From boardroom to break room, generative AI took this year by storm, stirring discussion across industries about how to best harness the technology to enhance innovation and creativity, improve customer service, transform product development and even boost communication.
The adoption of generative AI and large language models is rippling through nearly every industry, as incumbents and new entrants reimagine products and services to generate an estimated $1.3 trillion in revenue by 2032, according to a report by Bloomberg Intelligence.
Yet, some companies and startups are still slow to adopt AI, sticking to experimentation and siloed projects even as the technology advances at a dizzying pace. That’s partly because AI benefits vary by company, use case and level of investment.
Cautious approaches are giving way to optimism. Two-thirds of the respondents to Forrester Research’s 2024 State of AI Survey believe their organizations would require less than 50% return on investments to consider their AI initiatives successful.
The next big thing on the horizon is agentic AI, a form of autonomous or “reasoning” AI that requires using diverse language models, sophisticated retrieval-augmented generation stacks and advanced data architectures.
NVIDIA experts in industry verticals already shared their expectations for the year ahead. Now, hear from company experts driving innovation in AI across enterprises, research and the startup ecosystem:
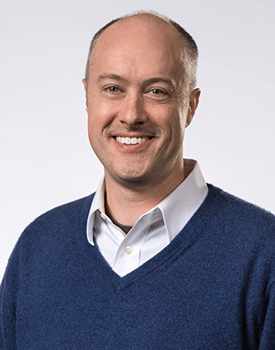
IAN BUCK
Vice President of Hyperscale and HPC
Inference drives the AI charge: As AI models grow in size and complexity, the demand for efficient inference solutions will increase.
The rise of generative AI has transformed inference from simple recognition of the query and response to complex information generation — including summarizing from multiple sources and large language models such as OpenAI o1 and Llama 450B — which dramatically increases computational demands. Through new hardware innovations, coupled with continuous software improvements, performance will increase and total cost of ownership is expected to shrink by 5x or more.
Accelerate everything: With GPUs becoming more widely adopted, industries will look to accelerate everything, from planning to production. New architectures will add to that virtuous cycle, delivering cost efficiencies and an order of magnitude higher compute performance with each generation.
As nations and businesses race to build AI factories to accelerate even more workloads, expect many to look for platform solutions and reference data center architectures or blueprints that can get a data center up and running in weeks versus months. This will help them solve some of the world’s toughest challenges, including quantum computing and drug discovery.
Quantum computing — all trials, no errors: Quantum computing will make significant strides as researchers focus on supercomputing and simulation to solve the greatest challenges to the nascent field: errors.
Qubits, the basic unit of information in quantum computing, are susceptible to noise, becoming unstable after performing only thousands of operations. This prevents today’s quantum hardware from solving useful problems. In 2025, expect to see the quantum computing community move toward challenging, but crucial, quantum error correction techniques. Error correction requires quick, low-latency calculations. Also expect to see quantum hardware that’s physically colocated within supercomputers, supported by specialized infrastructure.
AI will also play a crucial role in managing these complex quantum systems, optimizing error correction and enhancing overall quantum hardware performance. This convergence of quantum computing, supercomputing and AI into accelerated quantum supercomputers will drive progress in realizing quantum applications for solving complex problems across various fields, including drug discovery, materials development and logistics.
BRYAN CATANZARO
Vice President of Applied Deep Learning Research
Putting a face to AI: AI will become more familiar to use, emotionally responsive and marked by greater creativity and diversity. The first generative AI models that drew pictures struggled with simple tasks like drawing teeth. Rapid advances in AI are making image and video outputs much more photorealistic, while AI-generated voices are losing that robotic feel.
These advancements will be driven by the refinement of algorithms and datasets and enterprises’ acknowledgment that AI needs a face and a voice to matter to 8 billion people. This will also cause a shift from turn-based AI interactions to more fluid and natural conversations. Interactions with AI will no longer feel like a series of exchanges but instead offer a more engaging and humanlike conversational experience.
Rethinking industry infrastructure and urban planning: Nations and industries will begin examining how AI automates various aspects of the economy to maintain the current standard of living, even as the global population shrinks.
