Data augmentation is crucial to make machine learning models more robust and safe. However, augmenting data can be challenging as it requires generating diverse data points to rigorously evaluate model behavior on edge cases and mitigate potential harms. Creating high-quality augmentations that cover these “unknown unknowns” is a time- and creativity-intensive task. In this work, we introduce Amplio, an interactive tool to help practitioners navigate “unknown unknowns” in unstructured text datasets and improve data diversity by systematically identifying empty data spaces to explore. Amplio…Apple Machine Learning Research
Gemini 2.5: Our most intelligent AI model
Gemini 2.5 is our most intelligent AI model, now with thinking built in.Read More
NVIDIA NIM Microservices Now Available to Streamline Agentic Workflows on RTX AI PCs and Workstations
Generative AI is unlocking new capabilities for PCs and workstations, including game assistants, enhanced content-creation and productivity tools and more.
NVIDIA NIM microservices, available now, and AI Blueprints, coming in April, accelerate AI development and improve its accessibility. Announced at the CES trade show in January, NVIDIA NIM provides prepackaged, state-of-the-art AI models optimized for the NVIDIA RTX platform, including the NVIDIA GeForce RTX 50 Series and, now, the new NVIDIA Blackwell RTX PRO GPUs. The microservices are easy to download and run. They span the top modalities for PC development and are compatible with top ecosystem applications and tools.
The experimental System Assistant feature of Project G-Assist was also released today. Project G-Assist showcases how AI assistants can enhance apps and games. The System Assistant allows users to run real-time diagnostics, get recommendations on performance optimizations, or control system software and peripherals — all via simple voice or text commands. Developers and enthusiasts can extend its capabilities with a simple plug-in architecture and new plug-in builder.
Amid a pivotal moment in computing — where groundbreaking AI models and a global developer community are driving an explosion in AI-powered tools and workflows — NIM microservices, AI Blueprints and G-Assist are helping bring key innovations to PCs. This RTX AI Garage blog series will continue to deliver updates, insights and resources to help developers and enthusiasts build the next wave of AI on RTX AI PCs and workstations.
Ready, Set, NIM!
Though the pace of innovation with AI is incredible, it can still be difficult for the PC developer community to get started with the technology.
Bringing AI models from research to the PC requires curation of model variants, adaptation to manage all of the input and output data, and quantization to optimize resource usage. In addition, models must be converted to work with optimized inference backend software and connected to new AI application programming interfaces (APIs). This takes substantial effort, which can slow AI adoption.
NVIDIA NIM microservices help solve this issue by providing prepackaged, optimized, easily downloadable AI models that connect to industry-standard APIs. They’re optimized for performance on RTX AI PCs and workstations, and include the top AI models from the community, as well as models developed by NVIDIA.
NIM microservices support a range of AI applications, including large language models (LLMs), vision language models, image generation, speech processing, retrieval-augmented generation (RAG)-based search, PDF extraction and computer vision. Ten NIM microservices for RTX are available, supporting a range of applications, including language and image generation, computer vision, speech AI and more. Get started with these NIM microservices today:
- Language and Reasoning: Deepseek-R1-distill-llama-8B, Mistral-nemo-12B-instruct, Llama3.1-8B-instruct
- Image Generation: Flux.dev
- Audio: Riva Parakeet-ctc-0.6B-asr, Maxine Studio Voice
- RAG: Llama-3.2-NV-EmbedQA-1B-v2
- Computer Vision and Understanding: NV-CLIP, PaddleOCR, Yolo-X-v1
NIM microservices are also available through top AI ecosystem tools and frameworks.
For AI enthusiasts, AnythingLLM and ChatRTX now support NIM, making it easy to chat with LLMs and AI agents through a simple, user-friendly interface. With these tools, users can create personalized AI assistants and integrate their own documents and data, helping automate tasks and enhance productivity.
For developers looking to build, test and integrate AI into their applications, FlowiseAI and Langflow now support NIM and offer low- and no-code solutions with visual interfaces to design AI workflows with minimal coding expertise. Support for ComfyUI is coming soon. With these tools, developers can easily create complex AI applications like chatbots, image generators and data analysis systems.
In addition, Microsoft VS Code AI Toolkit, CrewAI and Langchain now support NIM and provide advanced capabilities for integrating the microservices into application code, helping ensure seamless integration and optimization.
Visit the NVIDIA technical blog and build.nvidia.com to get started.
NVIDIA AI Blueprints Will Offer Pre-Built Workflows
NVIDIA AI Blueprints, coming in April, give AI developers a head start in building generative AI workflows with NVIDIA NIM microservices.
Blueprints are ready-to-use, extensible reference samples that bundle everything needed — source code, sample data, documentation and a demo app — to create and customize advanced AI workflows that run locally. Developers can modify and extend AI Blueprints to tweak their behavior, use different models or implement completely new functionality.
