This post is co-authored by Daryl Martis, Director of Product, Salesforce Einstein AI.
This is the third post in a series discussing the integration of Salesforce Data Cloud and Amazon SageMaker.
In Part 1 and Part 2, we show how the Salesforce Data Cloud and Einstein Studio integration with SageMaker allows businesses to access their Salesforce data securely using SageMaker and use its tools to build, train, and deploy models to endpoints hosted on SageMaker. SageMaker endpoints can be registered to the Salesforce Data Cloud to activate predictions in Salesforce.
In this post, we demonstrate how business analysts and citizen data scientists can create machine learning (ML) models, without any code, in Amazon SageMaker Canvas and deploy trained models for integration with Salesforce Einstein Studio to create powerful business applications. SageMaker Canvas provides a no-code experience to access data from Salesforce Data Cloud and build, test, and deploy models using just a few clicks. SageMaker Canvas also enables you to understand your predictions using feature importance and SHAP values, making it straightforward for you to explain predictions made by ML models.
SageMaker Canvas
SageMaker Canvas enables business analysts and data science teams to build and use ML and generative AI models without having to write a single line of code. SageMaker Canvas provides a visual point-and-click interface to generate accurate ML predictions for classification, regression, forecasting, natural language processing (NLP), and computer vision (CV). In addition, you can access and evaluate foundation models (FMs) from Amazon Bedrock or public FMs from Amazon SageMaker JumpStart for content generation, text extraction, and text summarization to support generative AI solutions. SageMaker Canvas allows you to bring ML models built anywhere and generate predictions directly in SageMaker Canvas.
Salesforce Data Cloud and Einstein Studio
Salesforce Data Cloud is a data platform that provides businesses with real-time updates of their customer data from any touch point.
Einstein Studio is a gateway to AI tools on Salesforce Data Cloud. With Einstein Studio, admins and data scientists can effortlessly create models with a few clicks or using code. Einstein Studio’s bring your own model (BYOM) experience provides the capability to connect custom or generative AI models from external platforms such as SageMaker to Salesforce Data Cloud.
Solution overview
To demonstrate how you can build ML models using data in Salesforce Data Cloud using SageMaker Canvas, we create a predictive model to recommend a product. This model uses the features stored in Salesforce Data Cloud such as customer demographics, marketing engagements, and purchase history. The product recommendation model is built and deployed using the SageMaker Canvas no-code user interface using data in Salesforce Data Cloud.
We use the following sample dataset stored in Amazon Simple Storage Service (Amazon S3). To use this dataset in Salesforce Data Cloud, refer to Create Amazon S3 Data Stream in Data Cloud. The following attributes are needed to create the model:
- Club Member – If the customer is a club member
- Campaign – The campaign the customer is a part of
- State – The state or province the customer resides in
- Month – The month of purchase
- Case Count – The number of cases raised by the customer
- Case Type Return – Whether the customer returned any product within the last year
- Case Type Shipment Damaged – Whether the customer had any shipments damaged in the last year
- Engagement Score – The level of engagement the customer has (response to mailing campaigns, logins to the online store, and so on)
- Tenure – The tenure of the customer relationship with the company
- Clicks – The average number of clicks the customer has made within a week prior to purchase
- Pages Visited – The average number of pages the customer visited within a week prior to purchase
- Product Purchased – The actual product purchased
The following steps give an overview of how to use the Salesforce Data Cloud connector launched in SageMaker Canvas to access your enterprise data and build a predictive model:
- Configure the Salesforce connected app to register the SageMaker Canvas domain.
- Set up OAuth for Salesforce Data Cloud in SageMaker Canvas.
- Connect to Salesforce Data Cloud data using the built-in SageMaker Canvas Salesforce Data Cloud connector and import the dataset.
- Build and train models in SageMaker Canvas.
- Deploy the model in SageMaker Canvas and make predictions.
- Deploy an Amazon API Gateway endpoint as a front-end connection to the SageMaker inference endpoint.
- Register the API Gateway endpoint in Einstein Studio. For instructions, refer to Bring Your Own AI Models to Data Cloud.
The following diagram illustrates the solution architecture.
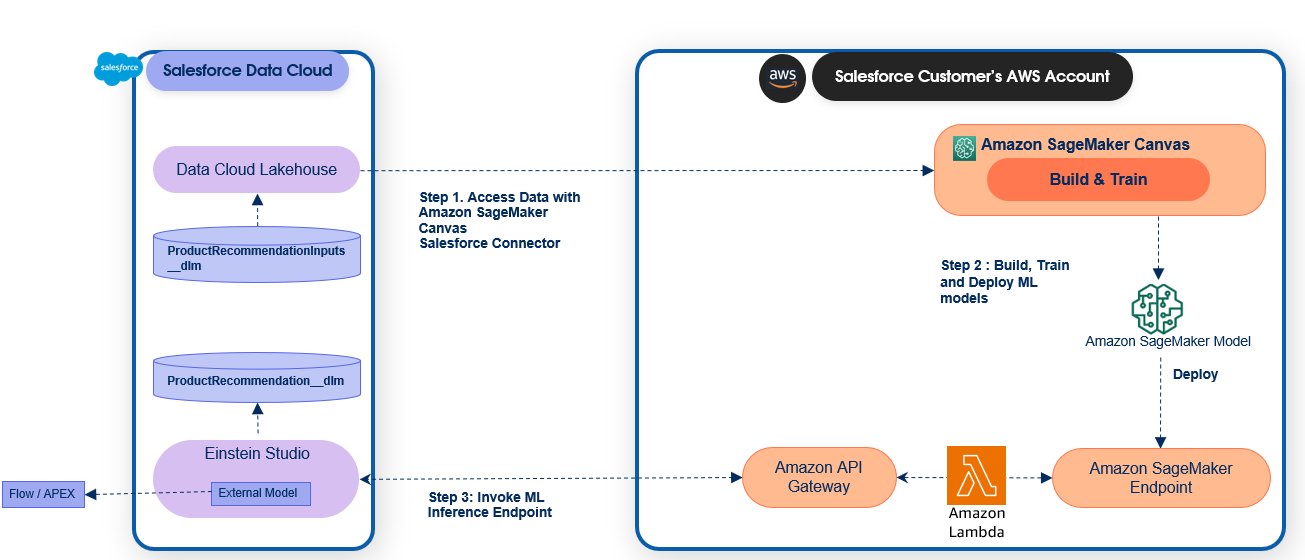
Prerequisites
Before you get started, complete the following prerequisite steps to create a SageMaker domain and enable SageMaker Canvas:
- Create an Amazon SageMaker Studio domain. For instructions, refer to Onboard to Amazon SageMaker Domain.
- Note down the domain ID and execution role that is created and will be used by your user profile. You add permissions to this role in subsequent steps.
The following screenshot shows the domain we created for this post.
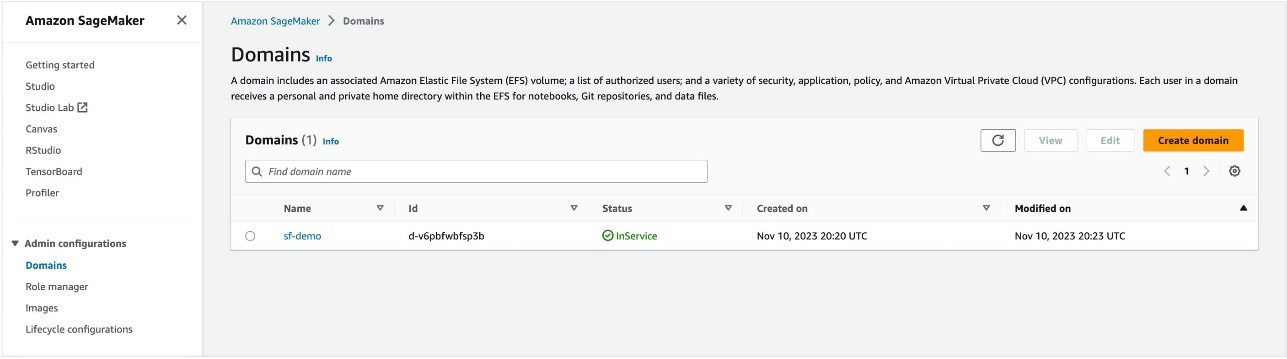
- Next, go to the user profile and choose Edit.
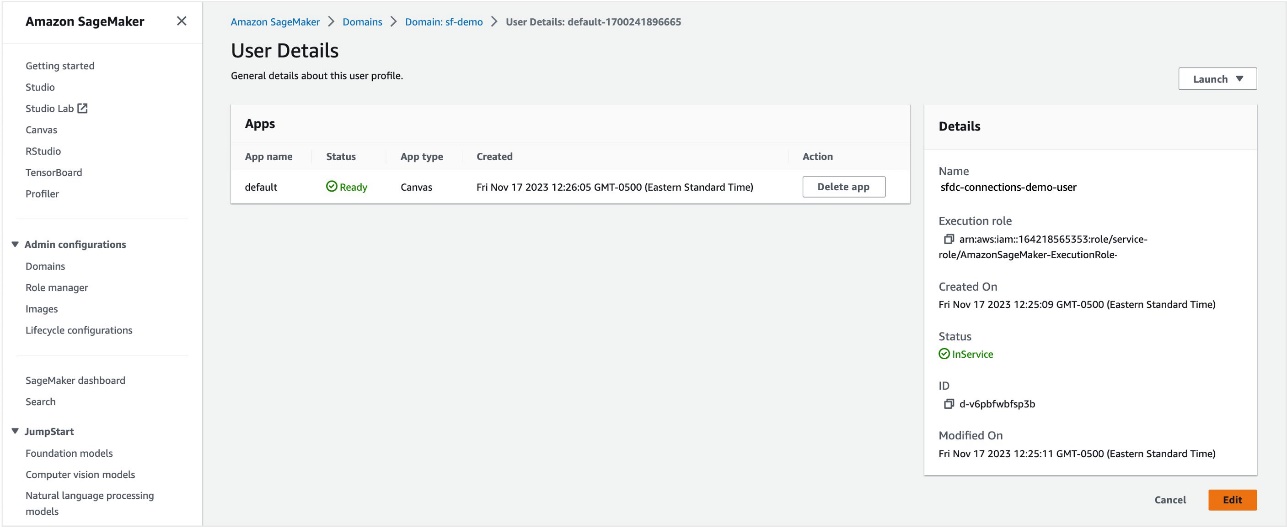
- Navigate to the Amazon SageMaker Canvas settings section and select Enable Canvas base permissions.
