NotebookLM is partnering with Spotify to create a personalized Wrapped AI podcast.Read More
Query structured data from Amazon Q Business using Amazon QuickSight integration
Amazon Q Business is a generative AI-powered assistant that can answer questions, provide summaries, generate content, and securely complete tasks based on data and information in your enterprise systems. Although generative AI is fueling transformative innovations, enterprises may still experience sharply divided data silos when it comes to enterprise knowledge, in particular between unstructured content (such as PDFs, Word documents, and HTML pages), and structured data (real-time data and reports stored in databases or data lakes). Both categories of data are typically queried and accessed using separate tools, from in-product browse and search functionality for unstructured data, to business intelligence (BI) tools like Amazon QuickSight for structured content.
Amazon Q Business offers an effective solution for quickly building conversational applications over unstructured content, with over 40 data connectors to popular content and storage management systems such as Confluence, SharePoint, and Amazon Simple Storage Service (Amazon S3), to aggregate enterprise knowledge. Customers are also looking for a unified conversational experience across all their knowledge repositories, regardless of the format the content is stored and organized as.
On December 3, 2024, Amazon Q Business announced the launch of its integration with QuickSight, allowing you to quickly connect your structured sources to your Amazon Q Business applications, creating a unified conversational experience for your end-users. The QuickSight integration offers an extensive set of over 20 structured data source connectors, including Amazon Redshift, PostgreSQL, MySQL, and Oracle, enabling you to quickly expand the conversational scope of your Amazon Q Business assistants to cover a wider range of knowledge sources. For the end-users, answers are returned in real time from your structured sources, combined with other relevant information found in unstructured repositories. Amazon Q Business uses the analytics and advanced visualization engine in QuickSight to generate accurate and simple-to-understand answers from structured sources.
In this post, we show you how to configure the QuickSight connection from Amazon Q Business and then ask questions to get real-time data and visualizations from QuickSight for structured data in addition to unstructured content.
Solution overview
The QuickSight feature in Amazon Q Business is available on the Amazon Q Business console as well as through Amazon Q Business APIs. This feature is implemented as a plugin within Amazon Q Business. After it’s enabled, this plugin will behave differently than other Amazon Q Business plugins—it will query QuickSight automatically for every user prompt, looking for relevant answers.
For AWS accounts that aren’t subscribed to QuickSight already, the Amazon Q Business admin completes the following steps:
- Create a QuickSight account.
- Connect your database in QuickSight to create a dataset.
- Create a topic in QuickSight, which is then used to make it searchable from your Amazon Q Business application.
When the feature is activated, Amazon Q Business will use your unstructured data sources configured in Amazon Q Business, as well as your structured content available using QuickSight, to generate a rich answer that includes narrative and visualizations. Depending on the question and data in QuickSight, Amazon Q Business may generate one or more visualizations as a response.
Prerequisites
You should have the following prerequisites:
- An AWS account where you can follow the instructions in this post.
- AWS IAM Identity Center set up to be used with Amazon Q Business. For more information, see Configure Amazon Q Business with AWS IAM Identity Center trusted identity propagation.
- At least one Amazon Q Business Pro user that has admin permissions to set up and configure Amazon Q Business. For pricing information, see Amazon Q Business pricing.
- An IAM Identity Center group that will be assigned the QuickSight Admin Pro role, for users who will manage and configure QuickSight.
- If a QuickSight account exists, then it needs to be in the same AWS account and AWS Region as Amazon Q Business, and configured with IAM Identity Center.
- A database that is installed and can be reached from QuickSight to load structured data (or you could create a dataset by uploading a CSV or XLS file). The database also needs credentials to create tables and insert data.
- Sample structured data to load into the database (along with insert statements).
Create an Amazon Q Business application
To use this feature, you need to have an Amazon Q Business application. If you don’t have an existing application, follow the steps in Discover insights from Amazon S3 with Amazon Q S3 connector to create an application along with an Amazon S3 data source. Upload the non-structured document(s) to Amazon S3 and sync the data source.
Create and configure a new QuickSight account
You can skip this section if you already have an existing QuickSight account. To create a QuickSight account, complete the following steps:
- On the Amazon Q Business console, navigate to your application.
- Choose Amazon QuickSight in the navigation pane.
- Choose Create QuickSight account.
- Under QuickSight account information, enter your account name and an email for account notifications.
- Under Assign QuickSight Admin Pro roles, choose the IAM Identity Center group you created as a prerequisite.
- Choose Next.
- Under Service access, select Create and use a new service role.
- Choose Authorize.
This will create a QuickSight account, assign the IAM Identity Center group as QuickSight Admin Pro, and authorize Amazon Q Business to access QuickSight.
You will see a dashboard with details for QuickSight. Currently, it will show zero datasets and topics.
- Choose Go to QuickSight.
You can now proceed to the next section to prepare your data.
Configure an existing QuickSight account
You can skip this section if you followed the previous steps and created a new QuickSight account.
If your current QuickSight account is not on IAM Identity Center, consider using a different AWS account without a QuickSight subscription for the purpose of testing this feature. From that account, you create an Amazon Q Business application on IAM Identity Center and go through the QuickSight integration setup steps on the Amazon Q Business console that will create the QuickSight account for you in IAM Identity Center. Remember to delete that new QuickSight account and Amazon Q Business application after your testing is done to avoid further billing.
Complete the following steps to set up the QuickSight connector from Amazon Q Business for an existing QuickSight account:
- On the Amazon Q Business console, navigate to your application.
- Choose Amazon QuickSight in the navigation pane.
- Choose Authorize QuickSight answers.
- Under Assign QuickSight Admin Pro roles, choose the IAM Identity Center group you created as a prerequisite.
- Under Service Access, select Create and use a new service role.
- Choose Save.
You will see a dashboard with details for QuickSight. If you already have a dataset and topics, they will show up here.
You’re now ready to add a dataset and topics in the next section.
Add data in QuickSight
In this section, we create an Amazon Redshift data source. You can instead create a data source from the database of your choice, use files in Amazon S3, or perform a direct upload of CSV files and connect to it. Refer to Creating a dataset from a database for more details.
To configure your data, complete the following steps:
- Create a new dataset with Amazon Redshift as a data source.
Configuring this connection offers multiple choices; choose the one that best fits your needs.
- Create a topic from the dataset. For more information, see Creating a topic.
- Optionally, create dashboards from the topic. If created, Amazon Q Business can use them.
Ask queries to Amazon Q Business
To start chatting with Amazon Q Business, complete the following steps:
- On the Amazon Q Business console, navigate to your application.
- Choose Amazon QuickSight in the navigation pane.
You should see the datasets and topics populated with values.
- Choose the link under Deployed URL.
We uploaded AWS Cost and Usage Reports for a specific AWS account in QuickSight using Amazon Redshift. We also uploaded Amazon service documentation into a data source using Amazon S3 into Amazon Q Business as unstructured data. We will ask questions related to our AWS costs and show how Amazon Q Business answers questions from both structured and unstructured data.
The following screenshot shows an example question that returns a response from only unstructured data.
The following screenshot shows an example question that returns a response from only structured data.
The following screenshot shows an example question that returns a response from both structured and unstructured data.
The following screenshot shows an example question that returns multiple visualizations from both structured and unstructured data.
Clean up
If you no longer want to use this Amazon Q Business feature, delete the resources you created to avoid future charges:
- Delete the Amazon Q Business application:
- On the Amazon Q Business console, choose Applications in the navigation pane.
- Select your application and on the Actions menu, choose Delete.
- Enter delete to confirm and choose Delete.
The process can take up to 15 minutes to complete.
- Delete the S3 bucket:
- Delete the QuickSight account:
- On the Amazon QuickSight console, choose Manage Amazon QuickSight.
- Choose Account setting and Manage.
- Delete the account.
- Delete your IAM Identity Center instance.
Conclusion
In this post, we showed how to include answers from your structured sources in your Amazon Q Business applications, using the QuickSight integration. This creates a unified conversational experience for your end-users that saves them time, helps them make better decisions through more complete answers, and improves their productivity.
At AWS re:Invent 2024, we also announced a similar unified experience enabling access to insights from unstructured data sources in Amazon Q in QuickSight powered by Amazon Q Business.
To learn about the new capabilities Amazon Q in QuickSight provides, see QuickSight Plugin.
To learn more about Amazon Q Business, refer to the Amazon Q Business User Guide.
To learn more about configuring a QuickSight dataset, see Manage your Amazon QuickSight datasets more efficiently with the new user interface.
QuickSight also offers querying unstructured data. For more details, refer to Integrate unstructured data into Amazon QuickSight using Amazon Q Business.