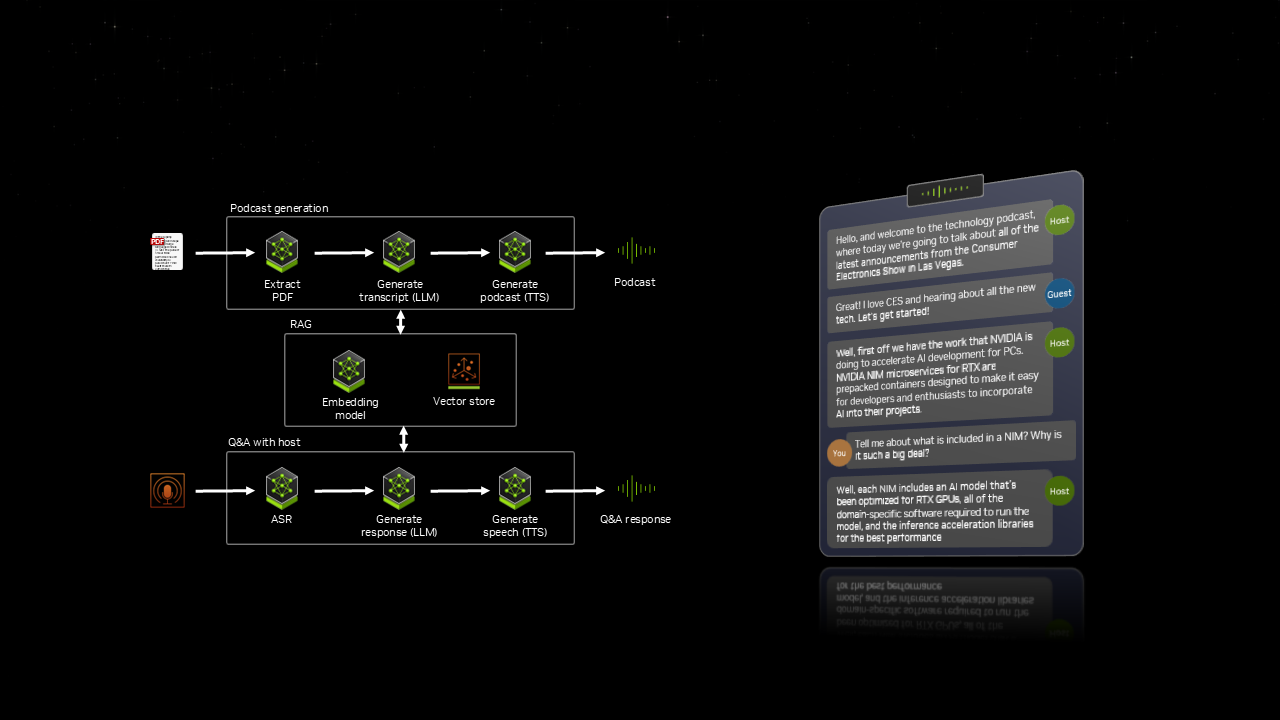
The PDF to podcast AI Blueprint will transform documents into audio content so users can learn on the go. By extracting text, images and tables from a PDF, the workflow uses AI to generate an informative podcast. For deeper dives into topics, users can then have an interactive discussion with the AI-powered podcast hosts.
The AI Blueprint for 3D-guided generative AI will give artists finer control over image generation. While AI can generate amazing images from simple text prompts, controlling image composition using only words can be challenging. With this blueprint, creators can use simple 3D objects laid out in a 3D renderer like Blender to guide AI image generation. The artist can create 3D assets by hand or generate them using AI, place them in the scene and set the 3D viewport camera. Then, a prepackaged workflow powered by the FLUX NIM microservice will use the current composition to generate high-quality images that match the 3D scene.
NVIDIA NIM on RTX With Windows Subsystem for Linux
One of the key technologies that enables NIM microservices to run on PCs is Windows Subsystem for Linux (WSL).
Microsoft and NVIDIA collaborated to bring CUDA and RTX acceleration to WSL, making it possible to run optimized, containerized microservices on Windows. This allows the same NIM microservice to run anywhere, from PCs and workstations to the data center and cloud.
Get started with NVIDIA NIM on RTX AI PCs at build.nvidia.com.
Project G-Assist Expands PC AI Features With Custom Plug-Ins
As part of Project G-Assist, an experimental version of the System Assistant feature for GeForce RTX desktop users is now available via the NVIDIA App, with laptop support coming soon.
G-Assist helps users control a broad range of PC settings — including optimizing game and system settings, charting frame rates and other key performance statistics, and controlling select peripherals settings such as lighting — all via basic voice or text commands.
G-Assist is built on NVIDIA ACE — the same AI technology suite game developers use to breathe life into non-player characters. Unlike AI tools that use massive cloud-hosted AI models that require online access and paid subscriptions, G-Assist runs locally on a GeForce RTX GPU. This means it’s responsive, free and can run without an internet connection. Manufacturers and software providers are already using ACE to create custom AI Assistants like G-Assist, including MSI’s AI Robot engine, the Streamlabs Intelligent AI Assistant and upcoming capabilities in HP’s Omen Gaming hub.
G-Assist was built for community-driven expansion. Get started with this NVIDIA GitHub repository, including samples and instructions for creating plug-ins that add new functionality. Developers can define functions in simple JSON formats and drop configuration files into a designated directory, allowing G-Assist to automatically load and interpret them. Developers can even submit plug-ins to NVIDIA for review and potential inclusion.
Currently available sample plug-ins include Spotify, to enable hands-free music and volume control, and Google Gemini — allowing G-Assist to invoke a much larger cloud-based AI for more complex conversations, brainstorming sessions and web searches using a free Google AI Studio API key.
In the clip below, you’ll see G-Assist ask Gemini about which Legend to pick in Apex Legends when solo queueing, and whether it’s wise to jump into Nightmare mode at level 25 in Diablo IV:
For even more customization, follow the instructions in the GitHub repository to generate G-Assist plug-ins using a ChatGPT-based “Plug-in Builder.” With this tool, users can write and export code, then integrate it into G-Assist — enabling quick, AI-assisted functionality that responds to text and voice commands.
Watch how a developer used the Plug-in Builder to create a Twitch plug-in for G-Assist to check if a streamer is live:
More details on how to build, share and load plug-ins are available in the NVIDIA GitHub repository.
Check out the G-Assist article for system requirements and additional information.
