In the rapidly evolving landscape of artificial intelligence, Retrieval Augmented Generation (RAG) has emerged as a game-changer, revolutionizing how Foundation Models (FMs) interact with organization-specific data. As businesses increasingly rely on AI-powered solutions, the need for accurate, context-aware, and tailored responses has never been more critical.
Enter the powerful trio of Amazon Bedrock, LlamaIndex, and RAGAS– a cutting-edge combination that’s set to redefine the evaluation and optimization of RAG responses. This blog post delves into how these innovative tools synergize to elevate the performance of your AI applications, ensuring they not only meet but exceed the exacting standards of enterprise-level deployments.
Whether you’re a seasoned AI practitioner or a business leader exploring the potential of generative AI, this guide will equip you with the knowledge and tools to:
- Harness the full potential of Amazon Bedrock robust foundation models
- Utilize RAGAS’s comprehensive evaluation metrics for RAG systems
In this post, we’ll explore how to leverage Amazon Bedrock, LlamaIndex, and RAGAS to enhance your RAG implementations. You’ll learn practical techniques to evaluate and optimize your AI systems, enabling more accurate, context-aware responses that align with your organization’s specific needs. Let’s dive in and discover how these powerful tools can help you build more effective and reliable AI-powered solutions.
RAG Evaluation
RAG evaluation is important to ensure that RAG models produce accurate, coherent, and relevant responses. By analyzing the retrieval and generator components both jointly and independently, RAG evaluation helps identify bottlenecks, monitor performance, and improve the overall system. Current RAG pipelines frequently employ similarity-based metrics such as ROUGE, BLEU, and BERTScore to assess the quality of the generated responses, which is essential for refining and enhancing the model’s capabilities.
Above mentioned probabilistic metrics ROUGE, BLEU, and BERTScore have limitations in assessing relevance and detecting hallucinations. More sophisticated metrics are needed to evaluate factual alignment and accuracy.
Evaluate RAG components with Foundation models
We can also use a Foundation Model as a judge to compute various metrics for both retrieval and generation. Here are some examples of these metrics:
- Retrieval component
- Context precision – Evaluates whether all of the ground-truth relevant items present in the contexts are ranked higher or not.
- Context recall – Ensures that the context contains all relevant information needed to answer the question.
- Generator component
- Faithfulness – Verifies that the generated answer is factually accurate based on the provided context, helping to identify errors or “hallucinations.”
- Answer relavancy : Measures how well the answer matches the question. Higher scores mean the answer is complete and relevant, while lower scores indicate missing or redundant information.
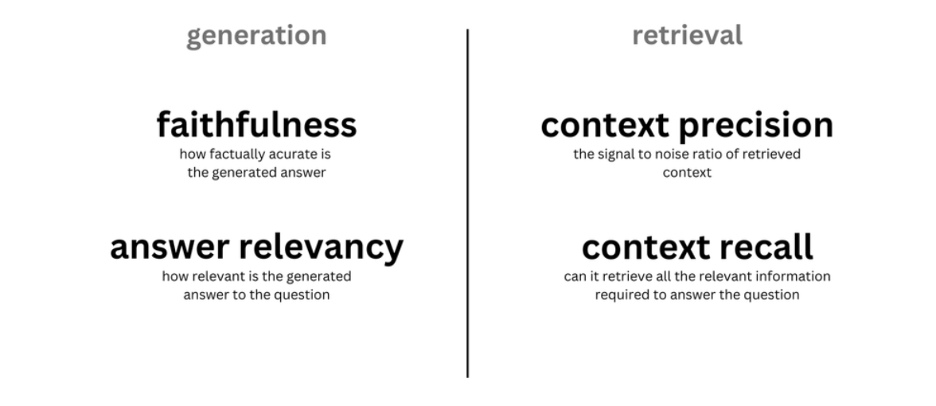
Overview of solution
This post guides you through the process of assessing quality of RAG response with evaluation framework such as RAGAS and LlamaIndex with Amazon Bedrock.
In this post, we are also going to leverage Langchain to create a sample RAG application.
Amazon Bedrock is a fully managed service that offers a choice of high-performing Foundation Models (FMs) from leading AI companies like AI21 Labs, Anthropic, Cohere, Meta, Stability AI, and Amazon via a single API, along with a broad set of capabilities you need to build generative AI applications with security, privacy, and responsible AI.
