Learn how retailers are benefiting from Cloud’s gen AI agents, AI-powered search and other AI technology.Read More
AI Gets Real for Retailers: 9 Out of 10 Retailers Now Adopting or Piloting AI, Latest NVIDIA Survey Finds
Artificial intelligence is rapidly becoming the cornerstone of innovation in the retail and consumer packaged goods (CPG) industries.
Forward-thinking companies are using AI to reimagine their entire business models, from in-store experiences to omnichannel digital platforms, including ecommerce, mobile and social channels. This technological wave is simultaneously transforming advertising and marketing, customer engagement and supply chain operations. By harnessing AI, retailers and CPG brands are not just adapting to change — they’re actively shaping the future of commerce.
NVIDIA’s second annual “State of AI in Retail and CPG” survey provides insights into the adoption, investment and impact of AI, including generative AI; the top use cases and challenges; and a special section this year examining the use of AI in the supply chain. It’s an in-depth look at the current ecosystem of AI in retail and CPG, and how it’s transforming the industries.
Drawn from hundreds of responses from industry professionals, key highlights of the survey show:
- 89% of respondents said they are either actively using AI in their operations or assessing AI projects, including trials and pilots (up from 82% in 2023)
- 87% said AI had a positive impact on increasing annual revenue
- 94% said AI has helped reduce annual operational costs
- 97% said spending on AI would increase in the next fiscal year
Generative AI in Retail Takes Center Stage
Generative AI has found a strong foothold in retail and CPG, with over 80% of companies either using or piloting projects. Companies are harnessing the technology, especially for content generation in marketing and advertising, as well as customer analysis and analytics.
Consistent with last year’s survey, over 50% of retailers believe that generative AI is a strategic technology that will be a differentiator in the market.
The top use cases for generative AI in retail include:
- Content generation for marketing (60%)
- Predictive analytics (44%)
- Personalized marketing and advertising (42%)
- Customer analysis and segmentation (41%)
- Digital shopping assistants or copilots (40%)
While some concerns about generative AI exist, specifically around data privacy, security and implementation costs, these concerns haven’t dampened retailers’ enthusiasm, with 93% of respondents saying they still plan to increase generative AI investment next year.
AI Across the Retail Landscape
AI use cases have proliferated across nearly every line of business in retail, with over 50% of retailers using AI in more than six different use cases throughout their operations.
In physical stores, the top three use cases are inventory management, analytics and insights, and adaptive advertising. For digital retail, they’re marketing and advertising content creation, and hyperpersonalized recommendations. And in the back office, the top use cases are customer analysis and predictive analytics.
AI has made a significant impact in retail and CPG, with improved insights and decision-making (43%) and enhanced employee productivity (42%) being listed as top benefits among survey respondents.
The most common AI challenge retailers faced in 2024 was a lack of easy to understand and explainable AI tools, underscoring a greater need for software and solutions — specifically around generative AI and AI agents — to enter the market to make it easier for companies to use AI solutions and understand how they work.
AI in the Supply Chain
Managing the supply chain has always been a challenge for retail and CPG companies, but it’s become increasingly difficult over the last several years due to tumultuous global events and shifting consumer preferences. Companies are feeling the pressure, with 59% of respondents saying that their supply chain challenges have grown in the last year.
Increasingly, companies are turning to AI to help address these challenges, and the impact of these AI solutions is starting to show up in results.
- 58% said AI is helping to improve operational efficiency and throughput.
- 45% are using AI to reduce supply chain costs.
- 42% are employing AI to meet shifting customer expectations.
Investment in AI for supply chain management is set to grow, with 82% of companies planning to increase spending in the next fiscal year.
As the retail and CPG industries continue to embrace the power of AI, the findings from the latest survey underscore a pivotal shift in how businesses operate in a complex new landscape. Leading companies are harnessing advanced technologies — such as AI agents and physical AI — to enhance efficiency and drive revenue, as well as to position themselves as leaders in innovation, helping redefine the future of retail and CPG.
Download the “State of AI in Retail and CPG: 2025 Trends” report for in-depth results and insights.
Explore NVIDIA’s AI solutions and enterprise-level platforms for retail.
Fingerprinting Codes Meet Geometry: Improved Lower Bounds for Private Query Release and Adaptive Data Analysis
Fingerprinting codes are a crucial tool for proving lower bounds in differential privacy. They have been used to prove tight lower bounds for several fundamental questions, especially in the “low accuracy” regime. Unlike reconstruction/discrepancy approaches however, they are more suited for proving worst-case lower bounds, for query sets that arise naturally from the fingerprinting codes construction. In this work, we propose a general framework for proving fingerprinting type lower bounds, that allows us to tailor the technique to the geometry of the query set.
Our approach allows us to…Apple Machine Learning Research
Build an Amazon Bedrock based digital lending solution on AWS
Digital lending is a critical business enabler for banks and financial institutions. Customers apply for a loan online after completing the know your customer (KYC) process. A typical digital lending process involves various activities, such as user onboarding (including steps to verify the user through KYC), credit verification, risk verification, credit underwriting, and loan sanctioning. Currently, some of these activities are done manually, leading to delays in loan sanctioning and impacting the customer experience.
In India, the KYC verification usually involves identity verification through identification documents for Indian citizens, such as a PAN card or Aadhar card, address verification, and income verification. Credit checks in India are normally done using the PAN number of a customer. The ideal way to address these challenges is to automate them to the extent possible.
The digital lending solution primarily needs orchestration of a sequence of steps and other features such as natural language understanding, image analysis, real-time credit checks, and notifications. You can seamlessly build automation around these features using Amazon Bedrock Agents. Amazon Bedrock is a fully managed service that offers a choice of high-performing foundation models (FMs) from leading AI companies such as AI21 Labs, Anthropic, Cohere, Meta, Mistral AI, Stability AI, and Amazon through a single API, along with a broad set of capabilities to build generative AI applications with security, privacy, and responsible AI. With Amazon Bedrock Agents, you can orchestrate multi-step processes and integrate with enterprise data using natural language instructions.
In this post, we propose a solution using DigitalDhan, a generative AI-based solution to automate customer onboarding and digital lending. The proposed solution uses Amazon Bedrock Agents to automate services related to KYC verification, credit and risk assessment, and notification. Financial institutions can use this solution to help automate the customer onboarding, KYC verification, credit decisioning, credit underwriting, and notification processes. This post demonstrates how you can gain a competitive advantage using Amazon Bedrock Agents based automation of a complex business process.
Why generative AI is best suited for assistants that support customer journeys
Traditional AI assistants that use rules-based navigation or natural language processing (NLP) based guidance fall short when handling the nuances of complex human conversations. For instance, in a real-world customer conversation, the customer might provide inadequate information (for example, missing documents), ask random or unrelated questions that aren’t part of the predefined flow (for example, asking for loan pre-payment options while verifying the identity documents), natural language inputs (such as using various currency modes, such as representing twenty thousand as “20K” or “20000” or “20,000”). Additionally, rules-based assistants don’t provide additional reasoning and explanations (such as why a loan was denied). Some of the rigid and linear flow-related rules either force customers to start the process over again or the conversation requires human assistance.
Generative AI assistants excel at handling these challenges. With well-crafted instructions and prompts, a generative AI-based assistant can ask for missing details, converse in human-like language, and handle errors gracefully while explaining the reasoning for their actions when required. You can add guardrails to make sure that these assistants don’t deviate from the main topic and provide flexible navigation options that account for real-world complexities. Context-aware assistants also enhance customer engagement by flexibly responding to the various off-the-flow customer queries.
Solution overview
DigitalDhan, the proposed digital lending solution, is powered by Amazon Bedrock Agents. They have developed a solution that fully automates the customer onboarding, KYC verification, and credit underwriting process. The DigitalDhan service provides the following features:
- Customers can understand the step-by-step loan process and the documents required through the solution
- Customers can upload KYC documents such as PAN and Aadhar, which DigitalDhan verifies through automated workflows
- DigitalDhan fully automates the credit underwriting and loan application process
- DigitalDhan notifies the customer about the loan application through email
We have modeled the digital lending process close to a real-world scenario. The high-level steps of the DigitalDhan solution are shown in the following figure.
The key business process steps are:
- The loan applicant initiates the loan application flow by accessing the DigitalDhan solution.
- The loan applicant begins the loan application journey. Sample prompts for the loan application include:
- “What is the process to apply for loan?”
- “I would like to apply for loan.”
- “My name is Adarsh Kumar. PAN is ABCD1234 and email is john_doe@example.org. I need a loan for 150000.”
- The applicant uploads their PAN card.
- The applicant uploads their Aadhar card.
- The DigitalDhan processes each of the natural language prompts. As part of the document verification process, the solution extracts the key details from the uploaded PAN and Aadhar cards such as name, address, date of birth, and so on. The solution then identifies whether the user is an existing customer using the PAN.
- If the user is an existing customer, the solution gets the internal risk score for the customer.
- If the user is a new customer, the solution gets the credit score based on the PAN details.
- The solution uses the internal risk score for an existing customer to check for credit worthiness.
- The solution uses the external credit score for a new customer to check for credit worthiness.
- The credit underwriting process involves credit decisioning based on the credit score and risk score, and calculates the final loan amount for the approved customer.
- The loan application details along with the decision are sent to the customer through email.
Technical solution architecture
The solution primarily uses Amazon Bedrock Agents (to orchestrate the multi-step process), Amazon Textract (to extract data from the PAN and Aadhar cards), and Amazon Comprehend (to identify the entities from the PAN and Aadhar card). The solution architecture is shown in the following figure.
The key solution components of the DigitalDhan solution architecture are:
- A user begins the onboarding process with the DigitalDhan application. They provide various documents (including PAN and Aadhar) and a loan amount as part of the KYC
- After the documents are uploaded, they’re automatically processed using various artificial intelligence and machine learning (AI/ML) services.
- Amazon Textract is used to extract text information from the uploaded documents.
- Amazon Comprehend is used to identify entities such as PAN and Aadhar.
- The credit underwriting flow is powered by Amazon Bedrock Agents.
- The knowledge base contains loan-related documents to respond to loan-related queries.
- The loan handler AWS Lambda function uses the information in the KYC documents to check the credit score and internal risk score. After the credit checks are complete, the function calculates the loan eligibility and processes the loan application.
- The notification Lambda function emails information about the loan application to the customer.
- The Lambda function can be integrated with external credit APIs.
- Amazon Simple Email Service (Amazon SES) is used to notify customers of the status of their loan application.
- The events are logged using Amazon CloudWatch.
Amazon Bedrock Agents deep dive
Because we used Amazon Bedrock Agents heavily in the DigitalDhan solution, let’s look at the overall functioning of Amazon Bedrock Agents. The flow of the various components of Amazon Bedrock Agents is shown in the following figure.
The Amazon Bedrock agents break each task into subtasks, determine the right sequence, and perform actions and knowledge searches. The detailed steps are:
- Processing the loan application is the primary task performed by the Amazon Bedrock agents in the DigitalDhan solution.
- The Amazon Bedrock agents use the user prompts, conversation history, knowledge base, instructions, and action groups to orchestrate the sequence of steps related to loan processing. The Amazon Bedrock agent takes natural language prompts as inputs. The following are the instructions given to the agent:
- We configured the agent preprocessing and orchestration instructions to validate and perform the steps in a predefined sequence. The few-shot examples specified during the agent instructions boost the accuracy of the agent performance. Based on the instructions and the API descriptions, the Amazon Bedrock agent creates a logical sequence of steps to complete an action. In the DigitalDhan example, instructions are specified such that the Amazon Bedrock agent creates the following sequence:
- Greet the customer.
- Collect the customer’s name, email, PAN, and loan amount.
- Ask for the PAN card and Aadhar card to read and verify the PAN and Aadhar number.
- Categorize the customer as an existing or new customer based on the verified PAN.
- For an existing customer, calculate the customer internal risk score.
- For a new customer, get the external credit score.
- Use the internal risk score (for existing customers) or credit score (for external customers) for credit underwriting. If the internal risk score is less than 300 or if the credit score is more than 700, sanction the loan amount.
- Email the credit decision to the customer’s email address.
- Action groups define the APIs for performing actions such as creating the loan, checking the user, fetching the risk score, and so on. We described each of the APIs in the OpenAPI schema, which the agent uses to select the most appropriate API to perform the action. Lambda is associated with the action group. The following code is an example of the
create_loan
API. The Amazon Bedrock agent uses the description for thecreate_loan
API while performing the action. The API schema also specifiescustomerName
,address
,loanAmt
,PAN
, andriskScore
as required elements for the APIs. Therefore, the corresponding APIs read the PAN number for the customer (verify_pan_card
API), calculate the risk score for the customer (fetch_risk_score
API), and identify the customer’s name and address (verify_aadhar_card
API) before calling thecreate_loan
API.
