Large language models (LLMs) have raised the bar for human-computer interaction where the expectation from users is that they can communicate with their applications through natural language. Beyond simple language understanding, real-world applications require managing complex workflows, connecting to external data, and coordinating multiple AI capabilities. Imagine scheduling a doctor’s appointment where an AI agent checks your calendar, accesses your provider’s system, verifies insurance, and confirms everything in one go—no more app-switching or hold times. In these real-world scenarios, agents can be a game changer, delivering more customized generative AI applications.
LLM agents serve as decision-making systems for application control flow. However, these systems face several operational challenges during scaling and development. The primary issues include tool selection inefficiency, where agents with access to numerous tools struggle with optimal tool selection and sequencing, context management limitations that prevent single agents from effectively managing increasingly complex contextual information, and specialization requirements as complex applications demand diverse expertise areas such as planning, research, and analysis. The solution lies in implementing a multi-agent architecture, which involves decomposing the main system into smaller, specialized agents that operate independently. Implementation options range from basic prompt-LLM combinations to sophisticated ReAct (Reasoning and Acting) agents, allowing for more efficient task distribution and specialized handling of different application components. This modular approach enhances system manageability and allows for better scaling of LLM-based applications while maintaining functional efficiency through specialized components.
This post demonstrates how to integrate open-source multi-agent framework, LangGraph, with Amazon Bedrock. It explains how to use LangGraph and Amazon Bedrock to build powerful, interactive multi-agent applications that use graph-based orchestration.
AWS has introduced a multi-agent collaboration capability for Amazon Bedrock Agents, enabling developers to build, deploy, and manage multiple AI agents working together on complex tasks. This feature allows for the creation of specialized agents that handle different aspects of a process, coordinated by a supervisor agent that breaks down requests, delegates tasks, and consolidates outputs. This approach improves task success rates, accuracy, and productivity, especially for complex, multi-step tasks.
Challenges with multi-agent systems
In a single-agent system, planning involves the LLM agent breaking down tasks into a sequence of small tasks, whereas a multi-agent system must have workflow management involving task distribution across multiple agents. Unlike single-agent environments, multi-agent systems require a coordination mechanism where each agent must maintain alignment with others while contributing to the overall objective. This introduces unique challenges in managing inter-agent dependencies, resource allocation, and synchronization, necessitating robust frameworks that maintain system-wide consistency while optimizing performance.
Memory management in AI systems differs between single-agent and multi-agent architectures. Single-agent systems use a three-tier structure: short-term conversational memory, long-term historical storage, and external data sources like Retrieval Augmented Generation (RAG). Multi-agent systems require more advanced frameworks to manage contextual data, track interactions, and synchronize historical records across agents. These systems must handle real-time interactions, context synchronization, and efficient data retrieval, necessitating careful design of memory hierarchies, access patterns, and inter-agent sharing.
Agent frameworks are essential for multi-agent systems because they provide the infrastructure for coordinating autonomous agents, managing communication and resources, and orchestrating workflows. Agent frameworks alleviate the need to build these complex components from scratch.
LangGraph, part of LangChain, orchestrates agentic workflows through a graph-based architecture that handles complex processes and maintains context across agent interactions. It uses supervisory control patterns and memory systems for coordination.
LangGraph Studio enhances development with graph visualization, execution monitoring, and runtime debugging capabilities. The integration of LangGraph with Amazon Bedrock empowers you to take advantage of the strengths of multiple agents seamlessly, fostering a collaborative environment that enhances the efficiency and effectiveness of LLM-based systems.
Understanding LangGraph and LangGraph Studio
LangGraph implements state machines and directed graphs for multi-agent orchestration. The framework provides fine-grained control over both the flow and state of your agent applications. LangGraph models agent workflows as graphs. You define the behavior of your agents using three key components:
- State – A shared data structure that represents the current snapshot of your application.
- Nodes – Python functions that encode the logic of your agents.
- Edges – Python functions that determine which Node to execute next based on the current state. They can be conditional branches or fixed transitions.
LangGraph implements a central persistence layer, enabling features that are common to most agent architectures, including:
- Memory – LangGraph persists arbitrary aspects of your application’s state, supporting memory of conversations and other updates within and across user interactions.
- Human-in-the-loop – Because state is checkpointed, execution can be interrupted and resumed, allowing for decisions, validation, and corrections at key stages through human input.
LangGraph Studio is an integrated development environment (IDE) specifically designed for AI agent development. It provides developers with powerful tools for visualization, real-time interaction, and debugging capabilities. The key features of LangGraph Studio are:
- Visual agent graphs – The IDE’s visualization tools allow developers to represent agent flows as intuitive graphic wheels, making it straightforward to understand and modify complex system architectures.
- Real-time debugging – The ability to interact with agents in real time and modify responses mid-execution creates a more dynamic development experience.
- Stateful architecture – Support for stateful and adaptive agents within a graph-based architecture enables more sophisticated behaviors and interactions.