Build, Create, Innovate
NVIDIA NIM microservices for RTX are available at build.nvidia.com, providing developers and AI enthusiasts with powerful, ready-to-use tools for building AI applications.
Download Project G-Assist through the NVIDIA App’s “Home” tab, in the “Discovery” section. G-Assist currently supports GeForce RTX desktop GPUs, as well as a variety of voice and text commands in the English language. Future updates will add support for GeForce RTX Laptop GPUs, new and enhanced G-Assist capabilities, as well as support for additional languages. Press “Alt+G” after installation to activate G-Assist.
Each week, RTX AI Garage features community-driven AI innovations and content for those looking to learn more about NIM microservices and AI Blueprints, as well as building AI agents, creative workflows, digital humans, productivity apps and more on AI PCs and workstations.
Plug in to NVIDIA AI PC on Facebook, Instagram, TikTok and X — and stay informed by subscribing to the RTX AI PC newsletter.
Follow NVIDIA Workstation on LinkedIn and X.
See notice regarding software product information.
Scaling Up Reinforcement Learning for Traffic Smoothing: A 100-AV Highway Deployment
We deployed 100 reinforcement learning (RL)-controlled cars into rush-hour highway traffic to smooth congestion and reduce fuel consumption for everyone. Our goal is to tackle “stop-and-go” waves, those frustrating slowdowns and speedups that usually have no clear cause but lead to congestion and significant energy waste. To train efficient flow-smoothing controllers, we built fast, data-driven simulations that RL agents interact with, learning to maximize energy efficiency while maintaining throughput and operating safely around human drivers.
Overall, a small proportion of well-controlled autonomous vehicles (AVs) is enough to significantly improve traffic flow and fuel efficiency for all drivers on the road. Moreover, the trained controllers are designed to be deployable on most modern vehicles, operating in a decentralized manner and relying on standard radar sensors. In our latest paper, we explore the challenges of deploying RL controllers on a large-scale, from simulation to the field, during this 100-car experiment.
Fundamental Challenges in Evaluating Text2SQL Solutions and Detecting Their Limitations
In this work, we dive into the fundamental challenges of evaluating Text2SQL solutions and highlight potential failure causes and the potential risks of relying on aggregate metrics in existing benchmarks. We identify two largely unaddressed limitations in current open benchmarks: (1) data quality issues in the evaluation data mainly attributed to the lack of capturing the probabilistic nature of translating a natural language description into a structured query (e.g., NL ambiguity), and (2) the bias that using different match functions as approximations for SQL equivalence can introduce.
To…Apple Machine Learning Research
UniVG: A Generalist Diffusion Model for Unified Image Generation and Editing
Text-to-Image (T2I) diffusion models have shown impressive results in generating visually compelling images following user prompts. Building on this, various methods further fine-tune the pre-trained T2I model for specific tasks. However, this requires separate model architectures, training designs, and multiple parameter sets to handle different tasks. In this paper, we introduce UniVG, a generalist diffusion model capable of supporting a diverse range of image generation tasks with a single set of weights. UniVG treats multi-modal inputs as unified conditions to enable various downstream…Apple Machine Learning Research
Build a generative AI enabled virtual IT troubleshooting assistant using Amazon Q Business
Today’s organizations face a critical challenge with the fragmentation of vital information across multiple environments. As businesses increasingly rely on diverse project management and IT service management (ITSM) tools such as ServiceNow, Atlassian Jira and Confluence, employees find themselves navigating a complex web of systems to access crucial data.
This isolated approach leads to several challenges for IT leaders, developers, program managers, and new employees. For example:
- Inefficiency: Employees need to access multiple systems independently to gather data insights and remediation steps during incident troubleshooting
- Lack of integration: Information is isolated across different environments, making it difficult to get a holistic view of ITSM activities
- Time-consuming: Searching for relevant information across multiple systems is time-consuming and reduces productivity
- Potential for inconsistency: Using multiple systems increases the risk of inconsistent data and processes across the organization.
Amazon Q Business is a fully managed, generative artificial intelligence (AI) powered assistant that can address challenges such as inefficient, inconsistent information access within an organization by providing 24/7 support tailored to individual needs. It handles a wide range of tasks such as answering questions, providing summaries, generating content, and completing tasks based on data in your organization. Amazon Q Business offers over 40 data source connectors that connect to your enterprise data sources and help you create a generative AI solution with minimal configuration. Amazon Q Business also supports over 50 actions across popular business applications and platforms. Additionally, Amazon Q Business offers enterprise-grade data security, privacy, and built-in guardrails that you can configure.
This blog post explores an innovative solution that harnesses the power of generative AI to bring value to your organization and ITSM tools with Amazon Q Business.
Solution overview
The solution architecture shown in the following figure demonstrates how to build a virtual IT troubleshooting assistant by integrating with multiple data sources such as Atlassian Jira, Confluence, and ServiceNow. This solution helps streamline information retrieval, enhance collaboration, and significantly boost overall operational efficiency, offering a glimpse into the future of intelligent enterprise information management.
This solution integrates with ITSM tools such as ServiceNow Online and project management software such as Atlassian Jira and Confluence using the Amazon Q Business data source connectors. You can use a data source connector to combine data from different places into a central index for your Amazon Q Business application. For this demonstration, we use the Amazon Q Business native index and retriever. We also configure an application environment and grant access to users to interact with an application environment using AWS IAM Identity Center for user management. Then, we provision subscriptions for IAM Identity Center users and groups.
Authorized users interact with the application environment through a web experience. You can share the web experience endpoint URL with your users so they can open the URL and authenticate themselves to start chatting with the generative AI application powered by Amazon Q Business.
Deployment
Start by setting up the architecture and data needed for the demonstration.