Build AI-powered malware analysis using Amazon Bedrock with Deep Instinct
This post is co-written with Yaniv Avolov, Tal Furman and Maor Ashkenazi from Deep Instinct.
Deep Instinct is a cybersecurity company that offers a state-of-the-art, comprehensive zero-day data security solution—Data Security X (DSX), for safeguarding your data repositories across the cloud, applications, network attached storage (NAS), and endpoints. DSX provides unmatched prevention and explainability by using a powerful combination of deep learning-based DSX Brain and generative AI DSX Companion to protect systems from known and unknown malware and ransomware in real-time.
Using deep neural networks (DNNs), Deep Instinct analyzes threats with unmatched accuracy, adapting to identify new and unknown risks that traditional methods might miss. This approach significantly reduces false positives and enables unparalleled threat detection rates, making it popular among large enterprises and critical infrastructure sectors such as finance, healthcare, and government.
In this post, we explore how Deep Instinct’s generative AI-powered malware analysis tool, DIANNA, uses Amazon Bedrock to revolutionize cybersecurity by providing rapid, in-depth analysis of known and unknown threats, enhancing the capabilities of AWS System and Organization Controls (SOC) teams and addressing key challenges in the evolving threat landscape.
Main challenges for SecOps
There are two main challenges for SecOps:
- The growing threat landscape – With a rapidly evolving threat landscape, SOC teams are becoming overwhelmed with a continuous increase of security alerts that require investigation. This situation hampers proactive threat hunting and exacerbates team burnout. Most importantly, the surge in alert storms increases the risk of missing critical alerts. A solution is needed that provides the explainability necessary to allow SOC teams to perform quick risk assessments regarding the nature of incidents and make informed decisions.
- The challenges of malware analysis – Malware analysis has become an increasingly critical and complex field. The challenge of zero-day attacks lies in the limited information about why a file was blocked and classified as malicious. Threat analysts often spend considerable time assessing whether it was a genuine exploit or a false positive.
Let’s explore some of the key challenges that make malware analysis demanding:
- Identifying malware – Modern malware has become incredibly sophisticated in its ability to disguise itself. It often mimics legitimate software, making it challenging for analysts to distinguish between benign and malicious code. Some malware can even disable security tools or evade scanners, further obfuscating detection.
- Preventing zero-day threats – The rise of zero-day threats, which have no known signatures, adds another layer of difficulty. Identifying unknown malware is crucial, because failure can lead to severe security breaches and potentially incapacitate organizations.
- Information overload – The powerful malware analysis tools currently available can be both beneficial and detrimental. Although they offer high explainability, they can also produce an overwhelming amount of data, forcing analysts to sift through a digital haystack to find indicators of malicious activity, increasing the possibility of analysts overlooking critical compromises.
- Connecting the dots – Malware often consists of multiple components interacting in complex ways. Not only do analysts need to identify the individual components, but they also need to understand how they interact. This process is like assembling a jigsaw puzzle to form a complete picture of the malware’s capabilities and intentions, with pieces constantly changing shape.
- Keeping up with cybercriminals – The world of cybercrime is fluid, with bad actors relentlessly developing new techniques and exploiting newly emerging vulnerabilities, leaving organizations struggling to keep up. The time window between the discovery of a vulnerability and its exploitation in the wild is narrowing, putting pressure on analysts to work faster and more efficiently. This rapid evolution means that malware analysts must constantly update their skill set and tools to stay one step ahead of the cybercriminals.
- Racing against the clock – In malware analysis, time is of the essence. Malicious software can spread rapidly across networks, causing significant damage in a matter of minutes, often before the organization realizes an exploit has occurred. Analysts face the pressure of conducting thorough examinations while also providing timely insights to prevent or mitigate exploits.
DIANNA, the DSX Companion
There is a critical need for malware analysis tools that can provide precise, real-time, in-depth malware analysis for both known and unknown threats, supporting SecOps efforts. Deep Instinct, recognizing this need, has developed DIANNA (Deep Instinct’s Artificial Neural Network Assistant), the DSX Companion. DIANNA is a groundbreaking malware analysis tool powered by generative AI to tackle real-world issues, using Amazon Bedrock as its large language model (LLM) infrastructure. It offers on-demand features that provide flexible and scalable AI capabilities tailored to the unique needs of each client. Amazon Bedrock is a fully managed service that grants access to high-performance foundation models (FMs) from top AI companies through a unified API. By concentrating our generative AI models on specific artifacts, we can deliver comprehensive yet focused responses to address this gap effectively.
DIANNA is a sophisticated malware analysis tool that acts as a virtual team of malware analysts and incident response experts. It enables organizations to shift strategically toward zero-day data security by integrating with Deep Instinct’s deep learning capabilities for a more intuitive and effective defense against threats.
DIANNA’s unique approach
Current cybersecurity solutions use generative AI to summarize data from existing sources, but this approach is limited to retrospective analysis with limited context. DIANNA enhances this by integrating the collective expertise of numerous cybersecurity professionals within the LLM, enabling in-depth malware analysis of unknown files and accurate identification of malicious intent.
DIANNA’s unique approach to malware analysis sets it apart from other cybersecurity solutions. Unlike traditional methods that rely solely on retrospective analysis of existing data, DIANNA harnesses generative AI to empower itself with the collective knowledge of countless cybersecurity experts, sources, blog posts, papers, threat intelligence reputation engines, and chats. This extensive knowledge base is effectively embedded within the LLM, allowing DIANNA to delve deep into unknown files and uncover intricate connections that would otherwise go undetected.
At the heart of this process are DIANNA’s advanced translation engines, which transform complex binary code into natural language that LLMs can understand and analyze. This unique approach bridges the gap between raw code and human-readable insights, enabling DIANNA to provide clear, contextual explanations of a file’s intent, malicious aspects, and potential system impact. By translating the intricacies of code into accessible language, DIANNA addresses the challenge of information overload, distilling vast amounts of data into concise, actionable intelligence.
This translation capability is key for linking between different components of complex malware. It allows DIANNA to identify relationships and interactions between various parts of the code, offering a holistic view of the threat landscape. By piecing together these components, DIANNA can construct a comprehensive picture of the malware’s capabilities and intentions, even when faced with sophisticated threats. DIANNA doesn’t stop at simple code analysis—it goes deeper. It provides insights into why unknown events are malicious, streamlining what is often a lengthy process. This level of understanding allows SOC teams to focus on the threats that matter most.
Solution overview
DIANNA’s integration with Amazon Bedrock allows us to harness the power of state-of-the-art language models while maintaining agility to adapt to evolving client requirements and security considerations. DIANNA benefits from the robust features of Amazon Bedrock, including seamless scaling, enterprise-grade security, and the ability to fine-tune models for specific use cases.
The integration offers the following benefits:
- Accelerated development with Amazon Bedrock – The fast-paced evolution of the threat landscape necessitates equally responsive cybersecurity solutions. DIANNA’s collaboration with Amazon Bedrock has played a crucial role in optimizing our development process and speeding up the delivery of innovative capabilities. The service’s versatility has enabled us to experiment with different FMs, exploring their strengths and weaknesses in various tasks. This experimentation has led to significant advancements in DIANNA’s ability to understand and explain complex malware behaviors. We have also benefited from the following features:
- Fine-tuning – Alongside its core functionalities, Amazon Bedrock provides a range of ready-to-use features for customizing the solution. One such feature is model fine-tuning, which allows you to train FMs on proprietary data to enhance your performance in specific domains. For example, organizations can fine-tune an LLM-based malware analysis tool to recognize industry-specific jargon or detect threats associated with particular vulnerabilities.
- Retrieval Augmented Generation – Another valuable feature is the use of Retrieval Augmented Generation (RAG), enabling access to and the incorporation of relevant information from external sources, such as knowledge bases or threat intelligence feeds. This enhances the model’s ability to provide contextually accurate and informative responses, improving the overall effectiveness of malware analysis.
- A landscape for innovation and comparison – Amazon Bedrock has also served as a valuable landscape for conducting LLM-related research and comparisons.
- Seamless integration, scalability, and customization – Integrating Amazon Bedrock into DIANNA’s architecture was a straightforward process. The user-friendly Amazon Bedrock API and well-documented facilitated seamless integration with our existing infrastructure. Furthermore, the service’s on-demand nature allows us to scale our AI capabilities up or down based on customer demand. This flexibility makes sure that DIANNA can handle fluctuating workloads without compromising performance.
- Prioritizing data security and compliance – Data security and compliance are paramount in the cybersecurity domain. Amazon Bedrock offers enterprise-grade security features that provide us with the confidence to handle sensitive customer data. The service’s adherence to industry-leading security standards, coupled with the extensive experience of AWS in data protection, makes sure DIANNA meets the highest regulatory requirements such as GDPR. By using Amazon Bedrock, we can offer our customers a solution that not only protects their assets, but also demonstrates our commitment to data privacy and security.
By combining Deep Instinct’s proprietary prevention algorithms with the advanced language processing capabilities of Amazon Bedrock, DIANNA offers a unique solution that not only identifies and analyzes threats with high accuracy, but also communicates its findings in clear, actionable language. This synergy between Deep Instinct’s expertise in cybersecurity and the leading AI infrastructure of Amazon positions DIANNA at the forefront of AI-driven malware analysis and threat prevention.
The following diagram illustrates DIANNA’s architecture.
Evaluating DIANNA’s malware analysis
In our task, the input is a malware sample, and the output is a comprehensive, in-depth report on the behaviors and intents of the file. However, generating ground truth data is particularly challenging. The behaviors and intents of malicious files aren’t readily available in standard datasets and require expert malware analysts for accurate reporting. Therefore, we needed a custom evaluation approach.
We focused our evaluation on two core dimensions:
- Technical features – This dimension focuses on objective, measurable capabilities. We used programmable metrics to assess how well DIANNA handled key technical aspects, such as extracting indicators of compromise (IOCs), detecting critical keywords, and processing the length and structure of threat reports. These metrics allowed us to quantitatively assess the model’s basic analysis capabilities.
- In-depth semantics – Because DIANNA is expected to generate complex, human-readable reports on malware behavior, we relied on domain experts (malware analysts) to assess the quality of the analysis. The reports were evaluated based on the following:
- Depth of information – Whether DIANNA provided a detailed understanding of the malware’s behavior and techniques.
- Accuracy – How well the analysis aligned with the true behaviors of the malware.
- Clarity and structure – Evaluating the organization of the report, making sure the output was clear and comprehensible for security teams.
Because human evaluation is labor-intensive, fine-tuning the key components (the model itself, the prompts, and the translation engines) involved iterative feedback loops. Small adjustments in a component led to significant variations in the output, requiring repeated validations by human experts. The meticulous nature of this process, combined with the continuous need for scaling, has subsequently led to the development of the auto-evaluation capability.
Fine-tuning process and human validation
The fine-tuning and validation process consisted of the following steps:
- Gathering a malware dataset – To cover the breadth of malware techniques, families, and threat types, we collected a large dataset of malware samples, each with technical metadata.
- Splitting the dataset – The data was split into subsets for training, validation, and evaluation. Validation data was continually used to test how well DIANNA adapted after each key component update.
- Human expert evaluation – Each time we fine-tuned DIANNA’s model, prompts, and translation mechanisms, human malware analysts reviewed a portion of the validation data. This made sure improvements or degradations in the quality of the reports were identified early. Because DIANNA’s outputs are highly sensitive to even minor changes, each update required a full reevaluation by human experts to verify whether the response quality was improved or degraded.
- Final evaluation on a broader dataset – After sufficient tuning based on the validation data, we applied DIANNA to a large evaluation set. Here, we gathered comprehensive statistics on its performance to confirm improvements in report quality, correctness, and overall technical coverage.
Automation of evaluation
To make this process more scalable and efficient, we introduced an automatic evaluation phase. We trained a language model specifically designed to critique DIANNA’s outputs, providing a level of automation in assessing how well DIANNA was generating reports. This critique model acted as an internal judge, allowing for continuous, rapid feedback on incremental changes during fine-tuning. This enabled us to make small adjustments across DIANNA’s three core components (model, prompts, and translation engines) while receiving real-time evaluations of the impact of those changes.
