Amazon Bedrock provides a broad range of high-performing foundation models from Amazon and other leading AI companies, including Anthropic, AI21, Meta, Cohere, and Stability AI, and covers a wide range of use cases, including text and image generation, searching, chat, reasoning and acting agents, and more. The new Amazon Titan Image Generator model allows content creators to quickly generate high-quality, realistic images using simple English text prompts. The advanced AI model understands complex instructions with multiple objects and returns studio-quality images suitable for advertising, ecommerce, and entertainment. Key features include the ability to refine images by iterating on prompts, automatic background editing, and generating multiple variations of the same scene. Creators can also customize the model with their own data to output on-brand images in a specific style. Importantly, Titan Image Generator has in-built safeguards, like invisible watermarks on all AI-generated images, to encourage responsible use and mitigate the spread of disinformation. This innovative technology makes producing custom images in large volume for any industry more accessible and efficient.
The new Amazon Titan Multimodal Embeddings model helps build more accurate search and recommendations by understanding text, images, or both. It converts images and English text into semantic vectors, capturing meaning and relationships in your data. You can combine text and images like product descriptions and photos to identify items more effectively. The vectors power speedy, accurate search experiences. Titan Multimodal Embeddings is flexible in vector dimensions, enabling optimization for performance needs. An asynchronous API and Amazon OpenSearch Service connector make it easy to integrate the model into your neural search applications.
In this post, we walk through how to use the Titan Image Generator and Titan Multimodal Embeddings models via the AWS Python SDK.
Image generation and editing
In this section, we demonstrate the basic coding patterns for using the AWS SDK to generate new images and perform AI-powered edits on existing images. Code examples are provided in Python, and JavaScript (Node.js) is also available in this GitHub repository.
Before you can write scripts that use the Amazon Bedrock API, you need to install the appropriate version of the AWS SDK in your environment. For Python scripts, you can use the AWS SDK for Python (Boto3). Python users may also want to install the Pillow module, which facilitates image operations like loading and saving images. For setup instructions, refer to the GitHub repository.
Additionally, enable access to the Amazon Titan Image Generator and Titan Multimodal Embeddings models. For more information, refer to Model access.
Helper functions
The following function sets up the Amazon Bedrock Boto3 runtime client and generates images by taking payloads of different configurations (which we discuss later in this post):
import boto3
import json, base64, io
from random import randint
from PIL import Image
bedrock_runtime_client = boto3.client("bedrock-runtime")
def titan_image(
payload: dict,
num_image: int = 2,
cfg: float = 10.0,
seed: int = None,
modelId: str = "amazon.titan-image-generator-v1",
) -> list:
# ImageGenerationConfig Options:
# - numberOfImages: Number of images to be generated
# - quality: Quality of generated images, can be standard or premium
# - height: Height of output image(s)
# - width: Width of output image(s)
# - cfgScale: Scale for classifier-free guidance
# - seed: The seed to use for reproducibility
seed = seed if seed is not None else randint(0, 214783647)
body = json.dumps(
{
**payload,
"imageGenerationConfig": {
"numberOfImages": num_image, # Range: 1 to 5
"quality": "premium", # Options: standard/premium
"height": 1024, # Supported height list above
"width": 1024, # Supported width list above
"cfgScale": cfg, # Range: 1.0 (exclusive) to 10.0
"seed": seed, # Range: 0 to 214783647
},
}
)
response = bedrock_runtime_client.invoke_model(
body=body,
modelId=modelId,
accept="application/json",
contentType="application/json",
)
response_body = json.loads(response.get("body").read())
images = [
Image.open(io.BytesIO(base64.b64decode(base64_image)))
for base64_image in response_body.get("images")
]
return images
Generate images from text
Scripts that generate a new image from a text prompt follow this implementation pattern:
- Configure a text prompt and optional negative text prompt.
- Use the
BedrockRuntime
client to invoke the Titan Image Generator model.
- Parse and decode the response.
