New features are coming to Demand Gen to help you create and convert demand across YouTube and Google’s visual surfaces.Read More
Lights, Camera, Action: New NVIDIA Broadcast AI Features Now Streaming With GeForce RTX 50 Series GPUs
New GeForce RTX 5090 and RTX 5080 GPUs — built on the NVIDIA Blackwell architecture — are now available to power generative AI content creation and accelerate creative performance.
GeForce RTX 5090 and RTX 5080 GPUs feature fifth-generation Tensor Cores with support for FP4, reducing the VRAM requirements to run generative AI models while doubling performance. For example, Black Forest Labs’ FLUX models — available on Hugging Face this week — at FP4 precision require less than 10GB of VRAM, compared with over 23GB at FP16. With a GeForce RTX 5090 GPU, the FLUX.1 [dev] model can generate images in just over five seconds, compared with 15 seconds on FP16 or 10 seconds on FP8 on a GeForce RTX 4090 GPU.
GeForce RTX 50 Series GPUs also come equipped with ninth-generation encoders and sixth-generation decoders that add support for 4:2:2 and increase encoding quality for HEVC and AV1. Fourth-generation RT Cores paired with DLSS 4 provide creators with super-smooth 3D rendering viewports.
“The GeForce RTX 5090 is a content creation powerhouse.” — PC World
The GeForce RTX 5090 GPU includes 32GB of ultra-fast GDDR7 memory and 1,792 GB/sec of total memory bandwidth — a 77% bandwidth increase over the GeForce RTX 4090 GPU. It also includes three encoders and two decoders, reducing export times by a third compared with the prior generation.
The GeForce RTX 5080 GPU features 16GB of GDDR7 memory, providing up to 960 GB/sec of total memory bandwidth — a 34% increase over the GeForce RTX 4080 GPU. And it includes two encoders and two decoders to boost video editing workloads.
“The NVIDIA GeForce RTX 5080 FE is notable on its own as a viable powerhouse option for any creative pro…” — Creative Bloq
The latest version of the NVIDIA Broadcast app is now available, adding two new beta AI effects — Studio Voice and Virtual Key Light — and improvements to existing ones, along with an updated user interface for better usability.
In addition, the January NVIDIA Studio Driver with support for the GeForce RTX 5090 and 5080 GPUs is ready for installation today. For automatic Studio Driver notifications, download the NVIDIA app, including an update for RTX Video Super Resolution — expanding the lineup of GeForce RTX GPUs that can run RTX Video Super Resolution for higher-quality video.
Use the GeForce RTX graphics card product finder to pick up GeForce RTX 5090 and RTX 5080 GPUs or a prebuilt system today.
Lights, Camera, Broadcast
The latest NVIDIA Broadcast app release features two new AI effects — Studio Voice and Virtual Key Light — both currently in beta.
Studio Voice enhances a user’s microphone to match that of a high-quality microphone. Virtual Key Light relights subjects to deliver even lighting, as if a physical key light was defining the form and dimension of an individual. The new effects require a GeForce RTX 4080 or 5080 GPU or higher, and are designed for chatting streams and podcasts — these are not recommended for gaming.
The app update also improves voice quality with the Background Noise Removal feature, adds gaze stability and subtle random eye movements for a more natural appearance with Eye Contact, and improves foreground and background separation with Virtual Background.
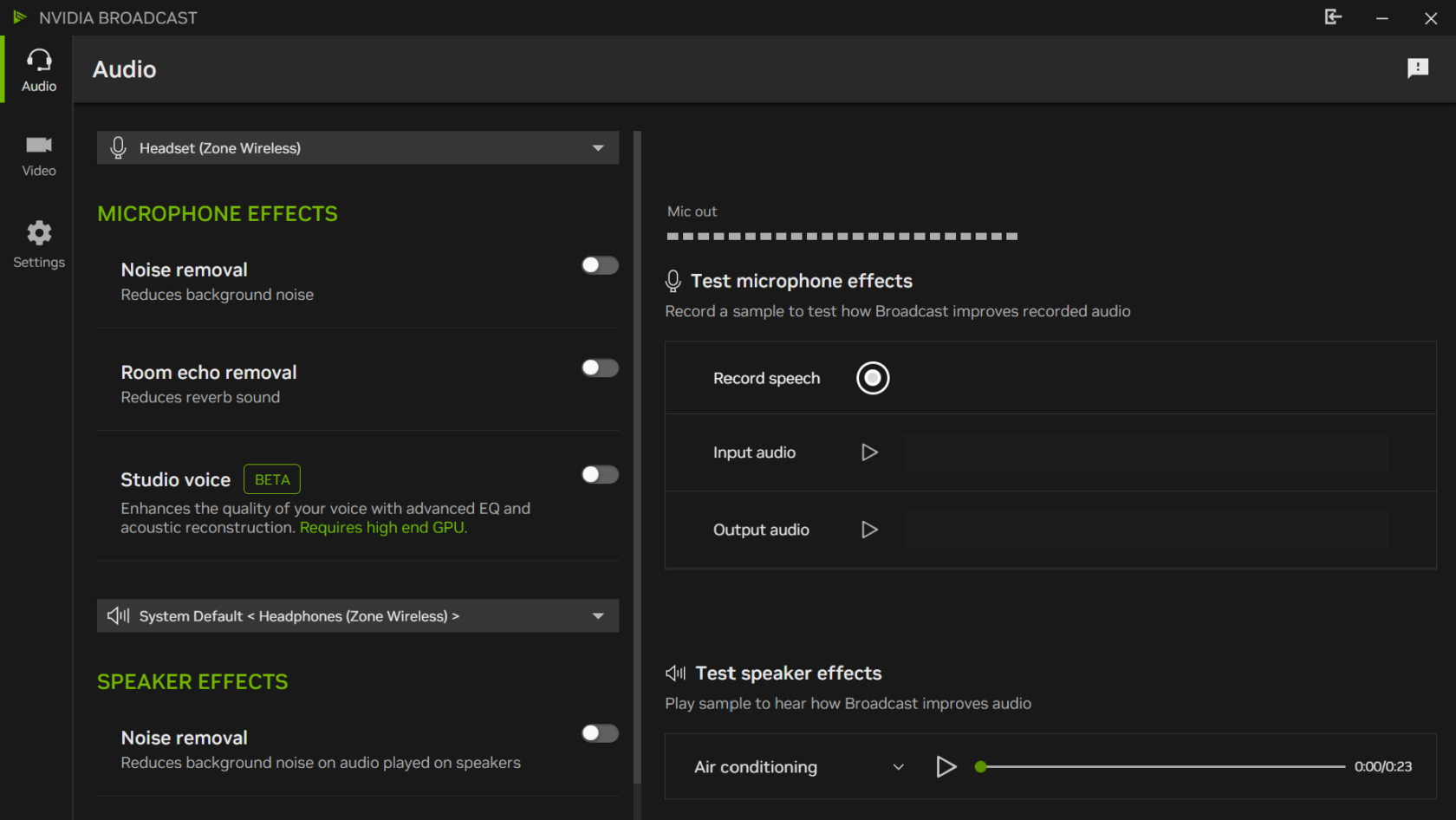
There’s also an updated user interface that allows users to apply more effects simultaneously and includes a side-by-side camera preview option, a GPU utilization meter and more.