About the authors
Jiten Dedhia is a Sr. AIML Solutions Architect with over 20 years of experience in the software industry. He has helped Fortune 500 companies with their AIML/Generative AI needs.
Jean-Pierre Dodel is a Principal Product Manager for Amazon Q Business, responsible for delivering key strategic product capabilities including structured data support in Q Business, RAG. and overall product accuracy optimizations. He brings extensive AI/ML and Enterprise search experience to the team with over 7 years of product leadership at AWS.
Elevate customer experience by using the Amazon Q Business custom plugin for New Relic AI
Digital experience interruptions can harm customer satisfaction and business performance across industries. Application failures, slow load times, and service unavailability can lead to user frustration, decreased engagement, and revenue loss. The risk and impact of outages increase during peak usage periods, which vary by industry—from ecommerce sales events to financial quarter-ends or major product launches. According to New Relic’s 2024 Observability Forecast, businesses face a median annual downtime of 77 hours from high-impact outages. These outages can cost up to $1.9 million per hour.
New Relic is addressing these challenges by creating the New Relic AI custom plugin for Amazon Q Business. This custom plugin creates a unified solution that combines New Relic AI’s observability insights and recommendations and Amazon Q Business’s Retrieval Augmented Generation (RAG) capabilities, in and a natural language interface for east of use.
The custom plugin streamlines incident response, enhances decision-making, and reduces cognitive load from managing multiple tools and complex datasets. It empowers team members to interpret and act quickly on observability data, improving system reliability and customer experience. By using AI and New Relic’s comprehensive observability data, companies can help prevent issues, minimize incidents, reduce downtime, and maintain high-quality digital experiences.
This post explores the use case, how this custom plugin works, how it can be enabled, and how it can help elevate customers’ digital experiences.
The challenge: Resolving application problems before they impact customers
New Relic’s 2024 Observability Forecast highlights three key operational challenges:
- Tool and context switching – Engineers use multiple monitoring tools, support desks, and documentation systems. 45% of support engineers, application engineers, and SREs use five different monitoring tools on average. This fragmentation can cause missed SLAs and SLOs, confusion during critical incidents, and increased negative fiscal impact. Tool switching slows decision-making during outages or ecommerce disruptions.
- Knowledge accessibility – Scattered, hard-to-access knowledge, including runbooks and post-incident reports, hinders effective incident response. This can cause slow escalations, uncertain decisions, longer disruptions, and higher operational costs from redundant engineer involvement.
- Complexity in data interpretation – Team members may struggle to interpret monitoring and observability data due to complex applications with numerous services and cloud infrastructure entities, and unclear symptom-problem relationships. This complexity hinders quick, accurate data analysis and informed decision-making during critical incidents.
The custom plugin for Amazon Q Business addresses these challenges with a unified, natural language interface for critical insights. It uses AI to research and translate findings into clear recommendations, providing quick access to indexed runbooks and post-incident reports. This custom plugin streamlines incident response, enhances decision-making, and reduces effort in managing multiple tools and complex datasets.
Solution Overview
The New Relic custom plugin for Amazon Q Business centralizes critical information and actions in one interface, streamlining your workflow. It allows you to inquire about specific services, hosts, or system components directly. For instance, you can investigate a sudden spike in web service response times or a slow database. NR AI responds by analyzing current performance data and comparing it to historical trends and best practices. It then delivers detailed insights and actionable recommendations based on up-to-date production environment information.
The following diagram illustrates the workflow.
When a user asks a question in the Amazon Q interface, such as “Show me problems with the checkout process,” Amazon Q queries the RAG ingested with the customers’ runbooks. Runbooks are troubleshooting guides maintained by operational teams to minimize application interruptions. Amazon Q gains contextual information, including the specific service names and infrastructure information related to the checkout service, and uses the custom plugin to communicate with New Relic AI. New Relic AI initiates a deep dive analysis of monitoring data since the checkout service problems began.
New Relic AI conducts a comprehensive analysis of the checkout service. It examines service performance metrics, forecasts of key indicators like error rates, error patterns and anomalies, security alerts, and overall system status and health. The analysis results in a summarized alert intelligence report that identifies and explains root causes of checkout service issues. This report provides clear, actionable recommendations and includes real-time application performance insights. It also offers direct links to detailed New Relic interfaces. Users can access this comprehensive summary without leaving the Amazon Q interface.
The custom plugin presents information and insights directly within the Amazon Q Business interface, eliminating the need to switch between the New Relic and Amazon Q interfaces, and enabling faster problem resolution.
Potential impacts
The New Relic Intelligent Observability platform provides comprehensive incident response and application and infrastructure performance monitoring capabilities for SREs, application engineers, support engineers, and DevOps professionals. Organizations using New Relic report significant improvements in their operations, achieving a 65% reduction in incidents, 10 times more deployments, and 50% faster release times while maintaining 99.99% uptime. When you combine New Relic insights with Amazon Q Business, you can further reduce incidents, deploy higher-quality code more frequently, and create more reliable experiences for your customers:
- Detect and resolve incidents faster – With this custom plugin, you can reduce undetected incidents and resolve issues more quickly. Incidents often occur when teams miss early warning signs or can’t connect symptoms to underlying problems, leading to extended service disruptions. Although New Relic collects and generates data that can identify these warning signs, teams working in separate tools might not have access to these critical insights. For instance, support specialists might not have direct access to monitoring dashboards, making it challenging to identify emerging issues. The custom plugin consolidates these monitoring insights, helping you more effectively identify and understand related issues.
- Simplify incident management – The custom plugin enhances support engineers’ and incident responders’ efficiency by streamlining their workflow. The custom plugin allows you to manage incidents without switching between New Relic AI and Amazon Q during critical moments. The integrated interface removes context switching, enabling both technical and non-technical users to access vital monitoring data quickly within the Amazon Q interface. This comprehensive approach speeds up troubleshooting, minimizes downtime, and boosts overall system reliability.
- Build reliability across teams – The custom plugin makes application and infrastructure performance monitoring insights accessible to team members beyond traditional observability users. translates complex production telemetry data into clear, actionable insights for product managers, customer service specialists, and executives. By providing a unified interface for querying and resolving issues, it empowers your entire team to maintain and improve digital services, regardless of their technical expertise. For example, when a customer service specialist receives user complaints, they can quickly investigate application performance issues without navigating complex monitoring tools or interpreting alert conditions. This unified view enables everyone supporting your enterprise software to understand and act on insights about application health and performance. The result is a more collaborative approach across multiple enterprise teams, leading to more reliable system maintenance and excellent customer experiences.
Conclusion
The New Relic AI custom plugin represents a step forward in digital experience management. By addressing key challenges such as tool fragmentation, knowledge accessibility, and data complexity, this solution empowers teams to deliver superior digital experiences. This collaboration between AWS and New Relic opens up possibilities for building more robust digital infrastructures, advancing innovation in customer-facing technologies, and setting new benchmarks in proactive IT problem-solving.
To learn more about improving your operational efficiency with AI-powered observability, refer to the Amazon Q Business User Guide and explore New Relic AI capabilities. To get started on training, enroll for free Amazon Q training from AWS Training and Certification.
About New Relic
New Relic is a leading cloud-based observability platform that helps businesses optimize the performance and reliability of their digital systems. New Relic processes 3 EB of data annually. Over 5 billion data points are ingested and 2.4 trillion queries are executed every minute across 75,000 active customers. The platform serves over 333 billion web requests each day. The median platform response time is 60 milliseconds.
About the authors
Meena Menon is a Sr. Customer Solutions Manager at AWS.
Sean Falconer is a Sr. Solutions Architect at AWS.
Nava Ajay Kanth Kota is a Senior Partner Solutions Architect at AWS. He is currently part of the Amazon Partner Network (APN) team that closely works with ISV Storage Partners. Prior to AWS, his experience includes running Storage, Backup, and Hybrid Cloud teams and his responsibilities included creating Managed Services offerings in these areas.
David Girling is a Senior AI/ML Solutions Architect with over 20 years of experience in designing, leading, and developing enterprise systems. David is part of a specialist team that focuses on helping customers learn, innovate, and utilize these highly capable services with their data for their use cases.
Camden Swita is Head of AI and ML Innovation at New Relic specializing in developing compound AI systems, agentic frameworks, and generative user experiences for complex data retrieval, analysis, and actioning.
Amazon SageMaker launches the updated inference optimization toolkit for generative AI
Today, Amazon SageMaker is excited to announce updates to the inference optimization toolkit, providing new functionality and enhancements to help you optimize generative AI models even faster. These updates build on the capabilities introduced in the original launch of the inference optimization toolkit (to learn more, see Achieve up to ~2x higher throughput while reducing costs by ~50% for generative AI inference on Amazon SageMaker with the new inference optimization toolkit – Part 1).