- Save the resulting images to disk.
Text-to-image
The following is a typical image generation script for the Titan Image Generator model:
# Text Variation
# textToImageParams Options:
# text: prompt to guide the model on how to generate variations
# negativeText: prompts to guide the model on what you don't want in image
images = titan_image(
{
"taskType": "TEXT_IMAGE",
"textToImageParams": {
"text": "two dogs walking down an urban street, facing the camera", # Required
"negativeText": "cars", # Optional
},
}
)
This will produce images similar to the following.
Response Image 1 |
Response Image 2 |
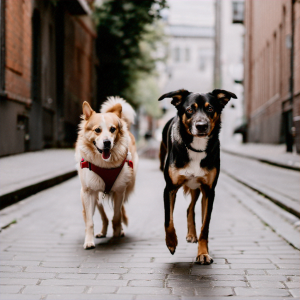 |
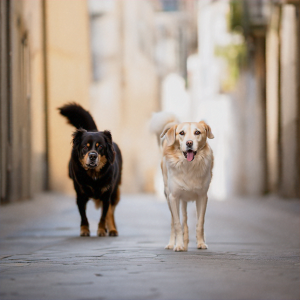 |
Image variants
Image variation provides a way to generate subtle variants of an existing image. The following code snippet uses one of the images generated in the previous example to create variant images:
# Import an input image like this (only PNG/JPEG supported):
with open("<YOUR_IMAGE_FILE_PATH>", "rb") as image_file:
input_image = base64.b64encode(image_file.read()).decode("utf8")
# Image Variation
# ImageVariationParams Options:
# text: prompt to guide the model on how to generate variations
# negativeText: prompts to guide the model on what you don't want in image
# images: base64 string representation of the input image, only 1 is supported
images = titan_image(
{
"taskType": "IMAGE_VARIATION",
"imageVariationParams": {
"text": "two dogs walking down an urban street, facing the camera", # Required
"images": [input_image], # One image is required
"negativeText": "cars", # Optional
},
},
)
This will produce images similar to the following.
Original Image |
Response Image 1 |
Response Image 2 |
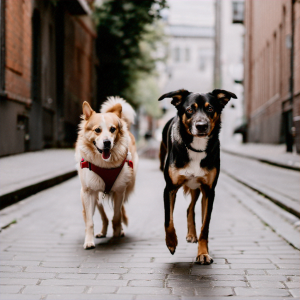 |
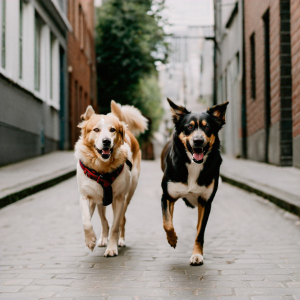 |
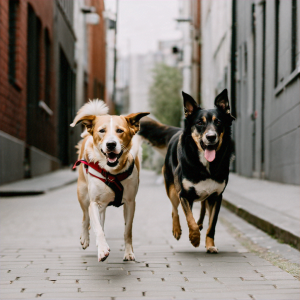 |
Edit an existing image
The Titan Image Generator model allows you to add, remove, or replace elements or areas within an existing image. You specify which area to affect by providing one of the following:
- Mask image – A mask image is a binary image in which the 0-value pixels represent the area you want to affect and the 255-value pixels represent the area that should remain unchanged.
- Mask prompt – A mask prompt is a natural language text description of the elements you want to affect, that uses an in-house text-to-segmentation model.
For more information, refer to Prompt Engineering Guidelines.
Scripts that apply an edit to an image follow this implementation pattern:
- Load the image to be edited from disk.
- Convert the image to a base64-encoded string.
- Configure the mask through one of the following methods:
- Load a mask image from disk, encoding it as base64 and setting it as the
maskImage
parameter.
- Set the
maskText
parameter to a text description of the elements to affect.
- Specify the new content to be generated using one of the following options:
- To add or replace an element, set the
text
parameter to a description of the new content.