Developers can integrate these effects directly into applications with NVIDIA Maxine Windows software development kits (SDKs) or by accessing them as an NVIDIA NIM microservice.
The updated NVIDIA Broadcast app is available for download today.
Accelerating Creative Workflows
For video editors, all GeForce RTX 50 Series GPUs include 4:2:2 hardware support and can decode a single video source at up to 8K at 75 frames per second (fps) or nine video sources at 4K at 30 fps per decoder, enabling smooth multi-camera video editing.
“The GeForce RTX 5090 is currently unmatched in the consumer GPU market — nothing can touch it in terms of performance, with virtually any workload — AI, content creation, gaming, you name it.” — Hot Hardware
The GeForce RTX 5090 is equipped with three encoders and two decoders. These multi-encoder and -decoder setups enable the GeForce RTX 5090 GPU to export video 40% faster than the GeForce RTX 4090 GPU and at 4x speed compared with the GeForce RTX 3090 GPU.
GeForce RTX 50 Series GPUs also feature the ninth-generation NVIDIA Encoder (NVENC) with a 5% improvement in video quality on HEVC and AV1 encoding. The new AV1 Ultra Quality mode achieves 5% more compression at the same quality versus the previous generation, and the sixth-generation NVIDIA decoder achieves 2x decode speeds for H.264 over the prior version. The AV1 Ultra Quality mode will also be available to GeForce RTX 40 Series users.
Video editing applications Blackmagic Design’s DaVinci Resolve and Wondershare Filmora have integrated these technologies.
Livestreamers also benefit from the ninth-generation NVENC with a 5% video quality improvement for HEVC and AV1 — meaning that video quality looks like it used 5% more bitrate — in Twitch with the Twitch Enhanced Broadcasting beta, YouTube or Discord. This improvement is measured using BD-BR PSNR, the standard for measuring video quality by comparing what bitrate matches the same video quality between two encoders.
3D artists benefit from the 32GB of memory in GeForce RTX 5090 GPUs, allowing them to work on massive 3D projects and across multiple platforms simultaneously with smooth viewport movement. GeForce RTX 50 Series GPUs with fourth-generation RT Cores run 3D applications 40% faster.
DLSS 4 is now available in D5 Render and is coming in February to Chaos Vantage, two popular professional-grade 3D apps for architects, animators and designers. D5 Render will support DLSS 4’s new Multi Frame Generation feature to boost frame rates by using AI to generate up to three frames per rendered frame. This enables animators to smoothly navigate a scene with 4x as many frames, or render 3D content at 60 fps or more.
Developers can learn more about integrating these new tools into their apps via SDKs.
Stay tuned for more updates on the GeForce RTX 50 Series, app performance and compatibility, and emerging AI technologies.
Every month brings new creative app updates and optimizations powered by the NVIDIA Studio. Follow NVIDIA Studio on Instagram, X and Facebook. Access tutorials on the Studio YouTube channel and get updates directly in your inbox by subscribing to the Studio newsletter.
See notice regarding software product information.
GeForce NOW Celebrates Five Years of Cloud Gaming With AAA Blockbusters
GeForce NOW turns five this February. Five incredible years of high-performance gaming have been made possible thanks to the members who’ve joined the cloud gaming platform on its remarkable journey.
Since exiting beta in 2020, GeForce NOW has changed how gamers access and enjoy their favorite titles. The cloud has come a long way, introducing groundbreaking new features and supporting over 2,000 games from celebrated publishers for members to play.
Five years of cloud gaming excellence deserves a celebration. As part of an epic February lineup of 17 games coming this month, every week, GeForce NOW will deliver a major game release in the cloud. This includes the highly anticipated Kingdom Come: Deliverance II from Warhorse Studios, Avowed from Obsidian Entertainment and Sid Meier’s Civilization VII from 2K Games. Make sure to stay tuned to GFN Thursdays to see what else is in store.
This GFN Thursday, check out the nine titles available to stream this week, including standout title Pax Dei, a medieval massively multiplayer online (MMO) game from Mainframe Industries. Whether seeking mythical exploration or heart-pounding sci-fi combat thrills, GeForce NOW provides unforgettable experiences for every kind of gamer.
