solutions using AWS services.
Siamak Nariman is a Senior Product Manager at AWS. He is focused on AI/ML technology, ML model management, and ML governance to improve overall organizational efficiency and productivity. He has extensive experience automating processes and deploying various technologies.
Madhubalasri B. is a Software Development Engineer at Amazon Web Services (AWS), focusing on the SageMaker Model Registry and machine learning governance domain. She has expertise in cross-account access and model sharing, ensuring secure, scalable, and compliant deployment of machine learning models. Madhubalasri is dedicated to driving innovation in ML governance and optimizing model management processes
Saumitra Vikaram is a Senior Software Engineer at AWS. He is focused on AI/ML technology, ML model management, ML governance, and MLOps to improve overall organizational efficiency and productivity.
Keshav Chandakis a Software Engineer at AWS with a focus on the SageMaker Repository Service. He specializes in developing capabilities to enhance governance and management of ML models.
Read More