- To remove an element, omit the
text
parameter completely.
- Use the
BedrockRuntime
client to invoke the Titan Image Generator model.
- Parse and decode the response.
- Save the resulting images to disk.
Object editing: Inpainting with a mask image
The following is a typical image editing script for the Titan Image Generator model using maskImage
. We take one of the images generated earlier and provide a mask image, where 0-value pixels are rendered as black and 255-value pixels as white. We also replace one of the dogs in the image with a cat using a text prompt.
with open("<YOUR_MASK_IMAGE_FILE_PATH>", "rb") as image_file:
mask_image = base64.b64encode(image_file.read()).decode("utf8")
# Import an input image like this (only PNG/JPEG supported):
with open("<YOUR_ORIGINAL_IMAGE_FILE_PATH>", "rb") as image_file:
input_image = base64.b64encode(image_file.read()).decode("utf8")
# Inpainting
# inPaintingParams Options:
# text: prompt to guide inpainting
# negativeText: prompts to guide the model on what you don't want in image
# image: base64 string representation of the input image
# maskImage: base64 string representation of the input mask image
# maskPrompt: prompt used for auto editing to generate mask
images = titan_image(
{
"taskType": "INPAINTING",
"inPaintingParams": {
"text": "a cat", # Optional
"negativeText": "bad quality, low res", # Optional
"image": input_image, # Required
"maskImage": mask_image,
},
},
num_image=3,
)
This will produce images similar to the following.
Original Image |
Mask Image |
Edited Image |
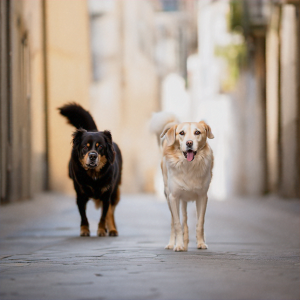 |
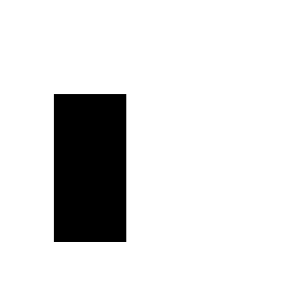 |
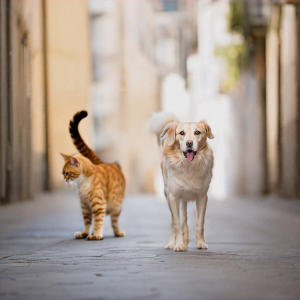 |
Object removal: Inpainting with a mask prompt
In another example, we use maskPrompt
to specify an object in the image, taken from the earlier steps, to edit. By omitting the text prompt, the object will be removed:
# Import an input image like this (only PNG/JPEG supported):
with open("<YOUR_IMAGE_FILE_PATH>", "rb") as image_file:
input_image = base64.b64encode(image_file.read()).decode("utf8")
images = titan_image(
{
"taskType": "INPAINTING",
"inPaintingParams": {
"negativeText": "bad quality, low res", # Optional
"image": input_image, # Required
"maskPrompt": "white dog", # One of "maskImage" or "maskPrompt" is required
},
},
)
This will produce images similar to the following.
Original Image |
Response Image |
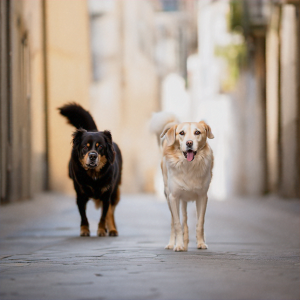 |
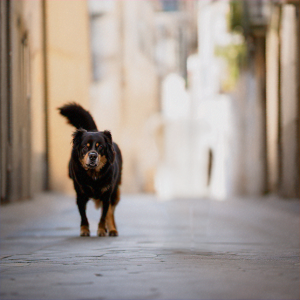 |
Background editing: Outpainting
Outpainting is useful when you want to replace the background of an image. You can also extend the bounds of an image for a zoom-out effect. In the following example script, we use maskPrompt
to specify which object to keep; you can also use maskImage
. The parameter outPaintingMode
specifies whether to allow modification of the pixels inside the mask. If set as DEFAULT
, pixels inside of the mask are allowed to be modified so that the reconstructed image will be consistent overall. This option is recommended if the maskImage
provided doesn’t represent the object with pixel-level precision. If set as PRECISE
, the modification of pixels inside of the mask is prevented. This option is recommended if using a maskPrompt
or a maskImage
that represents the object with pixel-level precision.