The Retrieval Augmented Generation Assessment (RAGAS) framework offers multiple metrics to evaluate each part of the RAG system pipeline, identifying areas for improvement. It utilizes foundation models to test individual components, aiding in pinpointing modules for development to enhance overall results.
LlamaIndex is a framework for building LLM applications. It simplifies data integration from various sources and provides tools for data indexing, engines, agents, and application integrations. Optimized for search and retrieval, it streamlines querying LLMs and retrieving documents. This blog post focuses on using its Observability/Evaluation modules.
LangChain is an open-source framework that simplifies the creation of applications powered by foundation models. It provides tools for chaining LLM operations, managing context, and integrating external data sources. LangChain is primarily used for building chatbots, question-answering systems, and other AI-driven applications that require complex language processing capabilities.
Diagram Architecture
The following diagram is a high-level reference architecture that explains how you can evaluate the RAG solution with RAGAS or LlamaIndex.
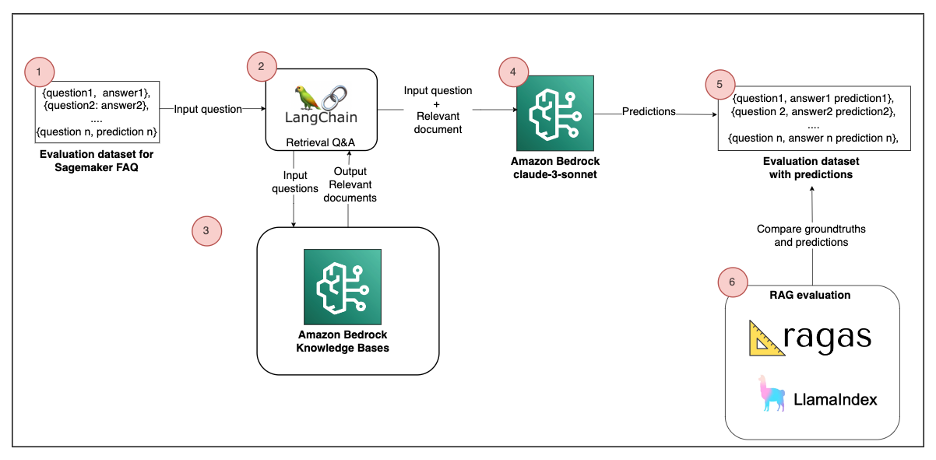
The solution consists of the following components:
- Evaluation dataset – The source data for the RAG comes from the Amazon SageMaker FAQ, which represents 170 question-answer pairs. This corresponds to Step 1 in the architecture diagram.
- Build sample RAG – Documents are segmented into chunks and stored in an Amazon Bedrock Knowledge Bases (Steps 2–4). We use Langchain Retrieval Q&A to answer user queries. This process retrieves relevant data from an index at runtime and passes it to the Foundation Model (FM).
- RAG evaluation – To assess the quality of the Retrieval-Augmented Generation (RAG) solution, we can use both RAGAS and LlamaIndex. An LLM performs the evaluation by comparing its predictions with ground truths (Steps 5–6).
You must follow the provided notebook to reproduce the solution. We elaborate on the main code components in this post.
Prerequisites
To implement this solution, you need the following:
- An AWS accountwith privileges to create AWS Identity and Access Management (IAM) roles and policies. For more information, see Overview of access management: Permissions and policies.
- Access enabled for the Amazon Titan Embeddings G1 – Text model and Anthropic Claude 3 Sonnet on Amazon Bedrock. For instructions, see Model access.