- We’ve provided an AWS CloudFormation template in our GitHub repository that you can use to set up the environment for this demonstration. If you don’t have existing Atlassian Jira, Confluence, and ServiceNow accounts follow these steps to create trial accounts for the demonstration
- Once step 1 is complete, open the AWS Management Console for Amazon Q Business. On the Applications tab, open your application to see the data sources. See Best practices for data source connector configuration in Amazon Q Business to understand best practices
- To improve retrieved results and customize the end user chat experience, use Amazon Q to map document attributes from your data sources to fields in your Amazon Q index. Choose the Atlassian Jira, Confluence Cloud and ServiceNow Online links to learn more about their document attributes and field mappings. Select the data source to edit its configurations under Actions. Select the appropriate fields that you think would be important for your search needs. Repeat the process for all of the data sources. The following figure is an example of some of the Atlassian Jira project field mappings that we selected
- Sync mode enables you to choose how you want to update your index when your data source content changes. Sync run schedule sets how often you want Amazon Q Business to synchronize your index with the data source. For this demonstration, we set the Sync mode to Full Sync and the Frequency to Run on demand. Update Sync mode with your changes and choose Sync Now to start syncing data sources. When you initiate a sync, Amazon Q will crawl the data source to extract relevant documents, then sync them to the Amazon Q index, making them searchable
- After syncing data sources, you can configure the metadata controls in Amazon Q Business. An Amazon Q Business index has fields that you can map your document attributes to. After the index fields are mapped to document attributes and are search-enabled, admins can use the index fields to boost results from specific sources, or by end users to filter and scope their chat results to specific data. Boosting chat responses based on document attributes helps you rank sources that are more authoritative higher than other sources in your application environment. See Boosting chat responses using metadata boosting to learn more about metadata boosting and metadata controls. The following figure is an example of some of the metadata controls that we selected
- For the purposes of the demonstration, use the Amazon Q Business web experience. Select your application under Applications and then select the Deployed URL link in the web experience settings
- Enter the same username, password and multi-factor authentication (MFA) authentication for the user that you created previously in IAM Identity Center to sign in to the Amazon Q Business web experience generative AI assistant
Demonstration
Now that you’ve signed in to the Amazon Q Business web experience generative AI assistant (shown in the previous figure), let’s try some natural language queries.
IT leaders: You’re an IT leader and your team is working on a critical project that needs to hit the market quickly. You can now ask questions in natural language to Amazon Q Business to get answers based on your company data.
Developers: Developers who want to know information such as the tasks that are assigned to them, specific tasks details, or issues in a particular sub segment. They can now get these questions answered from Amazon Q Business without necessarily signing in to either Atlassian Jira or Confluence.
Project and program managers: Project and program managers can monitor the activities or developments in their projects or programs from Amazon Q Business without having to contact various teams to get individual status updates.
New employees or business users: A newly hired employee who’s looking for information to get started on a project or a business user who needs tech support can use the generative AI assistant to get the information and support they need.
Benefits and outcomes
From the demonstrations, you saw that various users whether they are leaders, managers, developers, or business users can benefit from using a generative AI solution like our virtual IT assistant built using Amazon Q Business. It removes the undifferentiated heavy lifting of having to navigate multiple solutions and cross-reference multiple items and data points to get answers. Amazon Q Business can use the generative AI to provide responses with actionable insights in just few seconds. Now, let’s dive deeper into some of the additional benefits that this solution provides.
- Increased efficiency: Centralized access to information from ServiceNow, Atlassian Jira, and Confluence saves time and reduces the need to switch between multiple systems.
- Enhanced decision-making: Comprehensive data insights from multiple systems leads to better-informed decisions in incident management and problem-solving for various users across the organization.
- Faster incident resolution: Quick access to enterprise data sources and knowledge and AI-assisted remediation steps can significantly reduce mean time to resolutions (MTTR) for cases with elevated priorities.
- Improved knowledge management: Access to Confluence’s architectural documents and other knowledge bases such as ServiceNow’s Knowledge Articles promotes better knowledge sharing across the organization. Users can now get responses based on information from multiple systems.
- Seamless integration and enhanced user experience: Better integration between ITSM processes, project management, and software development streamlines operations. This is helpful for organizations and teams that incorporate agile methodologies.
- Cost savings: Reduction in time spent searching for information and resolving incidents can lead to significant cost savings in IT operations.
- Scalability: Amazon Q Business can grow with the organization, accommodating future needs and additional data sources as required. Organization can create more Amazon Q Business applications and share purpose-built Amazon Q Business apps within their organizations to manage repetitive tasks.
Clean up
After completing your exploration of the virtual IT troubleshooting assistant, delete the CloudFormation stack from your AWS account. This action terminates all resources created during deployment of this demonstration and prevents unnecessary costs from accruing in your AWS account.
Conclusion
By integrating Amazon Q Business with enterprise systems, you can create a powerful virtual IT assistant that streamlines information access and improves productivity. The solution presented in this post demonstrates the power of combining AI capabilities with existing enterprise systems to create powerful unified ITSM solutions and more efficient and user-friendly experiences.
We provide the sample virtual IT assistant using an Amazon Q Business solution as open source—use it as a starting point for your own solution and help us make it better by contributing fixes and features through GitHub pull requests. Visit the GitHub repository to explore the code, choose Watch to be notified of new releases, and check the README for the latest documentation updates.
Learn more:
For expert assistance, AWS Professional Services, AWS Generative AI partner solutions, and AWS Generative AI Competency Partners are here to help.
We’d love to hear from you. Let us know what you think in the comments section, or use the issues forum in the GitHub repository.