# Import an input image like this (only PNG/JPEG supported):
with open("<YOUR_IMAGE_FILE_PATH>", "rb") as image_file:
input_image = base64.b64encode(image_file.read()).decode("utf8")
# OutPaintingParams Options:
# text: prompt to guide outpainting
# negativeText: prompts to guide the model on what you don't want in image
# image: base64 string representation of the input image
# maskImage: base64 string representation of the input mask image
# maskPrompt: prompt used for auto editing to generate mask
# outPaintingMode: DEFAULT | PRECISE
images = titan_image(
{
"taskType": "OUTPAINTING",
"outPaintingParams": {
"text": "forest", # Required
"image": input_image, # Required
"maskPrompt": "dogs", # One of "maskImage" or "maskPrompt" is required
"outPaintingMode": "PRECISE", # One of "PRECISE" or "DEFAULT"
},
},
num_image=3,
)
This will produce images similar to the following.
Original Image |
Text |
Response Image |
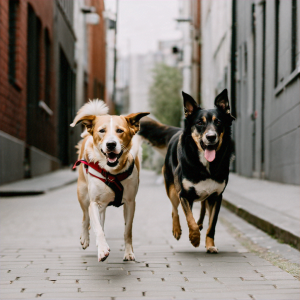 |
“beach” |
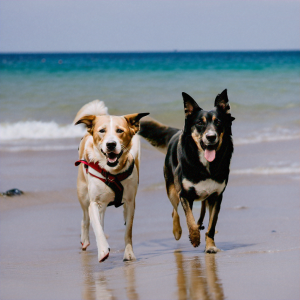 |
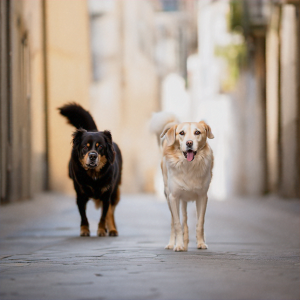 |
“forest” |
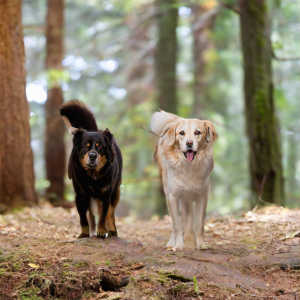 |
In addition, the effects of different values for outPaintingMode
, with a maskImage
that doesn’t outline the object with pixel-level precision, are as follows.