The following are the key additions to the inference optimization toolkit:
- Speculative decoding support for Meta Llama 3.1 models – The toolkit now supports speculative decoding for the latest Meta Llama 3.1 70B and 405B (FP8) text models, allowing you to accelerate inference process.
- Support for FP8 quantization – The toolkit has been updated to enable FP8 (8-bit floating point) quantization, helping you further optimize model size and inference latency for GPUs. FP8 offers several advantages over FP32 (32-bit floating point) for deep learning model inference, including reduced memory usage, faster computation, lower power consumption, and broader applicability because FP8 quantization can be applied to key model components like the KV cache, attention, and MLP linear layers.
- Compilation support for TensorRT-LLM – You can now use the toolkit’s compilation capabilities to integrate your generative AI models with NVIDIA’s TensorRT-LLM, delivering enhanced performance by optimizing the model with ahead-of-time compilation. You reduce the model’s deployment time and auto scaling latency because the model weights don’t require just-in-time compilation when the model deploys to a new instance.
These updates build on the toolkit’s existing capabilities, allowing you to reduce the time it takes to optimize generative AI models from months to hours, and achieve best-in-class performance for your use case. Simply choose from the available optimization techniques, apply them to your models, validate the improvements, and deploy the models in just a few clicks through SageMaker.
In this post, we discuss these new features of the toolkit in more detail.
Speculative decoding
Speculative decoding is an inference technique that aims to speed up the decoding process of large language models (LLMs) for latency-critical applications, without compromising the quality of the generated text. The key idea is to use a smaller, less powerful, but faster language model called the draft model to generate candidate tokens. These candidate tokens are then validated by the larger, more powerful, but slower target model. At each iteration, the draft model generates multiple candidate tokens. The target model verifies the tokens, and if it finds a particular token unacceptable, it rejects it and regenerates that token itself. This allows the larger target model to focus on verification, which is faster than auto-regressive token generation. The smaller draft model can quickly generate all the tokens and send them in batches to the target model for parallel evaluation, significantly speeding up the final response generation.
With the updated SageMaker inference toolkit, you get out-of-the-box support for speculative decoding that has been tested for performance at scale on various popular open source LLMs. The toolkit provides a pre-built draft model, eliminating the need to invest time and resources in building your own draft model from scratch. Alternatively, you can also use your own custom draft model, providing flexibility to accommodate your specific requirements. To showcase the benefits of speculative decoding, let’s look at the throughput (tokens per second) for a Meta Llama 3.1 70B Instruct model deployed on an ml.p4d.24xlarge instance using the Meta Llama 3.2 1B Instruct draft model.
Given the increase in throughput that is realized with speculative decoding, we can also see the blended price difference when using speculative decoding vs. when not using speculative decoding. Here we have calculated the blended price as a 3:1 ratio of input to output tokens. The blended price is defined as follows:
- Total throughput (tokens per second) = NumberOfOutputTokensPerRequest / (ClientLatency / 1,000) x concurrency
- Blended price ($ per 1 million tokens) = (1−(discount rate)) × (instance per hour price) ÷ ((total token throughput per second) × 60 × 60 ÷ 10^6)) ÷ 4
- Discount rate assuming a 26% Savings Plan
Quantization
Quantization is one of the most popular model compression methods to accelerate model inference. From a technical perspective, quantization has several benefits:
- It reduces model size, which makes it suitable for deploying using fewer GPUs with lower total device memory available.
- It reduces memory bandwidth pressure by using fewer-bit data types.
- If offers increased space for the KV cache. This enables larger batch sizes and sequence lengths.
- It significantly speeds up matrix multiplication (GEMM) operations on the NVIDIA architecture, for example, up to twofold for FP8 compared to the FP16/BF16 data type in microbenchmarks.
With this launch, the SageMaker inference optimization toolkit now supports FP8 and SmoothQuant (TensorRT-LLM only) quantization. SmoothQuant is a post-training quantization (PTQ) technique for LLMs that reduces memory and speeds up inference without sacrificing accuracy. It migrates quantization difficulty from activations to weights, which are easier to quantize. It does this by introducing a hyperparameter to calculate a per-channel scale that balances the quantization difficulty of activations and weights.
The current generation of instances like p5 and g6 provide support for FP8 using specialized tensor cores. FP8 represents float point numbers in 8 bits instead of the usual 16. At the time of writing, vLLM and TRT-LLM support quantizing the KV cache, attention, and linear layers for text-only LLMs. This reduces memory footprint, increases throughput, and lowers latency. Whereas both weights and activations can be quantized for p5 and g6 instances (W8A8), only weights can be quantized for p4d and g5 instances (W8A16). Though FP8 quantization has minimal impact on accuracy, you should always evaluate the quantized model on your data and for your use case. You can evaluate the quantized model through Amazon SageMaker Clarify. For more details, see Understand options for evaluating large language models with SageMaker Clarify.
The following graph compares the throughput of a FP8 quantized Meta Llama 3.1 70B Instruct model against a non-quantized Meta Llama 3.1 70B Instruct model on an ml.p4d.24xlarge instance.
The quantized model has a smaller memory footprint and it can be deployed to a smaller (and cheaper) instance type. In this post, we have deployed the quantized model on g5.12xlarge.
The following graph shows the price difference per million tokens between the FP8-quantized model deployed on g5.12xlarge and the non-quantized version deployed on p4d.24xlarge.
Our analysis shows a clear price-performance edge for the FP8 quantized model over the non-quantized approach. However, quantization has an impact on model accuracy, so we strongly testing the quantized version of the model on your datasets.
The following is the SageMaker Python SDK code snippet for quantization. You just need to provide the quantization_config
attribute in the optimize()
function:
Refer to the following code example to learn more about how to enable FP8 quantization and speculative decoding using the optimization toolkit for a pre-trained Amazon SageMaker JumpStart model. If you want to deploy a fine-tuned model with SageMaker JumpStart using speculative decoding, refer to the following notebook.
Compilation
Compilation optimizes the model to extract the best available performance on the chosen hardware type, without any loss in accuracy. For compilation, the SageMaker inference optimization toolkit provides efficient loading and caching of optimized models to reduce model loading and auto scaling time by up to 40–60 % for Meta Llama 3 8B and 70B.
Model compilation enables running LLMs on accelerated hardware, such as GPUs, while simultaneously optimizing the model’s computational graph for optimal performance on the target hardware. When using the Large Model Inference (LMI) Deep Learning Container (DLC) with the TensorRT-LLM framework, the compiler is invoked from within the framework and creates compiled artifacts. These compiled artifacts are unique for a combination of input shapes, precision of the model, tensor parallel degree, and other framework- or compiler-level configurations. Although the compilation process avoids overhead during inference and enables optimized inference, it can take a lot of time.
To avoid re-compiling every time a model is deployed onto a GPU with the TensorRT-LLM framework, SageMaker introduces the following features:
- A cache of pre-compiled artifacts – This includes popular models like Meta Llama 3.1. When using an optimized model with the compilation config, SageMaker automatically uses these cached artifacts when the configurations match.
- Ahead-of-time compilation – The inference optimization toolkit enables you to compile your models with the desired configurations before deploying them on SageMaker.
The following graph illustrates the improvement in model loading time when using pre-compiled artifacts with the SageMaker LMI DLC. The models were compiled with a sequence length of 4096 and a batch size of 16, with Meta Llama 3.1 8B deployed on a g5.12xlarge (tensor parallel degree = 4) and Meta Llama 3.1 70B Instruct on a p4d.24xlarge (tensor parallel degree = 8). As you can see on the graph, the bigger the model, the bigger the benefit of using a pre-compiled model (16% improvement for Meta Llama 3 8B and 43% improvement for Meta Llama 3 70B).
Compilation using the SageMaker Python SDK
For the SageMaker Python SDK, you can configure the compilation by changing the environment variables in the .optimize() function. For more details on compilation_config, refer to TensorRT-LLM ahead-of-time compilation of models tutorial.
Refer to the following notebook for more information on how to enable TensorRT-LLM compilation using the optimization toolkit for a pre-trained SageMaker JumpStart model.
Amazon SageMaker Studio UI experience
In this section, let’s walk through the Amazon SageMaker Studio UI experience to run an inference optimization job. In this case, we use the Meta Llama 3.1 70B Instruct model, and for the optimization option, we quantize the model using INT4-AWQ and then use the SageMaker JumpStart suggested draft model Meta Llama 3.2 1B Instruct for speculative decoding.