The following screenshot shows the nodes, edges, and state of a typical LangGraph agent workflow as viewed in LangGraph Studio.
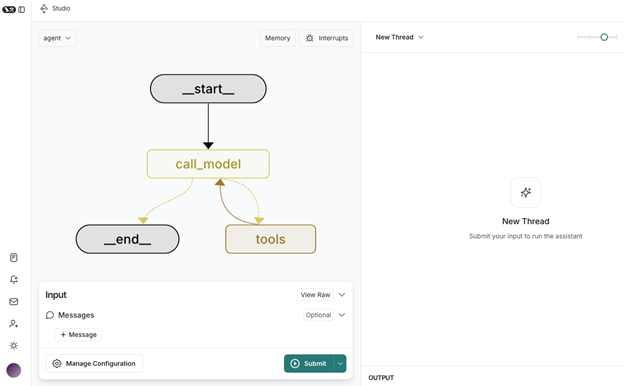
Figure 1: LangGraph Studio UI
In the preceding example, the state begins with __start__
and ends with __end__
. The nodes for invoking the model and tools are defined by you and the edges tell you which paths can be followed by the workflow.
LangGraph Studio is available as a desktop application for MacOS users. Alternatively, you can run a local in-memory development server that can be used to connect a local LangGraph application with a web version of the studio.
Solution overview
This example demonstrates the supervisor agentic pattern, where a supervisor agent coordinates multiple specialized agents. Each agent maintains its own scratchpad while the supervisor orchestrates communication and delegates tasks based on agent capabilities. This distributed approach improves efficiency by allowing agents to focus on specific tasks while enabling parallel processing and system scalability.
Let’s walk through an example with the following user query: “Suggest a travel destination and search flight and hotel for me. I want to travel on 15-March-2025 for 5 days.” The workflow consists of the following steps:
- The Supervisor Agent receives the initial query and breaks it down into sequential tasks:
- Destination recommendation required.
- Flight search needed for March 15, 2025.
- Hotel booking required for 5 days.
- The Destination Agent begins its work by accessing the user’s stored profile. It searches its historical database, analyzing patterns from similar user profiles to recommend the destination. Then it passes the destination back to the Supervisor Agent.
- The Supervisor Agent forwards the chosen destination to the Flight Agent, which searches available flights for the given date.
- The Supervisor Agent activates the Hotel Agent, which searches for hotels in the destination city.
- The Supervisor Agent compiles the recommendations into a comprehensive travel plan, presenting the user with a complete itinerary including destination rationale, flight options, and hotel suggestions.
The following figure shows a multi-agent workflow of how these agents connect to each other and which tools are involved with each agent.
Figure 2: Multi-agent workflow
Prerequisites
You will need the following prerequisites before you can proceed with this solution. For this post, we use the us-west-2
AWS Region. For details on available Regions, see Amazon Bedrock endpoints and quotas.
Core components
Each agent is structured with two primary components:
- graph.py – This script defines the agent’s workflow and decision-making logic. It implements the LangGraph state machine for managing agent behavior and configures the communication flow between different components. For example:
- The Flight Agent’s graph manages the flow between chat and tool operations.
- The Hotel Agent’s graph handles conditional routing between search, booking, and modification operations.
- The Supervisor Agent’s graph orchestrates the overall multi-agent workflow.
- tools.py – This script contains the concrete implementations of agent capabilities. It implements the business logic for each operation and handles data access and manipulation. It provides specific functionalities like:
- Flight tools:
search_flights
, book_flights
, change_flight_booking
, cancel_flight_booking
.
- Hotel tools:
suggest_hotels
, book_hotels
, change_hotel_booking
, cancel_hotel_booking
.
This separation between graph (workflow) and tools (implementation) allows for a clean architecture where the decision-making process is separate from the actual execution of tasks. The agents communicate through a state-based graph system implemented using LangGraph, where the Supervisor Agent directs the flow of information and tasks between the specialized agents.
To set up Amazon Bedrock with LangGraph, refer to the following GitHub repo. The high-level steps are as follows:
- Install the required packages:
pip install boto3 langchain-aws
These packages are essential for AWS Bedrock integration:
boto
: AWS SDK for Python, handles AWS service communication
langchain-aws
: Provides LangChain integrations for AWS services
- Import the modules:
from langchain_aws import ChatBedrockConverse
from langchain_aws import ChatBedrock
- Create an LLM object:
bedrock_client = boto3.client("bedrock-runtime", region_name="<region_name>")
llm = ChatBedrockConverse(
model="anthropic.claude-3-haiku-20240307-v1:0",
temperature=0,
max_tokens=None,
client=bedrock_client,
# other params...
)
LangGraph Studio configuration
This project uses a langgraph.json configuration file to define the application structure and dependencies. This file is essential for LangGraph Studio to understand how to run and visualize your agent graphs.
{
"dependencies": [
"boto3>=1.35.87",
"langchain-aws>=0.2.10",
"."