Original Image |
Mask Image |
Text |
outPaintingMode |
Response Image |
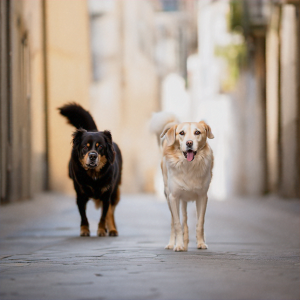 |
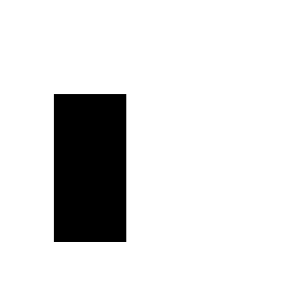 |
“forest” |
DEFAULT |
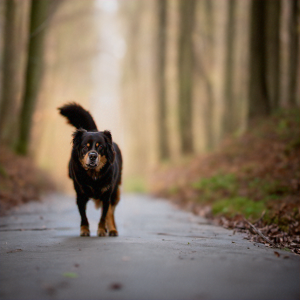 |
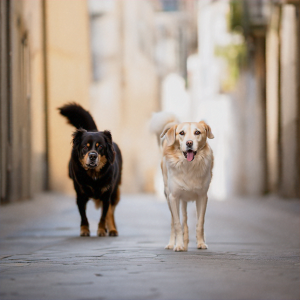 |
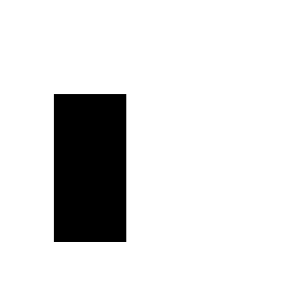 |
“forest” |
PRECISE |
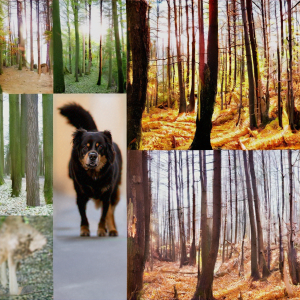 |
This section has given you an overview of the operations you can perform with the Titan Image Generator model. Specifically, these scripts demonstrate text-to-image, image variation, inpainting, and outpainting tasks. You should be able to adapt the patterns for your own applications by referencing the parameter details for those task types detailed in Amazon Titan Image Generator documentation.
Multimodal embedding and searching
You can use the Amazon Titan Multimodal Embeddings model for enterprise tasks such as image search and similarity-based recommendation, and it has built-in mitigation that helps reduce bias in searching results. There are multiple embedding dimension sizes for best latency/accuracy trade-offs for different needs, and all can be customized with a simple API to adapt to your own data while persisting data security and privacy. Amazon Titan Multimodal Embeddings is provided as simple APIs for real-time or asynchronous batch transform searching and recommendation applications, and can be connected to different vector databases, including Amazon OpenSearch Service.
Helper functions
The following function converts an image, and optionally text, into multimodal embeddings:
def titan_multimodal_embedding(
image_path: str = None, # maximum 2048 x 2048 pixels
description: str = None, # English only and max input tokens 128
dimension: int = 1024, # 1,024 (default), 384, 256
model_id: str = "amazon.titan-embed-image-v1",
):
payload_body = {}
embedding_config: dict = {"embeddingConfig": {"outputEmbeddingLength": dimension}}
# You can specify either text or image or both
if image_path:
# Maximum image size supported is 2048 x 2048 pixels
with open(image_path, "rb") as image_file:
payload_body["inputImage"] = base64.b64encode(image_file.read()).decode(
"utf8"
)
if description:
payload_body["inputText"] = description
assert payload_body, "please provide either an image and/or a text description"
print("n".join(payload_body.keys()))
response = bedrock_runtime_client.invoke_model(
body=json.dumps({**payload_body, **embedding_config}),
modelId=model_id,
accept="application/json",
contentType="application/json",
)
return json.loads(response.get("body").read())
The following function returns the top similar multimodal embeddings given a query multimodal embeddings. Note that in practice, you can use a managed vector database, such as OpenSearch Service. The following example is for illustration purposes:
from scipy.spatial.distance import cdist
import numpy as np
def search(query_emb: np.array, indexes: np.array, top_k: int = 1):
dist = cdist(query_emb, indexes, metric="cosine")
return dist.argsort(axis=-1)[0, :top_k], np.sort(dist, axis=-1)[:top_k]
Synthetic dataset
For illustration purposes, we use Anthropic’s Claude 2.1 model in Amazon Bedrock to randomly generate seven different products, each with three variants, using the following prompt:
Generate a list of 7 items description for an online e-commerce shop, each comes with 3 variants of color or type. All with separate full sentence description.
The following is the list of returned outputs:
1. T-shirt
- A red cotton t-shirt with a crew neck and short sleeves.