First, we search for the Meta Llama 3.1 70B Instruct model in the SageMaker JumpStart model hub and choose Optimize on the model card.
The Create inference optimization job page provides you options to choose the type of optimization. In this case, we choose to take advantage of the benefits of both INT4-AWQ quantization and speculative decoding.
For the draft model, you have a choice to use the SageMaker recommended draft model, choose one the SageMaker JumpStart models, or bring your own draft model.
For this scenario, we choose the SageMaker recommended Meta Llama 3.2 1B Instruct model as the draft model and start the optimization job.
When the optimization job is complete, you have an option to evaluate performance or deploy the model onto a SageMaker endpoint for inference.
Pricing
For compilation and quantization jobs, SageMaker will optimally choose the right instance type, so you don’t have to spend time and effort. You will be charged based on the optimization instance used. To learn more, see Amazon SageMaker pricing. For speculative decoding, there is no additional optimization cost involved; the SageMaker inference optimization toolkit will package the right container and parameters for the deployment on your behalf.
Conclusion
To get started with the inference optimization toolkit, refer to Achieve up to 2x higher throughput while reducing cost by up to 50% for GenAI inference on SageMaker with new inference optimization toolkit: user guide – Part 2. This post will walk you through how to use the inference optimization toolkit when using SageMaker inference with SageMaker JumpStart and the SageMaker Python SDK. You can use the inference optimization toolkit with supported models on SageMaker JumpStart. For the full list of supported models, refer to Inference optimization for Amazon SageMaker models.
About the Authors
Marc Karp is an ML Architect with the Amazon SageMaker Service team. He focuses on helping customers design, deploy, and manage ML workloads at scale. In his spare time, he enjoys traveling and exploring new places.
Dmitry Soldatkin is a Senior AI/ML Solutions Architect at Amazon Web Services (AWS), helping customers design and build AI/ML solutions. Dmitry’s work covers a wide range of ML use cases, with a primary interest in Generative AI, deep learning, and scaling ML across the enterprise. He has helped companies in many industries, including insurance, financial services, utilities, and telecommunications. He has a passion for continuous innovation and using data to drive business outcomes.
Raghu Ramesha is a Senior ML Solutions Architect with the Amazon SageMaker Service team. He focuses on helping customers build, deploy, and migrate ML production workloads to SageMaker at scale. He specializes in machine learning, AI, and computer vision domains, and holds a master’s degree in Computer Science from UT Dallas. In his free time, he enjoys traveling and photography.
Rishabh Ray Chaudhury is a Senior Product Manager with Amazon SageMaker, focusing on Machine Learning inference. He is passionate about innovating and building new experiences for Machine Learning customers on AWS to help scale their workloads. In his spare time, he enjoys traveling and cooking. You can find him on LinkedIn.
Lokeshwaran Ravi is a Senior Deep Learning Compiler Engineer at AWS, specializing in ML optimization, model acceleration, and AI security. He focuses on enhancing efficiency, reducing costs, and building secure ecosystems to democratize AI technologies, making cutting-edge ML accessible and impactful across industries.
Syngenta develops a generative AI assistant to support sales representatives using Amazon Bedrock Agents
This post was written with Zach Marston and Serg Masis from Syngenta.
Syngenta and AWS collaborated to develop Cropwise AI, an innovative solution powered by Amazon Bedrock Agents, to accelerate their sales reps’ ability to place Syngenta seed products with growers across North America. Cropwise AI harnesses the power of generative AI using AWS to enhance Syngenta’s seed selection tools and streamline the decision-making process for farmers and sales representatives. This conversational agent offers a new intuitive way to access the extensive quantity of seed product information to enable seed recommendations, providing farmers and sales representatives with an additional tool to quickly retrieve relevant seed information, complementing their expertise and supporting collaborative, informed decision-making.
Generative AI is reshaping businesses and unlocking new opportunities across various industries. As a global leader in agriculture, Syngenta has led the charge in using data science and machine learning (ML) to elevate customer experiences with an unwavering commitment to innovation. Building on years of experience in deploying ML and computer vision to address complex challenges, Syngenta introduced applications like NemaDigital, Moth Counter, and Productivity Zones. Now, Syngenta is advancing further by using large language models (LLMs) and Amazon Bedrock Agents to implement Cropwise AI on AWS, marking a new era in agricultural technology.
In this post, we discuss Syngenta’s journey in developing Cropwise AI.
The business challenge
Syngenta offers a diverse portfolio of seed products in North America, reflecting the company’s commitment to growers meeting them at the field. The seed selection process involves careful consideration of multiple factors, including seed product characteristics, specific growing environments, and the unique practices and goals of each farmer. Many farmers struggle to navigate the complex decision-making process and might not always choose the most suitable seeds for their specific conditions, potentially impacting crop yields and profitability.
Recognizing an opportunity to improve this selection process, Syngenta collaborated with the AWS Generative AI Innovation Center, an AWS program that fosters collaboration between customers and AWS experts. This collaboration yielded Cropwise AI, which improves the efficiency of sales rep’s interactions with customers to suggest Syngenta seed products.
Cropwise AI
Cropwise AI transforms the seed selection process in several powerful ways. It simplifies complex charts and tables into a natural, conversational experience, providing users with quick, on-demand access to detailed seed product information on a mobile device. The tool also streamlines data navigation, allowing users to efficiently explore and compare Syngenta’s extensive seed catalogue. Additionally, Cropwise AI enables personalized recommendations at scale, tailoring seed choices to align with local conditions and specific farm needs, creating a more precise and accessible selection process.
Cropwise AI enhances the seed selection process by acting as a valuable companion to the expertise of sales representatives and the decision-making process of farmers. For sales representatives, it empowers them with deeper insights, enabling more informed recommendations. For farmers, it offers an accessible resource for exploring and comparing seed options. The following example shows the Cropwise AI mobile app in GHX 2.0 describing a common seed product (left) and the transcribed conversation (right).
![]() |
Cropwise AI: Hello, Jack! What can I do for you? |
Me: Tell me about the G11V76 hybrid of golden harvest | |
Cropwise AI: G11V76 is a corn hybrid developed by Golden Harvest. Let’s delve into its characteristics:
|
The collaboration between Syngenta and AWS showcases the transformative power of LLMs and AI agents. With the capability to embed human expertise and communicate in natural language, generative AI amplifies human abilities, allowing organizations to utilize knowledge at scale. This project is just one example of how Syngenta is using advaned AWS AI services to drive innovation in agriculture.
In the following sections, we provide a detailed overview of the Cropwise AI solution by diving deep into the underlying workflows. We explore how you can use Amazon Bedrock Agents with generative AI and cutting-edge AWS technologies, which offer a transformative approach to supporting sales reps across this industry (and beyond).
Solution overview
Cropwise AI is built on an AWS architecture designed to address these challenges through scalability, maintainability, and security. The architecture is divided into two main components: the agent architecture and knowledge base architecture. This solution is also deployed by using the AWS Cloud Development Kit (AWS CDK), which is an open-source software development framework that defines cloud infrastructure in modern programming languages and provisions it through AWS CloudFormation.
Agent architecture
The following diagram illustrates the serverless agent architecture with standard authorization and real-time interaction, and an LLM agent layer using Amazon Bedrock Agents for multi-knowledge base and backend orchestration using API or Python executors. Domain-scoped agents enable code reuse across multiple agents.
Amazon Bedrock Agents offers several key benefits for Syngenta compared to other solutions like LangGraph:
- Flexible model selection – Syngenta can access multiple state-of-the-art foundation models (FMs) like Anthropic’s Claude 3.5 Haiku and Sonnet, Meta Llama 3.1, and others, and can switch between these models without changing code. They can select the model that is accurate enough for a specific workflow and yet cost-effective.
- Ease of deployment – It is seamlessly integrated with other AWS services and has a unified development and deployment workflow.
- Enterprise-grade security – With the robust security infrastructure of AWS, Amazon Bedrock is in scope for common compliance standards, including ISO, SOC, and CSA STAR Level 2; is HIPAA eligible; and you can use Amazon Bedrock in compliance with the GDPR.
- Scalability and integration – It allows for straightforward API integration with existing systems and has built-in support for orchestrating multiple actions. This enables Syngenta to effortlessly build and scale their AI application.
The agent architecture handles user interactions and processes data to deliver accurate recommendations. It uses the following AWS services:
- Serverless computing with AWS Lambda – The architecture begins with AWS Lambda, which provides serverless computing power, allowing for automatic scaling based on workload demands. When custom processing tasks are required, such as invoking the Amazon Bedrock agent or integrating with various data sources, the Lambda function is triggered to run these tasks efficiently.