This automated critique model enhanced our ability to test and refine DIANNA without having to rely solely on the time-consuming manual feedback loop from human experts. It provided a consistent, reliable measure of performance and allowed us to quickly identify which model adjustments led to meaningful improvements in DIANNA’s analysis.
Advanced integration and proactive analysis
DIANNA is integrated with Deep Instinct’s proprietary deep learning algorithms, enabling it to detect zero-day threats with high accuracy and a low false positive rate. This proactive approach helps security teams quickly identify unknown threats, reduce false positives, and allocate resources more effectively. Additionally, it streamlines investigations, minimizes cross-tool efforts, and automates repetitive tasks, making the decision-making process clearer and faster. This ultimately helps organizations strengthen their security posture and significantly reduce the mean time to triage.
This analysis offers the following key features and benefits:
- Performs on-the-fly file scans, allowing for immediate assessment without prior setup or delays
- Generates comprehensive malware analysis reports for a variety of file types in seconds, making sure users receive timely information about potential threats
- Streamlines the entire file analysis process, making it more efficient and user-friendly, thereby reducing the time and effort required for thorough evaluations
- Supports a wide range of common file formats, including Office documents, Windows executable files, script files, and Windows shortcut files (.lnk), providing compatibility with various types of data
- Offers in-depth contextual analysis, malicious file triage, and actionable insights, greatly enhancing the efficiency of investigations into potentially harmful files
- Empowers SOC teams to make well-informed decisions without relying on manual malware analysis by providing clear and concise insights into the behavior of malicious files
- Alleviates the need to upload files to external sandboxes or VirusTotal, thereby enhancing security and privacy while facilitating quicker analysis
Explainability and insights into better decision-making for SOC teams
DIANNA stands out by offering clear insights into why unknown events are flagged as malicious. Traditional AI tools often rely on lengthy, retrospective analyses that can take hours or even days to generate, and often lead to vague conclusions. DIANNA dives deeper, understanding the intent behind the code and providing detailed explanations of its potential impact. This clarity allows SOC teams to prioritize the threats that matter most.
Example scenario of DIANNA in action
In this section, we explore some DIANNA use cases.
For example, DIANNA can perform investigations on malicious files.
The following screenshot is an example of a Windows executable file analysis.
The following screenshot is an example of an Office file analysis.
You can also quickly triage incidents with enriched data on file analysis provided by DIANNA. The following screenshot is an example using Windows shortcut files (LNK) analysis.
The following screenshot is an example with a script file (JavaScript) analysis.
The following figure presents a before and after comparison of the analysis process.
Additionally, a key advantage of DIANNA is its ability to provide explainability by correlating and summarizing the intentions of malicious files in a detailed narrative. This is especially valuable for zero-day and unknown threats that aren’t yet recognized, making investigations challenging when starting from scratch without any clues.
Potential advancements in AI-driven cybersecurity
AI capabilities are enhancing daily operations, but adversaries are also using AI to create sophisticated malicious events and advanced persistent threats. This leaves organizations, particularly SOC and cybersecurity teams, dealing with more complex incidents.
Although detection controls are useful, they often require significant resources and can be ineffective on their own. In contrast, using AI engines for prevention controls—such as a high-efficacy deep learning engine—can lower the total cost of ownership and help SOC analysts streamline their tasks.
Conclusion
The Deep Instinct solution can predict and prevent known, unknown, and zero-day threats in under 20 milliseconds—750 times faster than the fastest ransomware encryption. This makes it essential for security stacks, offering comprehensive protection in hybrid environments.
DIANNA provides expert malware analysis and explainability for zero-day attacks and can enhance the incident response process for the SOC team, allowing them to efficiently tackle and investigate unknown threats with minimal time investment. This, in turn, reduces the resources and expenses that Chief Information Security Officers (CISOs) need to allocate, enabling them to invest in more valuable initiatives.
DIANNA’s collaboration with Amazon Bedrock accelerated development, enabled innovation through experimentation with various FMs, and facilitated seamless integration, scalability, and data security. The rise of AI-based threats is becoming more pronounced. As a result, defenders must outpace increasingly sophisticated bad actors by moving beyond traditional AI tools and embracing advanced AI, especially deep learning. Companies, vendors, and cybersecurity professionals must consider this shift to effectively combat the growing prevalence of AI-driven exploits.
About the Authors
Tzahi Mizrahi is a Solutions Architect at Amazon Web Services with experience in cloud architecture and software development. His expertise includes designing scalable systems, implementing DevOps best practices, and optimizing cloud infrastructure for enterprise applications. He has a proven track record of helping organizations modernize their technology stack and improve operational efficiency. In his free time, he enjoys music and plays the guitar.
Tal Panchek is a Senior Business Development Manager for Artificial Intelligence and Machine Learning with Amazon Web Services. As a BD Specialist, he is responsible for growing adoption, utilization, and revenue for AWS services. He gathers customer and industry needs and partner with AWS product teams to innovate, develop, and deliver AWS solutions.
Yaniv Avolov is a Principal Product Manager at Deep Instinct, bringing a wealth of experience in the cybersecurity field. He focuses on defining and designing cybersecurity solutions that leverage AIML, including deep learning and large language models, to address customer needs. In addition, he leads the endpoint security solution, ensuring it is robust and effective against emerging threats. In his free time, he enjoys cooking, reading, playing basketball, and traveling.
Tal Furman is a Data Science and Deep Learning Director at Deep Instinct. His focused on applying Machine Learning and Deep Learning algorithms to tackle real world challenges, and takes pride in leading people and technology to shape the future of cyber security. In his free time, Tal enjoys running, swimming, reading and playfully trolling his kids and dogs.
Maor Ashkenazi is a deep learning research team lead at Deep Instinct, and a PhD candidate at Ben-Gurion University of the Negev. He has extensive experience in deep learning, neural network optimization, computer vision, and cyber security. In his spare time, he enjoys traveling, cooking, practicing mixology and learning new things.
Email your conversations from Amazon Q
As organizations navigate the complexities of the digital realm, generative AI has emerged as a transformative force, empowering enterprises to enhance productivity, streamline workflows, and drive innovation. To maximize the value of insights generated by generative AI, it is crucial to provide simple ways for users to preserve and share these insights using commonly used tools such as email.
Amazon Q Business is a generative AI-powered assistant that can answer questions, provide summaries, generate content, and securely complete tasks based on data and information in your enterprise systems. It is redefining the way businesses approach data-driven decision-making, content generation, and secure task management. By using the custom plugin capability of Amazon Q Business, you can extend its functionality to support sending emails directly from Amazon Q applications, allowing you to store and share the valuable insights gleaned from your conversations with this powerful AI assistant.
Amazon Simple Email Service (Amazon SES) is an email service provider that provides a simple, cost-effective way for you to send and receive email using your own email addresses and domains. Amazon SES offers many email tools, including email sender configuration options, email deliverability tools, flexible email deployment options, sender and identity management, email security, email sending statistics, email reputation dashboard, and inbound email services.
This post explores how you can integrate Amazon Q Business with Amazon SES to email conversations to specified email addresses.
Solution overview
The following diagram illustrates the solution architecture.
The workflow includes the following steps:
- Create an Amazon Q Business application with an Amazon Simple Storage Service (Amazon S3) data source. Amazon Q uses Retrieval Augmented Generation (RAG) to answer user questions.
- Configure an AWS IAM Identity Center instance for your Amazon Q Business application environment with users and groups added. Amazon Q Business supports both organization- and account-level IAM Identity Center instances.
- Create a custom plugin that invokes an OpenAPI schema of the Amazon API Gateway This API sends emails to the users.
- Store OAuth information in AWS Secrets Manager and provide the secret information to the plugin.
- Provide AWS Identity Manager and Access Management (IAM) roles to access the secrets in Secrets Manager.
- The custom plugin takes the user to an Amazon Cognito sign-in page. The user provides credentials to log in. After authentication, the user session is stored in the Amazon Q Business application for subsequent API calls.
- Post-authentication, the custom plugin will pass the token to API Gateway to invoke the API.
- You can help secure your API Gateway REST API from common web exploits, such as SQL injection and cross-site scripting (XSS) attacks, using AWS WAF.
- AWS Lambda hosted in Amazon Virtual Private Cloud (Amazon VPC) internally calls the Amazon SES SDK.
- Lambda uses AWS Identity and Access Management (IAM) permissions to make an SDK call to Amazon SES.
- Amazon SES sends an email using SMTP to verified emails provided by the user.
In the following sections, we walk through the steps to deploy and test the solution. This solution is supported only in the us-east-1
AWS Region.
Prerequisites
Complete the following prerequisites:
- Have a valid AWS account.
- Enable an IAM Identity Center instance and capture the Amazon Resource Name (ARN) of the IAM Identity Center instance from the settings page.
- Add users and groups to IAM Identity Center.
- Have an IAM role in the account that has sufficient permissions to create the necessary resources. If you have administrator access to the account, no action is necessary.
- Enable Amazon CloudWatch Logs for API Gateway. For more information, see How do I turn on CloudWatch Logs to troubleshoot my API Gateway REST API or WebSocket API?
- Have two email addresses to send and receive emails that you can verify using the link sent to you. Do not use existing verified identities in Amazon SES for these email addresses. Otherwise, the AWS CloudFormation template will fail.
- Have an Amazon Q Business Pro subscription to create Amazon Q apps.
- Have the service-linked IAM role
AWSServiceRoleForQBusiness
. If you don’t have one, create it with the amazonaws.com service name. - Enable AWS CloudTrail logging for operational and risk auditing. For instructions, see Creating a trail for your AWS account.
- Enable budget policy notifications to help protect from unwanted billing.
Deploy the solution resources
In this step, we use a CloudFormation template to deploy a Lambda function, configure the REST API, and create identities. Complete the following steps:
- Open the AWS CloudFormation console in the
us-east-1
- Choose Create stack.
- Download the CloudFormation template and upload it in the Specify template
- Choose Next.
- For Stack name, enter a name (for example,
QIntegrationWithSES
). - In the Parameters section, provide the following:
- For IDCInstanceArn, enter your IAM Identity Center instance ARN.
- For LambdaName, enter the name of your Lambda function.
- For Fromemailaddress, enter the address to send email.
- For Toemailaddress, enter the address to receive email.
- Choose Next.
- Keep the other values as default and select I acknowledge that AWS CloudFormation might create IAM resources in the Capabilities
- Choose Submit to create the CloudFormation stack.
- After the successful deployment of the stack, on the Outputs tab, make a note of the value for
apiGatewayInvokeURL
. You will need this later to create a custom plugin.
Verification emails will be sent to the Toemailaddress
and Fromemailaddress
values provided as input to the CloudFormation template.
- Verify the newly created email identities using the link in the email.
This post doesn’t cover auto scaling of Lambda functions. For more information about how to integrate Lambda with Application Auto Scaling, see AWS Lambda and Application Auto Scaling.
To configure AWS WAF on API Gateway, refer to Use AWS WAF to protect your REST APIs in API Gateway.
This is sample code, for non-production usage. You should work with your security and legal teams to meet your organizational security, regulatory, and compliance requirements before deployment.
Create Amazon Cognito users
This solution uses Amazon Cognito to authorize users to make a call to API Gateway. The CloudFormation template creates a new Amazon Cognito user pool.
Complete the following steps to create a user in the newly created user pool and capture information about the user pool:
- On the AWS CloudFormation console, navigate to the stack you created.
- On the Resources tab, choose the link next to the physical ID for
CognitoUserPool
.
- On the Amazon Cognito console, choose User management and users in the navigation pane.
- Choose Create user.
- Enter an email address and password of your choice, then choose Create user.
- In the navigation pane, choose Applications and app clients.
- Capture the client ID and client secret. You will need these later during custom plugin development.
- On the Login pages tab, copy the values for Allowed callback URLs. You will need these later during custom plugin development.
- In the navigation pane, choose Branding.
- Capture the Amazon Cognito domain. You will need this information to update OpenAPI specifications.
Upload documents to Amazon S3
This solution uses the fully managed Amazon S3 data source to seamlessly power a RAG workflow, eliminating the need for custom integration and data flow management.
For this post, we use sample articles to upload to Amazon S3. Complete the following steps:
- On the AWS CloudFormation console, navigate to the stack you created.
- On the Resources tab, choose the link for the physical ID of
AmazonQDataSourceBucket
.
- Upload the sample articles file to the S3 bucket. For instructions, see Uploading objects.
Add users to the Amazon Q Business application
Complete the following steps to add users to the newly created Amazon Q business application:
- On the Amazon Q Business console, choose Applications in the navigation pane.