About the Authors
Jasmine Rasheed Syed is a Senior Customer Solutions manager at AWS, focused on accelerating time to value for the customers on their cloud journey by adopting best practices and mechanisms to transform their business at scale. Jasmine is a seasoned, result oriented leader with 20+ years of progressive experience in Insurance, Retail & CPG with exemplary track record spanning across Business Development, Cloud/Digital Transformation, Delivery, Operational & Process Excellence and Executive Management.
Suprakash Dutta is a Sr. Solutions Architect at Amazon Web Services. He focuses on digital transformation strategy, application modernization and migration, data analytics, and machine learning. He is part of the AI/ML community at AWS and designs Generative AI and Intelligent Document Processing(IDP) solutions.
Joshua Amah is a Partner Solutions Architect at Amazon Web Services, specializing in supporting SI partners with a focus on AI/ML and generative AI technologies. He is passionate about guiding AWS Partners in using cutting-edge technologies and best practices to build innovative solutions that meet customer needs. Joshua provides architectural guidance and strategic recommendations for both new and existing workloads.
Brad King is an Enterprise Account Executive at Amazon Web Services specializing in translating complex technical concepts into business value and making sure that clients achieve their digital transformation goals efficiently and effectively through long term partnerships.
Joseph Mart is an AI/ML Specialist Solutions Architect at Amazon Web Services (AWS). His core competence and interests lie in machine learning applications and generative AI. Joseph is a technology addict who enjoys guiding AWS customers on architecting their workload in the AWS Cloud. In his spare time, he loves playing soccer and visiting nature.
Process formulas and charts with Anthropic’s Claude on Amazon Bedrock
Research papers and engineering documents often contain a wealth of information in the form of mathematical formulas, charts, and graphs. Navigating these unstructured documents to find relevant information can be a tedious and time-consuming task, especially when dealing with large volumes of data. However, by using Anthropic’s Claude on Amazon Bedrock, researchers and engineers can now automate the indexing and tagging of these technical documents. This enables the efficient processing of content, including scientific formulas and data visualizations, and the population of Amazon Bedrock Knowledge Bases with appropriate metadata.
Amazon Bedrock is a fully managed service that provides a single API to access and use various high-performing foundation models (FMs) from leading AI companies. It offers a broad set of capabilities to build generative AI applications with security, privacy, and responsible AI practices. Anthropic’s Claude 3 Sonnet offers best-in-class vision capabilities compared to other leading models. It can accurately transcribe text from imperfect images—a core capability for retail, logistics, and financial services, where AI might glean more insights from an image, graphic, or illustration than from text alone. The latest of Anthropic’s Claude models demonstrate a strong aptitude for understanding a wide range of visual formats, including photos, charts, graphs and technical diagrams. With Anthropic’s Claude, you can extract more insights from documents, process web UIs and diverse product documentation, generate image catalog metadata, and more.
In this post, we explore how you can use these multi-modal generative AI models to streamline the management of technical documents. By extracting and structuring the key information from the source materials, the models can create a searchable knowledge base that allows you to quickly locate the data, formulas, and visualizations you need to support your work. With the document content organized in a knowledge base, researchers and engineers can use advanced search capabilities to surface the most relevant information for their specific needs. This can significantly accelerate research and development workflows, because professionals no longer have to manually sift through large volumes of unstructured data to find the references they need.
Solution overview
This solution demonstrates the transformative potential of multi-modal generative AI when applied to the challenges faced by scientific and engineering communities. By automating the indexing and tagging of technical documents, these powerful models can enable more efficient knowledge management and accelerate innovation across a variety of industries.
In addition to Anthropic’s Claude on Amazon Bedrock, the solution uses the following services:
- Amazon SageMaker JupyterLab – The SageMakerJupyterLab application is a web-based interactive development environment (IDE) for notebooks, code, and data. JupyterLab application’s flexible and extensive interface can be used to configure and arrange machine learning (ML) workflows. We use JupyterLab to run the code for processing formulae and charts.
- Amazon Simple Storage Service (Amazon S3) – Amazon S3 is an object storage service built to store and protect any amount of data. We use Amazon S3 to store sample documents that are used in this solution.
- AWS Lambda –AWS Lambda is a compute service that runs code in response to triggers such as changes in data, changes in application state, or user actions. Because services such as Amazon S3 and Amazon Simple Notification Service (Amazon SNS) can directly trigger a Lambda function, you can build a variety of real-time serverless data-processing systems.
The solution workflow contains the following steps:
- Split the PDF into individual pages and save them as PNG files.
- With each page:
- Extract the original text.
- Render the formulas in LaTeX.
- Generate a semantic description of each formula.
- Generate an explanation of each formula.
- Generate a semantic description of each graph.
- Generate an interpretation for each graph.
- Generate metadata for the page.
- Generate metadata for the full document.
- Upload the content and metadata to Amazon S3.
- Create an Amazon Bedrock knowledge base.
The following diagram illustrates this workflow.
Prerequisites
- If you’re new to AWS, you first need to create and set up an AWS account.
- Additionally, in your account under Amazon Bedrock, request access to
anthropic.claude-3-5-sonnet-20241022-v2:0
if you don’t have it already.
Deploy the solution
Complete the following steps to set up the solution:
- Launch the AWS CloudFormation template by choosing Launch Stack (this creates the stack in the
us-east-1
AWS Region):
- When the stack deployment is complete, open the Amazon SageMaker AI
- Choose Notebooks in the navigation pane.
- Locate the notebook
claude-scientific-docs-notebook
and choose Open JupyterLab.
- In the notebook, navigate to
notebooks/process_scientific_docs.ipynb
.
- Choose conda_python3 as the kernel, then choose Select.
- Walk through the sample code.
Explanation of the notebook code
In this section, we walk through the notebook code.