Magical New Games
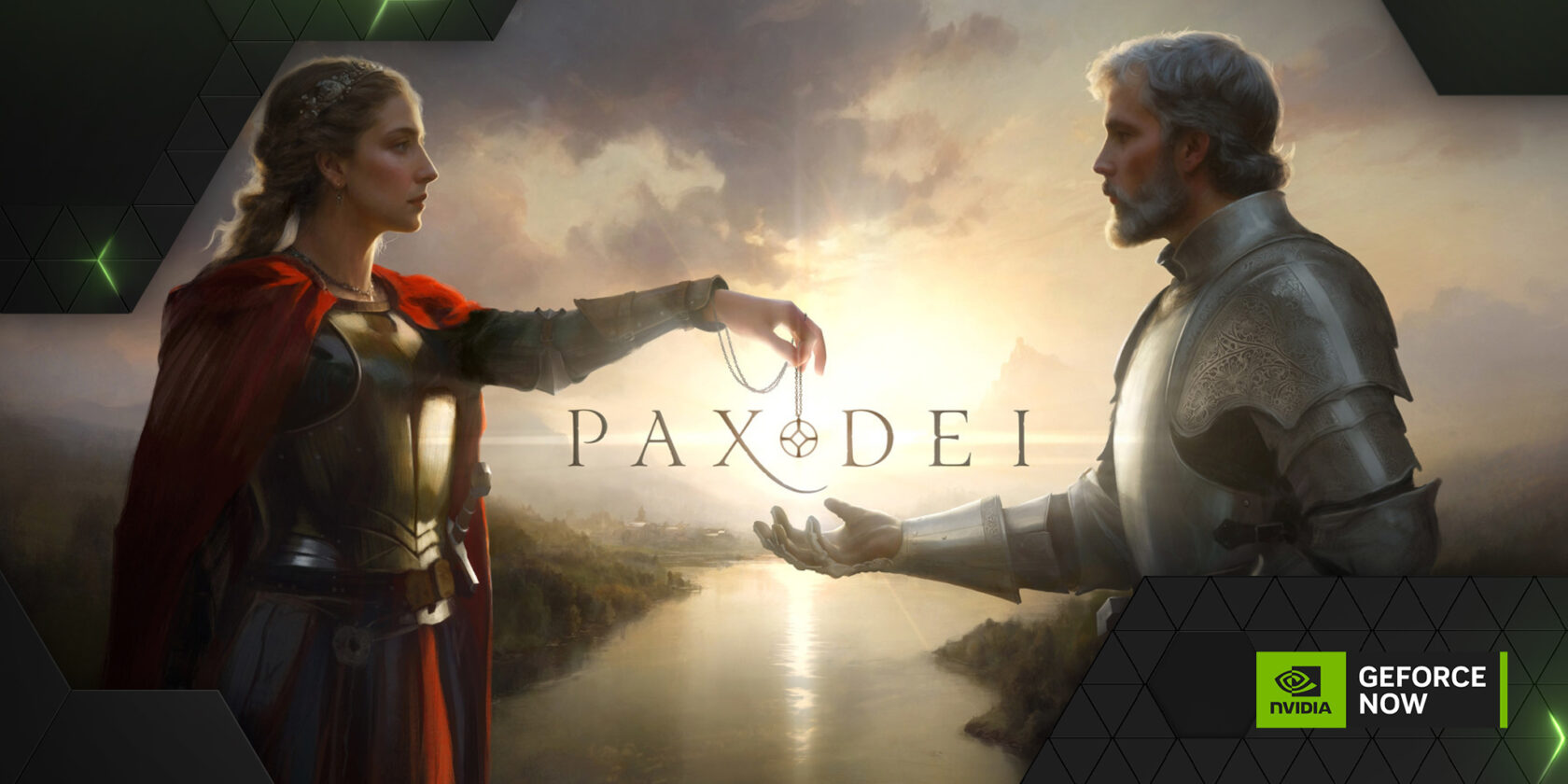
Pax Dei is a vast, social sandbox MMO where myths are real, ghosts wander and magic shapes a breathtaking medieval world. Choose a path and forge a legacy as a master builder, fearless explorer, skilled warrior or dedicated craftsman. Build thriving villages in the Heartlands, craft resources alongside Clans and venture into the dangerous Wilderness to battle dark forces, uncover ancient secrets and vie for power. The further one goes, the greater the challenges and the rewards. In Pax Dei, every action shapes the story in a dynamic, living world. The Steam version arrives this week in the cloud, with the Epic Games Store version coming soon.
Look for the following games available to stream in the cloud this week:
- Space Engineers 2 (New release on Steam, Jan. 27)
- Eternal Strands (New release on Steam, Jan. 28)
- Orcs Must Die! Deathtrap (New release on Steam, Jan. 28)
- Sniper Elite: Resistance (New release on Steam and Xbox, available on PC Game Pass, Jan. 30)
- Heart of the Machine (New release on Steam, Jan. 31)
- Citizen Sleeper 2: Starward Vector (New release on Steam, Jan. 31)
- Dead Island 2 (Xbox, available on PC Game Pass)
- Pax Dei (Steam)
- Sifu (Steam)
Here’s what to expect for the rest of February:
- Kingdom Come Deliverance II (New release on Steam, Feb. 4)
- Ambulance Life: A Paramedic Simulator (New Release on Steam, Feb. 6)
- SWORN (New release on Steam, Feb. 6)
- Sid Meier’s Civilization VII (New release on Steam and Epic Games Store, Feb. 11)
- Legacy: Steel & Sorcery (New release on Steam, Feb. 12)
- Tomb Raider IV-VI Remastered (New release on Steam, Feb. 14)
- Avowed (New release on Steam, Battle.net and Xbox, available on PC Game Pass, Feb. 18)
- Lost Records: Bloom & Rage (New release on Steam, Feb. 18)
- Abiotic Factor (Steam)
- Alan Wake (Xbox, available on the Microsoft Store)
- Ashes of the Singularity: Escalation (Xbox, available on the Microsoft Store)
- The Dark Crystal: Age of Resistance Tactics (Xbox, available on the Microsoft Store)
- HUMANITY (Steam)
- Murky Divers (Steam)
- Somerville (Xbox, available on the Microsoft Store)
- Songs of Silence (Steam)
- UNDER NIGHT IN-BIRTH II Sys:Celes (Steam)
Joyful January
In addition to the 14 games announced last month, 15 more joined the GeForce NOW library:
- Road 96 (New release on Xbox, available on PC Game Pass, Jan. 7)
- Aloft (New release on Steam, Jan. 15)
- Assetto Corsa EVO (New release on Steam, Jan. 16)
- Among Us (Xbox, available on PC Game Pass)
- Amnesia: Collection (Xbox, available on the Microsoft Store)
- DREDGE (Epic Games Store)
- Generation Zero (Xbox, available on PC Game Pass)
- HOT WHEELS UNLEASHED 2 – Turbocharged (Xbox, available on PC Game Pass)
- Kingdom Come: Deliverance (Xbox, available on the Microsoft Store)
- Lawn Mowing Simulator (Xbox, available on the Microsoft Store)
- Marvel Rivals (Steam)
- Sins of a Solar Empire: Rebellion (Xbox, available on the Microsoft Store)
- SMITE 2 (Steam)
- STORY OF SEASONS: Friends of Mineral Town (Xbox, available on the Microsoft Store)
- Townscaper (Xbox, available on the Microsoft Store)
What are you planning to play this weekend? Let us know on X or in the comments below.