- Choose the application you created using the CloudFormation template.
- Under User access, choose Manage user access.
- On the Manage access and subscriptions page, choose Add groups and users.
- Select Assign existing users and groups, then choose Next.
- Search for your IAM Identity Center user group.
- Choose the group and choose Assign to add the group and its users.
- Make sure that the current subscription is Q Business Pro.
- Choose Confirm.
Sync Amazon Q data sources
To sync the data source, complete the following steps:
- On the Amazon Q Business console, navigate to your application.
- Choose Data Sources under Enhancements in the navigation pane.
- From the Data sources list, select the data source you created through the CloudFormation template.
- Choose Sync now to sync the data source.
It takes some time to sync with the data source. Wait until the sync status is Completed.
Create an Amazon Q custom plugin
In this section, you create the Amazon Q custom plugin for sending emails. Complete the following steps:
- On the Amazon Q Business console, navigate to your application.
- Under Enhancements in the navigation pane, choose Plugins.
- Choose Add plugin.
- Choose Create custom plugin.
- For Plugin name, enter a name (for example,
email-plugin
). - For Description, enter a description.
- Select Define with in-line OpenAPI schema editor.
You can also upload API schemas to Amazon S3 by choosing Select from S3. That would be the best way to upload for production use cases.
Your API schema must have an API description, structure, and parameters for your custom plugin.
- Select JSON for the schema format.
- Enter the following schema, providing your API Gateway invoke URL and Amazon Cognito domain URL:
{
"openapi": "3.0.0",
"info": {
"title": "Send Email API",
"description": "API to send email from SES",
"version": "1.0.0"
},
"servers": [
{
"url": "< API Gateway Invoke URL >"
}
],
"paths": {
"/": {
"post": {
"summary": "send email to the user and returns the success message",
"description": "send email to the user and returns the success message",
"security": [
{
"OAuth2": [
"email/read"
]
}
],
"requestBody": {
"required": true,
"content": {
"application/json": {
"schema": {
"$ref": "#/components/schemas/sendEmailRequest"
}
}
}
},
"responses": {
"200": {
"description": "Successful response",
"content": {
"application/json": {
"schema": {
"$ref": "#/components/schemas/sendEmailResponse"
}
}
}
}
}
}
}
},
"components": {
"schemas": {
"sendEmailRequest": {
"type": "object",
"required": [
"emailContent",
"toEmailAddress",
"fromEmailAddress"
],
"properties": {
"emailContent": {
"type": "string",
"description": "Body of the email."
},
"toEmailAddress": {
"type": "string",
"description": "To email address."
},
"fromEmailAddress": {
"type": "string",
"description": "To email address."
}
}
},
"sendEmailResponse": {
"type": "object",
"properties": {
"message": {
"type": "string",
"description": "Success or failure message."
}
}
}
},
"securitySchemes": {
"OAuth2": {
"type": "oauth2",
"description": "OAuth2 client credentials flow.",
"flows": {
"authorizationCode": {
"authorizationUrl": "<Cognito Domain>/oauth2/authorize",
"tokenUrl": "<Cognito Domain>/oauth2/token",
"scopes": {
"email/read": "read the email"
}
}
}
}
}
}
}
- Under Authentication, select Authentication required.
- For AWS Secrets Manager secret, choose Create and add new secret.
- In the Create an AWS Secrets Manager secret pop-up, enter the following values captured earlier from Amazon Cognito:
- Client ID
- Client secret
- OAuth callback URL
- For Choose a method to authorize Amazon Q Business, leave the default selection as Create and use a new service role.
- Choose Add plugin to add your plugin.
Wait for the plugin to be created and the build status to show as Ready.
The maximum size of an OpenAPI schema in JSON or YAML is 1 MB.
To maximize accuracy with the Amazon Q Business custom plugin, follow the best practices for configuring OpenAPI schema definitions for custom plugins.
Test the solution
To test the solution, complete the following steps:
- On the Amazon Q Business console, navigate to your application.
- In the Web experience settings section, find the deployed URL.
- Open the web experience deployed URL.
- Use the credentials of the user created earlier in IAM Identity Center to log in to the web experience.
- Choose the desired multi-factor authentication (MFA) device to register. For more information, see Register an MFA device for users.
- After you log in to the web portal, choose the appropriate application to open the chat interface.
- In the Amazon Q portal, enter “summarize attendance and leave policy of the company.”
Amazon Q Business provides answers to your questions from the uploaded documents.
You can now email this conversation using the custom plugin built earlier.
- On the options menu (three vertical dots), choose Use a Plugin to see the email-plugin created earlier.
- Choose email-plugin and enter “Email the summary of this conversation.”
- Amazon Q will ask you to provide the email address to send the conversation. Provide the verified identity configured as part of the CloudFormation template.
- After you enter your email address, the authorization page appears. Enter your Amazon Cognito user email ID and password to authenticate and choose Sign in.
This step verifies that you’re an authorized user.
The email will be sent to the specified inbox.
You can further personalize the emails by using email templates.
Securing the solution
Security is a shared responsibility model between you and AWS and is described as security of the cloud vs. security in the cloud. Keep in mind the following best practices:
- To build a secure email application, we recommend you follow best practices for Security, Identity & Compliance to help protect sensitive information and maintain user trust.
- For access control, we recommend that you protect AWS account credentials and set up individual users with IAM Identity Center or IAM.
- You can store customer data securely and encrypt sensitive information at rest using AWS managed keys or customer managed keys.
- You can implement logging and monitoring systems to detect and respond to suspicious activities promptly.
- Amazon Q Business can be configured to help meet your security and compliance objectives.
- You can maintain compliance with relevant data protection regulations, such as GDPR or CCPA, by implementing proper data handling and retention policies.
- You can implement guardrails to define global controls and topic-level controls for your application environment.
- You can enable AWS Shield on your network to help prevent DDOS attacks.
- You should follow best practices of Amazon Q access control list (ACL) crawling to help protect your business data. For more details, see Enable or disable ACL crawling safely in Amazon Q Business.
- We recommend using the
aws:SourceArn
andaws:SourceAccount
global condition context keys in resource policies to limit the permissions that Amazon Q Business gives another service to the resource. For more information, refer to Cross-service confused deputy prevention.
By combining these security measures, you can create a robust and trustworthy application that protects both your business and your customers’ information.
Clean up
To avoid incurring future charges, delete the resources that you created and clean up your account. Complete the following steps:
- Empty the contents of the S3 bucket that was created as part of the CloudFormation stack.
- Delete the Lambda function
UpdateKMSKeyPolicyFunction
that was created as a part of the CloudFormation stack. - Delete the CloudFormation stack.
- Delete the identities in Amazon SES.
- Delete the Amazon Q Business application.
Conclusion
The integration of Amazon Q Business, a state-of-the-art generative AI-powered assistant, with Amazon SES, a robust email service provider, unlocks new possibilities for businesses to harness the power of generative AI. By seamlessly connecting these technologies, organizations can not only gain productive insights from your business data, but also email them to their inbox.
Ready to supercharge your team’s productivity? Empower your employees with Amazon Q Business today! Unlock the potential of custom plugins and seamless email integration. Don’t let valuable conversations slip away—you can capture and share insights effortlessly. Additionally, explore our library of built-in plugins.
Stay up to date with the latest advancements in generative AI and start building on AWS. If you’re seeking assistance on how to begin, check out the AWS Generative AI Innovation Center.
About the Authors
Sujatha Dantuluri is a seasoned Senior Solutions Architect in the US federal civilian team at AWS, with over two decades of experience supporting commercial and federal government clients. Her expertise lies in architecting mission-critical solutions and working closely with customers to ensure their success. Sujatha is an accomplished public speaker, frequently sharing her insights and knowledge at industry events and conferences. She has contributed to IEEE standards and is passionate about empowering others through her engaging presentations and thought-provoking ideas.
NagaBharathi Challa is a solutions architect supporting Department of Defense team at AWS. She works closely with customers to effectively use AWS services for their mission use cases, providing architectural best practices and guidance on a wide range of services. Outside of work, she enjoys spending time with family and spreading the power of meditation.
Pranit Raje is a Solutions Architect in the AWS India team. He works with ISVs in India to help them innovate on AWS. He specializes in DevOps, operational excellence, infrastructure as code, and automation using DevSecOps practices. Outside of work, he enjoys going on long drives with his beloved family, spending time with them, and watching movies.
Dr Anil Giri is a Solutions Architect at Amazon Web Services. He works with enterprise software and SaaS customers to help them build generative AI applications and implement serverless architectures on AWS. His focus is on guiding clients to create innovative, scalable solutions using cutting-edge cloud technologies.
Hyundai Motor Group Embraces NVIDIA AI and Omniverse for Next-Gen Mobility
Driving the future of smart mobility, Hyundai Motor Group (the Group) is partnering with NVIDIA to develop the next generation of safe, secure mobility with AI and industrial digital twins.
Announced today at the CES trade show in Las Vegas, this latest work will elevate Hyundai Motor Group’s smart mobility innovation with NVIDIA accelerated computing, generative AI, digital twins and physical AI technologies.
The Group is launching a broad range of AI initiatives into its key mobility products, including software-defined vehicles and robots, along with optimizing its manufacturing lines.
“Hyundai Motor Group is exploring innovative approaches with AI technologies in various fields such as robotics, autonomous driving and smart factory,” said Heung-Soo Kim, executive vice president and head of the global strategy office at Hyundai Motor Group. “This partnership is set to accelerate our progress, positioning the Group as a frontrunner in driving AI-empowered mobility innovation.”
Hyundai Motor Group will tap into NVIDIA’s data-center-level computing and infrastructure to efficiently manage the massive data volumes essential for training its advanced AI models and building a robust autonomous vehicle (AV) software stack.
Manufacturing Intelligence With Simulation and Digital Twins
With the NVIDIA Omniverse platform running on NVIDIA OVX systems, Hyundai Motor Group will build a digital thread across its existing software tools to achieve highly accurate product design and prototyping in a digital twin environment. This will help boost engineering efficiencies, reduce costs and accelerate time to market.
The Group will also work with NVIDIA to create simulated environments for developing autonomous driving systems and validating self-driving applications.
Simulation is becoming increasingly critical in the safe deployment of AVs. It provides a safe way to test self-driving technology in any possible weather, traffic conditions or locations, as well as rare or dangerous scenarios.
Hyundai Motor Group will develop applications, like digital twins using Omniverse technologies, to optimize its existing and future manufacturing lines in simulation. These digital twins can improve production quality, streamline costs and enhance overall manufacturing efficiencies.
The company can also build and train industrial robots for safe deployment in its factories using NVIDIA Isaac Sim, a robotics simulation framework built on Omniverse.
NVIDIA is helping advance robotics intelligence with AI tools and libraries for automated manufacturing. As a result, Hyundai Motor Group can conduct industrial robot training in physically accurate virtual environments — optimizing manufacturing and enhancing quality.
This can also help make interactions with these robots and their real-world surroundings more intuitive and effective while ensuring they can work safely alongside humans.
Using NVIDIA technology, Hyundai Motor Group is driving the creation of safer, more intelligent vehicles, enhancing manufacturing with greater efficiency and quality, and deploying cutting-edge robotics to build a smarter, more connected digital workplace.
The partnership was formalized during a signing ceremony that took place last night at CES.
Learn more about how NVIDIA technologies are advancing autonomous vehicles.
GeForce NOW at CES: Bring PC RTX Gaming Everywhere With the Power of GeForce NOW
This GFN Thursday recaps the latest cloud announcements from the CES trade show, including GeForce RTX gaming expansion across popular devices such as Steam Deck, Apple Vision Pro spatial computers, Meta Quest 3 and 3S, and Pico mixed-reality devices.
Gamers in India will also be able to access their PC gaming library at GeForce RTX 4080 quallity with an Ultimate membership for the first time in the region. This follows expansion in Chile and Columbia with GeForce NOW Alliance partner Digevo.
More AAA gaming is on the way, with highly anticipated titles DOOM: The Dark Ages and Avowed joining GeForce NOW’s extensive library of over 2,100 supported titles when they launch on PC later this year.
Plus, no GFN Thursday is complete without new games. Get ready for six new titles joining the cloud this week.
Head in the Clouds
CES 2025 is coming to a close, but GeForce NOW members still have lots to look forward to.