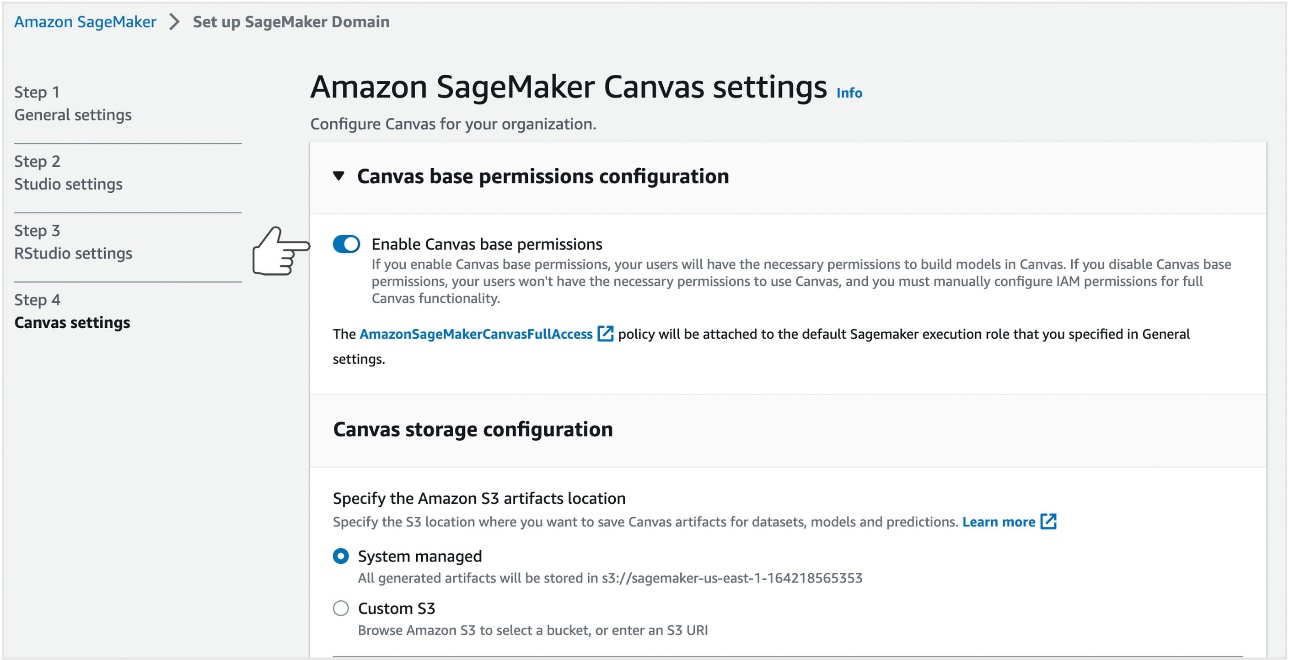
- Select Enable direct deployments of Canvas models and Enable model registry permissions for all users.
This allows SageMaker Canvas to deploy models to endpoints on the SageMaker console. These settings can be configured at the domain or user profile level. User profile settings take precedence over domain settings.
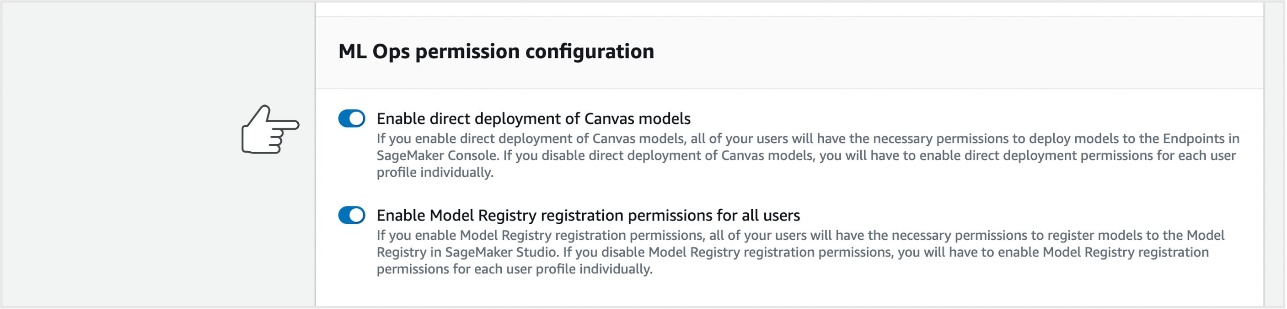
Create or update the Salesforce connected app
Next, we create a Salesforce connected app to enable the OAuth flow from SageMaker Canvas to Salesforce Data Cloud. Complete the following steps:
- Log in to Salesforce and navigate to Setup.
- Search for App Manager and create a new connected app.
- Provide the following inputs:
- For Connected App Name, enter a name.
- For API Name, leave as default (it’s automatically populated).
- For Contact Email, enter your contact email address.
- Select Enable OAuth Settings.
- For Callback URL, enter
https://<domain-id>.studio.<region>.sagemaker.aws/canvas/default/lab
, and provide the domain ID and Region from your SageMaker domain.
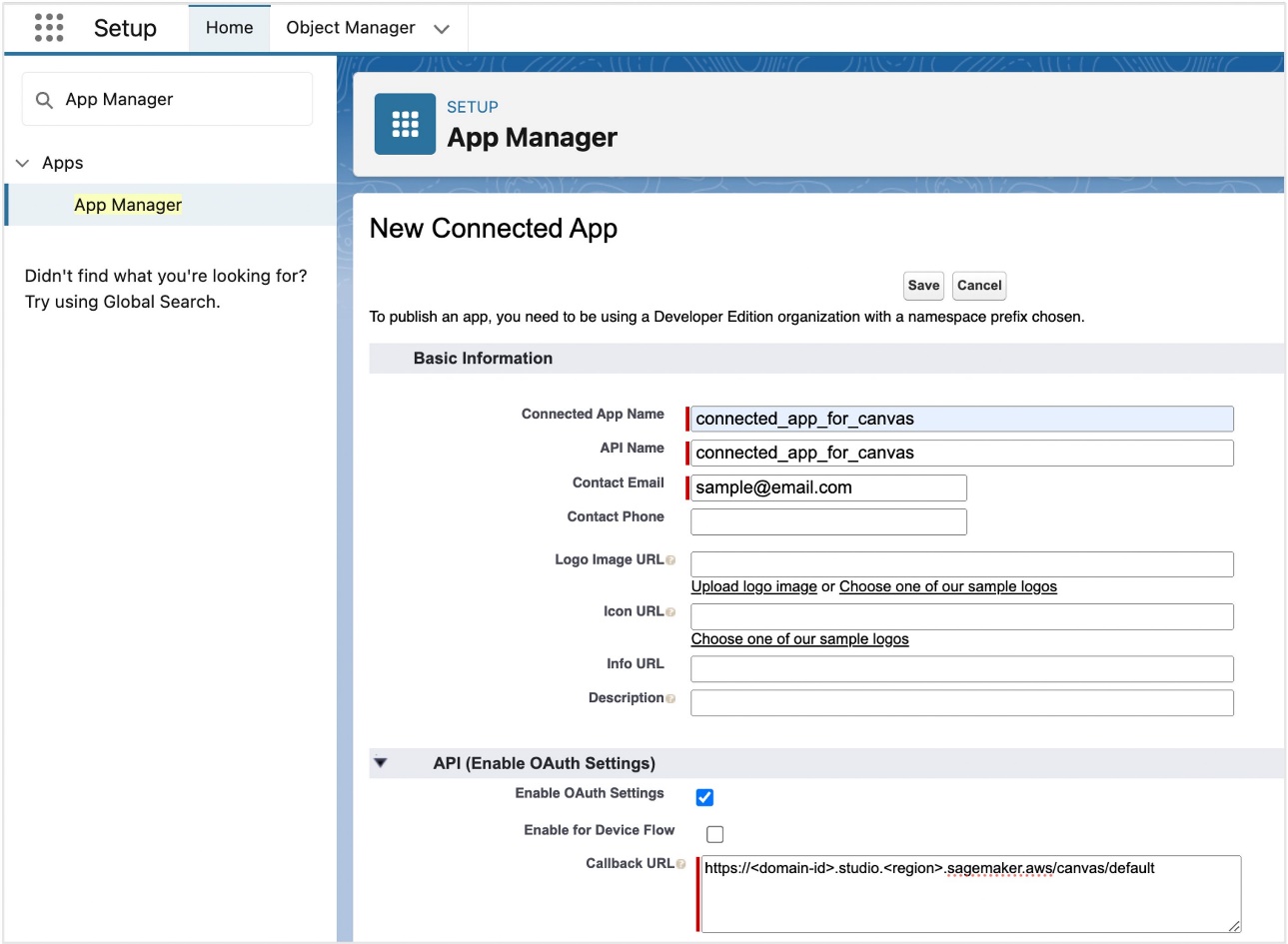
- Configure the following scopes on your connected app:
- Manage user data via APIs (
api
).
- Perform requests at any time (
refresh_token
, offline_access
).
- Perform ANSI SQL queries on Salesforce Data Cloud data (Data
Cloud_query_api
).
- Manage Data Cloud profile data (
Data Cloud_profile_api
).
- Access the identity URL service (
id
, profile
, email
, address
, phone
).
- Access unique user identifiers (
openid
).
- Set your connected app IP Relaxation setting to Relax IP restrictions.
Configure OAuth settings for the Salesforce Data Cloud connector
SageMaker Canvas uses AWS Secrets Manager to securely store connection information from the Salesforce connected app. SageMaker Canvas allows administrators to configure OAuth settings for an individual user profile or at the domain level. Note that you can add a secret to both a domain and user profile, but SageMaker Canvas looks for secrets in the user profile first.
To configure your OAuth settings, complete the following steps:
- Navigate to edit Domain or User Profile Settings in SageMaker Console.
- Choose Canvas Settings in the navigation pane.
- Under OAuth settings, for Data Source, choose Salesforce Data Cloud.
- For Secret setup, you can create a new secret or use an existing secret. For this example, we create a new secret and input the client ID and client secret from the Salesforce connected app.
For more details on enabling OAuth in SageMaker Canvas, refer to Set up OAuth for Salesforce Data Cloud.
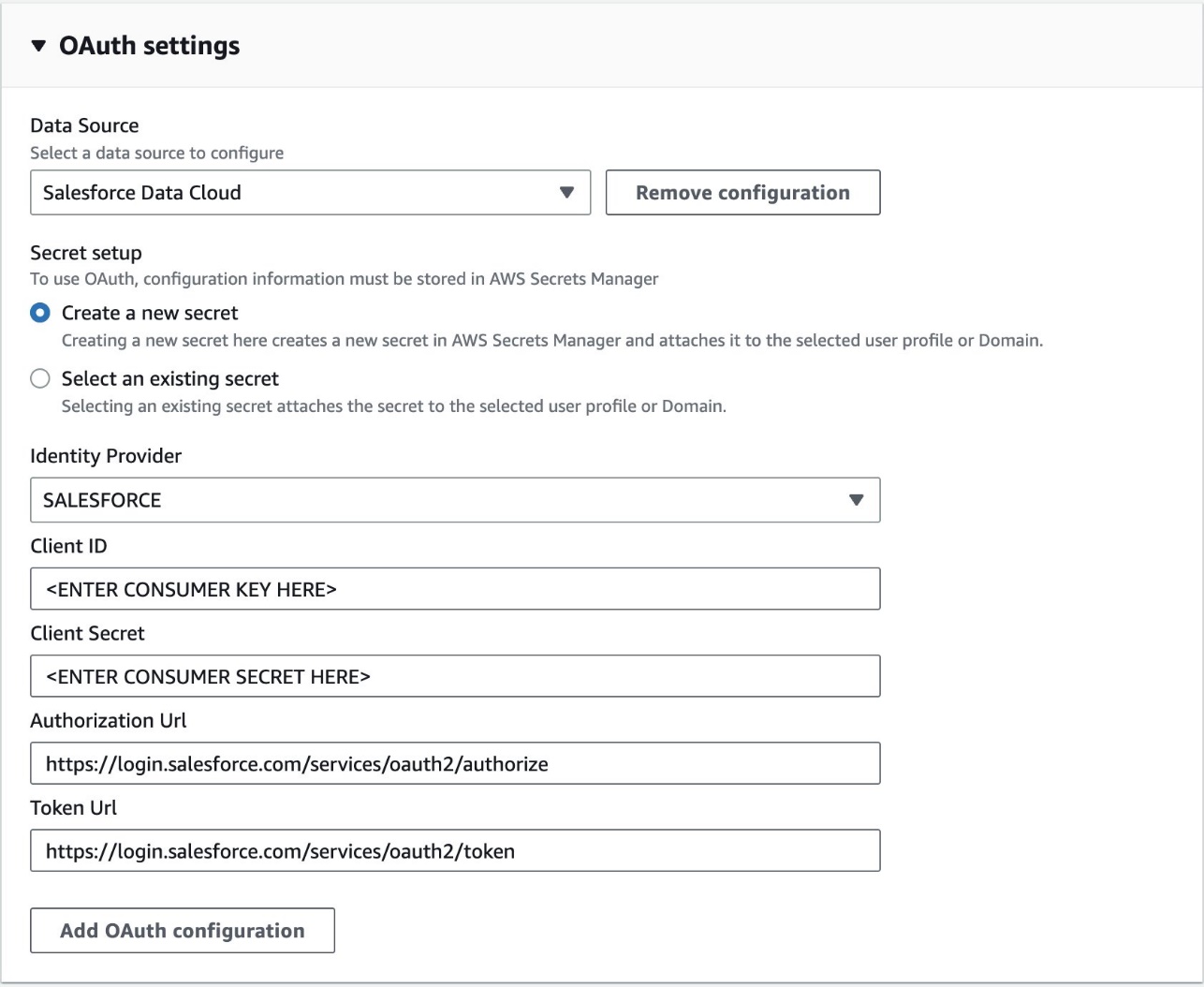
This completes the setup to enable data access from Salesforce Data Cloud to SageMaker Canvas to build AI and ML models.