- A blue cotton t-shirt with a v-neck and short sleeves.
- A black polyester t-shirt with a scoop neck and cap sleeves.
2. Jeans
- Classic blue relaxed fit denim jeans with a mid-rise waist.
- Black skinny fit denim jeans with a high-rise waist and ripped details at the knees.
- Stonewash straight leg denim jeans with a standard waist and front pockets.
3. Sneakers
- White leather low-top sneakers with an almond toe cap and thick rubber outsole.
- Gray mesh high-top sneakers with neon green laces and a padded ankle collar.
- Tan suede mid-top sneakers with a round toe and ivory rubber cupsole.
4. Backpack
- A purple nylon backpack with padded shoulder straps, front zipper pocket and laptop sleeve.
- A gray canvas backpack with brown leather trims, side water bottle pockets and drawstring top closure.
- A black leather backpack with multiple interior pockets, top carry handle and adjustable padded straps.
5. Smartwatch
- A silver stainless steel smartwatch with heart rate monitor, GPS tracker and sleep analysis.
- A space gray aluminum smartwatch with step counter, phone notifications and calendar syncing.
- A rose gold smartwatch with activity tracking, music controls and customizable watch faces.
6. Coffee maker
- A 12-cup programmable coffee maker in brushed steel with removable water tank and keep warm plate.
- A compact 5-cup single serve coffee maker in matt black with travel mug auto-dispensing feature.
- A retro style stovetop percolator coffee pot in speckled enamel with stay-cool handle and glass knob lid.
7. Yoga mat
- A teal 4mm thick yoga mat made of natural tree rubber with moisture-wicking microfiber top.
- A purple 6mm thick yoga mat made of eco-friendly TPE material with integrated carrying strap.
- A patterned 5mm thick yoga mat made of PVC-free material with towel cover included.
Assign the above response to variable response_cat
. Then we use the Titan Image Generator model to create product images for each item:
import re
def extract_text(input_string):
pattern = r"- (.*?)($|n)"
matches = re.findall(pattern, input_string)
extracted_texts = [match[0] for match in matches]
return extracted_texts
product_description = extract_text(response_cat)
titles = []
for prompt in product_description:
images = titan_image(
{
"taskType": "TEXT_IMAGE",
"textToImageParams": {
"text": prompt, # Required
},
},
num_image=1,
)
title = "_".join(prompt.split()[:4]).lower()
titles.append(title)
images[0].save(f"{title}.png", format="png")
All the generated images can be found in the appendix at the end of this post.
Multimodal dataset indexing
Use the following code for multimodal dataset indexing:
multimodal_embeddings = []
for image_filename, description in zip(titles, product_description):
embedding = titan_multimodal_embedding(f"{image_filename}.png", dimension=1024)["embedding"]
multimodal_embeddings.append(embedding)
Multimodal searching
Use the following code for multimodal searching:
query_prompt = "<YOUR_QUERY_TEXT>"
query_embedding = titan_multimodal_embedding(description=query_prompt, dimension=1024)["embedding"]
# If searching via Image
# query_image_filename = "<YOUR_QUERY_IMAGE>"
# query_emb = titan_multimodal_embedding(image_path=query_image_filename, dimension=1024)["embedding"]
idx_returned, dist = search(np.array(query_embedding)[None], np.array(multimodal_embeddings))
The following are some search results.