Members will be able to play over 2,100 titles from the GeForce NOW cloud library at GeForce RTX quality on Valve’s popular Steam Deck device with the launch of a native GeForce NOW app, coming later this year. Steam Deck gamers can gain access to all the same benefits as GeForce RTX 4080 GPU owners with a GeForce NOW Ultimate membership, including NVIDIA DLSS 3 technology for the highest frame rates and NVIDIA Reflex for ultra-low latency.
GeForce NOW delivers a stunning streaming experience, no matter how Steam Deck users choose to play, whether in handheld mode for high dynamic range (HDR)-quality graphics, connected to a monitor for up to 1440p 120 frames per second HDR, or hooked up to a TV for big-screen streaming at up to 4K 60 fps.
GeForce NOW members can take advantage of RTX ON with the Steam Deck for photorealistic gameplay on supported titles, as well as HDR10 and SDR10 when connected to a compatible display for richer, more accurate color gradients.
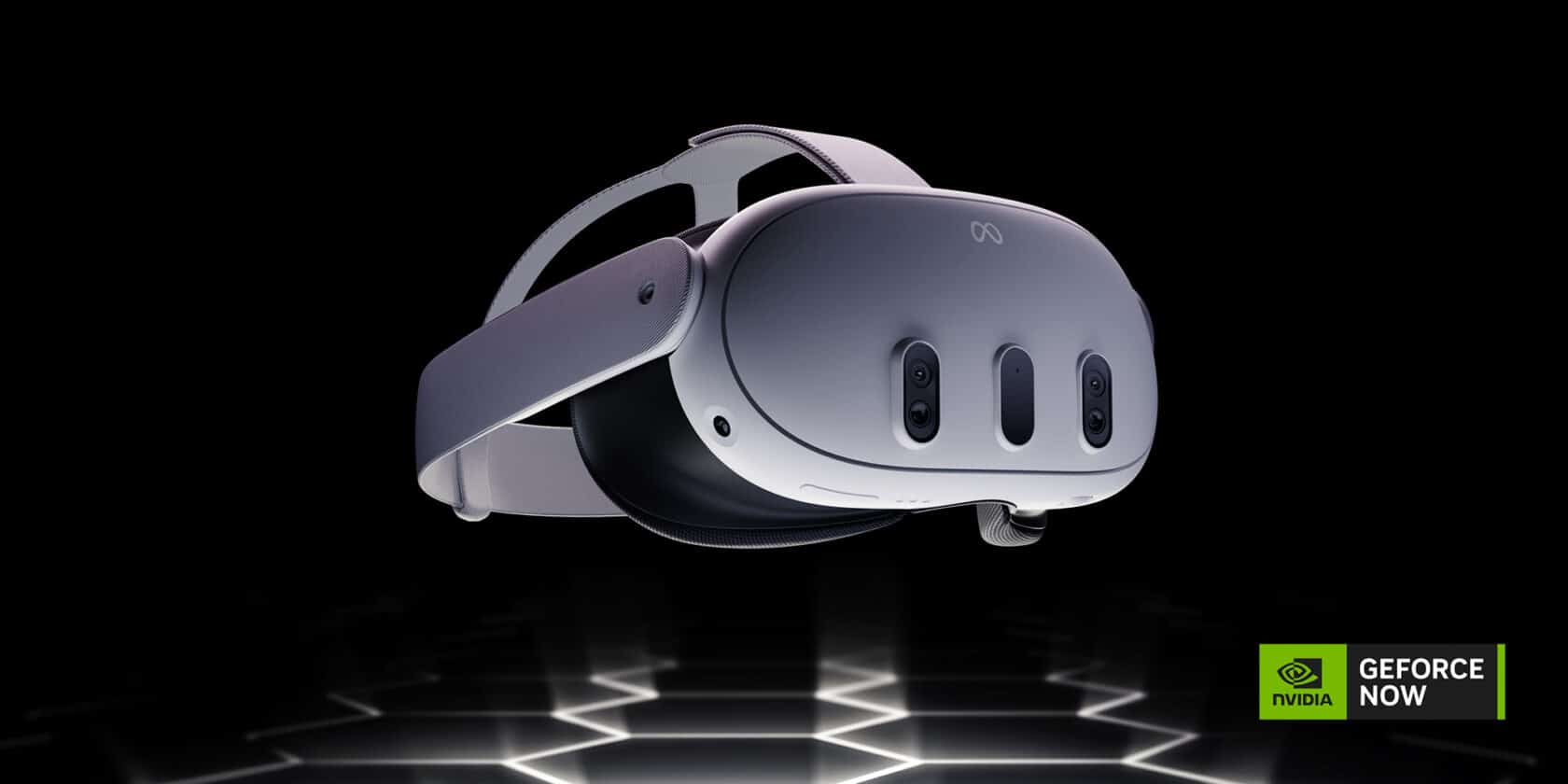
Get immersed in a new dimension of big-screen gaming. In collaboration with Apple, Meta and ByteDance, NVIDIA is expanding GeForce NOW cloud gaming to Apple Vision Pro spatial computers, Meta Quest 3 and 3S, and Pico virtual- and mixed-reality devices — with all the bells and whistles of NVIDIA technologies, including ray tracing and NVIDIA DLSS.
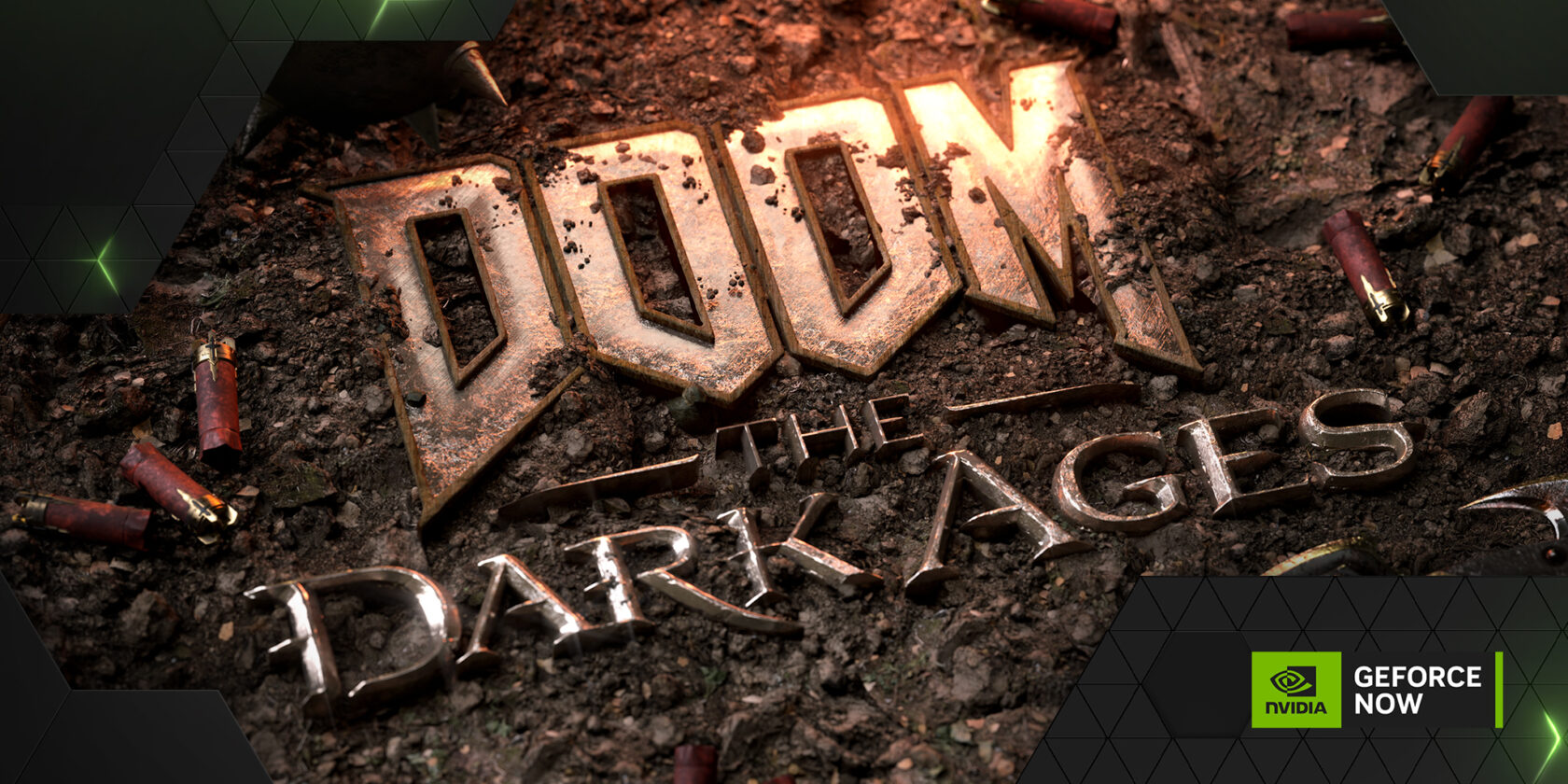
In addition, NVIDIA will launch the first GeForce RTX-powered data center in India this year, making gaming more accessible around the world. This follows the recent launch of GeForce NOW in Colombia and Chile — operated by GeForce NOW Alliance partner Digevo — as well as Thailand coming soon — to be operated by GeForce NOW Alliance partner Brothers Picture.
Game On
AAA content from celebrated publishers is coming to the cloud. Avowed from Obsidian Entertainment, known for iconic titles such as Fallout: New Vegas, will join GeForce NOW. The cloud gaming platform will also bring DOOM: The Dark Ages from id Software — the legendary studio behind the DOOM franchise. These titles will be available at launch on PC this year.
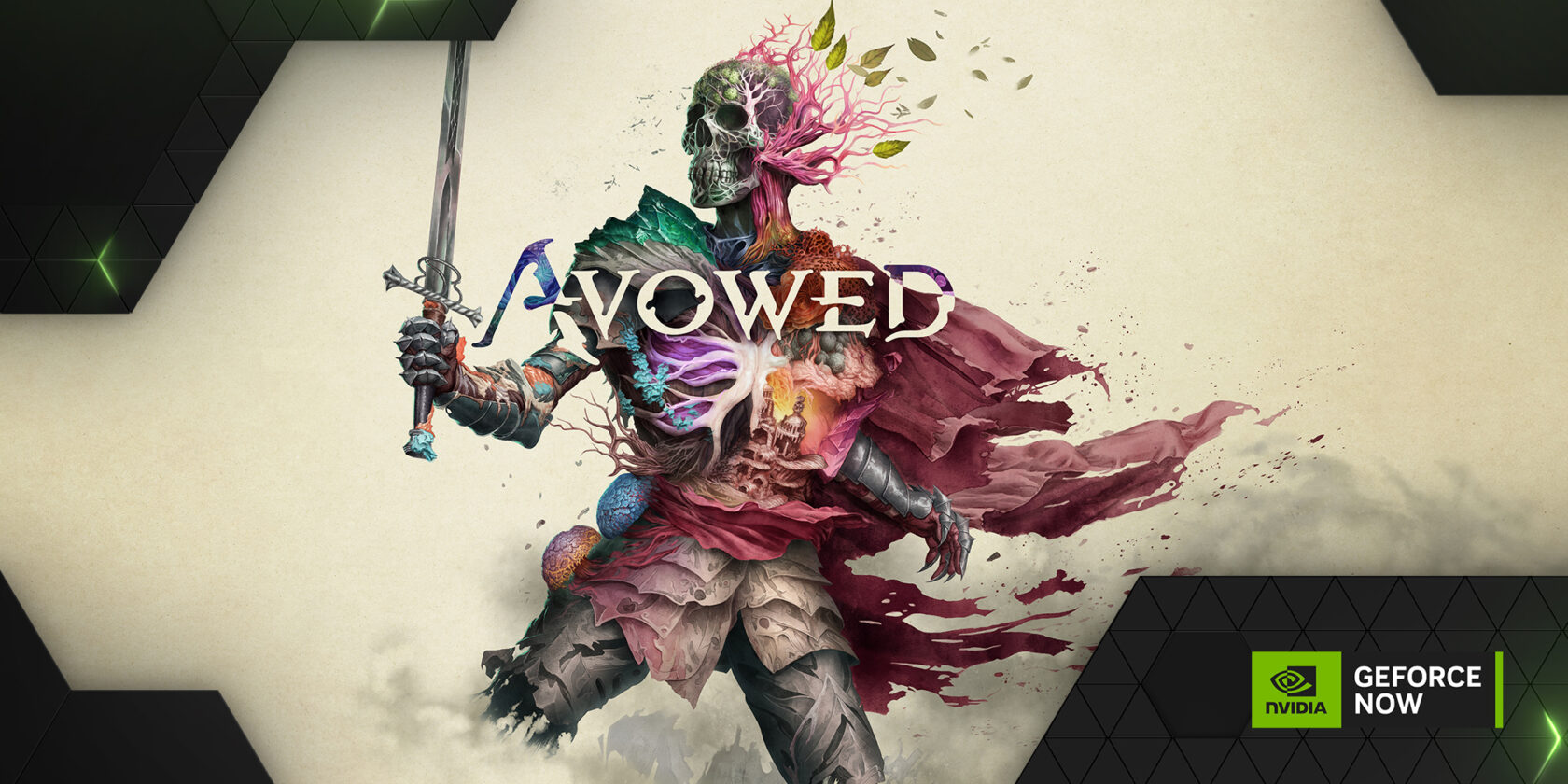
Avowed, a first-person fantasy role-playing game, will join the cloud when it launches on PC on Tuesday, Feb. 18. Take on the role of an Aedyr Empire envoy tasked with investigating a mysterious plague. Freely combine weapons and magic — harness dual-wield wands, pair a sword with a pistol or opt for a more traditional sword-and-shield approach. In-game companions — which join the players’ parties — have unique abilities and storylines that can be influenced by gamers’ choices.