- Lambda-based action groups – The Amazon Bedrock agent directs user queries to functional actions which may use API-connections to gather data for use in workflows from various sources, model integrations to generate recommendations using the gathered data, or Python executions to extract specific pieces of information relevant to a user’s workflow and aid in product comparisons.
- Secure user and data management – User authentication and authorization are managed centrally and securely through Amazon Cognito. This service makes sure user identities and access rights are handled effectively, maintaining the confidentiality and security of the system. The user identity gets propagated over a secure side channel (session attributes) to the agent and action groups. This allows them to access user-specific or restricted information, whereas each access can be authorized within the workflow. The session attributes aren’t shared with the LLM, making sure that authorization decisions are done on validated and tamper-proof data. For more information about this approach, see Implement effective data authorization mechanisms to secure your data used in generative AI applications.
- Real-time data synchronization with AWS AppSync – To make sure that users always have access to the most up-to-date information, the solution uses AWS AppSync. It facilitates real-time data synchronization and updates by using GraphQL APIs, providing seamless and responsive user experiences.
- Efficient metadata storage with Amazon DynamoDB – To support quick and efficient data retrieval, document metadata is stored in Amazon DynamoDB. This NoSQL database is optimized for rapid access, making sure the knowledge base remains responsive and searchable.
- Centralized logging and monitoring with Amazon CloudWatch – To maintain operational excellence, Amazon CloudWatch is employed for centralized logging and monitoring. It provides deep operational insights, aiding in troubleshooting, performance tuning, and making sure the system runs smoothly.
The architecture is designed for flexibility and resilience. AWS Lambda enables the seamless execution of various tasks, including data processing and API integration, and AWS AppSync provides real-time interaction and data flow between the user and the system. By using Amazon Cognito for authentication, the agent maintains confidentiality, protecting sensitive user data.
Knowledge base architecture
The following diagram illustrates the knowledge base architecture.
The knowledge base architecture focuses on processing and storing agronomic data, providing quick and reliable access to critical information. Key components include:
- Orchestrated document processing with AWS Step Functions – The document processing workflow begins with AWS Step Functions, which orchestrates each step in the process. From the initial ingestion of documents to their final storage, Step Functions makes sure that data handling is seamless and efficient.
- Automated text extraction with Amazon Textract – As documents are uploaded to Amazon Simple Storage Service (Amazon S3), Amazon Textract is triggered to automatically extract text from these documents. This extracted text is then available for further analysis and the creation of metadata, adding layout-based structure and meaning to the raw data.
- Primary data storage with Amazon S3 – The processed documents, along with their associated metadata, are securely stored in Amazon S3. This service acts as the primary storage solution, providing consistent access and organized data management for all stored content.
- Efficient metadata storage with DynamoDB – To support quick and efficient data retrieval, document metadata is stored in DynamoDB.
- Amazon Bedrock Knowledge Bases – The final textual content and metadata information gets ingested into Amazon Bedrock Knowledge Bases for efficient retrieval during the agentic workflow, backed by an Amazon OpenSearch Service vector store. Agents can use one or multiple knowledge bases, depending on the context in which they are used.
This architecture enables comprehensive data management and retrieval, supporting the agent’s ability to deliver precise recommendations. By integrating Step Functions with Amazon Textract, the system automates document processing, reducing manual intervention and improving efficiency.
Use cases
Cropwise AI addresses several critical use cases, providing tangible benefits to sales representatives and growers:
- Product recommendation – A sales representative or grower seeks advice on the best seed choices for specific environmental conditions, such as “My region is very dry and windy. What corn hybrids do you suggest for my field?”. The agent uses natural language processing (NLP) to understand the query and uses underlying agronomy models to recommend optimal seed choices tailored to specific field conditions and agronomic needs. By integrating multiple data sources and explainable research results, including weather patterns and soil data, the agent delivers personalized and context-aware recommendations.
- Querying agronomic models – A grower has questions about plant density or other agronomic factors affecting yield, such as “What are the yields I can expect with different seeding rates for corn hybrid G11V76?” The agent interprets the query, accesses the appropriate agronomy model, and provides a simple explanation that is straightforward for the grower to understand. This empowers growers to make informed decisions based on scientific insights, enhancing crop management strategies.
- Integration of multiple data sources – A grower can ask for a recommendation that considers real-time data like current weather conditions or market prices, such as “Is it a good time to apply herbicides to my corn field?” The agent pulls data from various sources, integrates it with existing agronomy models, and provides a recommendation that accounts for current conditions. This holistic approach makes sure that recommendations are timely, relevant, and actionable.
Results
The implementation of Cropwise AI has yielded significant improvements in the efficiency and accuracy of agricultural product recommendations:
- Sales representatives are now able to generate recommendations with analytical models five times faster, allowing them to focus more time on building relationships with customers and exploring new opportunities
- The natural language interface simplifies interactions, reducing the learning curve for new users and minimizing the need for extensive training
- The agent’s ability to track recommendation outcomes provides valuable insights into customer preferences and helps to improve personalization over time
To evaluate the results, Syngenta collected a dataset of 100 Q&A pairs from sales representatives and ran them against the agent. Next to manual human evaluation, they also used an LLM as a judge (Ragas) to assess the answers generated by Cropwise AI. The following graph shows the results of this evaluation, which indicate that the provided answer relevancy, conciseness, and faithfulness are very high.
![]() |
Conclusion
Cropwise AI is revolutionizing the agricultural industry by addressing the unique challenges faced by seed representatives, particularly those managing multiple seed products for growers. This AI-powered tool streamlines the process of placing diverse seed products, making it effortless for sales reps to deliver precise recommendations tailored to each grower’s unique needs. By using advanced generative AI and AWS technologies, such as Amazon Bedrock Agents, Cropwise AI significantly boosts operational efficiency, enhancing the accuracy, speed, and user experience of product recommendations.
The success of this solution highlights AI’s potential to transform traditional agricultural practices, opening doors for further innovations across the sector. As Cropwise AI continues to evolve, efforts will focus on expanding capabilities, enhancing data integration, and maintaining compliance with shifting regulatory standards.
Ultimately, Cropwise AI not only refines the sales process but also empowers sales representatives and growers with actionable insights and robust tools essential for thriving in a dynamic agricultural environment. By fostering an efficient, intuitive recommendation process, Cropwise AI optimizes crop yields and enhances overall customer satisfaction, positioning it as an invaluable resource for the modern agricultural sales force.
For more details, explore the Amazon Bedrock Samples GitHub repo and Syngenta Cropwise AI.
About the Authors
Zach Marston is a Digital Product Manager at Syngenta, focusing on computational agronomy solutions. With a PhD in Entomology and Plant Pathology, he combines scientific knowledge with over a decade of experience in agricultural machine learning. Zach is dedicated to exploring innovative ways to enhance farming efficiency and sustainability through AI and data-driven approaches.
Serg Masis is a Senior Data Scientist at Syngenta, and has been at the confluence of the internet, application development, and analytics for the last two decades. He’s the author of the bestselling book “Interpretable Machine Learning with Python,” and the upcoming book “DIY AI.” He’s passionate about sustainable agriculture, data-driven decision-making, responsible AI, and making AI more accessible.
Arlind Nocaj is a Senior Solutions Architect at AWS in Zurich, Switzerland, who guides enterprise customers through their digital transformation journeys. With a PhD in network analytics and visualization (Graph Drawing) and over a decade of experience as a research scientist and software engineer, he brings a unique blend of academic rigor and practical expertise to his role. His primary focus lies in using the full potential of data, algorithms, and cloud technologies to drive innovation and efficiency. His areas of expertise include machine learning and MLOps, with particular emphasis on document processing, natural language processing, and large language models.
Victor Antonino, M.Eng, is a Senior Machine Learning Engineer at AWS with over a decade of experience in generative AI, computer vision, and MLOps. At AWS, Victor has led transformative projects across industries, enabling customers to use cutting-edge machine learning technologies. He designs modern data architectures and enables seamless machine learning deployments at scale, supporting diverse use cases in finance, manufacturing, healthcare, and media. Victor holds several patents in AI technologies, has published extensively on clustering and neural networks, and actively contributes to the open source community with projects that democratize access to AI tools.
Laksh Puri is a Generative AI Strategist at the AWS Generative AI Innovation Center, based in London. He works with large organizations across EMEA on their AI strategy, including advising executive leadership to define and deploy impactful generative AI solutions.
Hanno Bever is a Senior Machine Learning Engineer in the AWS Generative AI Innovation Center based in Berlin. In his 5 years at Amazon, he has helped customers across all industries run machine learning workloads on AWS. He is specialized in migrating foundation model training and inference tasks to AWS silicon chips AWS Trainium and AWS Inferentia.