Query |
Results |
“sneaker” |
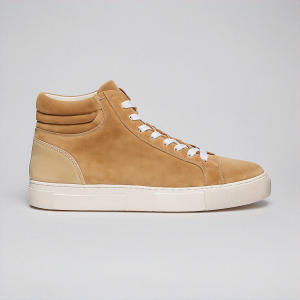 |
“white sneaker” |
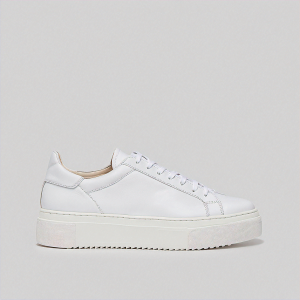 |
“leather backpack” |
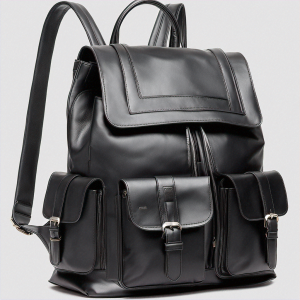 |
“purple backpack” |
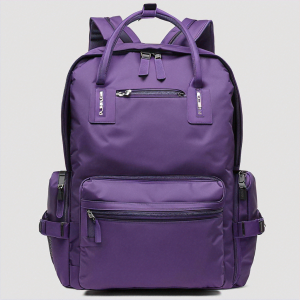 |
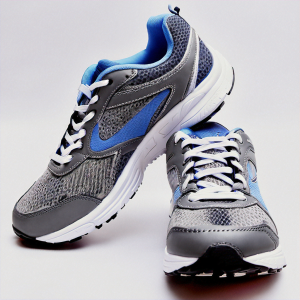 |
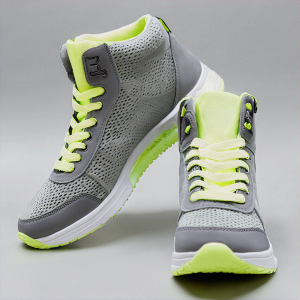 |
Conclusion
The post introduces the Amazon Titan Image Generator and Amazon Titan Multimodal Embeddings models. Titan Image Generator enables you to create custom, high-quality images from text prompts. Key features include iterating on prompts, automatic background editing, and data customization. It has safeguards like invisible watermarks to encourage responsible use. Titan Multimodal Embeddings converts text, images, or both into semantic vectors to power accurate search and recommendations. We then provided Python code samples for using these services, and demonstrated generating images from text prompts and iterating on those images; editing existing images by adding, removing, or replacing elements specified by mask images or mask text; creating multimodal embeddings from text, images, or both; and searching for similar multimodal embeddings to a query. We also demonstrated using a synthetic e-commerce dataset indexed and searched using Titan Multimodal Embeddings. The aim of this post is to enable developers to start using these new AI services in their applications. The code patterns can serve as templates for custom implementations.
All the code is available on the GitHub repository. For more information, refer to the Amazon Bedrock User Guide.
About the Authors
Rohit Mittal is a Principal Product Manager at Amazon AI building multi-modal foundation models. He recently led the launch of Amazon Titan Image Generator model as part of Amazon Bedrock service. Experienced in AI/ML, NLP, and Search, he is interested in building products that solves customer pain points with innovative technology.
Dr. Ashwin Swaminathan is a Computer Vision and Machine Learning researcher, engineer, and manager with 12+ years of industry experience and 5+ years of academic research experience. Strong fundamentals and proven ability to quickly gain knowledge and contribute to newer and emerging areas.
Dr. Yusheng Xie is a Principal Applied Scientist at Amazon AGI. His work focuses building multi-modal foundation models. Before joining AGI, he was leading various multi-modal AI development at AWS such as Amazon Titan Image Generator and Amazon Textract Queries.
Dr. Hao Yang is a Principal Applied Scientist at Amazon. His main research interests are object detection and learning with limited annotations. Outside work, Hao enjoys watching films, photography, and outdoor activities.
Dr. Davide Modolo is an Applied Science Manager at Amazon AGI, working on building large multimodal foundational models. Before joining Amazon AGI, he was a manager/lead for 7 years in AWS AI Labs (Amazon Bedrock and Amazon Rekognition). Outside of work, he enjoys traveling and playing any kind of sport, especially soccer.