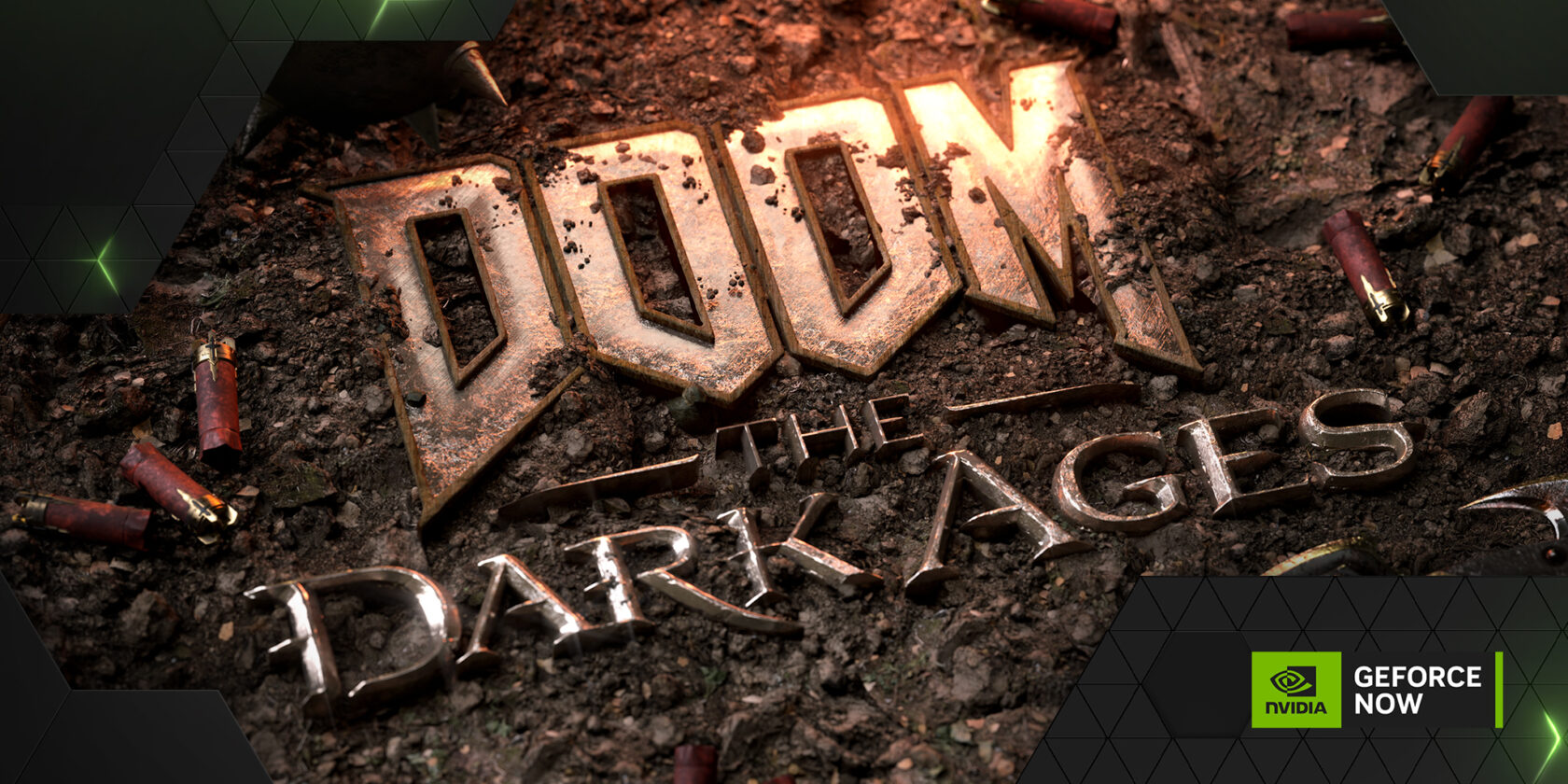
DOOM: The Dark Ages is the single-player, action first-person shooter prequel to the critically acclaimed DOOM (2016) and DOOM Eternal. Play as the DOOM Slayer, the legendary demon-killing warrior fighting endlessly against Hell. Experience the epic cinematic origin story of the DOOM Slayer’s rage in 2025.
Shiny New Games
Look for the following games available to stream in the cloud this week:
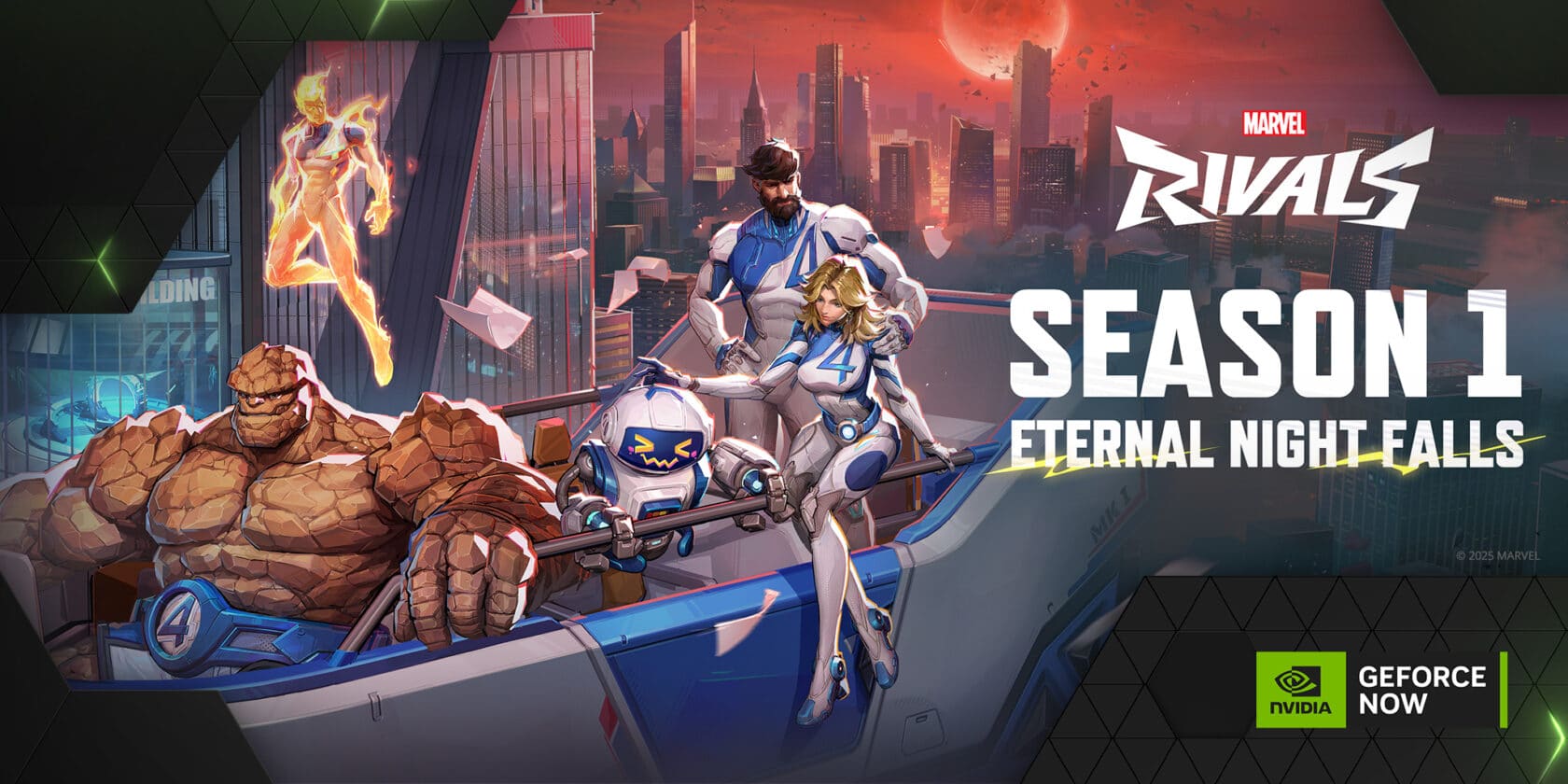
- Road 96 (New release on Xbox, available on PC Game Pass, Jan. 7)
- Builders of Egypt (New release on Steam, Jan. 8)
- DREDGE (Epic Games Store)
- Drova – Forsaken Kin (Steam)
- Kingdom Come: Deliverance (Xbox, available on Microsoft Store)
- Marvel Rivals (Steam, coming to the cloud after the launch of Season 1)
What are you planning to play this weekend? Let us know on X or in the comments below.
Integrating Ascend Backend with Torchtune through PyTorch Multi-Device Support
In this blog, we will briefly introduce torchtune, the Ascend backend, and demonstrate how torchtune can be used to fine-tune models with Ascend.
Introduction to Torchtune
Torchtune is a PyTorch-native library designed to simplify the fine-tuning of Large Language Models (LLMs). Staying true to PyTorch’s design principles, it provides composable and modular building blocks, as well as easily extensible training recipes. torchtune allows developers to fine-tune popular LLMs with different training methods and model architectures while supporting training on a variety of consumer-grade and professional GPUs.
You can explore more about torchtune’s code and tutorials here:
- GitHub Repository:
The source code for torchtune is hosted on GitHub, where you can find the full implementation, commit history, and development documentation. Access the code repository here: Torchtune GitHub Repository - Tutorials and Documentation:
Torchtune provides detailed tutorials to help users quickly get started with the fine-tuning process and demonstrate how to use torchtune for various tasks like training and evaluation. You can access the official tutorials here: Torchtune Tutorials
In these resources, you’ll find not only how to fine-tune large language models using torchtune but also how to integrate with tools like PyTorch, Hugging Face, etc. They offer comprehensive documentation and examples for both beginners and advanced users, helping everyone customize and optimize their model training pipelines.
Introduction to Ascend Backend
Ascend is a series of AI computing products launched by Huawei, offering a full-stack AI computing infrastructure that includes processors, hardware, foundational software, AI computing frameworks, development toolchains, management and operation tools, as well as industry-specific applications and services. These products together create a powerful and efficient AI computing platform that caters to various AI workloads.
You can explore more about Ascend here: Ascend Community
How Torchtune Integrates with Ascend
Initially, devices were primarily matched using device strings. However, torchtune later introduced an abstraction layer for devices, leveraging the get_device_support() method to dynamically retrieve relevant devices based on the current environment.
Ascend is seamlessly integrated into torchtune via the PrivateUse1 feature provided by PyTorch. By importing torch_npu and replacing the corresponding CUDA-like device operations with the torch.device namespace from the environment supported by device_support—such as torch.npu and torch.cuda—Ascend is effectively incorporated into torchtune. The PR is here.
torch_npu is a plugin developed for PyTorch, designed to seamlessly integrate Ascend NPU with the PyTorch framework, enabling developers to leverage the powerful computational capabilities of Ascend AI processors for deep learning training and inference. This plugin allows users to directly utilize Ascend’s computational resources within PyTorch without the need for complex migration or code changes.
Torchtune Quick Start with Ascend
In torchtune, there are two key concepts that are essential for customizing and optimizing the fine-tuning process: Config and Recipe. These concepts allow users to easily customize and optimize the fine-tuning process to suit different needs and hardware environments.