These efforts could help with sustainability and climate change. For instance, the agriculture industry will begin investing in autonomous robots that can clean fields and remove pests and weeds mechanically. This will reduce the need for pesticides and herbicides, keeping the planet healthier and freeing up human capital for other meaningful contributions. Expect to see new thinking in urban planning offices to account for autonomous vehicles and improve traffic management.
Longer term, AI can help find solutions for reducing carbon emissions and storing carbon, an urgent global challenge.
KARI BRISKI
Vice President of Generative AI Software
A symphony of agents — AI orchestrators: Enterprises are set to have a slew of AI agents, which are semiautonomous, trained models that work across internal networks to help with customer service, human resources, data security and more. To maximize these efficiencies, expect to see a rise in AI orchestrators that work across numerous agents to seamlessly route human inquiries and interpret collective results to recommend and take actions for users.
These orchestrators will have access to deeper content understanding, multilingual capabilities and fluency with multiple data types, ranging from PDFs to video streams. Powered by self-learning data flywheels, AI orchestrators will continuously refine business-specific insights. For instance, in manufacturing, an AI orchestrator could optimize supply chains by analyzing real-time data and making recommendations on production schedules and supplier negotiations.
This evolution in enterprise AI will significantly boost productivity and innovation across industries while becoming more accessible. Knowledge workers will be more productive because they can tap into a personalized team of AI-powered experts. Developers will be able to build these advanced agents using customizable AI blueprints.
Multistep reasoning amplifies AI insights: AI for years has been good at giving answers to specific questions without having to delve into the context of a given query. With advances in accelerated computing and new model architectures, AI models will tackle increasingly complex problems and respond with greater accuracy and deeper analysis.
Using a capability called multistep reasoning, AI systems increase the amount of “thinking time” by breaking down large, complex questions into smaller tasks — sometimes even running multiple simulations — to problem-solve from various angles. These models dynamically evaluate each step, ensuring contextually relevant and transparent responses. Multistep reasoning also involves integrating knowledge from various sources to enable AI to make logical connections and synthesize information across different domains.
This will likely impact fields ranging from finance and healthcare to scientific research and entertainment. For example, a healthcare model with multistep reasoning could make a number of recommendations for a doctor to consider, depending on the patient’s diagnosis, medications and response to other treatments.
Start your AI query engine: With enterprises and research organizations sitting on petabytes of data, the challenge is gaining quick access to the data to deliver actionable insights.
AI query engines will change how businesses mine that data, and company-specific search engines will be able to sift through structured and unstructured data, including text, images and videos, using natural language processing and machine learning to interpret a user’s intent and provide more relevant and comprehensive results.
This will lead to more intelligent decision-making processes, improved customer experiences and enhanced productivity across industries. The continuous learning capabilities of AI query engines will create self-improving data flywheels that help applications become increasingly effective.
CHARLIE BOYLE
Vice President of DGX Platforms
Agentic AI makes high-performance inference essential for enterprises: The dawn of agentic AI will drive demand for near-instant responses from complex systems of multiple models. This will make high-performance inference just as important as high-performance training infrastructure. IT leaders will need scalable, purpose-built and optimized accelerated computing infrastructure that can keep pace with the demands of agentic AI to deliver the performance required for real-time decision-making.
Enterprises expand AI factories to process data into intelligence: Enterprise AI factories transform raw data into business intelligence. Next year, enterprises will expand these factories to leverage massive amounts of historical and synthetic data, then generate forecasts and simulations for everything from consumer behavior and supply chain optimization to financial market movements and digital twins of factories and warehouses. AI factories will become a key competitive advantage that helps early adopters anticipate and shape future scenarios, rather than just react to them.
Chill factor — liquid-cooled AI data centers: As AI workloads continue to drive growth, pioneering organizations will transition to liquid cooling to maximize performance and energy efficiency. Hyperscale cloud providers and large enterprises will lead the way, using liquid cooling in new AI data centers that house hundreds of thousands of AI accelerators, networking and software.
Enterprises will increasingly choose to deploy AI infrastructure in colocation facilities rather than build their own — in part to ease the financial burden of designing, deploying and operating intelligence manufacturing at scale. Or, they will rent capacity as needed. These deployments will help enterprises harness the latest infrastructure without needing to install and operate it themselves. This shift will accelerate broader industry adoption of liquid cooling as a mainstream solution for AI data centers.