],
"graphs": {
"supervisor": "./src/supervisor_agent/graph.py:graph",
"flight": "./src/flight_agent/graph.py:graph",
"hotel": "./src/hotel_agent/graph.py:graph"
},
"env": "./.env"
}
LangGraph Studio uses this file to build and visualize the agent workflows, allowing you to monitor and debug the multi-agent interactions in real time.
Testing and debugging
You’re now ready to test the multi-agent travel assistant. You can start the graph using the langgraph dev
command. It will start the LangGraph API server in development mode with hot reloading and debugging capabilities. As shown in the following screenshot, the interface provides a straightforward way to select which graph you want to test through the dropdown menu at the top left. The Manage Configuration button at the bottom lets you set up specific testing parameters before you begin. This development environment provides everything you need to thoroughly test and debug your multi-agent system with real-time feedback and monitoring capabilities.
Figure 3: LangGraph studio with Destination Agent recommendation
LangGraph Studio offers flexible configuration management through its intuitive interface. As shown in the following screenshot, you can create and manage multiple configuration versions (v1, v2, v3) for your graph execution. For example, in this scenario, we want to use user_id
to fetch historic use information. This versioning system makes it simple to track and switch between different test configurations while debugging your multi-agent system.
Figure 4: Runnable configuration details
In the preceding example, we set up the user_id
that tools can use to retrieve history or other details.
Let’s test the Planner Agent. This agent has the compare_and_recommend_destination
tool, which can check past travel data and recommend travel destinations based on the user profile. We use user_id
in the configuration so that can it be used by the tool.
LangGraph has concept of checkpoint memory that is managed using a thread. The following screenshot shows that you can quickly manage threads in LangGraph Studio.
Figure 5: View graph state in the thread
In this example, destination_agent
is using a tool; you can also check the tool’s output. Similarly, you can test flight_agent
and hotel_agent
to verify each agent.
When all the agents are working well, you’re ready to test the full workflow. You can evaluate the state a verify input and output of each agent.
The following screenshot shows the full view of the Supervisor Agent with its sub-agents.
Figure 6: Supervisor Agent with complete workflow
Considerations
Multi-agent architectures must consider agent coordination, state management, communication, output consolidation, and guardrails, maintaining processing context, error handling, and orchestration. Graph-based architectures offer significant advantages over linear pipelines, enabling complex workflows with nonlinear communication patterns and clearer system visualization. These structures allow for dynamic pathways and adaptive communication, ideal for large-scale deployments with simultaneous agent interactions. They excel in parallel processing and resource allocation but require sophisticated setup and might demand higher computational resources. Implementing these systems necessitates careful planning of system topology, robust monitoring, and well-designed fallback mechanisms for failed interactions.
When implementing multi-agent architectures in your organization, it’s crucial to align with your company’s established generative AI operations and governance frameworks. Prior to deployment, verify alignment with your organization’s AI safety protocols, data handling policies, and model deployment guidelines. Although this architectural pattern offers significant benefits, its implementation should be tailored to fit within your organization’s specific AI governance structure and risk management frameworks.
Clean up
Delete any IAM roles and policies created specifically for this post. Delete the local copy of this post’s code. If you no longer need access to an Amazon Bedrock FM, you can remove access from it. For instructions, see Add or remove access to Amazon Bedrock foundation models
Conclusion
The integration of LangGraph with Amazon Bedrock significantly advances multi-agent system development by providing a robust framework for sophisticated AI applications. This combination uses LangGraph’s orchestration capabilities and FMs in Amazon Bedrock to create scalable, efficient systems. It addresses challenges in multi-agent architectures through state management, agent coordination, and workflow orchestration, offering features like memory management, error handling, and human-in-the-loop capabilities. LangGraph Studio’s visualization and debugging tools enable efficient design and maintenance of complex agent interactions. This integration offers a powerful foundation for next-generation multi-agent systems, providing effective workflow handling, context maintenance, reliable results, and optimal resource utilization.
For the example code and demonstration discussed in this post, refer to the accompanying GitHub repository. You can also refer to the following GitHub repo for Amazon Bedrock multi-agent collaboration code samples.
About the Authors
Jagdeep Singh Soni is a Senior Partner Solutions Architect at AWS based in the Netherlands. He uses his passion for generative AI to help customers and partners build generative AI applications using AWS services. Jagdeep has 15 years of experience in innovation, experience engineering, digital transformation, cloud architecture, and ML applications.
Ajeet Tewari is a Senior Solutions Architect for Amazon Web Services. He works with enterprise customers to help them navigate their journey to AWS. His specialties include architecting and implementing scalable OLTP systems and leading strategic AWS initiatives.
Rupinder Grewal is a Senior AI/ML Specialist Solutions Architect with AWS. He currently focuses on serving of models and MLOps on Amazon SageMaker. Prior to this role, he worked as a Machine Learning Engineer building and hosting models. Outside of work, he enjoys playing tennis and biking on mountain trails.
Read More