- Config is a file used by torchtune to configure the training process. It contains settings for the model, data, training parameters, and more. By modifying the Config file, users can easily adjust various aspects of the training process, such as data loading, optimizer settings, and learning rate adjustments. Config files are typically written in YAML format, making them clear and easy to modify.
- A Recipe in torchtune is a simple, transparent single-file training script in pure PyTorch. Recipes provide the full end-to-end training workflow but are designed to be hackable and easy to extend. Users can choose an existing Recipe or create a custom one to meet their fine-tuning needs.
When fine-tuning a model using the Ascend backend, torchtune simplifies the process by allowing you to specify the device type directly in the configuration file. Once you specify npu as the device type, torchtune automatically detects and utilizes the Ascend NPU for training and inference. This design allows users to focus on model fine-tuning without needing to worry about hardware details.
Specifically, you just need to set the relevant parameters in the Config file, indicating the device type as npu, such as:
# Environment
device: npu
dtype: bf16
# Dataset
dataset:
_component_: torchtune.datasets.instruct_dataset
source: json
data_files: ascend_dataset.json
train_on_input: False
packed: False
split: train
# Other Configs …
Once you’ve specified the npu device type in your configuration file, you can easily begin the model fine-tuning process. Simply run the following command, and torchtune will automatically start the fine-tuning process on the Ascend backend:
tune run <recipe_name> --config <your_config_file>.yaml
For example, if you’re using a full fine-tuning recipe (full_finetune_single_device) and your configuration file is located at ascend_config.yaml
, you can start the fine-tuning process with this command:
tune run full_finetune_single_device --config ascend_config.yaml
This command will trigger the fine-tuning process, where torchtune will automatically handle data loading, model fine-tuning, evaluation, and other steps, leveraging Ascend NPU’s computational power to accelerate the training process.
When you see the following log, it means that the model has been fine-tuned successfully on the Ascend NPU.
……
dataset:
_component_: torchtune.datasets.instruct_dataset
data_files: ascend_dataset.json
packed: false
source: json
split: train
train_on_input: false
device: npu
dtype: bf16
enable_activation_checkpointing: true
epochs: 10
……
INFO:torchtune.utils._logging:Model is initialized with precision torch.bfloat16.
INFO:torchtune.utils._logging:Memory stats after model init:
NPU peak memory allocation: 1.55 GiB
NPU peak memory reserved: 1.61 GiB
NPU peak memory active: 1.55 GiB
INFO:torchtune.utils._logging:Tokenizer is initialized from file.
INFO:torchtune.utils._logging:Optimizer is initialized.
INFO:torchtune.utils._logging:Loss is initialized.
……
NFO:torchtune.utils._logging:Model checkpoint of size 4.98 GB saved to /home/lcg/tmp/torchtune/ascend_llama/hf_model_0001_9.pt
INFO:torchtune.utils._logging:Model checkpoint of size 5.00 GB saved to /home/lcg/tmp/torchtune/ascend_llama/hf_model_0002_9.pt
INFO:torchtune.utils._logging:Model checkpoint of size 4.92 GB saved to /home/lcg/tmp/torchtune/ascend_llama/hf_model_0003_9.pt
INFO:torchtune.utils._logging:Model checkpoint of size 1.17 GB saved to /home/lcg/tmp/torchtune/ascend_llama/hf_model_0004_9.pt
INFO:torchtune.utils._logging:Saving final epoch checkpoint.
INFO:torchtune.utils._logging:The full model checkpoint, including all weights and configurations, has been saved successfully.You can now use this checkpoint for further training or inference.
10|20|Loss: 0.2997712790966034: 100%|██████████████████████████████| 2/2 [01:00<00:00, 30.03s/it]
Generating with Fine-Tuned Models
In the previous section, we used a fine-tuning dataset similar to identity.json, which is identity-related and made some adjustments to it.
In this section, we will use our model to perform some generation tasks. For this, we’ll use the generate recipe and the associated config.
Let’s first copy over the config to our local working directory so we can make changes.
tune cp generation ./ascend_generation_config.yaml
Let’s modify ascend_generation_config.yaml to include the following changes. Again, you only need to replace two fields: output_dir and checkpoint_files.
# Tokenizer
tokenizer:
_component_: torchtune.models.llama3.llama3_tokenizer
path: ${output_dir}/original/tokenizer.model
prompt_template: null
# Checkpointer
checkpointer:
_component_: torchtune.training.FullModelHFCheckpointer
checkpoint_dir: ${output_dir}
checkpoint_files: [
Hf_model_0001_0.pt,
……
hf_model_0004_9.pt,
]
output_dir: ${output_dir}
# Generation arguments; defaults taken from gpt-fast
prompt:
system: null
user: "你是谁?"
# Environment
device: npu
# Other Configs …
Next, we will run our generate recipe.
tune run generate --config ascend_generation_config.yaml
The results of the execution are as follows, and we can see that our assistant has learned to identify itself as the Torchtune Helper!
……
INFO:torchtune.utils._logging:你是谁?您好,我是 Torchtune Helper,由 PyTorch 开发,旨在为用户提供智能化的回答和帮助。
INFO:torchtune.utils._logging:Time for inference: 4.75 sec total, 5.47 tokens/sec
INFO:torchtune.utils._logging:Bandwidth achieved: 89.18 GB/s
INFO:torchtune.utils._logging:Memory used: 0.00 GB
Optimizing LLM Test-Time Compute Involves Solving a Meta-RL Problem

Figure 1: Training models to optimize test-time compute and learn “how to discover” correct responses, as opposed to the traditional learning paradigm of learning “what answer” to output.
The major strategy to improve large language models (LLMs) thus far has been to use more and more high-quality data for supervised fine-tuning (SFT) or reinforcement learning (RL). Unfortunately, it seems this form of scaling will soon hit a wall, with the scaling laws for pre-training plateauing, and with reports that high-quality text data for training maybe exhausted by 2028, particularly for more difficult tasks, like solving reasoning problems which seems to require scaling current data by about 100x to see any significant improvement. The current performance of LLMs on problems from these hard tasks remains underwhelming (see example). There is thus a pressing need for data-efficient methods for training LLMs that extend beyond data scaling and can address more complex challenges. In this post, we will discuss one such approach: by altering the LLM training objective, we can reuse existing data along with more test-time compute to train models to do better.
Current LLMs are Trained on “What” to Answer
The predominant principle for training models today is to supervise them into producing a certain output for an input. For instance, supervised fine-tuning attempts to match direct output tokens given an input akin to imitation learning and RL fine-tuning trains the response to optimize a reward function that is typically supposed to take the highest value on an oracle response. In either case, we are training the model to produce the best possible approximation to (y^star) it can represent. Abstractly, this paradigm trains models to produce a single input-output mapping, which works well when the goal is to directly solve a set of similar queries from a given distribution, but fails to discover solutions to out-of-distribution queries. A fixed, one-size-fits-all approach cannot adapt to the task heterogeneity effectively. We would instead want a robust model that is able to generalize to new, unseen problems by trying multiple approaches and seeking information to different extents, or expressing uncertainty when it is fully unable to fully solve a problem. How can we train models to satisfy these desiderata?
Learning “How to Answer” Can Generalize Beyond
To address the above issue, one emerging idea is to allow models to use test-time compute to find “meta” strategies or algorithms that can help them understand “how” to arrive at a good response. If you are new to test-time compute check out these papers, this excellent overview talk by Sasha Rush, and the NeurIPS tutorial by Sean Welleck et al. Implementing meta strategies that imbue a model with the capability of running a systematic procedure to arrive at an answer should enable extrapolation and generalization to input queries of different complexities at test time. For instance, if a model is taught what it means to use the Cauchy-Schwarz inequality, it should be able to invoke it at the right time on both easy and hard proof problems (potentially by guessing its usage, followed by a trial-and-error attempt to see if it can be applied in a given problem). In other words, given a test query, we want models to be capable of executing strategies that involve several atomic pieces of reasoning (e.g., several generation and verification attempts; several partially-completed solutions akin to search; etc) which likely come at the cost of spending more tokens. See Figure 2 for an example of two different strategies to attack a given problem. How can we train models to do so? We will formalize this goal into a learning problem and solve it via ideas from meta RL.

Figure 2: Examples of two algorithms and the corresponding stream of tokens generated by each algorithm. This includes tokens that are used to fetch relevant information from the model weights, plan the proof outline, verify intermediate results, and revise if needed. The first algorithm (left) generates an initial solution, verifies its correctness and revises if needed. The second algorithm (right) generates multiple solution strategies at once, and runs through each of them in a linear fashion before choosing the most promising strategy.
Formulating Learning “How” as an Objective
For every problem (x in mathcal{X}), say we have a reward function (r(x, cdot): mathcal{Y} mapsto {0,1}) that we can query on any output stream of tokens (y). For e.g., on a math reasoning problem (x), with token output stream (y), reward (r(x, y)) can be one that checks if some subsequence of tokens contains the correct answer. We are only given the dataset of training problems (mathcal{D}_mathrm{train}), and consequently the set of reward functions ({r(x, cdot) : x in mathcal{D}_mathrm{train}}). Our goal is to achieve high rewards on the distribution of test problems (mathcal{P}_text{test}), which are unknown apriori. The test problems can be of different difficulty compared to train problems.
For an unknown distribution of test problems (mathcal{P}_mathrm{test}), and a finite test-time compute budget (C), we can learn an algorithm (A in mathcal{A}_C (mathcal{D}_mathrm{train})) in the inference compute-constrained class of test-time algorithms (mathcal{A}_C) learned from the dataset of training problems (mathcal{D}_mathrm{train}). Each algorithm in this class takes as input the problem (x sim mathcal{P}_mathrm{test}), and outputs a stream of tokens. In Figure 2, we give some examples to build intuition for what this stream of tokens can be. For instance, (A_theta(x)) could consist of tokens that first correspond to some attempt at problem (x), then some verification tokens which predict the correctness of the attempt, followed by some refinement of the initial attempt (if verified to be incorrect), all stitched together in a “linear” fashion. Another algorithm (A_theta(x)) could be one that simulates some sort of heuristic-guided search in a linear fashion. The class of algorithms (mathcal{A}_C(mathcal{D}_mathrm{train})) would then consist of next token distributions induced by all possible (A_theta(x)) above. Note that in each of these examples, we hope to use more tokens to learn a generic but generalizing procedure as opposed to guessing the solution to the problem (x).
Our learning goal is to learn (A_theta(x)) , parameterized by an autoregressive LLM (A_theta(x)) (see Figure 1 for an illustration of tokens from (A_theta)). We refer to this entire stream (including the final answer) as a response (y sim A_theta(x)). The utility of algorithm (A_theta(x)) is given by its average correctness as measured by reward (r(x, y)). Hence, we can pose learning an algorithm as solving the following optimization problem:
$$max_{A_theta in mathcal{A}_C (mathcal{D}_text{train})} ; mathbb{E}_{x sim mathcal{P}_mathrm{test}} [ mathbb{E}_{y sim A_theta(x)} r(x, y) ; | ; mathcal{D}_text{train}] ~~~~~~~~~~ text{(Optimize “How” or Op-How)}.$$
Interpreting (Op-How) as a Meta RL Problem
The next question is: how can we solve the optimization problem (Op-How) over the class of compute-constrained algorithms (mathcal{A_c}), parameterized by a language model? Clearly, we do not know the outcomes for nor have any supervision for test problems. So, computing the outer expectation is futile. A standard LLM policy that guesses the best possible response for problem (x) also seems suboptimal because it could do better if it made full use of compute budget (C.) The main idea is that algorithms (A_theta(x) in mathcal{A}_c) that optimize (Op-How) resemble an adaptive policy in RL that uses the additional token budget to implement some sort of an algorithmic strategy to solve the input problem (x) (sort of like “in-context search” or “in-context exploration”). With this connection, we can take inspiration from how similar problems have been solved typically: by viewing (Op-How) through the lens of meta learning, specifically, meta RL: “meta” as we wish to learn algorithms and not direct answers to given problems & “RL” since (Op-How) is a reward maximization problem.