GILAD SHAINER
Senior Vice President of Networking
Goodbye network, hello computing fabric: The term “networking” in the data center will seem dated as data center architecture transforms into an integrated compute fabric that enables thousands of accelerators to efficiently communicate with one another via scale-up and scale-out communications, spanning miles of cabling and multiple data center facilities.
This integrated compute fabric will include NVIDIA NVLink, which enables scale-up communications, as well as scale-out capabilities enabled by intelligent switches, SuperNICs and DPUs. This will help securely move data to and from accelerators and perform calculations on the fly that drastically minimize data movement. Scale-out communication across networks will be crucial to large-scale AI data center deployments — and key to getting them up and running in weeks versus months or years.
As agentic AI workloads grow — requiring communication across multiple interconnected AI models working together rather than monolithic and localized AI models — compute fabrics will be essential to delivering real-time generative AI.
Distributed AI: All data centers will become accelerated as new approaches to Ethernet design emerge that enable hundreds of thousands of GPUs to support a single workload. This will help democratize AI factory rollouts for multi-tenant generative AI clouds and enterprise AI data centers.
This breakthrough technology will also enable AI to expand quickly into enterprise platforms and simplify the buildup and management of AI clouds.
Companies will build data center resources that are more geographically dispersed — located hundreds or even thousands of miles apart — because of power limitations and the need to build closer to renewable energy sources. Scale-out communications will ensure reliable data movement over these long distances.
LINXI (JIM) FAN
Senior Research Scientist, AI Agents
Robotics will evolve more into humanoids: Robots will begin to understand arbitrary language commands. Right now, industry robots must be programmed by hand, and they don’t respond intelligently to unpredictable inputs or languages other than those programmed. Multimodal robot foundation models that incorporate vision, language and arbitrary actions will evolve this “AI brain,” as will agentic AI that allows for greater AI reasoning.
To be sure, don’t expect to immediately see intelligent robots in homes, restaurants, service areas and factories. But these use cases may be closer than you think, as governments look for solutions to aging societies and shrinking labor pools. Physical automation is going to happen gradually, in 10 years being as ubiquitous as the iPhone.
AI agents are all about inferencing: In September, OpenAI announced a new large language model trained with reinforcement learning to perform complex reasoning. OpenAI o1, dubbed Strawberry, thinks before it answers: It can produce a long internal chain of thought, correcting mistakes and breaking down tricky steps into simple ones, before responding to the user.
2025 will be the year a lot of computation begins to shift to inference at the edge. Applications will need hundreds of thousands of tokens for a single query, as small language models make one query after another in microseconds before churning out an answer.
Small models will be more energy efficient and will become increasingly important for robotics, creating humanoids and robots that can assist humans in everyday jobs and promoting mobile intelligence applications..
BOB PETTE
Vice President of Enterprise Platforms
Seeking sustainable scalability: As enterprises prepare to embrace a new generation of semiautonomous AI agents to enhance various business processes, they’ll focus on creating robust infrastructure, governance and human-like capabilities for effective large-scale deployment. At the same time, AI applications will increasingly use local processing power to enable more sophisticated AI features to run directly on workstations, including thin, lightweight laptops and compact form factors, and improve performance while reducing latency for AI-driven tasks.
Validated reference architectures, which provide guidance on appropriate hardware and software platforms, will become crucial to optimize performance and accelerate AI deployments. These architectures will serve as essential tools for organizations navigating the complex terrain of AI implementation by helping ensure that their investments align with current needs and future technological advancements.
Revolutionizing construction, engineering and design with AI: Expect to see a rise in generative AI models tailored to the construction, engineering and design industries that will boost efficiency and accelerate innovation.
In construction, agentic AI will extract meaning from massive volumes of construction data collected from onsite sensors and cameras, offering insights that lead to more efficient project timelines and budget management.
AI will evaluate reality capture data (lidar, photogrammetry and radiance fields) 24/7 and derive mission-critical insights on quality, safety and compliance — resulting in reduced errors and worksite injuries.
For engineers, predictive physics based on physics-informed neural networks will accelerate flood prediction, structural engineering and computational fluid dynamics for airflow solutions tailored to individual rooms or floors of a building — allowing for faster design iteration.