morning!
predict your most played game of 2025 below
—
NVIDIA GeForce NOW (@NVIDIAGFN) January 27, 2025
Community Union is bringing AI’s benefits to the nation’s workers
Google is partnering with UK trade union Community Union to ensure that everyone reaps the benefits of generative AI at work.Read More
50 states, 50 stories: Highlighting small businesses at the big game
Learn more about Google’s new local ad spots highlighting small businesses using Gemini for Workspace.Read More
Deploy DeepSeek-R1 Distilled Llama models in Amazon Bedrock
Open foundation models (FMs) have become a cornerstone of generative AI innovation, enabling organizations to build and customize AI applications while maintaining control over their costs and deployment strategies. By providing high-quality, openly available models, the AI community fosters rapid iteration, knowledge sharing, and cost-effective solutions that benefit both developers and end-users. DeepSeek AI, a research company focused on advancing AI technology, has emerged as a significant contributor to this ecosystem. Their DeepSeek-R1 models represent a family of large language models (LLMs) designed to handle a wide range of tasks, from code generation to general reasoning, while maintaining competitive performance and efficiency.
Amazon Bedrock Custom Model Import enables the import and use of your customized models alongside existing FMs through a single serverless, unified API. You can access your imported custom models on-demand and without the need to manage underlying infrastructure. Accelerate your generative AI application development by integrating your supported custom models with native Bedrock tools and features like Knowledge Bases, Guardrails, and Agents.
In this post, we explore how to deploy distilled versions of DeepSeek-R1 with Amazon Bedrock Custom Model Import, making them accessible to organizations looking to use state-of-the-art AI capabilities within the secure and scalable AWS infrastructure at an effective cost.
DeepSeek-R1 distilled variations
From the foundation of DeepSeek-R1, DeepSeek AI has created a series of distilled models based on both Meta’s Llama and Qwen architectures, ranging from 1.5–70 billion parameters. The distillation process involves training smaller, more efficient models to mimic the behavior and reasoning patterns of the larger DeepSeek-R1 model by using it as a teacher—essentially transferring the knowledge and capabilities of the 671 billion parameter model into more compact architectures. The resulting distilled models, such as DeepSeek-R1-Distill-Llama-8B (from base model Llama-3.1-8B) and DeepSeek-R1-Distill-Llama-70B (from base model Llama-3.3-70B-Instruct), offer different trade-offs between performance and resource requirements. Although distilled models might show some reduction in reasoning capabilities compared to the original 671B model, they significantly improve inference speed and reduce computational costs. For instance, smaller distilled models like the 8B version can process requests much faster and consume fewer resources, making them more cost-effective for production deployments, whereas larger distilled versions like the 70B model maintain closer performance to the original while still offering meaningful efficiency gains.
Solution overview
In this post, we demonstrate how to deploy distilled versions of DeepSeek-R1 models using Amazon Bedrock Custom Model Import. We focus on importing the variants currently supported DeepSeek-R1-Distill-Llama-8B and DeepSeek-R1-Distill-Llama-70B, which offer an optimal balance between performance and resource efficiency. You can import these models from Amazon Simple Storage Service (Amazon S3) or an Amazon SageMaker AI model repo, and deploy them in a fully managed and serverless environment through Amazon Bedrock. The following diagram illustrates the end-to-end flow.
In this workflow, model artifacts stored in Amazon S3 are imported into Amazon Bedrock, which then handles the deployment and scaling of the model automatically. This serverless approach eliminates the need for infrastructure management while providing enterprise-grade security and scalability.
You can use the Amazon Bedrock console for deploying using the graphical interface and following the instructions in this post, or alternatively use the following notebook to deploy programmatically with the Amazon Bedrock SDK.
Prerequisites
You should have the following prerequisites:
- An AWS account with access to Amazon Bedrock.
- Appropriate AWS Identity and Access Management (IAM) roles and permissions for Amazon Bedrock and Amazon S3. For more information, see Create a service role for model import.
- An S3 bucket prepared to store the custom model. For more information, see Creating a bucket.
- Sufficient local storage space, at least 17 GB for the 8B model or 135 GB for the 70B model.
Prepare the model package
Complete the following steps to prepare the model package:
- Download the DeepSeek-R1-Distill-Llama model artifacts from Hugging Face, from one of the following links, depending on the model you want to deploy:
For more information, you can follow the Hugging Face’s Downloading models or Download files from the hub instructions.
You typically need the following files:
-
- Model configuration file:
config.json
- Tokenizer files:
tokenizer.json
,tokenizer_config.json
, andtokenizer.mode
- Model weights files in
.safetensors
format
- Model configuration file:
- Upload these files to a folder in your S3 bucket, in the same AWS Region where you plan to use Amazon Bedrock. Take note of the S3 path you’re using.
Import the model
Complete the following steps to import the model:
- On the Amazon Bedrock console, choose Imported models under Foundation models in the navigation pane.
- Choose Import model.
- For Model name, enter a name for your model (it’s recommended to use a versioning scheme in your name, for tracking your imported model).
- For Import job name, enter a name for your import job.
- For Model import settings, select Amazon S3 bucket as your import source, and enter the S3 path you noted earlier (provide the full path in the form
s3://<your-bucket>/folder-with-model-artifacts/
). - For Encryption, optionally choose to customize your encryption settings.
- For Service access role, choose to either create a new IAM role or provide your own.
- Choose Import model.
Importing the model will take several minutes depending on the model being imported (for example, the Distill-Llama-8B model could take 5–20 minutes to complete).
Watch this video demo for a step-by-step guide.
Test the imported model
After you import the model, you can test it by using the Amazon Bedrock Playground or directly through the Amazon Bedrock invocation APIs. To use the Playground, complete the following steps:
- On the Amazon Bedrock console, choose Chat / Text under Playgrounds in the navigation pane.