Load data
We use example research papers from arXiv to demonstrate the capability outlined here. arXiv is a free distribution service and an open-access archive for nearly 2.4 million scholarly articles in the fields of physics, mathematics, computer science, quantitative biology, quantitative finance, statistics, electrical engineering and systems science, and economics.
We download the documents and store them under a samples folder locally. Multi-modal generative AI models work well with text extraction from image files, so we start by converting the PDF to a collection of images, one for each page.
Get Metadata from formulas
After the image documents are available, you can use Anthropic’s Claude to extract formulas and metadata with the Amazon Bedrock Converse API. Additionally, you can use the Amazon Bedrock Converse API to obtain an explanation of the extracted formulas in plain language. By combining the formula and metadata extraction capabilities of Anthropic’s Claude with the conversational abilities of the Amazon Bedrock Converse API, you can create a comprehensive solution for processing and understanding the information contained within the image documents.
We start with the following example PNG file.
We use the following request prompt:
We get the following response, which shows the extracted formula converted to LaTeX format and described in plain language, enclosed in double dollar signs.
Get metadata from charts
Another useful capability of multi-modal generative AI models is the ability to interpret graphs and generate summaries and metadata. The following is an example of how you can obtain metadata of the charts and graphs using simple natural language conversation with models. We use the following graph.
We provide the following request:
The response returned provides its interpretation of the graph explaining the color-coded lines and suggesting that overall, the DSC model is performing well on the training data, achieving a high Dice coefficient of around 0.98. However, the lower and fluctuating validation Dice coefficient indicates potential overfitting and room for improvement in the model’s generalization performance.
Generate metadata
Using natural language processing, you can generate metadata for the paper to aid in searchability.
We use the following request:
We get the following response, including formula markdown and a description.
Use your extracted data in a knowledge base
Now that we’ve prepared our data with formulas, analyzed charts, and metadata, we will create an Amazon Bedrock knowledge base. This will make the information searchable and enable question-answering capabilities.
Prepare your Amazon Bedrock knowledge base
To create a knowledge base, first upload the processed files and metadata to Amazon S3:
When your files have finished uploading, complete the following steps:
- Create an Amazon Bedrock knowledge base.
- Create an Amazon S3 data source for your knowledge base, and specify hierarchical chunking as the chunking strategy.
Hierarchical chunking involves organizing information into nested structures of child and parent chunks.
The hierarchical structure allows for faster and more targeted retrieval of relevant information, first by performing semantic search on the child chunk and then returning the parent chunk during retrieval. By replacing the children chunks with the parent chunk, we provide large and comprehensive context to the FM.
Hierarchical chunking is best suited for complex documents that have a nested or hierarchical structure, such as technical manuals, legal documents, or academic papers with complex formatting and nested tables.
Query the knowledge base
You can query the knowledge base to retrieve information from the extracted formula and graph metadata from the sample documents. With a query, relevant chunks of text from the source of data are retrieved and a response is generated for the query, based off the retrieved source chunks. The response also cites sources that are relevant to the query.
We use the custom prompt template feature of knowledge bases to format the output as markdown:
We get the following response, which provides information on when the Focal Tversky Loss is used.
Clean up
To clean up and avoid incurring charges, run the cleanup steps in the notebook to delete the files you uploaded to Amazon S3 along with the knowledge base. Then, on the AWS CloudFormation console, locate the stack claude-scientific-doc
and delete it.
Conclusion
Extracting insights from complex scientific documents can be a daunting task. However, the advent of multi-modal generative AI has revolutionized this domain. By harnessing the advanced natural language understanding and visual perception capabilities of Anthropic’s Claude, you can now accurately extract formulas and data from charts, enabling faster insights and informed decision-making.
Whether you are a researcher, data scientist, or developer working with scientific literature, integrating Anthropic’s Claude into your workflow on Amazon Bedrock can significantly boost your productivity and accuracy. With the ability to process complex documents at scale, you can focus on higher-level tasks and uncover valuable insights from your data.
Embrace the future of AI-driven document processing and unlock new possibilities for your organization with Anthropic’s Claude on Amazon Bedrock. Take your scientific document analysis to the next level and stay ahead of the curve in this rapidly evolving landscape.
For further exploration and learning, we recommend checking out the following resources:
- Prompt engineering techniques and best practices: Learn by doing with Anthropic’s Claude 3 on Amazon Bedrock
- Intelligent document processing using Amazon Bedrock and Anthropic Claude
- Automate document processing with Amazon Bedrock Prompt Flows (preview)
About the Authors
Erik Cordsen is a Solutions Architect at AWS serving customers in Georgia. He is passionate about applying cloud technologies and ML to solve real life problems. When he is not designing cloud solutions, Erik enjoys travel, cooking, and cycling.
Renu Yadav is a Solutions Architect at Amazon Web Services (AWS), where she works with enterprise-level AWS customers providing them with technical guidance and help them achieve their business objectives. Renu has a strong passion for learning with her area of specialization in DevOps. She leverages her expertise in this domain to assist AWS customers in optimizing their cloud infrastructure and streamlining their software development and deployment processes.
Venkata Moparthi is a Senior Solutions Architect at AWS who empowers financial services organizations and other industries to navigate cloud transformation with specialized expertise in Cloud Migrations, Generative AI, and secure architecture design. His customer-focused approach combines technical innovation with practical implementation, helping businesses accelerate digital initiatives and achieve strategic outcomes through tailored AWS solutions that maximize cloud potential.