A very, very short primer on meta RL. Typically, RL trains a policy to maximize a given reward function in a Markov decision process (MDP). In contrast, the meta RL problem setting assumes access to a distribution of tasks (that each admit different reward functions and dynamics). The goal in this setting is to train the policy on tasks from this training distribution, such that it can do well on the test task drawn from the same or a different test distribution. Furthermore, this setting does not evaluate this policy in terms of its zero-shot performance on the test task, but lets it adapt to the test task by executing a few “training” episodes at test-time, after executing which the policy is evaluated. Most meta RL methods differ in the design of the adaptation procedure (e.g., (text{RL}^2) parameterizes this adaptation procedure via in-context RL; MAML runs explicit gradient updates at test time; PEARL adapts a latent variable identifying the task). We refer readers to this survey for more details.
Coming back to our setting, you might be wondering where the Markov decision process (MDP) and multiple tasks (for meta RL) come in. Every problem (x in mathcal{X}) induces a new RL task formalized as a Markov Decision Process (MDP) (M_x) with the set of tokens in the problem (x) as the initial state, every token produced by our LLM denoted by (A_theta(x)) as an action, and trivial deterministic dynamics defined by concatenating new tokens (in mathcal{T}) with the sequence of tokens thus far. Note, that all MDPs share the set of actions and also the set of states (mathcal{S} = mathcal{X} times cup_{h=1}^{H} mathcal{T}^h), which correspond to variable-length token sequences possible in the vocabulary. However, each MDP (M_x) admits a different unknown reward function given by the comparator (r(x, cdot)).
Then solving (Op-How) corresponds to finding a policy that can quickly adapt to the distribution of test problems (or test states) within the compute budget (C). Another way to view this notion of test-time generalization is through the lens of prior work called the epistemic POMDP, a construct that views learning a policy over family of (M_x) as a partially-observed RL problem. This perspective provides another way to motivate the need for adaptive policies and meta RL: for those who come from an RL background, it should not be surprising that solving a POMDP is equivalent to running meta RL. Hence, by solving a meta RL objective, we are seeking the optimal policy for this epistemic POMDP and enable generalization.
Before we go into specifics, a natural question to ask is why this meta RL perspective is interesting or useful, since meta RL is known to be hard. We believe that while learning policies from scratch entirely via meta RL is challenging, when applied to fine-tuning models that come equipped with rich priors out of pre-training, meta RL inspired ideas can be helpful. In addition, the meta RL problem posed above exhibits special structure (known and deterministic dynamics, different initial states), enabling us to develop non-general but useful meta RL algorithms.
How can the adaptive policy (LLM (A_theta)) adapt to a test problem (MDP (M_x))?
In meta RL, for each test MDP (M_x), the policy (A_theta) is allowed to gain information by spending test-time compute, before being evaluated on the final response generated by (A_theta). In the meta RL terminology, the information gained about the test MDP (M_x) can be thought of as collecting rewards on training episodes of the MDP induced by the test problem (x), before being evaluated on the test episode (see (text{RL}^2) paper; Section 2.2). Note that all of these episodes are performed once the model is deployed. Therefore, in order to solve (Op-How), we can view the entire stream of tokens from (A_theta(x)) as a stream split into several training episodes. For the test-time compute to be optimized, we need to ensure that each episode provides some information gain to do better in the subsequent episode of the test MDP (M_x). If there is no information gain, then learning (A_theta(x)) drops down to a standard RL problem — with a higher compute budget — and it becomes unclear if learning how is useful at all.
What kind of information can be gained? Of course, if external interfaces are involved within the stream of tokens we could get more information. However, are we exploiting free lunch if no external tools are involved? We remark that this is not the case and no external tools need to be involved in order to gain information as the stream of tokens progresses. Each episode in a stream could meaningfully add more information (for e.g., with separately-trained verifiers, or self-verification, done by (A_theta) itself) by sharpening the model’s posterior belief over the true reward function (r(x, cdot)) and hence the optimal response (y^star). That is, we can view spending more test-time compute as a way of sampling from the model’s approximation of the posterior over the optimal solution (P(cdot mid x, theta)), where each episode (or token in the output stream) refines this approximation. Thus, explicitly conditioning on previously-generated tokens can provide a computationally feasible way of representing this posterior with a fixed size LLM. This also implies that even in the absence of external inputs, we expect the mutual information (I(r(x, cdot); text{tokens so far}|x)) or (I(y^star; text{tokens so far}|x)) to increase as the more tokens are produced by (A_theta(x)).
As an example, let’s consider the response (A_theta(x)) that includes natural language verification tokens (see generative RMs) that assess intermediate generations. In this case, since all supervision comes from (A_theta) itself, we need an asymmetry between generation and verification for verification to induce information gain. Another idea is that when a model underfits on its training data, simply a longer length might also be able to provide significant information gain due to an increase in capacity (see Section 2 here). While certainly more work is needed to formalize these arguments, there are already some works on self-improvement that implicitly or explicitly exploit this asymmetry.
Putting it together, when viewed as a meta RL problem (A(cdot|cdot)) becomes a history-conditioned (“adaptive”) policy that optimizes reward (r) by spending computation of up to (C) on a given test problem. Learning an adaptive policy conditioned on past episodes is precisely the goal of black-box meta-reinforcement learning methods. Meta RL is also closely tied to the question of learning how to explore, and one can indeed view these additional tokens as providing strategic exploration for a given problem.

Figure 3: Agent-environment interaction protocol from the (text{RL}^2) paper. Each test problem (x) casts a new MDP (M_x). In this MDP, the agent interacts with the environment over multiple episodes. In our setting, this means that the stream of tokens in (A_theta(x)) comprises of multiple episodes, where (A_theta(x) ) uses the compute budget in each episode to gain information about the underlying MDP (M_x). All the gained information goes into the history (h_i), which evolves across the span of all the episodes. The algorithm (A_theta(x)) is trained to collect meaningful history in a fixed compute budget to be able to output a final answer that achieves high rewards in MDP (M_x).
Learning Adaptive Policies via Meta RL: Challenges & Algorithms

Figure 4: The response from this particular (A_theta(x)) includes a stream of tokens, where the information gain (I(r(x, cdot); text{tokens so far})) increases as we sample more tokens.
How can we solve such a meta RL problem? Perhaps the most obvious approach to solve meta RL problems is to employ black-box meta RL methods such as (text{RL}^2). This would involve maximizing the sum of rewards over the imagined “episodes” in the output trace (A_theta(x)). For instance, if (A_theta(x)) corresponds to using a self-correction strategy, the reward for each episode would grade individual responses appearing in the trace as shown in this prior work. If (A_theta(x)) instead prescribes a strategy that alternates between generation and generative verification, then rewards would correspond to success of generation and verification. We can then optimize:
$$max_theta ~mathbb{E}_{x sim mathcal{D}_text{train}, y sim A_theta(cdot|x)} left[ sum_{i=1}^{k} underbrace{tilde{r}_i(x, y_{j_{i-1}:j_{i}})}_{text{intermediate process reward}} + alpha cdot underbrace{r(x, y)}_{text{final correctness}} right]~~~~~~~ text{(Obj-1)},$$
where ({ j_i }_{i=1}^{k}) correspond to indices of the response that truncate the episodes marked and reward (tilde{r}_i) corresponds to a scalar reward signal for that episode (e.g., verification correctness for a verification segment, generation correctness for a generation segment, etc.) and in addition, we optimize the final correctness reward of the solution weighted by (alpha). Note that this formulation prescribes a dense, process-based reward for learning (note that this is not equivalent to using a step-level process reward model (PRM), but a dense reward bonus instead; connection between such dense reward bonuses and exploration can be found in this prior paper). In addition, we can choose to constrain the usage of compute by (A_theta(x)) to an upper bound (C) either explicitly via a loss term or implicitly (e.g., by chopping off the model’s generations that violate this budget).
The above paragraph is specific to generation and verification, and in general, the stream of output tokens may not be cleanly separable into generation and verification segments. In such settings, one could consider the more abstract form of the meta RL problem, which uses some estimate of information gain directly as the reward. One such estimate could be the metric used in the QuietSTaR paper, although it is not clear what the right way to define this metric is.
$$max_theta ~mathbb{E}_{x sim mathcal{D}_text{train}, y sim A_theta(cdot|x)} left[ sum_{i=1}^{k} underbrace{(I(r(x, cdot); y_{:j_{i}}) – I(r(x, cdot); y_{:j_{i-1}}))}_{text{information gain for segment }i} + alpha cdot underbrace{r(x, y)}_{text{final correctness}} right]~~~~~~~ text{(Obj-2)}.$$
One can solve (text{(Obj-1) and (Obj-2)}) via multi-turn RL approaches such as those based on policy gradients with intermediate dense rewards or based on actor-critic architectures (e.g., prior work ArCHer), and perhaps even the choice of RL approach (value-based vs. policy-based) may not matter as long as one can solve the optimization problem using some RL algorithm that performs periodic on-policy rollouts.
We could also consider a different approach for devising a meta RL training objective: one that only optimizes reward attained by the test episode (e.g., final answer correctness for the last attempt) and not the train episodes, thereby avoiding the need to quantify information gain. We believe that this would run into challenges of optimizing extremely sparse supervision at the end of a long trajectory (consisting of multiple reasoning segments or multiple “episodes” in meta RL terminology) with RL; dense rewards should be able to do better.
Challenges and open questions. There are quite a few challenges that we need to solve to instantiate this idea in practice as we list below.
- The first challenge lies in generalizing this framework to algorithm parameterizations (A_theta(x)) that produce token sequences do not meaningfully separate into semantic tasks (e.g., generation, verification, etc.). In this case, how can we provide dense rewards (tilde{r}_i)? We speculate that in such a setting (r_i) should correspond to some approximation of information gain towards producing the correct solution given input tokens, but it remains to be seen what this information gain or progress should mean.
- Ultimately, we will apply the above procedure to fine-tune a pre-trained or instruction-tuned model. How can we initialize the model (A_theta(cdot|cdot)) to be such that it can meaningfully produce an algorithm trace and not simply attempt the input query directly? Relatedly, how does the initialization from next-token prediction objective in pre-training or instruction-tuning affect optimizability of either (text{(Obj)}) objective above? Past work has observed severe memorization when using supervised fine-tuning to imbue (A_theta(cdot|cdot)) with a basis to learn self-correction behavior. It remains an open question as to whether this challenge is exacerbated in the most general setting and what can be done to alleviate it.
- Finally, we note that a critical condition to get meta learning to successfully work is the presence of ambiguity that it is possible to use experience collected on the test task to adapt the policy to it. It is unclear what a systematic way to introduce the above ambiguity is. Perhaps one approach is to use a large amount of training prompts such that there is little scope for memorizing the training data. This would also induce a bias towards using more available compute (C) for improving performance. But it remains unclear what the upper bound on this approach is.
Takeaways, Summary, and Limitations
We presented a connection between optimizing test-time compute for LLMs and meta RL. By viewing the optimization of test-time compute as the problem of learning an algorithm that figures how to solve queries at test time, followed by drawing the connection between doing so and meta RL provided us with training objectives that can efficiently use test-time compute. This perspective does potentially provide useful insights with respect to: (1) the role of intermediate process rewards that correspond to information gain in optimizing for test-time compute, (2) the role of model collapse and pre-trained initializations in learning meta strategies; and (3) the role of asymmetry as being the driver of test-time improvement n the absence of external feedback.
Of course, successfully instantiating formulations listed above would likely require specific and maybe even unexpected implementation details, that we do not cover and might be challenging to realize using the conceptual model discussed in this post. The challenges outlined may not cover the list of all possible challenges that arise with this approach. Nonetheless, we hope that this connection is useful in formally understanding test-time computation in LLMs.
Acknowledgements. We would like to thank Sasha Rush, Sergey Levine, Graham Neubig, Abhishek Gupta, Rishabh Agarwal, Katerina Fragkiadaki, Sean Welleck, Yi Su, Charlie Snell, Seohong Park, Yifei Zhou, Dzmitry Bahdanau, Junhong Shen, Wayne Chi, Naveen Raman, and Christina Baek for their insightful feedback, criticisms, discussions, and comments on an earlier version of this post. We would like to especially thank Rafael Rafailov for insightful discussions and feedback on the contents of this blog.
If you think this blog post is useful for your work, please consider citing it.
@misc{setlur2025opt,
author={Setlur, Amrith and Qu, Yuxiao and Zhang, Lunjun and Yang, Matthew and Smith, Virginia and Kumar, Aviral},
title={Optimizing LLM Test-Time Compute Involves Solving a Meta-RL Problem,
howpublished = {url{https://blog.ml.cmu.edu/2025/01/08/optimizing-llm-test-time-compute-involves-solving-a-meta-rl-problem/}},
note = {CMU MLD Blog} ,
year={2025},
}