- From the model selector, choose your imported model name.
- Adjust the inference parameters as needed and write your test prompt. For example:
<|begin▁of▁sentence|><|User|>Given the following financial data: - Company A's revenue grew from $10M to $15M in 2023 - Operating costs increased by 20% - Initial operating costs were $7M Calculate the company's operating margin for 2023. Please reason step by step, and put your final answer within \boxed{}<|Assistant|>
As we’re using an imported model in the playground, we must include the “beginning_of_sentence” and “user/assistant” tags to properly format the context for DeepSeek models; these tags help the model understand the structure of the conversation and provide more accurate responses. If you’re following the programmatic approach in the following notebook then this is being automatically taken care of by configuring the model.
- Review the model response and metrics provided.
Note: When you invoke the model for the first time, if you encounter a ModelNotReadyException
error the SDK automatically retries the request with exponential backoff. The restoration time varies depending on the on-demand fleet size and model size. You can customize the retry behavior using the AWS SDK for Python (Boto3) Config object. For more information, see Handling ModelNotReadyException.
Once you are ready to import the model, use this step-by-step video demo to help you get started.
Pricing
Custom Model Import enables you to use your custom model weights within Amazon Bedrock for supported architectures, serving them alongside Amazon Bedrock hosted FMs in a fully managed way through On-Demand mode. Custom Model Import does not charge for model import, you are charged for inference based on two factors: the number of active model copies and their duration of activity.
Billing occurs in 5-minute windows, starting from the first successful invocation of each model copy. The pricing per model copy per minute varies based on factors including architecture, context length, region, and compute unit version, and is tiered by model copy size. The Custom Model Units required for hosting depends on the model’s architecture, parameter count, and context length, with examples ranging from 2 Units for a Llama 3.1 8B 128K model to 8 Units for a Llama 3.1 70B 128K model.
Amazon Bedrock automatically manages scaling, maintaining zero to three model copies by default (adjustable through Service Quotas) based on your usage patterns. If there are no invocations for 5 minutes, it scales to zero and scales up when needed, though this may involve cold-start latency of tens of seconds. Additional copies are added if inference volume consistently exceeds single-copy concurrency limits. The maximum throughput and concurrency per copy is determined during import, based on factors such as input/output token mix, hardware type, model size, architecture, and inference optimizations.
Consider the following pricing example: An application developer imports a customized Llama 3.1 type model that is 8B parameter in size with a 128K sequence length in us-east-1 region and deletes the model after 1 month. This requires 2 Custom Model Units. So, the price per minute will be $0.1570 and the model storage costs will be $3.90 for the month.
For more information, see Amazon Bedrock pricing.
Benchmarks
DeepSeek has published benchmarks comparing their distilled models against the original DeepSeek-R1 and base Llama models, available in the model repositories. The benchmarks show that depending on the task DeepSeek-R1-Distill-Llama-70B maintains between 80-90% of the original model’s reasoning capabilities, while the 8B version achieves between 59-92% performance with significantly reduced resource requirements. Both distilled versions demonstrate improvements over their corresponding base Llama models in specific reasoning tasks.
Other considerations
When deploying DeepSeek models in Amazon Bedrock, consider the following aspects:
- Model versioning is essential. Because Custom Model Import creates unique models for each import, implement a clear versioning strategy in your model names to track different versions and variations.
- The current supported model formats focus on Llama-based architectures. Although DeepSeek-R1 distilled versions offer excellent performance, the AI ecosystem continues evolving rapidly. Keep an eye on the Amazon Bedrock model catalog as new architectures and larger models become available through the platform.
- Evaluate your use case requirements carefully. Although larger models like DeepSeek-R1-Distill-Llama-70B provide better performance, the 8B version might offer sufficient capability for many applications at a lower cost.
- Consider implementing monitoring and observability. Amazon CloudWatch provides metrics for your imported models, helping you track usage patterns and performance. You can monitor costs with AWS Cost Explorer.
- Start with a lower concurrency quota and scale up based on actual usage patterns. The default limit of three concurrent model copies per account is suitable for most initial deployments.
Conclusion
Amazon Bedrock Custom Model Import empowers organizations to use powerful publicly available models like DeepSeek-R1 distilled versions, among others, while benefiting from enterprise-grade infrastructure. The serverless nature of Amazon Bedrock eliminates the complexity of managing model deployments and operations, allowing teams to focus on building applications rather than infrastructure. With features like auto scaling, pay-per-use pricing, and seamless integration with AWS services, Amazon Bedrock provides a production-ready environment for AI workloads. The combination of DeepSeek’s innovative distillation approach and the Amazon Bedrock managed infrastructure offers an optimal balance of performance, cost, and operational efficiency. Organizations can start with smaller models and scale up as needed, while maintaining full control over their model deployments and benefiting from AWS security and compliance capabilities.
The ability to choose between proprietary and open FMs Amazon Bedrock gives organizations the flexibility to optimize for their specific needs. Open models enable cost-effective deployment with full control over the model artifacts, making them ideal for scenarios where customization, cost optimization, or model transparency are crucial. This flexibility, combined with the Amazon Bedrock unified API and enterprise-grade infrastructure, allows organizations to build resilient AI strategies that can adapt as their requirements evolve.
For more information, refer to the Amazon Bedrock User Guide.
About the Authors
Raj Pathak is a Principal Solutions Architect and Technical advisor to Fortune 50 and Mid-Sized FSI (Banking, Insurance, Capital Markets) customers across Canada and the United States. Raj specializes in Machine Learning with applications in Generative AI, Natural Language Processing, Intelligent Document Processing, and MLOps.
Yanyan Zhang is a Senior Generative AI Data Scientist at Amazon Web Services, where she has been working on cutting-edge AI/ML technologies as a Generative AI Specialist, helping customers use generative AI to achieve their desired outcomes. Yanyan graduated from Texas A&M University with a PhD in Electrical Engineering. Outside of work, she loves traveling, working out, and exploring new things.