- Run the prerequisite code provided in the Python
Ingest FAQ data
The first step is to ingest the SageMaker FAQ data. For this purpose, LangChain provides a WebBaseLoader object to load text from HTML webpages into a document format. Then we split each document in multiple chunks of 2,000 tokens with a 100-token overlap. See the following code below:
text_chunks = split_document_from_url(SAGEMAKER_URL, chunck_size= 2000, chunk_overlap=100)
retriever_db= get_retriever(text_chunks, bedrock_embeddings)
Set up embeddings and LLM with Amazon Bedrock and LangChain
In order to build a sample RAG application, we need an LLM and an embedding model:
- LLM – Anthropic Claude 3 Sonnet
- Embedding – Amazon Titan Embeddings – Text V2
This code sets up a LangChain application using Amazon Bedrock, configuring embeddings with Titan and a Claude 3 Sonnet model for text generation with specific parameters for controlling the model’s output. See the following code below from the notebook :
from botocore.client import Config
from langchain.llms.bedrock import Bedrock
from langchain_aws import ChatBedrock
from langchain.embeddings import BedrockEmbeddings
from langchain.retrievers.bedrock import AmazonKnowledgeBasesRetriever
from langchain.chains import RetrievalQA
import nest_asyncio
nest_asyncio.apply()
#URL to fetch the document
SAGEMAKER_URL="https://aws.amazon.com/sagemaker/faqs/"
#Bedrock parameters
EMBEDDING_MODEL="amazon.titan-embed-text-v2:0"
BEDROCK_MODEL_ID="anthropic.claude-3-sonnet-20240229-v1:0"
bedrock_embeddings = BedrockEmbeddings(model_id=EMBEDDING_MODEL,client=bedrock_client)
model_kwargs = {
"temperature": 0,
"top_k": 250,
"top_p": 1,
"stop_sequences": ["\n\nHuman:"]
}
llm_bedrock = ChatBedrock(
model_id=BEDROCK_MODEL_ID,
model_kwargs=model_kwargs
)
Set up Knowledge Bases
We will create Amazon Bedrock knowledgebases Web Crawler datasource and process Sagemaker FAQ data.
In the code below, we load the embedded documents in Knowledge bases and we set up the retriever with LangChain:
from utils import split_document_from_url, get_bedrock_retriever
from botocore.exceptions import ClientError
text_chunks = split_document_from_url(SAGEMAKER_URL, chunck_size= 2000, chunk_overlap=100)
retriever_db= get_bedrock_retriever(text_chunks, region)
Build a Q&A chain to query the retrieval API
After the database is populated, create a Q&A retrieval chain to perform question answering with context extracted from the vector store. You also define a prompt template following Claude prompt engineering guidelines. See the following code below from the notebook:
from langchain_core.prompts import ChatPromptTemplate
from langchain.chains.combine_documents import create_stuff_documents_chain
from langchain.chains import create_retrieval_chain
system_prompt = (
"Use the given context to answer the question. "
"If you don't know the answer, say you don't know. "
"Use three sentence maximum and keep the answer concise and short. "
"Context: {context}"
)
prompt_template = ChatPromptTemplate.from_messages([
("system", system_prompt),
("human", "{input}")
]
)
question_answer_chain = create_stuff_documents_chain(llm_bedrock, prompt_template)
chain = create_retrieval_chain(retriever_db, question_answer_chain)
Build Dataset to evaluate RAG application
To evaluate a RAG application, we need a combination of the following datasets:
- Questions – The user query that serves as input to the RAG pipeline
- Context – The information retrieved from enterprise or external data sources based on the provided query
- Answers – The responses generated by LLMs
- Ground truths – Human-annotated, ideal responses for the questions that can be used as the benchmark to compare against the LLM-generated answers
We are ready to evaluate the RAG application. As describe in the introduction, we select 3 metrics to assess our RAG solution:
- Faithfulness
- Answer Relevancy
- Answer Correctness
For more information, refer to Metrics.
This step involves defining an evaluation dataset with a set of ground truth questions and answers. For this post, we choose four random questions from the SageMaker FAQ. See the following code below from the notebook:
EVAL_QUESTIONS = [
"Can I stop a SageMaker Autopilot job manually?",
"Do I get charged separately for each notebook created and run in SageMaker Studio?",
"Do I get charged for creating and setting up an SageMaker Studio domain?",
"Will my data be used or shared to update the base model that is offered to customers using SageMaker JumpStart?",
]
#Defining the ground truth answers for each question
EVAL_ANSWERS = [
"Yes. You can stop a job at any time. When a SageMaker Autopilot job is stopped, all ongoing trials will be stopped and no new trial will be started.",
"""No. You can create and run multiple notebooks on the same compute instance.
You pay only for the compute that you use, not for individual items.
You can read more about this in our metering guide.
In addition to the notebooks, you can also start and run terminals and interactive shells in SageMaker Studio, all on the same compute instance.""",
"No, you don’t get charged for creating or configuring an SageMaker Studio domain, including adding, updating, and deleting user profiles.",
"No. Your inference and training data will not be used nor shared to update or train the base model that SageMaker JumpStart surfaces to customers."
]
Evaluation of RAG with RAGAS
Evaluating the RAG solution requires to compare LLM predictions with ground truth answers. To do so, we use the batch() function from LangChain to perform inference on all questions inside our evaluation dataset.