Latest NVIDIA AI, Robotics and Quantum Computing Software Comes to AWS
Expanding what’s possible for developers and enterprises in the cloud, NVIDIA and Amazon Web Services are converging at AWS re:Invent in Las Vegas this week to showcase new solutions designed to accelerate AI and robotics breakthroughs and simplify research in quantum computing development.
AWS re:Invent is a conference for the global cloud-computing community packed with keynotes and more than 2,000 technical sessions.
Announcement highlights include the availability of NVIDIA DGX Cloud on AWS and enhanced AI, quantum computing and robotics tools.
NVIDIA DGX Cloud on AWS for AI at Scale
The NVIDIA DGX Cloud AI computing platform is now available through AWS Marketplace Private Offers, offering a high-performance, fully managed solution for enterprises to train and customize AI models.
DGX Cloud offers flexible terms, a fully managed and optimized platform, and direct access to NVIDIA experts to help businesses scale their AI capabilities quickly.
Early adopter Leonardo.ai, part of the Canva family, is already using DGX Cloud on AWS to develop advanced design tools.
AWS Liquid-Cooled Data Centers With NVIDIA Blackwell
Newer AI servers benefit from liquid cooling to cool high-density compute chips more efficiently for better performance and energy efficiency. AWS has developed solutions that provide configurable liquid-to-chip cooling across its data centers.
The cooling solution announced today will seamlessly integrate air- and liquid-cooling capabilities for the most powerful rack-scale AI supercomputing systems like NVIDIA GB200 NVL72, as well as AWS’ network switches and storage servers.
This flexible, multimodal cooling design provides maximum performance and efficiency for running AI models and will be used for the next-generation NVIDIA Blackwell platform.
Blackwell will be the foundation of Amazon EC2 P6 instances, DGX Cloud on AWS and Project Ceiba.
NVIDIA Advances Physical AI With Accelerated Robotics Simulation on AWS
NVIDIA is also expanding the reach of NVIDIA Omniverse on AWS with NVIDIA Isaac Sim, now running on high-performance Amazon EC2 G6e instances accelerated by NVIDIA L40S GPUs.
Available now, this reference application built on NVIDIA Omniverse enables developers to simulate and test AI-driven robots in physically based virtual environments.
One of the many workflows enabled by Isaac Sim is synthetic data generation. This pipeline is now further accelerated with the infusion of OpenUSD NIM microservices, from scene creation to data augmentation.
Robotics companies such as Aescape, Cohesive Robotics, Cobot, Field AI, Standard Bots, Swiss Mile and Vention are using Isaac Sim to simulate and validate the performance of their robots prior to deployment.
In addition, Rendered.ai, SoftServe and Tata Consultancy Services are using the synthetic data generation capabilities of Omniverse Replicator and Isaac Sim to bootstrap perception AI models that power various robotics applications.
NVIDIA BioNeMo on AWS for Advanced AI-Based Drug Discovery
NVIDIA BioNeMo NIM microservices and AI Blueprints, developed to advance drug discovery, are now integrated into AWS HealthOmics, a fully managed biological data compute and storage service designed to accelerate scientific breakthroughs in clinical diagnostics and drug discovery.
This collaboration gives researchers access to AI models and scalable cloud infrastructure tailored to drug discovery workflows. Several biotech companies already use NVIDIA BioNeMo on AWS to drive their research and development pipelines.
For example, A-Alpha Bio, a biotechnology company based in Seattle, recently published a study in biorxiv describing a collaborative effort with NVIDIA and AWS to develop and deploy an antibody AI model called AlphaBind.
Using AlphaBind via the BioNeMo framework on Amazon EC2 P5 instances equipped with NVIDIA H100 Tensor Core GPUs, A-Alpha Bio achieved a 12x increase in inference speed and processed over 108 million inference calls in two months.
Additionally, SoftServe today launched Drug Discovery, its generative AI solution built with NVIDIA Blueprints, to enable computer-aided drug discovery and efficient drug development. This solution is set to deliver faster workflows and will soon be available in AWS Marketplace.
Real-Time AI Blueprints: Ready-to-Deploy Options for Video, Cybersecurity and More
NVIDIA’s latest AI Blueprints are available for instant deployment on AWS, making real-time applications like vulnerability analysis for container security, and video search and summarization agents readily accessible.
Developers can easily integrate these blueprints into existing workflows to speed deployments.
Developers and enterprises can use the NVIDIA AI Blueprint for video search and summarization to build visual AI agents that can analyze real-time or archived videos to answer user questions, generate summaries and enable alerts for specific scenarios.
AWS collaborated with NVIDIA to provide a reference architecture applying the NVIDIA AI Blueprint for vulnerability analysis to augment early security patching in continuous integration pipelines on AWS cloud-native services.
NVIDIA CUDA-Q on Amazon Braket: Quantum Computing Made Practical
NVIDIA CUDA-Q is now integrated with Amazon Braket to streamline quantum computing development. CUDA-Q users can use Amazon Braket’s quantum processors, while Braket users can tap CUDA-Q’s GPU-accelerated workflows for development and simulation.
The CUDA-Q platform allows developers to build hybrid quantum-classical applications and run them on many different types of quantum processors, simulated and physical.
Now preinstalled on Amazon Braket, CUDA-Q provides a seamless development platform for hybrid quantum-classical applications, unlocking new potential in quantum research.
Enterprise Platform Providers and Consulting Leaders Advance AI With NVIDIA on AWS
Leading software platforms and global system integrators are helping enterprises rapidly scale generative AI applications built with NVIDIA AI on AWS to drive innovation across industries.
Cloudera is using NVIDIA AI on AWS to enhance its new AI inference solution, helping Mercy Corps improve the precision and effectiveness of its aid distribution technology.
Cohesity has integrated NVIDIA NeMo Retriever microservices in its generative AI-powered conversational search assistant, Cohesity Gaia, to improve the recall performance of retrieval-augmented generation. Cohesity customers running on AWS can take advantage of the NeMo Retriever integration within Gaia.
DataStax announced that Wikimedia Deutschland is applying the DataStax AI Platform to make Wikidata available to developers as an embedded vectorized database. The Datastax AI Platform is built with NVIDIA NeMo Retriever and NIM microservices, and available on AWS.
Deloitte’s C-Suite AI now supports NVIDIA AI Enterprise software, including NVIDIA NIM microservices and NVIDIA NeMo for CFO-specific use cases, including financial statement analysis, scenario modeling and market analysis.
RAPIDS Quick Start Notebooks Now Available on Amazon EMR
NVIDIA and AWS are also speeding data science and data analytics workloads with the RAPIDS Accelerator for Apache Spark, which accelerates analytics and machine learning workloads with no code change and reduces data processing costs by up to 80%.
Quick Start notebooks for RAPIDS Accelerator for Apache Spark are now available on Amazon EMR, Amazon EC2 and Amazon EMR on EKS. These offer a simple way to qualify Spark jobs tuned to maximize the performance of RAPIDS on GPUs, all within AWS EMR.
NVIDIA and AWS Power the Next Generation of Industrial Edge Systems
The NVIDIA IGX Orin and Jetson Orin platforms now integrate seamlessly with AWS IoT Greengrass to streamline the deployment and running of AI models at the edge and to efficiently manage fleets of connected devices at scale. This combination enhances scalability and simplifies the deployment process for industrial and robotics applications.
Developers can now tap into NVIDIA’s advanced edge computing power with AWS’ purpose-built IoT services, creating a secure, scalable environment for autonomous machines and smart sensors. A guide for getting started, authored by AWS, is now available to support developers putting these capabilities to work.
The integration underscores NVIDIA’s work in advancing enterprise-ready industrial edge systems to enable rapid, intelligent operations in real-world applications.
Catch more of NVIDIA’s work at AWS: re:Invent 2024 through live demos, technical sessions and hands-on labs.
See notice regarding software product information.
NVIDIA Advances Physical AI With Accelerated Robotics Simulation on AWS
Field AI is building robot brains that enable robots to autonomously manage a wide range of industrial processes. Vention creates pretrained skills to ease development of robotic tasks. And Cobot offers Proxie, an AI-powered cobot designed to handle material movement and adapt to dynamic environments, working seamlessly alongside humans.
These leading robotics startups are all making advances using NVIDIA Isaac Sim on Amazon Web Services. Isaac Sim is a reference application built on NVIDIA Omniverse for developers to simulate and test AI-driven robots in physically based virtual environments.
NVIDIA announced at AWS re:Invent today that Isaac Sim now runs on Amazon Elastic Cloud Computing (EC2) G6e instances accelerated by NVIDIA L40S GPUs. And with NVIDIA OSMO, a cloud-native orchestration platform, developers can easily manage their complex robotics workflows across their AWS computing infrastructure.