In design, retrieval-augmented generation will enable compliance early in the design phase by ensuring that information modeling for designing and constructing buildings complies with local building codes. Diffusion AI models will accelerate conceptual design and site planning by enabling architects and designers to combine keyword prompts and rough sketches to generate richly detailed conceptual images for client presentations. That will free up time to focus on research and design.
SANJA FIDLER
Vice President of AI Research
Predicting unpredictability: Expect to see more models that can learn in the everyday world, helping digital humans, robots and even autonomous cars understand chaotic and sometimes unpredictable situations, using very complex skills with little human intervention.
From the research lab to Wall Street, we’re entering a hype cycle similar to the optimism about autonomous driving 5-7 years ago. It took many years for companies like Waymo and Cruise to deliver a system that works — and it’s still not scalable because the troves of data these companies and others, including Tesla, have collected may be applicable in one region but not another.
With models introduced this year, we can now move more quickly — and with much less capital expense — to use internet-scale data to understand natural language and emulate movements by observing human and other actions. Edge applications like robots, cars and warehouse machinery will quickly learn coordination, dexterity and other skills in order to navigate, adapt and interact with the real world.
Will a robot be able to make coffee and eggs in your kitchen, and then clean up after? Not yet. But it may come sooner than you think.
Getting real: Fidelity and realism is coming to generative AI across the graphics and simulation pipeline, leading to hyperrealistic games, AI-generated movies and digital humans.
Unlike with traditional graphics, the vast majority of images will come from generated pixels instead of renderings, resulting in more natural motions and appearances. Tools that develop and iterate on contextual behaviors will result in more sophisticated games for a fraction of the cost of today’s AAA titles.
Industries adopt generative AI: Nearly every industry is poised to use AI to enhance and improve the way people live and play.
Agriculture will use AI to optimize the food chain, improving the delivery of food. For example, AI can be used to predict the greenhouse gas emissions from different crops on individual farms. These analyses can help inform design strategies that help reduce greenhouse gas in supply chains. Meanwhile, AI agents in education will personalize learning experiences, speaking in a person’s native language and asking or answering questions based on level of education in a particular subject.
As next-generation accelerators enter the marketplace, you’ll also see a lot more efficiency in delivering these generative AI applications. By improving the training and efficiency of the models in testing, businesses and startups will see better and faster returns on investment across those applications.
ANDREW FENG
Vice President of GPU Software
Accelerated data analytics offers insights with no code change: In 2025, accelerated data analytics will become mainstream for organizations grappling with ever-increasing volumes of data.
Businesses generate hundreds of petabytes of data annually, and every company is seeking ways to put it to work. To do so, many will adopt accelerated computing for data analytics.
The future lies in accelerated data analytics solutions that support “no code change” and “no configuration change,” enabling organizations to combine their existing data analytics applications with accelerated computing with minimum effort. Generative AI-empowered analytics technology will further widen the adoption of accelerated data analytics by empowering users — even those who don’t have traditional programming knowledge — to create new data analytics applications.
The seamless integration of accelerated computing, facilitated by a simplified developer experience, will help eliminate adoption barriers and allow organizations to harness their unique data for new AI applications and richer business intelligence.
NADER KHALIL
Director of Developer Technology
The startup workforce: If you haven’t heard much about prompt engineers or AI personality designers, you will in 2025. As businesses embrace AI to increase productivity, expect to see new categories of essential workers for both startups and enterprises that blend new and existing skills.
A prompt engineer designs and refines precise text strings that optimize AI training and produce desired outcomes based on the creation, testing and iteration of prompt designs for chatbots and agentic AI. The demand for prompt engineers will extend beyond tech companies to sectors like legal, customer support and publishing. As AI agents proliferate, businesses and startups will increasingly lean in to AI personality designers to enhance agents with unique personalities.
Just as the rise of computers spawned job titles like computer scientists, data scientists and machine learning engineers, AI will create different types of work, expanding opportunities for people with strong analytical skills and natural language processing abilities.
Understanding employee efficiency: Startups incorporating AI into their practices increasingly will add revenue per employee (RPE) to their lexicon when talking to investors and business partners.
Instead of a “growth at all costs” mentality, AI supplementation of the workforce will allow startup owners to home in on how hiring each new employee helps everyone else in the business generate more revenue. In the world of startups, RPE fits into discussions about the return on investment in AI and the challenges of filling roles in competition against big enterprises and tech companies.
Read More