Import data from Salesforce Data Cloud
To import your data, complete the following steps:
- From the user profile you created with your SageMaker domain, choose Launch and select Canvas.
The first time you access your Canvas app, it will take about 10 minutes to create.
- Choose Data Wrangler in the navigation pane.
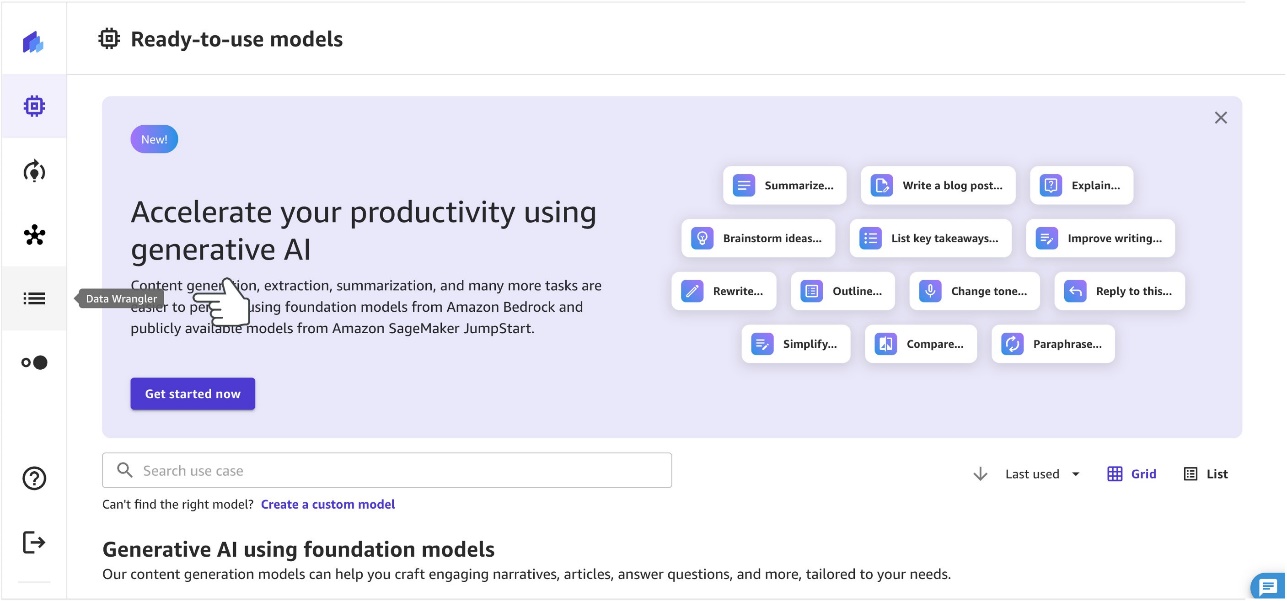
- On the Create menu, choose Tabular to create a tabular dataset.
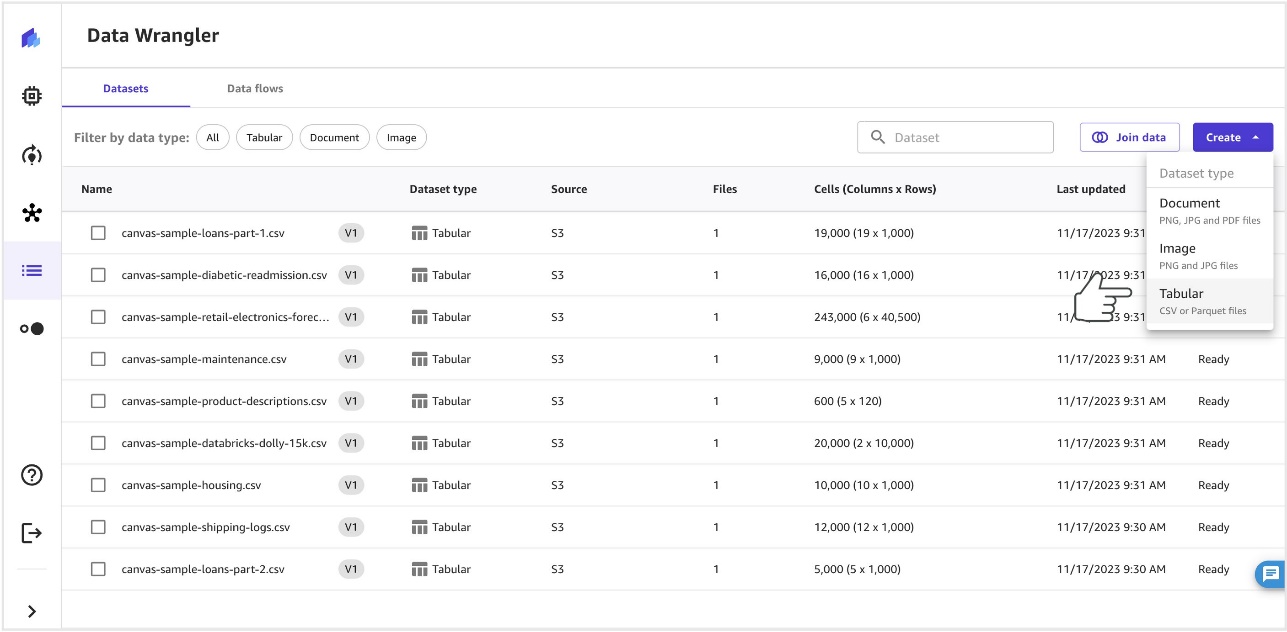
- Name the dataset and choose Create.
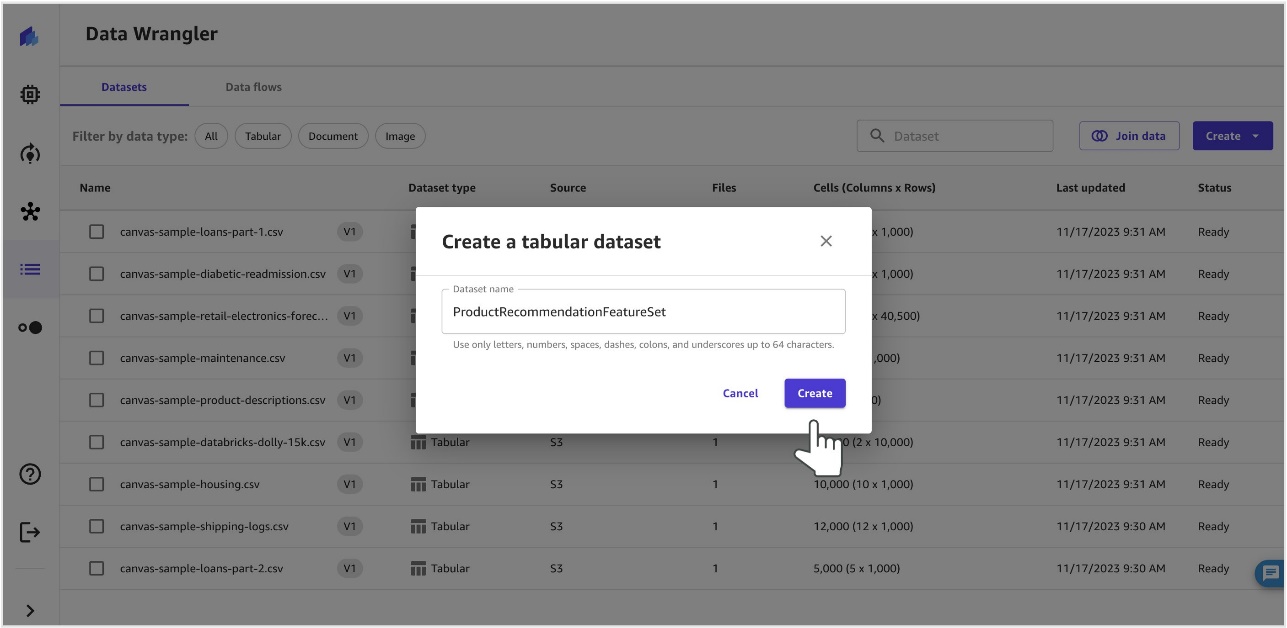
- For Data Source, choose Salesforce Data Cloud and Add Connection to import the data lake object.
If you’ve previously configured a connection to Salesforce Data Cloud, you will see an option to use that connection instead of creating a new one.
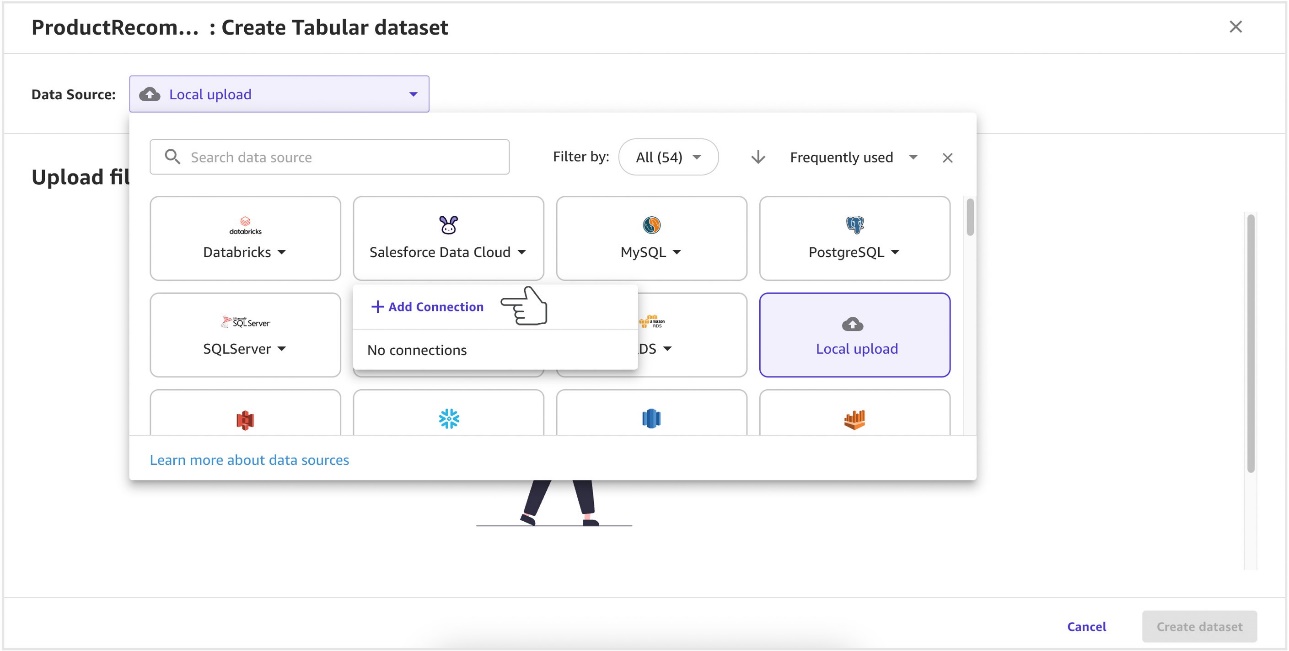
- Provide a name for a new Salesforce Data Cloud connection and choose Add connection.