This combination of NVIDIA-accelerated hardware and software — available on the cloud — allows teams of any size to scale their physical AI workflows.
Physical AI describes AI models that can understand and interact with the physical world. It embodies the next wave of autonomous machines and robots, such as self-driving cars, industrial manipulators, mobile robots, humanoids and even robot-run infrastructure like factories and warehouses.
With physical AI, developers are embracing a three computer solution for training, simulation and inference to make breakthroughs.
Yet physical AI for robotics systems requires robust training datasets to achieve precision inference in deployment. Developing such datasets, however, and testing them in real situations can be impractical and costly.
Simulation offers an answer, as it can significantly accelerate the training, testing and deployment of AI-driven robots.
Harnessing L40S GPUs in the Cloud to Scale Robotics Simulation and Training
Simulation is used to verify, validate and optimize robot designs as well as the systems and their algorithms before deployment. Simulation can also optimize facility and system designs before construction or remodeling starts for maximum efficiencies, reducing costly manufacturing change orders.
Amazon EC2 G6e instances accelerated by NVIDIA L40S GPUs provide a 2x performance gain over the prior architecture, while allowing the flexibility to scale as scene and simulation complexity grows. The instances are used to train many computer vision models that power AI-driven robots. This means the same instances can be extended for various tasks, from data generation to simulation to model training.
Using NVIDIA OSMO in the cloud allows teams to orchestrate and scale complex robotics development workflows across distributed computing resources, whether on premises or in the AWS cloud.
Isaac Sim provides access to the latest robotics simulation capabilities and the cloud, fostering collaboration. One of the critical workflows is generating synthetic data for perception model training.
Using a reference workflow that combines NVIDIA Omniverse Replicator, a framework for building custom synthetic data generation (SDG) pipelines and a core extension of Isaac Sim, with NVIDIA NIM microservices, developers can build generative AI-enabled SDG pipelines.
These include the USD Code NIM microservice for generating Python USD code and answering OpenUSD queries, and the USD Search NIM microservice for exploring OpenUSD assets using natural language or image inputs. The Edify 360 HDRi NIM microservice generates 360-degree environment maps, while the Edify 3D NIM microservice creates ready-to-edit 3D assets from text or image prompts. This eases the synthetic data generation process by reducing many tedious and manual steps, from asset creation to image augmentation, using the power of generative AI.
Rendered.ai’s synthetic data engineering platform integrated with Omniverse Replicator enables companies to generate synthetic data for computer vision models used in industries from security and intelligence to manufacturing and agriculture.
SoftServe, an IT consulting and digital services provider, uses Isaac Sim to generate synthetic data and validate robots used in vertical farming with Pfeifer & Langen, a leading European food producer.
Tata Consultancy Services is building custom synthetic data generation pipelines to power its Mobility AI suite to address automotive and autonomous use cases by simulating real-world scenarios. Its applications include defect detection, end-of-line quality inspection and hazard avoidance.
Learning to Be Robots in Simulation
While Isaac Sim enables developers to test and validate robots in physically accurate simulation, Isaac Lab, an open-source robot learning framework built on Isaac Sim, provides a virtual playground for building robot policies that can run on AWS Batch.
Because these simulations are repeatable, developers can easily troubleshoot and reduce the number of cycles required for validation and testing.
Several robotics developers are embracing NVIDIA Isaac on AWS to develop physical AI, such as:
- Aescape’s robots are able to provide precision-tailored massages by accurately modeling and tuning onboard sensors in Isaac Sim.
- Cobot has used Isaac Sim with its AI-powered cobot, Proxie, to optimize logistics in warehouses, hospitals, manufacturing sites, and more.
- Cohesive Robotics has integrated Isaac Sim into its software framework called Argus OS for developing and deploying robotic workcells used in high-mix manufacturing environments.
- Field AI, a builder of robot foundation models, uses Isaac Sim and Isaac Lab to evaluate the performance of its models in complex, unstructured environments across industries such as construction, manufacturing, oil and gas, mining and more.
- Standard Bots is simulating and validating the performance of its R01 robot used in manufacturing and machining setup.
- Swiss Mile is using Isaac Sim and Isaac Lab for robot learning so that wheeled quadruped robots can perform tasks autonomously with new levels of efficiency in factories and warehouses.
- Vention, which offers a full-stack cloud-based automation platform, is harnessing Isaac Sim for developing and testing new capabilities for robot cells used by small to medium-size manufacturers.
Learn more about Isaac Sim 4.2, now available on Amazon EC2 G6e instances powered by NVIDIA L40S GPUs on AWS Marketplace.
New NVIDIA Certifications Expand Professionals’ Credentials in AI Infrastructure and Operations
As generative AI continues to grow, implementing and managing the right infrastructure becomes even more critical to ensure the secure and efficient development and deployment of AI-based solutions.
To meet these needs, NVIDIA has introduced two professional-level certifications that offer structured paths for infrastructure and operations practitioners to enhance and validate the skills needed to work effectively with advanced AI technologies.
The certification exams and recommended training to prepare for them are designed for network and system administrators, DevOps and MLOps engineers, and others who need to understand AI infrastructure and operations.
NVIDIA’s certification program equips professionals with skills in areas such as AI infrastructure, deep learning and accelerated computing to enhance their career prospects and give them a competitive edge in these high-demand fields.
Developed in collaboration with industry experts, the program features relevant content that emphasizes practical application alongside theoretical knowledge.
The NVIDIA-Certified Professional: AI Infrastructure certification is designed for practitioners seeking to showcase advanced skills in deploying AI infrastructure. Candidates must demonstrate expertise in GPU and DPU installation, hardware validation and system optimization for both AI and HPC workloads. The exam also tests proficiency in managing physical layers, configuring MIG, deploying the NVIDIA BlueField operating system, and integrating NVIDIA’s cloud-native stack with Docker and NVIDIA NGC.
To prepare for this professional-level certification, candidates are encouraged to attend the AI Infrastructure Professional Workshop. This hands-on training covers critical AI data center technologies, including compute platforms, GPU operations, networking, storage solutions and BlueField DPUs. The workshop is recommended for professionals aiming to elevate their AI infrastructure expertise.
The NVIDIA-Certified Professional: AI Operations certification is tailored for individuals seeking proficiency in managing and optimizing AI operations. The exam tests expertise in managing AI data centers, including the use of Kubernetes, Slurm, MIG, BCM, NGC containers, storage configuration and DPU services.
To prepare for this professional-level certification, candidates are encouraged to attend the AI Operations Professional Workshop, where they will gain hands-on experience in managing AI data centers, including compute platforms, networking, storage and GPU virtualization. The workshop also provides practical experience with NVIDIA AI software and solutions, including NGC containers and the NVIDIA AI Enterprise software suite, making it ideal for professionals looking to deepen their AI operations expertise.
Both of these professional-level certifications build upon the foundational knowledge covered in the NVIDIA-Certified Associate: AI Infrastructure and Operations certification.
Additional NVIDIA certifications include:
- NVIDIA-Certified Associate: Generative AI LLMs — validates skills in using generative AI and large language models
- NVIDIA-Certified Associate: Generative AI Multimodal — focuses on multimodal AI content creation
Saleh Hassan, an embedded software engineer at Two Six Technologies, successfully completed three NVIDIA certification exams at the NVIDIA AI Summit in Washington, D.C., earlier this year.
“The knowledge I gained has definitely made me a better developer when it comes to integrating AI,” said Hassan, who encourages others to pursue certifications as a key milestone for advancing their AI careers.