Then we can use the evaluate() function from RAGAS to perform evaluation on each metric (answer relevancy, faithfulness and answer corectness). It uses an LLM to compute metrics. Feel free to use other Metrics from RAGAS.
See the following code below from the notebook:
from ragas.metrics import answer_relevancy, faithfulness, answer_correctness
from ragas import evaluate
#Batch invoke and dataset creation
result_batch_questions = chain.batch([{"input": q} for q in EVAL_QUESTIONS])
dataset= build_dataset(EVAL_QUESTIONS,EVAL_ANSWERS,result_batch_questions, text_chunks)
result = evaluate(dataset=dataset, metrics=[ answer_relevancy, faithfulness, answer_correctness ],llm=llm_bedrock, embeddings=bedrock_embeddings, raise_exceptions=False )
df = result.to_pandas()
df.head()
The following screenshot shows the evaluation results and the RAGAS answer relevancy score.
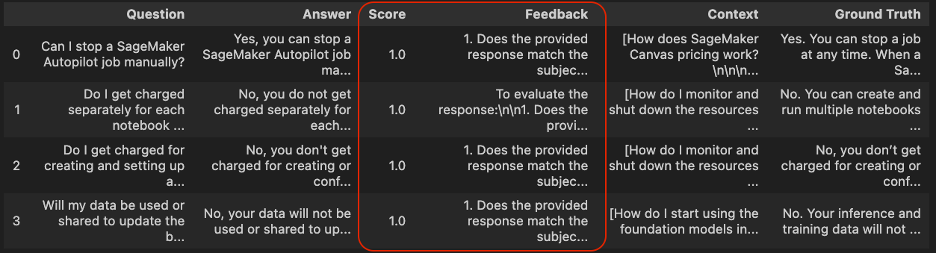
Answer Relevancy
In the answer_relevancy_score
column, a score closer to 1 indicates the response generated is relevant to the input query.
Faithfulness
In the second column, the first query result has a lower faithfulness_score
(0.2), which indicates the responses are not derived from the context and are hallucinations. The rest of the query results have a higher faithfulness_score
(1.0), which indicates the responses are derived from the context.
Answer Correctness
In the last column answer_correctness
, the second and last row have high answer correctness, meaning that answer provided by the LLM is closer to to from the groundtruth.
Evaluation of RAG with LlamaIndex
LlamaIndex, similar to Ragas, provides a comprehensive RAG (Retrieval-Augmented Generation) evaluation module. This module offers a variety of metrics to assess the performance of your RAG system. The evaluation process generates two key outputs:
- Feedback: The judge LLM (Language Model) provides detailed evaluation feedback in the form of a string, offering qualitative insights into the system’s performance.
- Score: This numerical value indicates how well the answer meets the evaluation criteria. The scoring system varies depending on the specific metric being evaluated. For example, metrics like Answer Relevancy and Faithfulness are typically scored on a scale from 0 to 1.
These outputs allow for both qualitative and quantitative assessment of your RAG system’s performance, enabling you to identify areas for improvement and track progress over time.
The following is a code sample from the notebook:
from llama_index.llms.bedrock import Bedrock
from llama_index.core.evaluation import (
AnswerRelevancyEvaluator,
CorrectnessEvaluator,
FaithfulnessEvaluator
)
from utils import evaluate_llama_index_metric
bedrock_llm_llama = Bedrock(model=BEDROCK_MODEL_ID)
faithfulness= FaithfulnessEvaluator(llm=bedrock_llm_llama)
answer_relevancy= AnswerRelevancyEvaluator(llm=bedrock_llm_llama)
correctness= CorrectnessEvaluator(llm=bedrock_llm_llama)
Answer Relevancy
df_answer_relevancy= evaluate_llama_index_metric(answer_relevancy, dataset)
df_answer_relevancy.head()
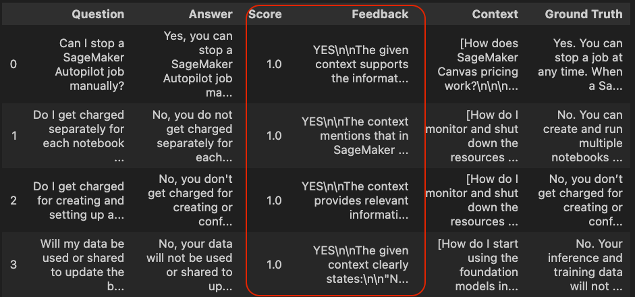
The column Score defines the result for the answer_relevancy
evaluation criteria. All passing values are set to 1, meaning that all predictions are relevant with the context retrieved.