It will take a few minutes to complete.
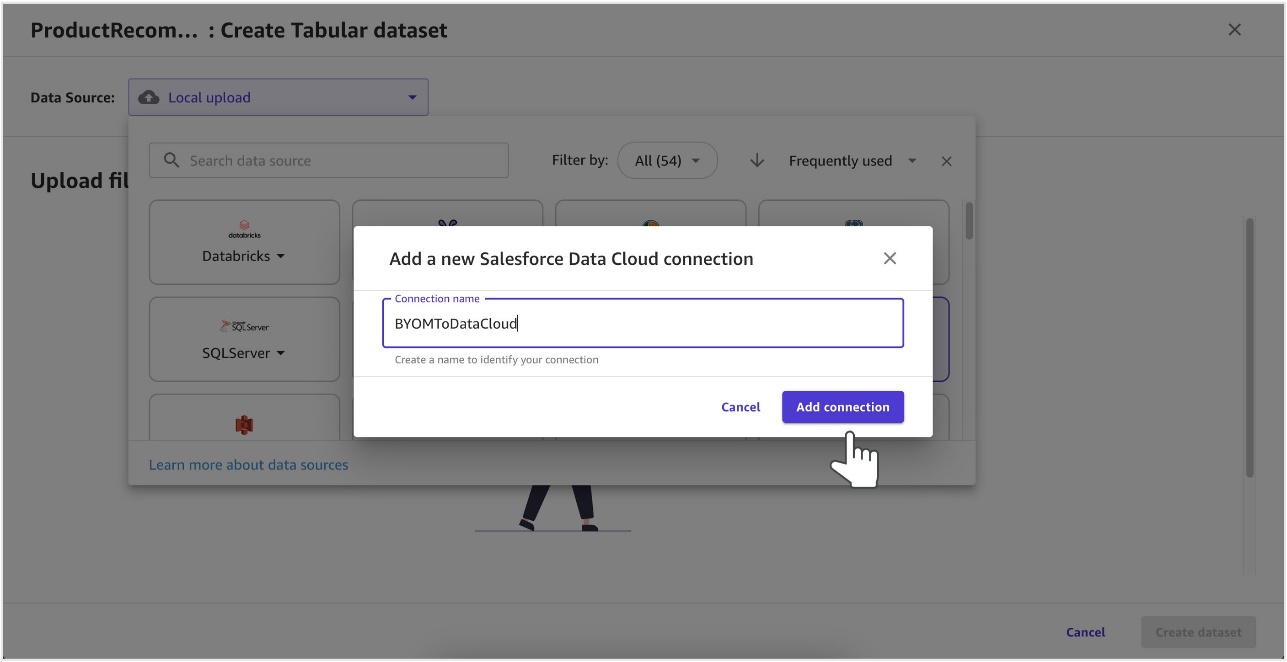
- You will be redirected to the Salesforce login page to authorize the connection.
After the login is successful, the request will be redirected back to SageMaker Canvas with the data Lake object listing.
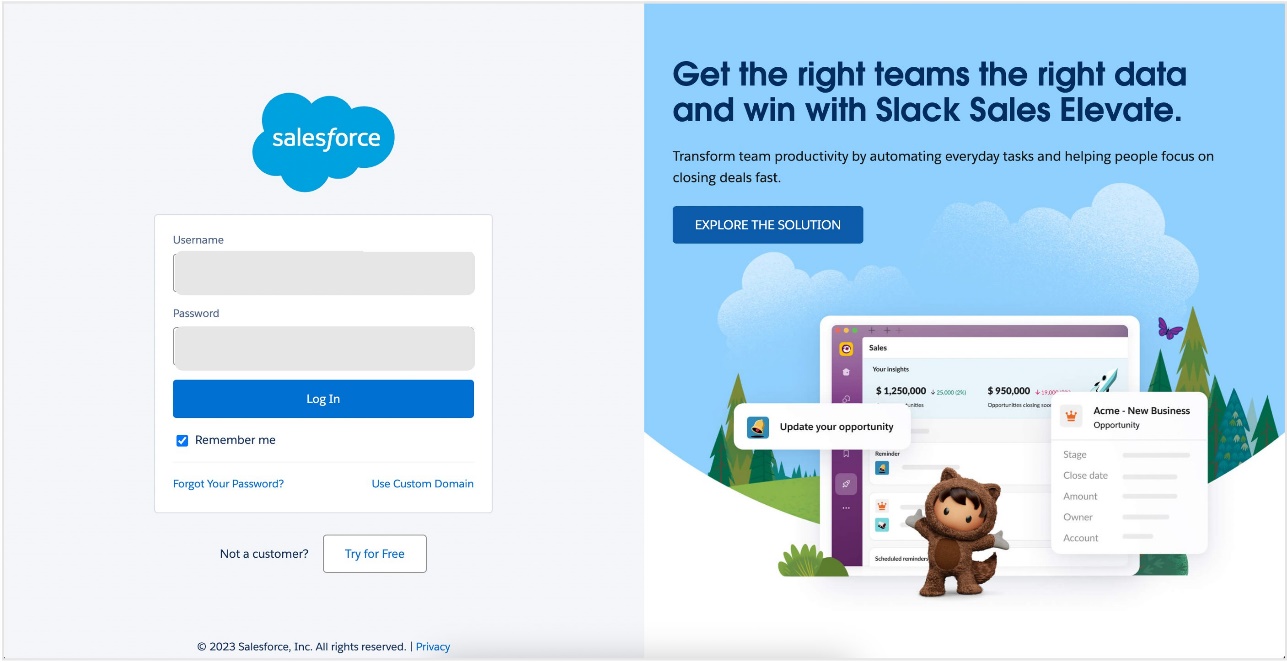
- Select the dataset that contains the features for model training that was uploaded via Amazon S3.
- Drag and drop the file, then choose Edit in SQL.
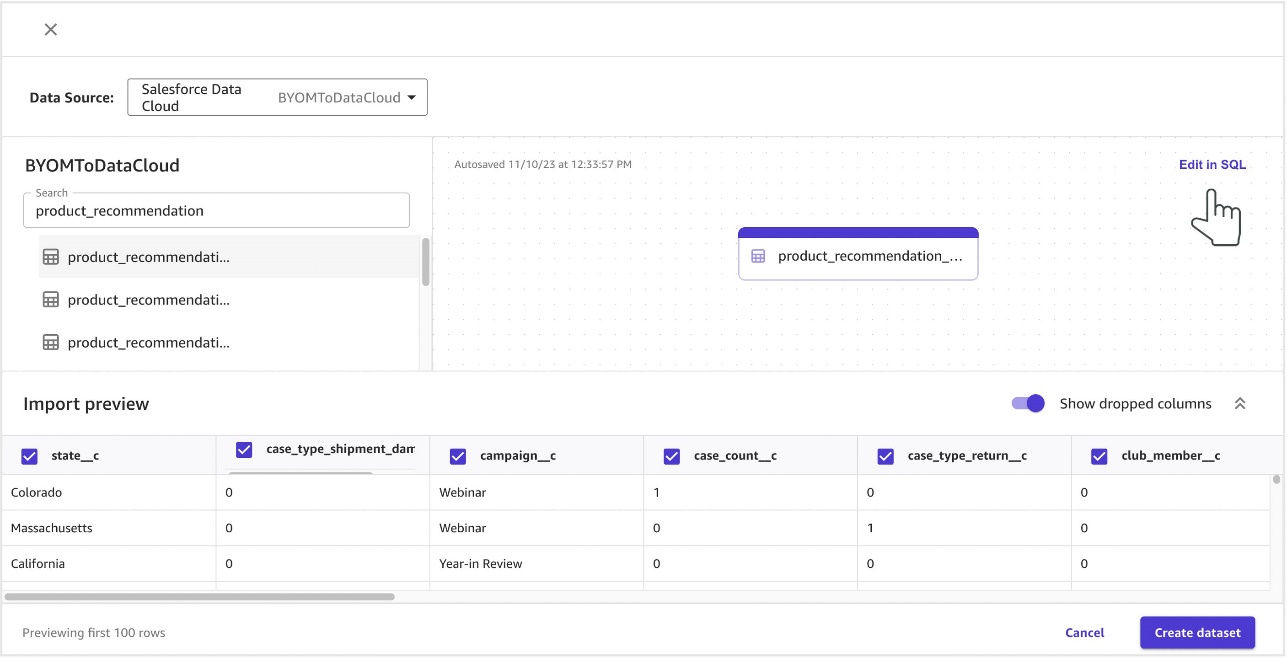
Salesforce adds a “__c
“ to all the Data Cloud object fields. As per SageMaker Canvas naming convention, ”__“
is not allowed in the field names.
- Edit the SQL to rename the columns and drop metadata that isn’t relevant for model training. Replace the table name with your object name.
SELECT "state__c" as state,
"case_type_shipment_damaged__c" as case_type_shipment_damaged,
"campaign__c" as campaign,
"engagement_score__c" as engagement_score,
"case_count__c" as case_count,
"case_type_return__c" as case_type_return,
"club_member__c" as club_member,
"pages_visited__c" as pages_visited,
"product_purchased__c" as product_purchased,
"clicks__c" as clicks,
"tenure__c" as tenure,
"month__c" as month FROM product_recommendation__dlm;
- Choose Run SQL and then Create dataset.