Automate IT operations with Amazon Bedrock Agents
IT operations teams face the challenge of providing smooth functioning of critical systems while managing a high volume of incidents filed by end-users. Manual intervention in incident management can be time-consuming and error prone because it relies on repetitive tasks, human judgment, and potential communication gaps. Using generative AI for IT operations offers a transformative solution that helps automate incident detection, diagnosis, and remediation, enhancing operational efficiency.
AI for IT operations (AIOps) is the application of AI and machine learning (ML) technologies to automate and enhance IT operations. AIOps helps IT teams manage and monitor large-scale systems by automatically detecting, diagnosing, and resolving incidents in real time. It combines data from various sources—such as logs, metrics, and events—to analyze system behavior, identify anomalies, and recommend or execute automated remediation actions. By reducing manual intervention, AIOps improves operational efficiency, accelerates incident resolution, and minimizes downtime.
This post presents a comprehensive AIOps solution that combines various AWS services such as Amazon Bedrock, AWS Lambda, and Amazon CloudWatch to create an AI assistant for effective incident management. This solution also uses Amazon Bedrock Knowledge Bases and Amazon Bedrock Agents. The solution uses the power of Amazon Bedrock to enable the deployment of intelligent agents capable of monitoring IT systems, analyzing logs and metrics, and invoking automated remediation processes.
Amazon Bedrock is a fully managed service that makes foundation models (FMs) from leading AI startups and Amazon available through a single API, so you can choose from a wide range of FMs to find the model that is best suited for your use case. With the Amazon Bedrock serverless experience, you can get started quickly, privately customize FMs with your own data, and integrate and deploy them into your applications using AWS tools without having to manage the infrastructure. Amazon Bedrock Knowledge Bases is a fully managed capability with built-in session context management and source attribution that helps you implement the entire Retrieval Augmented Generation (RAG) workflow, from ingestion to retrieval and prompt augmentation, without having to build custom integrations to data sources and manage data flows. Amazon Bedrock Agents is a fully managed capability that make it straightforward for developers to create generative AI-based applications that can complete complex tasks for a wide range of use cases and deliver up-to-date answers based on proprietary knowledge sources.
Generative AI is rapidly transforming businesses and unlocking new possibilities across industries. This post highlights the transformative impact of large language models (LLMs). With the ability to encode human expertise and communicate in natural language, generative AI can help augment human capabilities and allow organizations to harness knowledge at scale.
Challenges in IT operations with runbooks
Runbooks are detailed, step-by-step guides that outline the processes, procedures, and tasks needed to complete specific operations, typically in IT and systems administration. They are commonly used to document repetitive tasks, troubleshooting steps, and routine maintenance. By standardizing responses to issues and facilitating consistency in task execution, runbooks help teams improve operational efficiency and streamline workflows. Most organizations rely on runbooks to simplify complex processes, making it straightforward for teams to handle routine operations and respond effectively to system issues. For organizations, managing hundreds of runbooks, monitoring their status, keeping track of failures, and setting up the right alerting can become difficult. This creates visibility gaps for IT teams. When you have multiple runbooks for various processes, managing the dependencies and run order between them can become complex and tedious. It’s challenging to handle failure scenarios and make sure everything runs in the right sequence.
The following are some of the challenges that most organizations face with manual IT operations:
- Manual diagnosis through run logs and metrics
- Runbook dependency and sequence mapping
- No automated remediation processes
- No real-time visibility into runbook progress
Solution overview
Amazon Bedrock is the foundation of this solution, empowering intelligent agents to monitor IT systems, analyze data, and automate remediation. The solution provides sample AWS Cloud Development Kit (AWS CDK) code to deploy this solution. The AIOps solution provides an AI assistant using Amazon Bedrock Agents to help with operations automation and runbook execution.
The following architecture diagram explains the overall flow of this solution.
The agent uses Anthropic’s Claude LLM available on Amazon Bedrock as one of the FMs to analyze incident details and retrieve relevant information from the knowledge base, a curated collection of runbooks and best practices. This equips the agent with business-specific context, making sure responses are precise and backed by data from Amazon Bedrock Knowledge Bases. Based on the analysis, the agent dynamically generates a runbook tailored to the specific incident and invokes appropriate remediation actions, such as creating snapshots, restarting instances, scaling resources, or running custom workflows.
Amazon Bedrock Knowledge Bases create an Amazon OpenSearch Serverless vector search collection to store and index incident data, runbooks, and run logs, enabling efficient search and retrieval of information. Lambda functions are employed to run specific actions, such as sending notifications, invoking API calls, or invoking automated workflows. The solution also integrates with Amazon Simple Email Service (Amazon SES) for timely notifications to stakeholders.
The solution workflow consists of the following steps:
- Existing runbooks in various formats (such as Word documents, PDFs, or text files) are uploaded to Amazon Simple Storage Service (Amazon S3).
- Amazon Bedrock Knowledge Bases converts these documents into vector embeddings using a selected embedding model, configured as part of the knowledge base setup.
- These vector embeddings are stored in OpenSearch Serverless for efficient retrieval, also configured during the knowledge base setup.
- Agents and action groups are then set up with the required APIs and prompts for handling different scenarios.
- The OpenAPI specification defines which APIs need to be called, along with their input parameters and expected output, allowing Amazon Bedrock Agents to make informed decisions.
- When a user prompt is received, Amazon Bedrock Agents uses RAG, action groups, and the OpenAPI specification to determine the appropriate API calls. If more details are needed, the agent prompts the user for additional information.
- Amazon Bedrock Agents can iterate and call multiple functions as needed until the task is successfully complete.