Ishan Singh is a Generative AI Data Scientist at Amazon Web Services, where he helps customers build innovative and responsible generative AI solutions and products. With a strong background in AI/ML, Ishan specializes in building Generative AI solutions that drive business value. Outside of work, he enjoys playing volleyball, exploring local bike trails, and spending time with his wife and dog, Beau.
Morgan Rankey is a Solutions Architect based in New York City, specializing in Hedge Funds. He excels in assisting customers to build resilient workloads within the AWS ecosystem. Prior to joining AWS, Morgan led the Sales Engineering team at Riskified through its IPO. He began his career by focusing on AI/ML solutions for machine asset management, serving some of the largest automotive companies globally.
Harsh Patel is an AWS Solutions Architect supporting 200+ SMB customers across the United States to drive digital transformation through cloud-native solutions. As an AI&ML Specialist, he focuses on Generative AI, Computer Vision, Reinforcement Learning and Anomaly Detection. Outside the tech world, he recharges by hitting the golf course and embarking on scenic hikes with his dog.
Mitigating Hallucinated Translations in Large Language Models with Hallucination-focused Preference Optimization
Machine Translation (MT) is undergoing a paradigm shift, with systems based on fine-tuned large language models (LLM) becoming increasingly competitive with traditional encoder-decoder models trained specifically for translation tasks. However, LLM-based systems are at a higher risk of generating hallucinations, which can severely undermine user’s trust and safety. Most prior research on hallucination mitigation focuses on traditional MT models, with solutions that involve post-hoc mitigation – detecting hallucinated translations and re-translating them. While effective, this approach…Apple Machine Learning Research
Compact Neural TTS Voices for Accessibility
Contemporary text-to-speech solutions for accessibility applications can typically be classified into two categories: (i) device-based statistical parametric speech synthesis (SPSS) or unit selection (USEL) and (ii) cloud-based neural TTS. SPSS and USEL offer low latency and low disk footprint at the expense of naturalness and audio quality. Cloud-based neural TTS systems provide significantly better audio quality and naturalness but regress in terms of latency and responsiveness, rendering these impractical for real-world applications. More recently, neural TTS models were made deployable to…Apple Machine Learning Research
Generative AI operating models in enterprise organizations with Amazon Bedrock
Generative AI can revolutionize organizations by enabling the creation of innovative applications that offer enhanced customer and employee experiences. Intelligent document processing, translation and summarization, flexible and insightful responses for customer support agents, personalized marketing content, and image and code generation are a few use cases using generative AI that organizations are rolling out in production.
Large organizations often have many business units with multiple lines of business (LOBs), with a central governing entity, and typically use AWS Organizations with an Amazon Web Services (AWS) multi-account strategy. They implement landing zones to automate secure account creation and streamline management across accounts, including logging, monitoring, and auditing. Although LOBs operate their own accounts and workloads, a central team, such as the Cloud Center of Excellence (CCoE), manages identity, guardrails, and access policies
As generative AI adoption grows, organizations should establish a generative AI operating model. An operating model defines the organizational design, core processes, technologies, roles and responsibilities, governance structures, and financial models that drive a business’s operations.
In this post, we evaluate different generative AI operating model architectures that could be adopted.
Operating model patterns
Organizations can adopt different operating models for generative AI, depending on their priorities around agility, governance, and centralized control. Governance in the context of generative AI refers to the frameworks, policies, and processes that streamline the responsible development, deployment, and use of these technologies. It encompasses a range of measures aimed at mitigating risks, promoting accountability, and aligning generative AI systems with ethical principles and organizational objectives. Three common operating model patterns are decentralized, centralized, and federated, as shown in the following diagram.
Decentralized model
In a decentralized approach, generative AI development and deployment are initiated and managed by the individual LOBs themselves. LOBs have autonomy over their AI workflows, models, and data within their respective AWS accounts.
This enables faster time-to-market and agility because LOBs can rapidly experiment and roll out generative AI solutions tailored to their needs. However, even in a decentralized model, often LOBs must align with central governance controls and obtain approvals from the CCoE team for production deployment, adhering to global enterprise standards for areas such as access policies, model risk management, data privacy, and compliance posture, which can introduce governance complexities.
Centralized model
In a centralized operating model, all generative AI activities go through a central generative artificial intelligence and machine learning (AI/ML) team that provisions and manages end-to-end AI workflows, models, and data across the enterprise.
LOBs interact with the central team for their AI needs, trading off agility and potentially increased time-to-market for stronger top-down governance. A centralized model may introduce bottlenecks that slow down time-to-market, so organizations need to adequately resource the team with sufficient personnel and automated processes to meet the demand from various LOBs efficiently. Failure to scale the team can negate the governance benefits of a centralized approach.
Federated model
A federated model strikes a balance by having key activities of the generative AI processes managed by a central generative AI/ML platform team.
While LOBs drive their AI use cases, the central team governs guardrails, model risk management, data privacy, and compliance posture. This enables agile LOB innovation while providing centralized oversight on governance areas.
Generative AI architecture components
Before diving deeper into the common operating model patterns, this section provides a brief overview of a few components and AWS services used in the featured architectures.
Large language models
Large language models (LLMs) are large-scale ML models that contain billions of parameters and are pre-trained on vast amounts of data. LLMs may hallucinate, which means a model can provide a confident but factually incorrect response. Furthermore, the data that the model was trained on might be out of date, which leads to providing inaccurate responses. One way to mitigate LLMs from giving incorrect information is by using a technique known as Retrieval Augmented Generation (RAG). RAG is an advanced natural language processing technique that combines knowledge retrieval with generative text models. RAG combines the powers of pre-trained language models with a retrieval-based approach to generate more informed and accurate responses. To set up RAG, you need to have a vector database to provide your model with related source documents. Using RAG, the relevant document segments or other texts are retrieved and shared with LLMs to generate targeted responses with enhanced content quality and relevance.
Amazon Bedrock is a fully managed service that offers a choice of high-performing foundation models (FMs) from leading AI companies, including AI21 Labs, Anthropic, Cohere, Meta, Mistral AI, Stability AI, and Amazon using a single API, along with a broad set of capabilities you need to build generative AI applications with security, privacy, and responsible AI.
Amazon SageMaker JumpStart provides access to proprietary FMs from third-party providers such as AI21 Labs, Cohere, and LightOn. In addition, Amazon SageMaker JumpStart onboards and maintains open source FMs from third-party sources such as Hugging Face.