Additionally, the column Feedback provides a clear explanation of the result of the passing score. We can observe that all answers align with the context extracted from the retriever.
Answer Correctness
df_correctness= evaluate_llama_index_metric(correctness, dataset)
df_correctness.head()
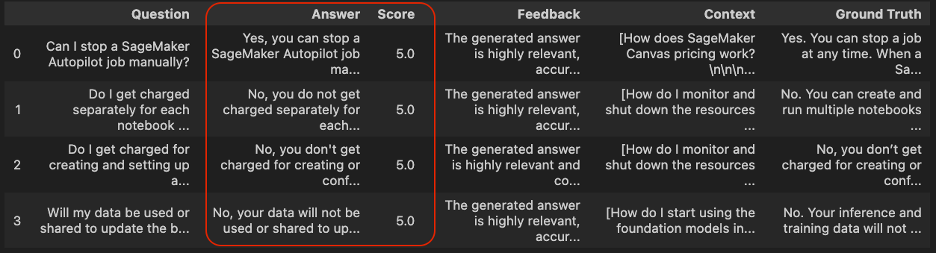
All values from the column Score are set to 5.0, meaning that all predictions are coherent with ground truth answers.
Faithfulness
The following screenshot shows the evaluation results for answer faithfulness.
df_faithfulness= evaluate_llama_index_metric(faithfulness, dataset)
df_faithfulness.head()
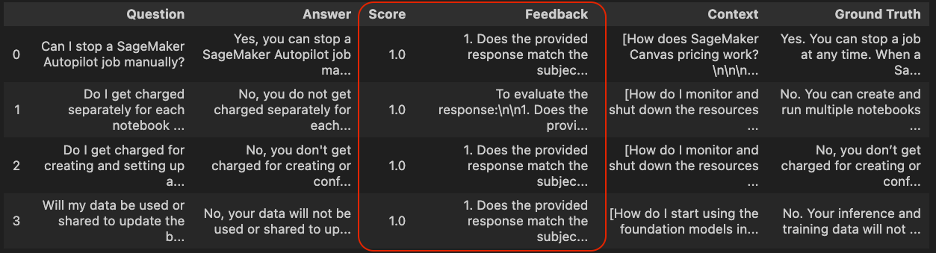
All values from the Score column are set to 1.0, which means all answers generated by LLM are coherent given the context retrieved.
Conclusion
While Foundation Models offer impressive generative capabilities, their effectiveness in addressing organization-specific queries has been a persistent challenge. The Retrieval Augmented Generation framework emerges as a powerful solution, bridging this gap by enabling LLMs to leverage external, organization-specific data sources.
To truly unlock the potential of RAG pipelines, the RAGAS framework, in conjunction with LlamaIndex, provides a comprehensive evaluation solution. By meticulously assessing both retrieval and generation components, this approach empowers organizations to pinpoint areas for improvement and refine their RAG implementations. The result? Responses that are not only factually accurate but also highly relevant to user queries.
By adopting this holistic evaluation approach, enterprises can fully harness the transformative power of generative AI applications. This not only maximizes the value derived from these technologies but also paves the way for more intelligent, context-aware, and reliable AI systems that can truly understand and address an organization’s unique needs.
As we continue to push the boundaries of what’s possible with AI, tools like Amazon Bedrock, LlamaIndex, and RAGAS will play a pivotal role in shaping the future of enterprise AI applications. By embracing these innovations, organizations can confidently navigate the exciting frontier of generative AI, unlocking new levels of efficiency, insight, and competitive advantage.
For further exploration, readers interested in enhancing the reliability of AI-generated content may want to look into Amazon Bedrock’s Guardrails feature, which offers additional tools like the Contextual Grounding Check.
About the authors
Madhu is a Senior Partner Solutions Architect specializing in worldwide public sector cybersecurity partners. With over 20 years in software design and development, he collaborates with AWS partners to ensure customers implement solutions that meet strict compliance and security objectives. His expertise lies in building scalable, highly available, secure, and resilient applications for diverse enterprise needs.
Babu Kariyaden Parambath is a Senior AI/ML Specialist at AWS. At AWS, he enjoys working with customers in helping them identify the right business use case with business value and solve it using AWS AI/ML solutions and services. Prior to joining AWS, Babu was an AI evangelist with 20 years of diverse industry experience delivering AI driven business value for customers.
Read More