Prerequisites
To implement this AIOps solution, you need an active AWS account and basic knowledge of the AWS CDK and the following AWS services:
- Amazon Bedrock
- Amazon CloudWatch
- AWS Lambda
- Amazon OpenSearch Serverless
- Amazon SES
- Amazon S3
Additionally, you need to provision the required infrastructure components, such as Amazon Elastic Compute Cloud (Amazon EC2) instances, Amazon Elastic Block Store (Amazon EBS) volumes, and other resources specific to your IT operations environment.
Build the RAG pipeline with OpenSearch Serverless
This solution uses a RAG pipeline to find relevant content and best practices from operations runbooks to generate responses. The RAG approach helps make sure the agent generates responses that are grounded in factual documentation, which avoids hallucinations. The relevant matches from the knowledge base guide Anthropic’s Claude 3 Haiku model so it focuses on the relevant information. The RAG process is powered by Amazon Bedrock Knowledge Bases, which stores information that the Amazon Bedrock agent can access and use. For this use case, our knowledge base contains existing runbooks from the organization with step-by-step procedures to resolve different operational issues on AWS resources.
The pipeline has the following key tasks:
- Ingest documents in an S3 bucket – The first step ingests existing runbooks into an S3 bucket to create a searchable index with the help of OpenSearch Serverless.
- Monitor infrastructure health using CloudWatch – An Amazon Bedrock action group is used to invoke Lambda functions to get CloudWatch metrics and alerts for EC2 instances from an AWS account. These specific checks are then used as Anthropic’s Claude 3 Haiku model inputs to form a health status overview of the account.
Configure Amazon Bedrock Agents
Amazon Bedrock Agents augment the user request with the right information from Amazon Bedrock Knowledge Bases to generate an accurate response. For this use case, our knowledge base contains existing runbooks from the organization with step-by-step procedures to resolve different operational issues on AWS resources.
By configuring the appropriate action groups and populating the knowledge base with relevant data, you can tailor the Amazon Bedrock agent to assist with specific tasks or domains and provide accurate and helpful responses within its intended scopes.
Amazon Bedrock agents empower Anthropic’s Claude 3 Haiku to use tools, overcoming LLM limitations like knowledge cutoffs and hallucinations, for enhanced task completion through API calls and other external interactions.
The agent’s workflow is to check for resource alerts using an API, then if found, fetch and execute the relevant runbook’s steps (for example, create snapshots, restart instances, and send emails).
The overall system enables automated detection and remediation of operational issues on AWS while enforcing adherence to documented procedures through the runbook approach.
To set up this solution using Amazon Bedrock Agents, refer to the GitHub repo that provisions the following resources. Make sure to verify the AWS Identity and Access Management (IAM) permissions and follow IAM best practices while deploying the code. It is advised to apply least-privilege permissions for IAM policies.
- S3 bucket
- Amazon Bedrock agent
- Action group
- Amazon Bedrock agent IAM role
- Amazon Bedrock agent action group
- Lambda function
- Lambda service policy permission
- Lambda IAM role
Benefits
With this solution, organizations can automate their operations and save a lot of time. The automation is also less prone to errors compared to manual execution. It offers the following additional benefits:
- Reduced manual intervention – Automating incident detection, diagnosis, and remediation helps minimize human involvement, reducing the likelihood of errors, delays, and inconsistencies that often arise from manual processes.
- Increased operational efficiency – By using generative AI, the solution speeds up incident resolution and optimizes operational workflows. The automation of tasks such as runbook execution, resource monitoring, and remediation allows IT teams to focus on more strategic initiatives.
- Scalability – As organizations grow, managing IT operations manually becomes increasingly complex. Automating operations using generative AI can scale with the business, managing more incidents, runbooks, and infrastructure without requiring proportional increases in personnel.
Clean up
To avoid incurring unnecessary costs, it’s recommended to delete the resources created during the implementation of this solution when not in use. You can do this by deleting the AWS CloudFormation stacks deployed as part of the solution, or manually deleting the resources on the AWS Management Console or using the AWS Command Line Interface (AWS CLI).
Conclusion
The AIOps pipeline presented in this post empowers IT operations teams to streamline incident management processes, reduce manual interventions, and enhance operational efficiency. With the power of AWS services, organizations can automate incident detection, diagnosis, and remediation, enabling faster incident resolution and minimizing downtime.
Through the integration of Amazon Bedrock, Anthropic’s Claude on Amazon Bedrock, Amazon Bedrock Agents, Amazon Bedrock Knowledge Bases, and other supporting services, this solution provides real-time visibility into incidents, automated runbook generation, and dynamic remediation actions. Additionally, the solution provides timely notifications and seamless collaboration between AI agents and human operators, fostering a more proactive and efficient approach to IT operations.
Generative AI is rapidly transforming how businesses can take advantage of cloud technologies with ease. This solution using Amazon Bedrock demonstrates the immense potential of generative AI models to enhance human capabilities. By providing developers expert guidance grounded in AWS best practices, this AI assistant enables DevOps teams to review and optimize cloud architecture across of AWS accounts.
Try out the solution yourself and leave any feedback or questions in the comments.
About the Authors
Upendra V is a Sr. Solutions Architect at Amazon Web Services, specializing in Generative AI and cloud solutions. He helps enterprise customers design and deploy production-ready Generative AI workloads, implement Large Language Models (LLMs) and Agentic AI systems, and optimize cloud deployments. With expertise in cloud adoption and machine learning, he enables organizations to build and scale AI-driven applications efficiently.
Deepak Dixit is a Solutions Architect at Amazon Web Services, specializing in Generative AI and cloud solutions. He helps enterprises architect scalable AI/ML workloads, implement Large Language Models (LLMs), and optimize cloud-native applications.
Empowering disaster preparedness: AI’s role in navigating complex climate risks
AI systems that integrate meteorological, geospatial, and socioeconomic data can deliver warnings that are more localized and more timely.Read More