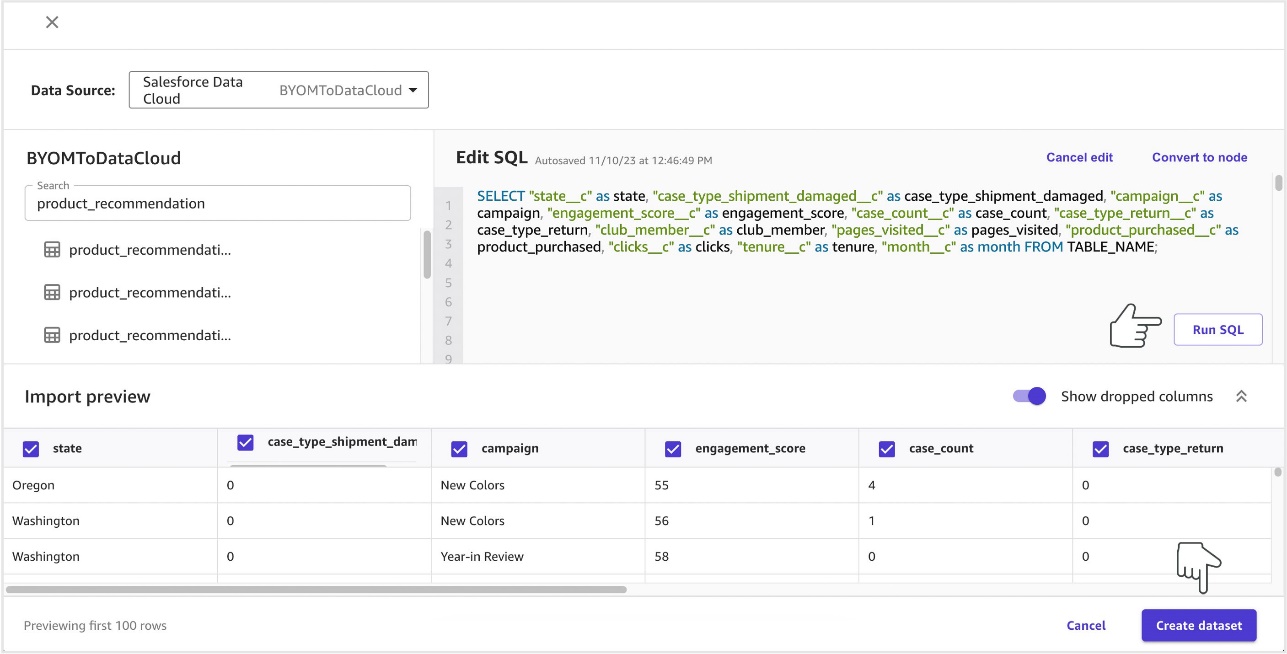
- Select the dataset and choose Create a model.
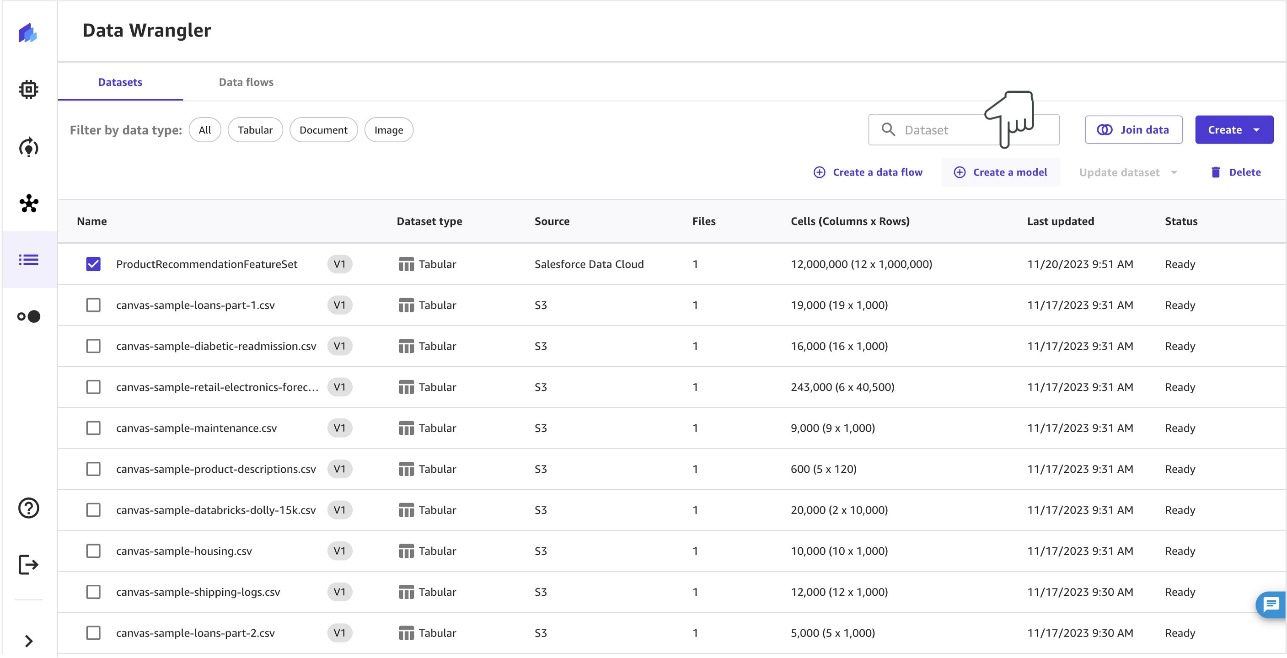
- To create a model to predict a product recommendation, provide a model name, choose Predictive analysis for Problem type, and choose Create.
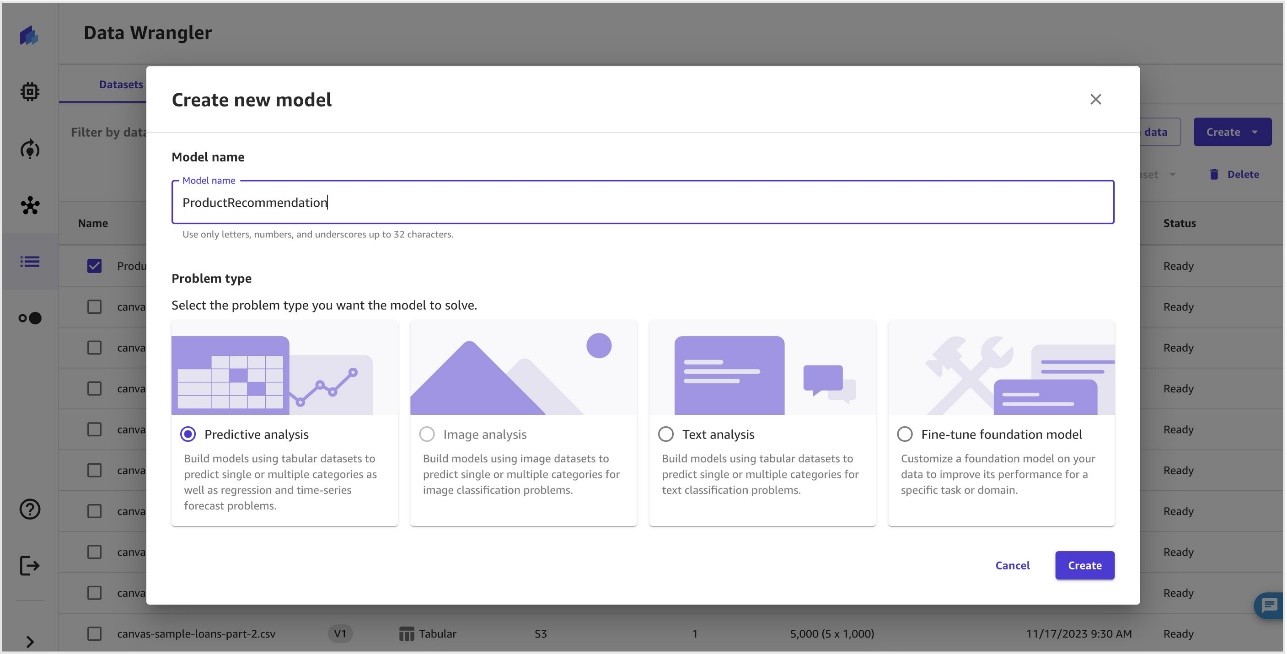
Build and train the model
Complete the following steps to build and train your model:
- After the model is launched, set the target column to
product_purchased
.
SageMaker Canvas displays key statistics and correlations of each column to the target column. SageMaker Canvas provides you with tools to preview your model and validate data before you begin building.
- Use the preview model feature to see the accuracy of your model and validate your dataset to prevent issues while building the model.
- After reviewing your data and making any changes to your dataset, choose your build type. The Quick build option may be faster, but it will only use a subset of your data to build a model. For the purpose of this post, we selected the Standard build option.
A standard build can take 2–4 hours to complete.
SageMaker Canvas automatically handles missing values in your dataset while it builds the model. It will also apply other data prep transformations for you to get the data ready for ML.
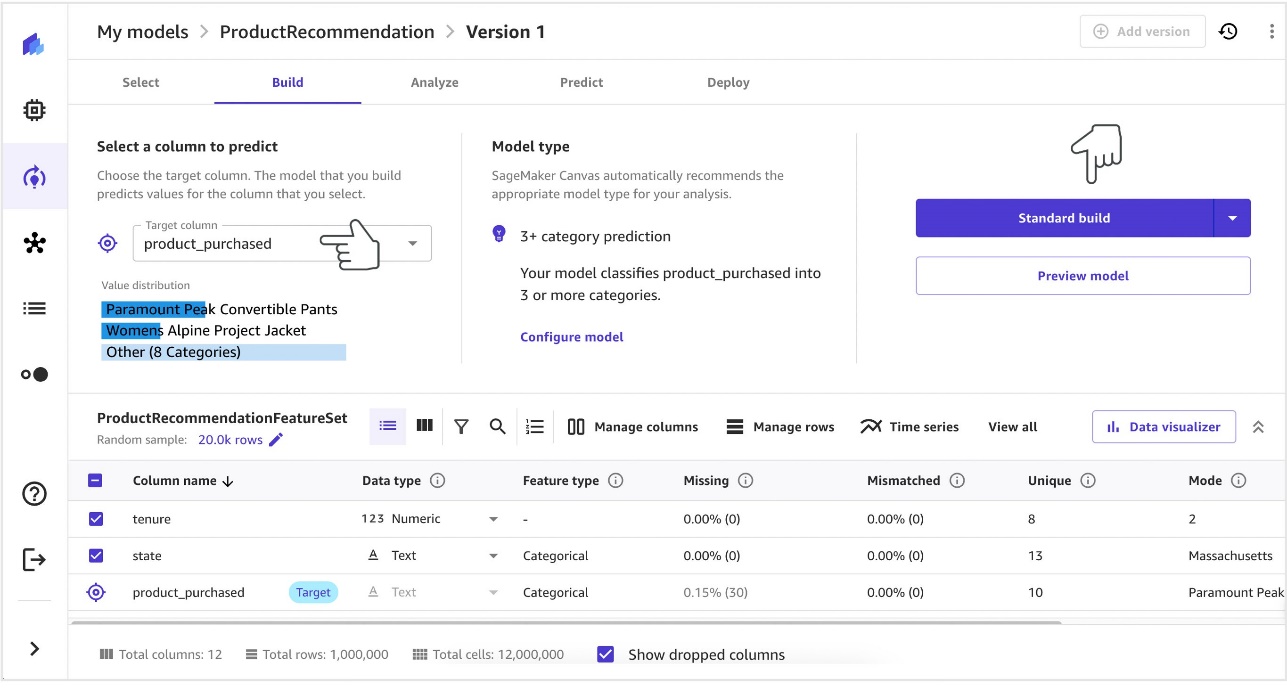
- After your model begins building, you can leave the page.
When the model shows as Ready on the My models page, it’s ready for analysis and predictions.