Data sources, embeddings, and vector store
Organizations’ domain-specific data, which provides context and relevance, typically resides in internal databases, data lakes, unstructured data repositories, or document stores, collectively referred to as organizational data sources or proprietary data stores.
A vector store is a system you can use to store and query vectors at scale, with efficient nearest neighbor query algorithms and appropriate indexes to improve data retrieval. It includes not only the embeddings of an organization’s data (mathematical representation of data in the form of vectors) but also raw text from the data in chunks. These vectors are generated by specialized embedding LLMs, which process the organization’s text chunks to create numerical representations (vectors), which are stored with the text chunks in the vector store. For a comprehensive read about vector store and embeddings, you can refer to The role of vector databases in generative AI applications.
With Amazon Bedrock Knowledge Bases, you securely connect FMs in Amazon Bedrock to your company data for RAG. Amazon Bedrock Knowledge Bases facilitates data ingestion from various supported data sources; manages data chunking, parsing, and embeddings; and populates the vector store with the embeddings. With all that provided as a service, you can think of Amazon Bedrock Knowledge Bases as a fully managed and serverless option to build powerful conversational AI systems using RAG.
Guardrails
Content filtering mechanisms are implemented as safeguards to control user-AI interactions, aligning with application requirements and responsible AI policies by minimizing undesirable and harmful content. Guardrails can check user inputs and FM outputs and filter or deny topics that are unsafe, redact personally identifiable information (PII), and enhance content safety and privacy in generative AI applications.
Amazon Bedrock Guardrails is a feature of Amazon Bedrock that you can use to put safeguards in place. You determine what qualifies based on your company policies. These safeguards are FM agnostic. You can create multiple guardrails with different configurations tailored to specific use cases. For a review on Amazon Bedrock Guardrails, you can refer to these blog posts: Guardrails for Amazon Bedrock helps implement safeguards customized to your use cases and responsible AI policies and Guardrails for Amazon Bedrock now available with new safety filters and privacy controls.
Operating model architectures
This section provides an overview of the three kinds of operating models.
Decentralized operating model
In a decentralized operating model, LOB teams maintain control and ownership of their AWS accounts. Each LOB configures and orchestrates generative AI components, common functionalities, applications, and Amazon Bedrock configurations within their respective AWS accounts. This model empowers LOBs to tailor their generative AI solutions according to their specific requirements, while taking advantage of the power of Amazon Bedrock.
With this model, the LOBs configure the core components, such as LLMs and guardrails, and the Amazon Bedrock service account manages the hosting, execution, and provisioning of interface endpoints. These endpoints enable LOBs to access and interact with the Amazon Bedrock services they’ve configured.
Each LOB performs monitoring and auditing of their configured Amazon Bedrock services within their account, using Amazon CloudWatch Logs and AWS CloudTrail for log capture, analysis, and auditing tailored to their needs. Amazon Bedrock cost and usage will be recorded in each LOB’s AWS accounts. By adopting this decentralized model, LOBs retain control over their generative AI solutions through a decentralized configuration, while benefiting from the scalability, reliability, and security of Amazon Bedrock.
The following diagram shows the architecture of the decentralized operating model.
Centralized operating model
The centralized AWS account serves as the primary hub for configuring and managing the core generative AI functionalities, including reusable agents, prompt flows, and shared libraries. LOB teams contribute their business-specific requirements and use cases to the centralized team, which then integrates and orchestrates the appropriate generative AI components within the centralized account.
Although the orchestration and configuration of generative AI solutions reside in the centralized account, they often require interaction with LOB-specific resources and services. To facilitate this, the centralized account uses API gateways or other integration points provided by the LOBs’ AWS accounts. These integration points enable secure and controlled communication between the centralized generative AI orchestration and the LOBs’ business-specific applications, data sources, or services. This centralized operating model promotes consistency, governance, and scalability of generative AI solutions across the organization.
The centralized team maintains adherence to common standards, best practices, and organizational policies, while also enabling efficient sharing and reuse of generative AI components. Furthermore, the core components of Amazon Bedrock, such as LLMs and guardrails, continue to be hosted and executed by AWS in the Amazon Bedrock service account, promoting secure, scalable, and high-performance execution environments for these critical components. In this centralized model, monitoring and auditing of Amazon Bedrock can be achieved within the centralized account, allowing for comprehensive monitoring, auditing, and analysis of all generative AI activities and configurations. Amazon CloudWatch Logs provides a unified view of generative AI operations across the organization.
By consolidating the orchestration and configuration of generative AI solutions in a centralized account while enabling secure integration with LOB-specific resources, this operating model promotes standardization, governance, and centralized control over generative AI operations. It uses the scalability, reliability, security, and centralized monitoring capabilities of AWS managed infrastructure and services, while still allowing for integration with LOB-specific requirements and use cases.
The following is the architecture for a centralized operating model.
Federated operating model
In a federated model, Amazon Bedrock enables a collaborative approach where LOB teams can develop and contribute common generative AI functionalities within their respective AWS accounts. These common functionalities, such as reusable agents, prompt flows, or shared libraries, can then be migrated to a centralized AWS account managed by a dedicated team or CCoE.
The centralized AWS account acts as a hub for integrating and orchestrating these common generative AI components, providing a unified platform for action groups and prompt flows. Although the orchestration and configuration of generative AI solutions remain within the LOBs’ AWS accounts, they can use the centralized Amazon Bedrock agents, prompt flows, and other shared components defined in the centralized account.
This federated model allows LOBs to retain control over their generative AI solutions, tailoring them to specific business requirements while benefiting from the reusable and centrally managed components. The centralized account maintains consistency, governance, and scalability of these shared generative AI components, promoting collaboration and standardization across the organization.
Organizations frequently prefer storing sensitive data, including Payment Card Industry (PCI), PII, General Data Protection Regulation (GDPR), and Health Insurance Portability and Accountability Act (HIPAA) information, within their respective LOB AWS accounts. This approach makes sure that LOBs maintain control over their sensitive business data in the vector store while preventing centralized teams from accessing it without proper governance and security measures.