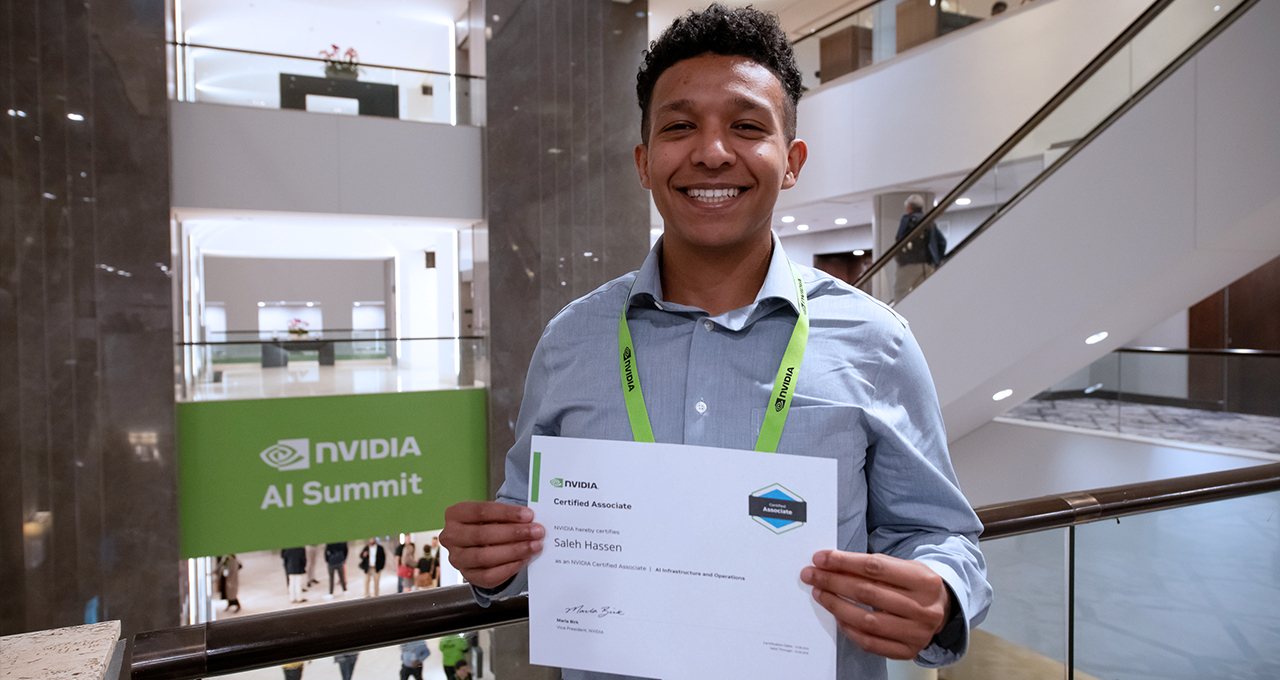
All NVIDIA certifications are part of a comprehensive learning path that offers foundational courses, advanced training and hands-on labs to thoroughly prepare candidates for real-world applications.
The certifications support individual career growth and organizations can use them to enhance workforce capabilities.
Explore the options on the NVIDIA Certification portal and sign up for NVIDIA’s monthly newsletter to stay updated on the latest offerings.
7 pieces of AI news we announced in November
From a powerful new AI-flood forecasting initiative to help from AI in advancing quantum computers.Read More
How AI Can Enhance Disability Inclusion, Special Education
A recent survey from the Special Olympics Global Center for Inclusion in Education shows that while a majority of students with an intellectual and developmental disability (IDD) and their parents view AI as a potentially transformative technology, only 35% of educators believe that AI developers currently account for the needs and priorities of students with IDD.
In this episode of the NVIDIA AI Podcast, U.S. Special Advisor on International Disability Rights at the U.S. Department of State Sara Minkara and Timothy Shriver, chairman of the board of Special Olympics, discuss AI’s potential to enhance special education and disability inclusion.
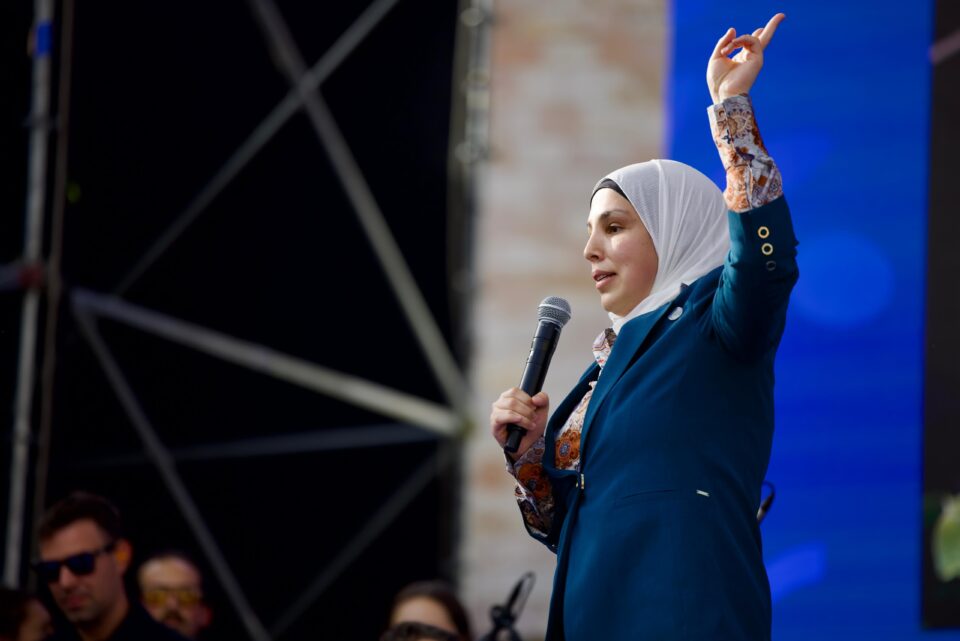
They highlight the critical need to include the voices from disability communities in AI development and policy conversations. Minkara and Shriver also explain the cultural, financial and social importance of building an inclusive future.
Time Stamps
2:12: Minkara and Shriver’s work on disability inclusion
9:47: Benefits of AI for people with disabilities
20:46: Notes from the recent G7 ministerial meeting on inclusion and disability
24:51: Challenges and future directions of AI in disability inclusion
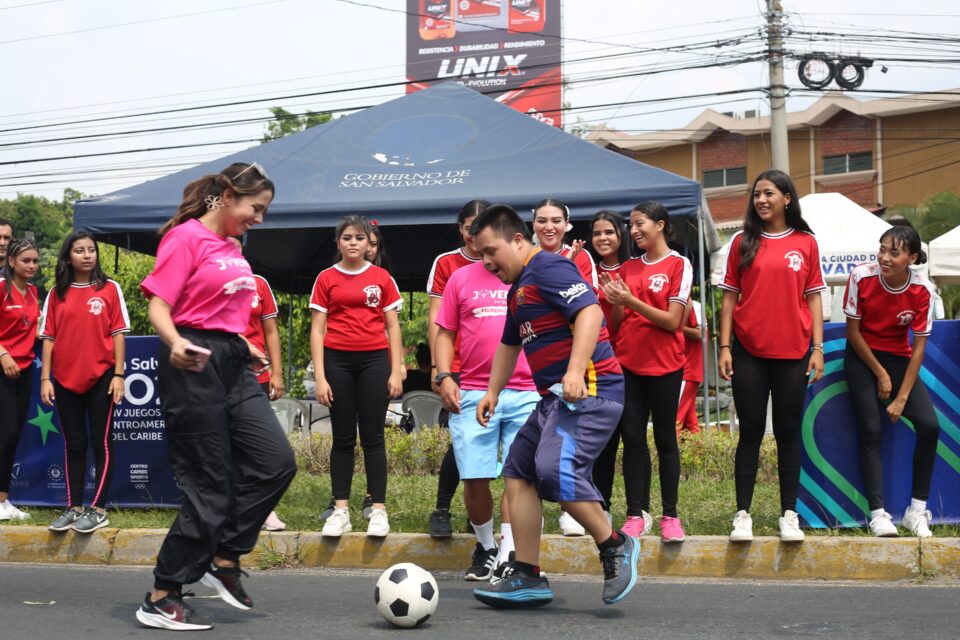
You Might Also Like…
Taking AI to School: A Conversation With MIT’s Anant Agarwal – Ep. 197
Educators and technologists alike have long been excited about AI’s potential to transform teaching and learning. Anant Agarwal, founder of edX and chief platform officer at 2U, talked about the future of online education and how AI is revolutionizing the learning experience.
NVIDIA’s Louis Stewart on How AI Is Shaping Workforce Development – Ep. 237
Workforce development is central to ensuring the changes brought by AI benefit all of us. Louis Stewart, head of strategic initiatives for NVIDIA’s global developer ecosystem, explains what workforce development looks like in the age of AI, and why it all starts with education.
Dotlumen CEO Cornel Amariei on Assistive Technology for the Visually Impaired – Ep. 217
Equipped with sensors and powered by AI, Dotlumen Glasses compute a safely walkable path for persons who are blind or have low vision, and offer haptic — or tactile — feedback on how to proceed via corresponding vibrations. Dotlumen founder and CEO Cornel Amariei discusses the challenges and breakthroughs of developing assistive technology.
How the Ohio Supercomputer Center Drives the Future of Computing – Ep. 213
Alan Chalker, director of strategic programs at the Ohio Supercomputing Center, dives into the history and evolution of the OSC, how it’s working with client companies like NASCAR, and how the center’s Open OnDemand program empowers Ohio higher education institutions and industries with computational services and training and educational programs.