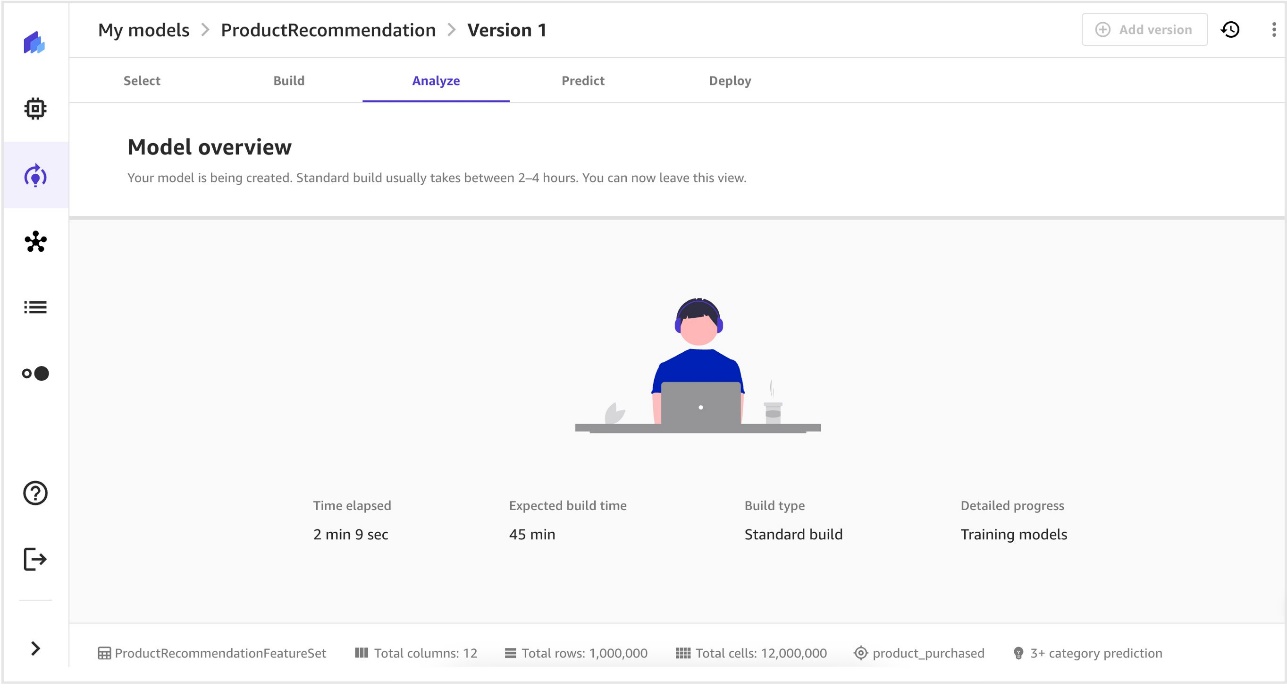
- After the model is built, navigate to My models, choose View to view the model you created, and choose the most recent version.
- Go to the Analyze tab to see the impact of each feature on the prediction.
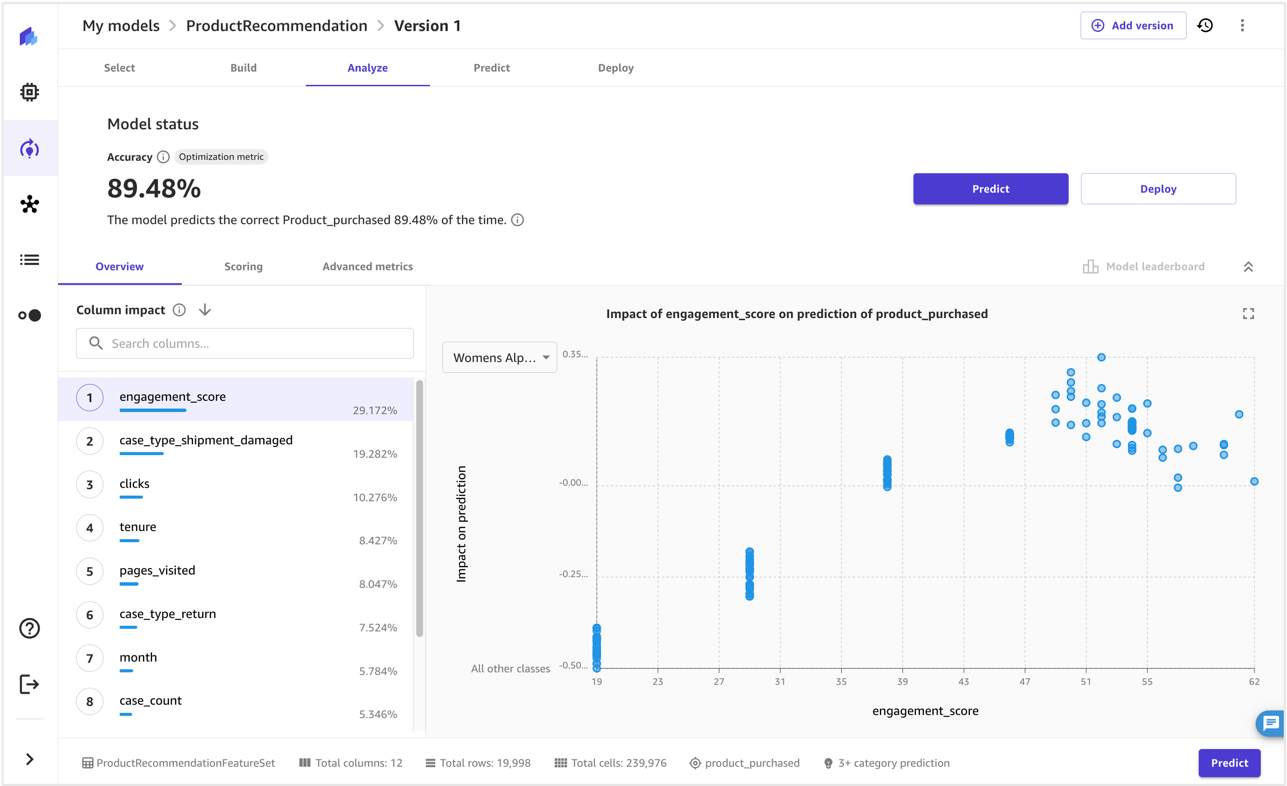
- For additional information on the model’s predictions, navigate to the Scoring tab.
- Choose Predict to initiate a product prediction.
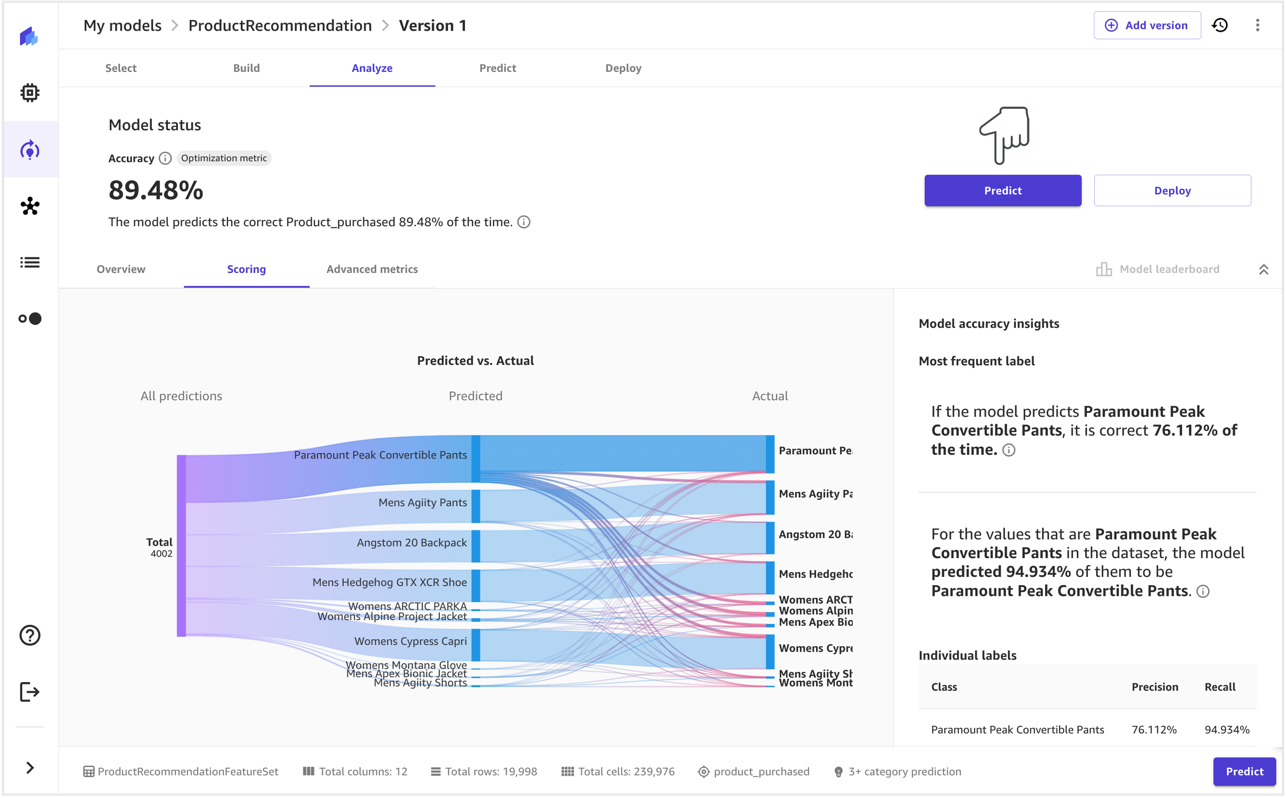
Deploy the model and make predictions
Complete the following steps to deploy your model and start making predictions:
- You can choose to make either batch or single predictions. For the purpose of this post, we choose Single prediction.
When you choose Single prediction, SageMaker Canvas displays the features that you can provide inputs for.
- You can change the values by choosing Update and view the real-time prediction.
The accuracy of the model as well as the impact of each feature for that specific prediction will be displayed.
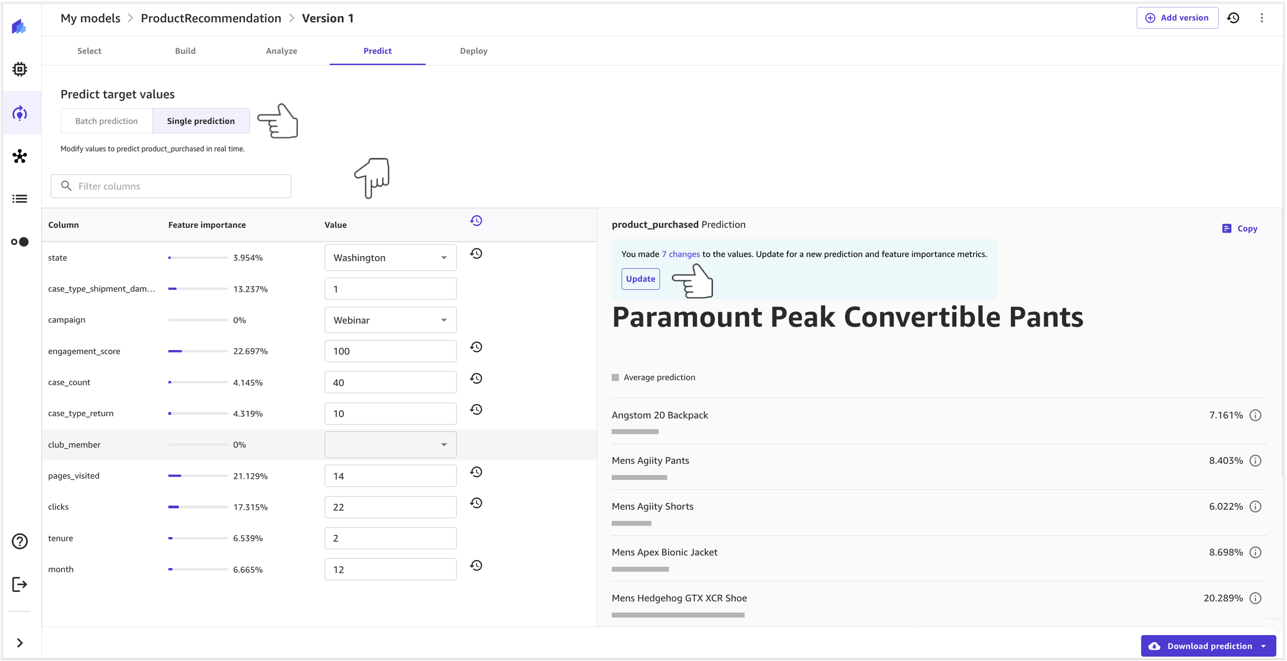
- To deploy the model, provide a deployment name, select an instance type and instance count, and choose Deploy.