A federated model combines decentralized development, centralized integration, and centralized monitoring. This operating model fosters collaboration, reusability, and standardization while empowering LOBs to retain control over their generative AI solutions. It uses the scalability, reliability, security, and centralized monitoring capabilities of AWS managed infrastructure and services, promoting a harmonious balance between autonomy and governance.
The following is the architecture for a federated operating model.
Cost management
Organizations may want to analyze Amazon Bedrock usage and costs per LOB. To track the cost and usage of FMs across LOBs’ AWS accounts, solutions that record model invocations per LOB can be implemented.
Amazon Bedrock now supports model invocation resources that use inference profiles. Inference profiles can be defined to track Amazon Bedrock usage metrics, monitor model invocation requests, or route model invocation requests to multiple AWS Regions for increased throughput.
There are two types of inference profiles. Cross-Region inference profiles, which are predefined in Amazon Bedrock and include multiple AWS Regions to which requests for a model can be routed. The other is application inference profiles, which are user created to track cost and model usage when submitting on-demand model invocation requests. You can attach custom tags, such as cost allocation tags, to your application inference profiles. When submitting a prompt, you can include an inference profile ID or its Amazon Resource Name (ARN). This capability enables organizations to track and monitor costs for various LOBs, cost centers, or applications. For a detailed explanation of application inference profiles refer to this post: Track, allocate, and manage your generative AI cost and usage with Amazon Bedrock.
Conclusion
Although enterprises often begin with a centralized operating model, the rapid pace of development in generative AI technologies, the need for agility, and the desire to quickly capture value often lead organizations to converge on a federated operating model.
In a federated operating model, lines of business have the freedom to innovate and experiment with generative AI solutions, taking advantage of their domain expertise and proximity to business problems. Key aspects of the AI workflow, such as data access policies, model risk management, and compliance monitoring, are managed by a central cloud governance team. Successful generative AI solutions developed by a line of business can be promoted and productionized by the central team for enterprise-wide re-use.
This federated model fosters innovation from the lines of business closest to domain problems. Simultaneously, it allows the central team to curate, harden, and scale those solutions adherent to organizational policies, then redeploy them efficiently to other relevant areas of the business.
To sustain this operating model, enterprises often establish a dedicated product team with a business owner that works in partnership with lines of business. This team is responsible for continually evolving the operating model, refactoring and enhancing the generative AI services to help meet the changing needs of the lines of business and keep up with the rapid advancements in LLMs and other generative AI technologies.
Federated operating models strike a balance, mitigating the risks of fully decentralized initiatives while minimizing bottlenecks from overly centralized approaches. By empowering business agility with curation by a central team, enterprises can accelerate compliant, high-quality generative AI capabilities aligned with their innovation goals, risk tolerances, and need for rapid value delivery in the evolving AI landscape.
As enterprises look to capitalize on the generative AI revolution, Amazon Bedrock provides the ideal foundation to establish a flexible operating model tailored to their organization’s needs. Whether you’re starting with a centralized, decentralized, or federated approach, AWS offers a comprehensive suite of services to support the full generative AI lifecycle.
Try Amazon Bedrock and let us know your feedback on how you’re planning to implement the operating model that suits your organization.
About the Authors
Martin Tunstall is a Principal Solutions Architect at AWS. With over three decades of experience in the finance sector, he helps global finance and insurance customers unlock the full potential of Amazon Web Services (AWS).
Yashar Araghi is a Senior Solutions Architect at AWS. He has over 20 years of experience designing and building infrastructure and application security solutions. He has worked with customers across various industries such as government, education, finance, energy, and utilities. In the last 6 years at AWS, Yashar has helped customers design, build, and operate their cloud solutions that are secure, reliable, performant and cost optimized in the AWS Cloud.
Leveling Up User Experiences With Agentic AI, From Bots to Autonomous Agents
AI agents with advanced perception and cognition capabilities are making digital experiences more dynamic and personalized across retail, finance, entertainment and other industries.
In this episode of the NVIDIA AI Podcast, Chris Covert, director of product experiences at Inworld AI, highlights how intelligent digital humans and characters are reshaping interactive experiences, from gaming to healthcare.
With expertise on the intersection of autonomous systems and human-centered design, Covert explains the different stages of AI agents — from basic conversational interfaces to fully autonomous systems. He emphasizes that the key to developing meaningful AI experiences is focusing on user value rather than technology alone.
In addition, Covert discusses how livestreaming and recording software company Streamlabs announced a collaboration with Inworld and NVIDIA at this year’s CES trade show, unveiling an AI-powered streaming assistant that can provide real-time commentary, clip gameplay moments and interact dynamically with streamers thanks to NVIDIA ACE integrations.
Learn more about the latest advancements in agentic AI and other technologies by registering for NVIDIA GTC, the conference for the era of AI, taking place March 17-21 at the San Jose Convention Center.
Time Stamps
5:34 — The definition of digital humans and their current state in industries.
10:30 — The evolution of AI agents.
18:10 — The design philosophy behind building digital humans and why teams should start with a “moonshot” approach.
You Might Also Like…
How World Foundation Models Will Advance Physical AI
World foundation models are powerful neural networks that can simulate and predict outcomes in physical environments, enabling teams to enhance AI workflows and development. Ming-Yu Liu, vice president of research at NVIDIA and an IEEE Fellow, joined the NVIDIA AI Podcast to discuss how world foundation models will impact various industries.
How Roblox Uses Generative AI to Enhance User Experiences
Roblox is a colorful online platform that aims to reimagine the way that people come together. Now, generative AI is augmenting that vision. Anupam Singh, vice president of AI and growth engineering at Roblox, explains how the company uses the technology to enhance virtual experiences, power coding assistants to help creators, and increase inclusivity and user safety.
Exploring AI-Powered Filmmaking With Cuebric’s Pinar Seyhan Demirdag
Cuebric is on a mission to offer new solutions in filmmaking and content creation through immersive, two-and-a-half-dimensional cinematic environments. The company’s AI-powered application aims to help creators quickly bring their ideas to life, making high-quality production more accessible. Pinar Seyhan Demirdag, cofounder and CEO of Cuebric, talks about the current landscape of content creation and the role of AI in simplifying the creative process.