Dr. Baichuan Sun, is currently serving as a Sr. AI/ML Solutions Architect at AWS, focusing on generative AI and applies his knowledge in data science and machine learning to provide practical, cloud-based business solutions. With experience in management consulting and AI solution architecture, he addresses a range of complex challenges, including robotics computer vision, time series forecasting, and predictive maintenance, among others. His work is grounded in a solid background of project management, software R&D, and academic pursuits. Outside of work, Dr. Sun enjoys the balance of traveling and spending time with family and friends.
Dr. Kai Zhu currently works as Cloud Support Engineer at AWS, helping customers with issues in AI/ML related services like SageMaker, Bedrock, etc. He is a SageMaker Subject Matter Expert. Experienced in data science and data engineering, he is interested in building generative AI powered projects.
Kris Schultz has spent over 25 years bringing engaging user experiences to life by combining emerging technologies with world class design. In his role as Senior Product Manager, Kris helps design and build AWS services to power Media & Entertainment, Gaming, and Spatial Computing.
Appendix
In the following sections, we demonstrate challenging sample use cases like text insertion, hands, and reflections to highlight the capabilities of the Titan Image Generator model. We also include the sample output images produced in earlier examples.
Text
The Titan Image Generator model excels at complex workflows like inserting readable text into images. This example demonstrates Titan’s ability to clearly render uppercase and lowercase letters in a consistent style within an image.
a corgi wearing a baseball cap with text “genai” |
a happy boy giving a thumbs up, wearing a tshirt with text “generative AI” |
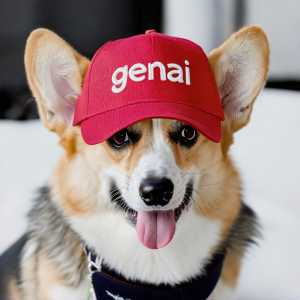 |
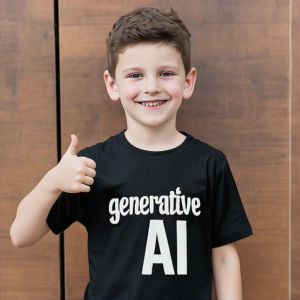 |
Hands
The Titan Image Generator model also has the ability to generate detailed AI images. The image shows realistic hands and fingers with visible detail, going beyond more basic AI image generation that may lack such specificity. In the following examples, notice the precise depiction of the pose and anatomy.
a person’s hand viewed from above |
a close look at a person’s hands holding a coffee mug |
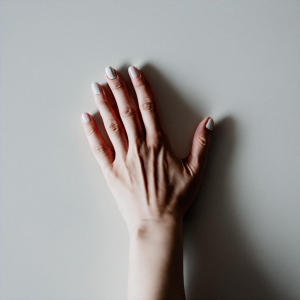 |
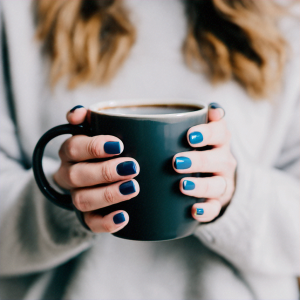 |
Mirror
The images generated by the Titan Image Generator model spatially arrange objects and accurately reflect mirror effects, as demonstrated in the following examples.
A cute fluffy white cat stands on its hind legs, peering curiously into an ornate golden mirror. In the reflection the cat sees itself |
beautiful sky lake with reflections on the water |
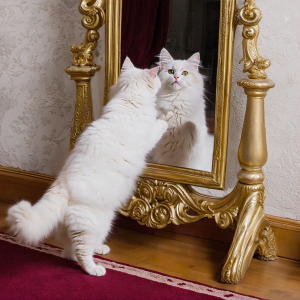 |
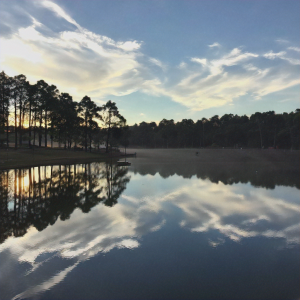 |
Synthetic product images
The following are the product images generated earlier in this post for the Titan Multimodal Embeddings model.
Read More