Model deployment will take a few minutes.
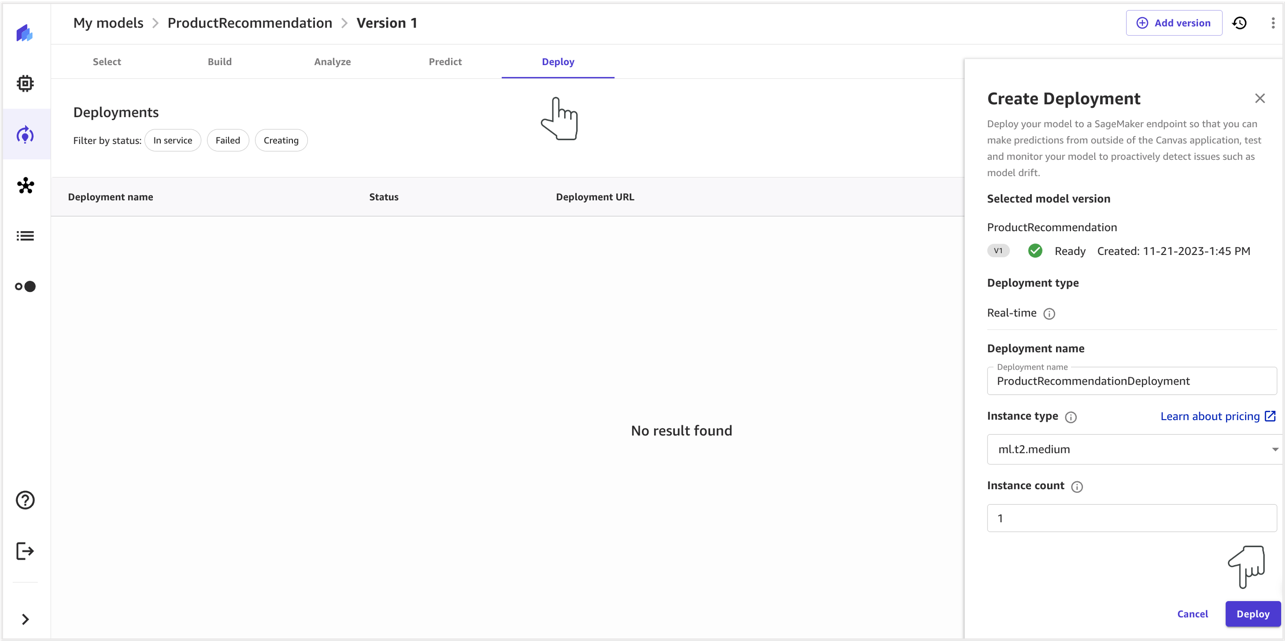
Model status is updated to In Service after the deployment is successful.
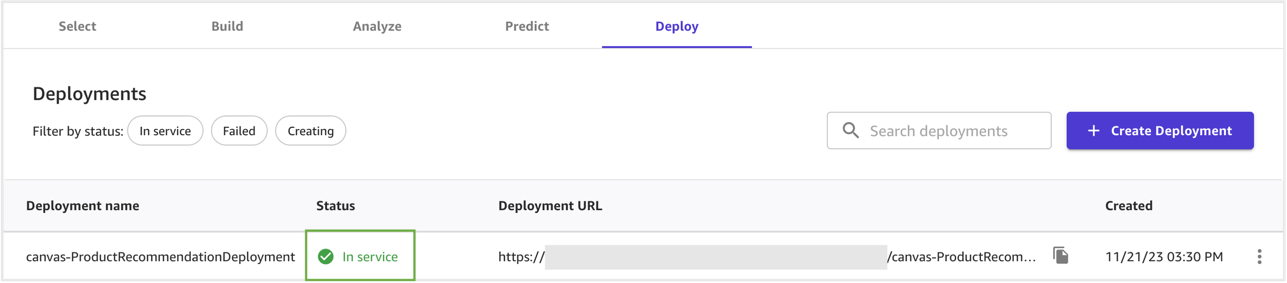
SageMaker Canvas provides an option to test the deployment.
- Choose View details.
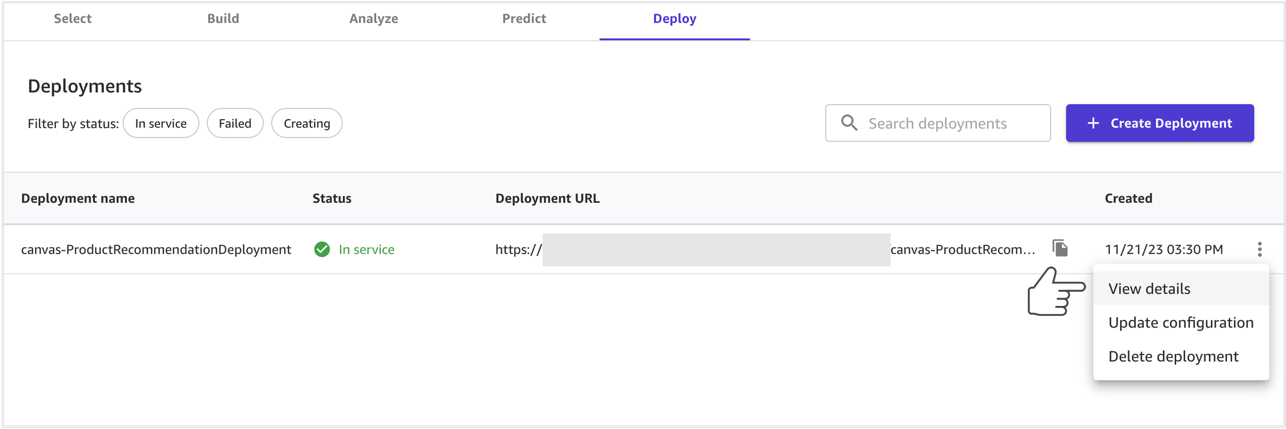
The Details tab provides the model endpoint details. Instance type, count, input format, response content, and endpoint are some of key details displayed.
- Choose Test deployment to test the deployed endpoint.
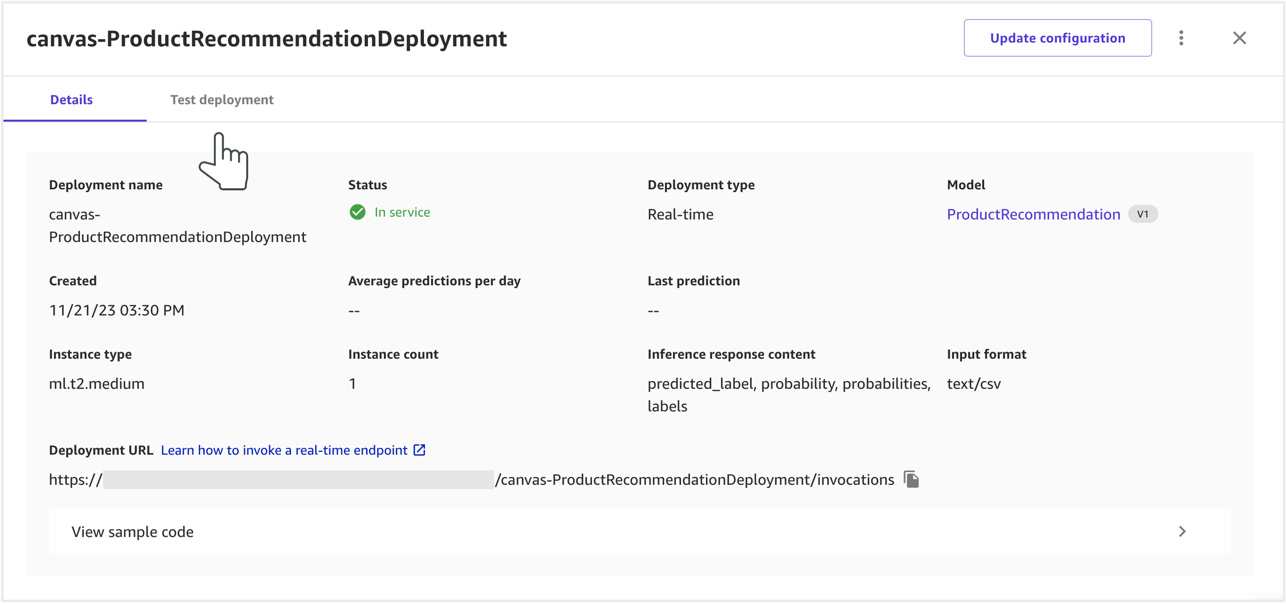
Similar to single prediction, the view displays the input features and provides an option to update and test the endpoint in real time.
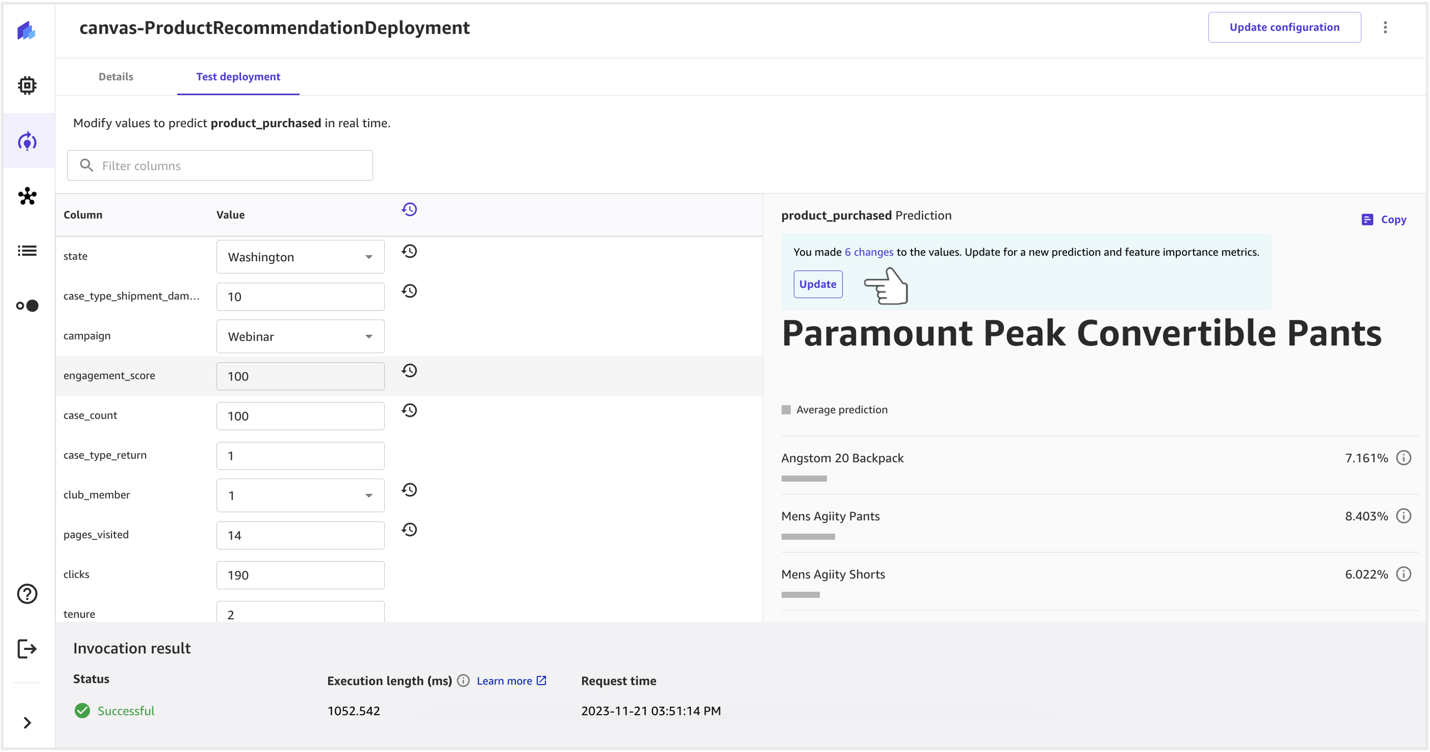
The new prediction along with the endpoint invocation result is returned to the user.
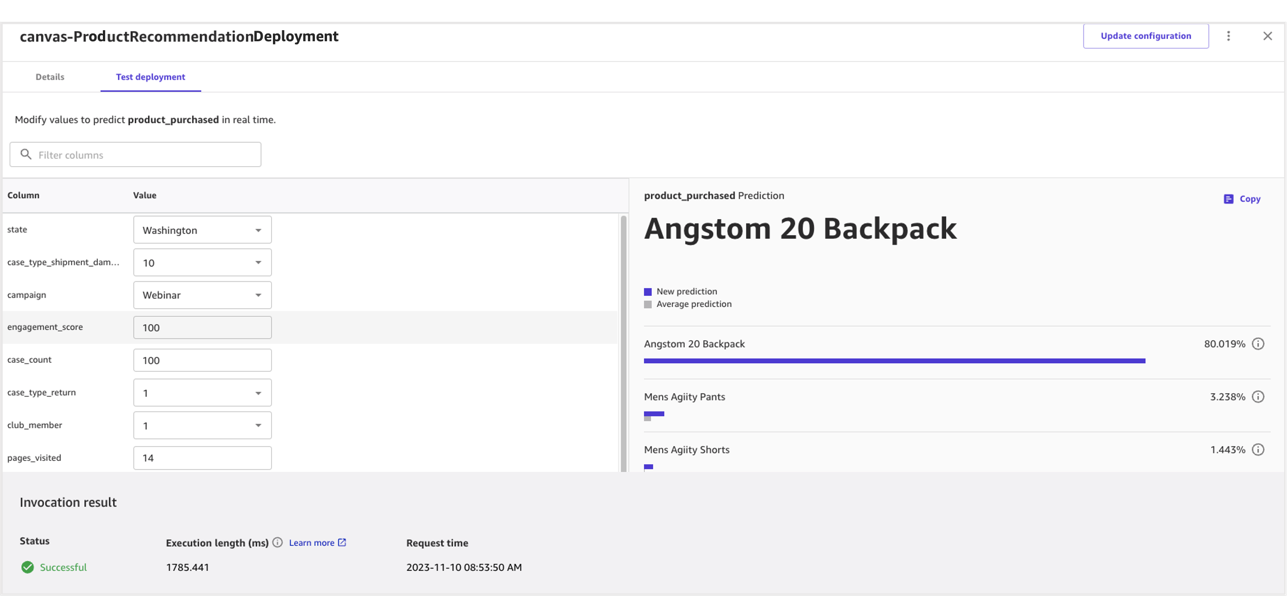
Create API to expose SageMaker Endpoint
To generate predictions that power business applications in Salesforce, you need to expose the SageMaker inference endpoint created by your SageMaker Canvas deployment via API Gateway and register it in Salesforce Einstein.
The request and response formats vary between Salesforce Einstein and SageMaker inference endpoint. You could either use API Gateway to perform the transformation or use AWS Lambda to transform the request and map the response. Refer to Call an Amazon SageMaker model endpoint using Amazon API Gateway and AWS Lambda to expose a SageMaker endpoint via Lambda and API Gateway.
The following code snippet is a Lambda function to transform the request and the response
import json
import boto3
import os
client = boto3.client("runtime.sagemaker")
endpoint = os.environ['SAGEMAKER_ENDPOINT_NAME']
prediction_label = 'product_purchased__c'
def lambda_handler(event, context):
features=[]
# Input Sample : {"instances": [{"features": ["Washington", 1, "New Colors", 1, 1, 1, 1, 1, 1, 1, 1]}, {"features": ["California", 1, "Web", 100, 1, 1, 100, 1, 10, 1, 1]}]}
for instance in event["instances"]:
features.append(','.join(map(str, instance["features"])))
body='n'.join(features)
response = client.invoke_endpoint(EndpointName=endpoint,ContentType="text/csv",Body=body,Accept="application/json")
response = json.loads(response['Body'].read().decode('utf-8'))
prediction_response={"predictions":[]}
for prediction in response.get('predictions'):
prediction_response['predictions'].append({prediction_label:prediction['predicted_label']})
return prediction_response
Update the endpoint
and prediction_label
values in the Lambda function based on your configuration.
- Add an environment variable
SAGEMAKER_ENDPOINT_NAME
to capture the SageMaker inference endpoint.
- Set the prediction label to match the model output JSON key that is registered in Einstein Studio.
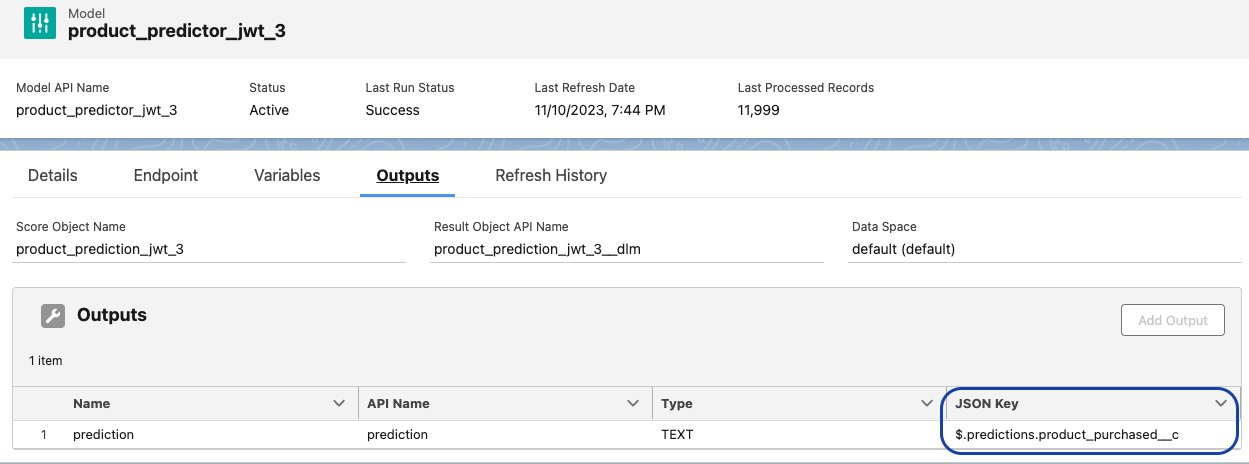
The default timeout for a Lambda function is 3 seconds. Depending on the prediction request input size, the SageMaker real-time inference API may take more than 3 seconds to respond.
- Increase the Lambda function timeout but keep it below the API Gateway default integration timeout, which is 29 seconds.
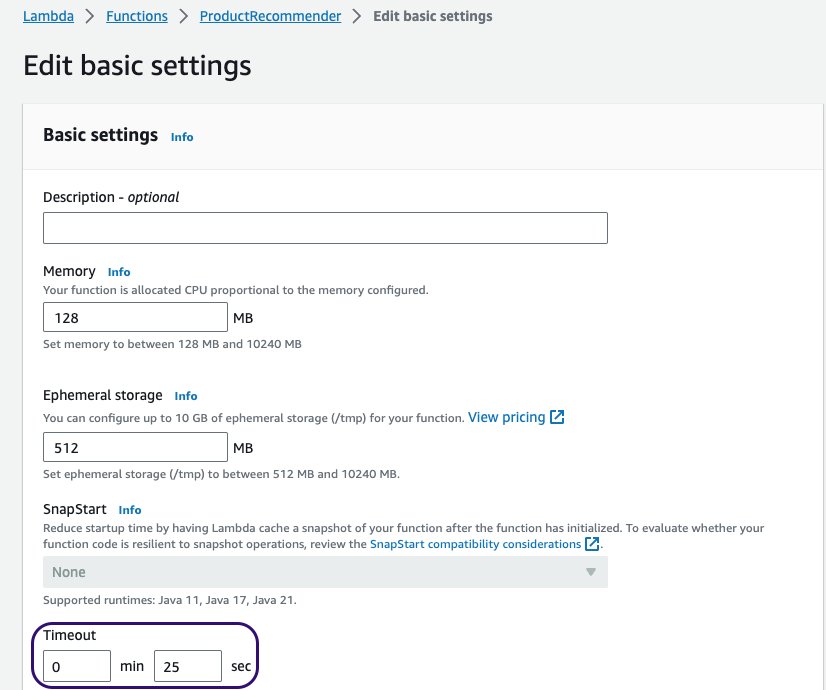
Register the model in Salesforce Einstein Studio
To register the API Gateway endpoint in Einstein Studio, refer to Bring Your Own AI Models to Data Cloud.
Conclusion
In this post, we explained how you can use SageMaker Canvas to connect to Salesforce Data Cloud and generate predictions through automated ML features without writing a single line of code. We demonstrated the SageMaker Canvas model build capability to conduct an early preview of your model performance before running the standard build that trains the model with the full dataset. We also showcased post-model creation activities like using the single predictions interface within SageMaker Canvas and understanding your predictions using feature importance. Next, we used the SageMaker endpoint created in SageMaker Canvas and made it available as an API so you can integrate it with Salesforce Einstein Studio and create powerful Salesforce applications.
In an upcoming post, we will show you how to use data from Salesforce Data Cloud in SageMaker Canvas to make data insights and preparation even more straightforward by using a visual interface and simple natural language prompts.
To get started with SageMaker Canvas, see SageMaker Canvas immersion day and refer to Getting started with Amazon SageMaker Canvas.
About the authors
Daryl Martis is the Director of Product for Einstein Studio at Salesforce Data Cloud. He has over 10 years of experience in planning, building, launching, and managing world-class solutions for enterprise customers, including AI/ML and cloud solutions. He has previously worked in the financial services industry in New York City. Follow him on Linkedin.
Rachna Chadha is a Principal Solutions Architect AI/ML in Strategic Accounts at AWS. Rachna is an optimist who believes that ethical and responsible use of AI can improve society in the future and bring economic and social prosperity. In her spare time, Rachna likes spending time with her family, hiking, and listening to music.
Ife Stewart is a Principal Solutions Architect in the Strategic ISV segment at AWS. She has been engaged with Salesforce Data Cloud over the last 2 years to help build integrated customer experiences across Salesforce and AWS. Ife has over 10 years of experience in technology. She is an advocate for diversity and inclusion in the technology field.
Ravi Bhattiprolu is a Sr. Partner Solutions Architect at AWS. Ravi works with strategic partners, Salesforce and Tableau, to deliver innovative and well-architected products and solutions that help joint customers realize their business objectives.
Miriam Lebowitz is a Solutions Architect in the Strategic ISV segment at AWS. She is engaged with teams across Salesforce, including Salesforce Data Cloud, and specializes in data analytics. Outside of work, she enjoys baking, traveling, and spending quality time with friends and family.
Read More