In his book The Book of Why, Judea Pearl advocates for teaching cause and effect principles to machines in order to enhance their intelligence. The accomplishments of deep learning are essentially just a type of curve fitting, whereas causality could be used to uncover interactions between the systems of the world under various constraints without testing hypotheses directly. This could provide answers that lead us to AGI (artificial generalized intelligence).
This solution proposes a causal inference framework using Bayesian networks to represent causal dependencies and draw causal conclusions based on observed satellite imagery and experimental trial data in the form of simulated weather and soil conditions. The case study is the causal relationship between nitrogen-based fertilizer application and the corn yields.
The satellite imagery is processed using purpose-built Amazon SageMaker geospatial capabilities and enriched with custom-built Amazon SageMaker Processing operations. The causal inference engine is deployed with Amazon SageMaker Asynchronous Inference.
In this post, we demonstrate how to create this counterfactual analysis using Amazon SageMaker JumpStart solutions.
Solution overview
The following diagram shows the architecture for the end-to-end workflow.
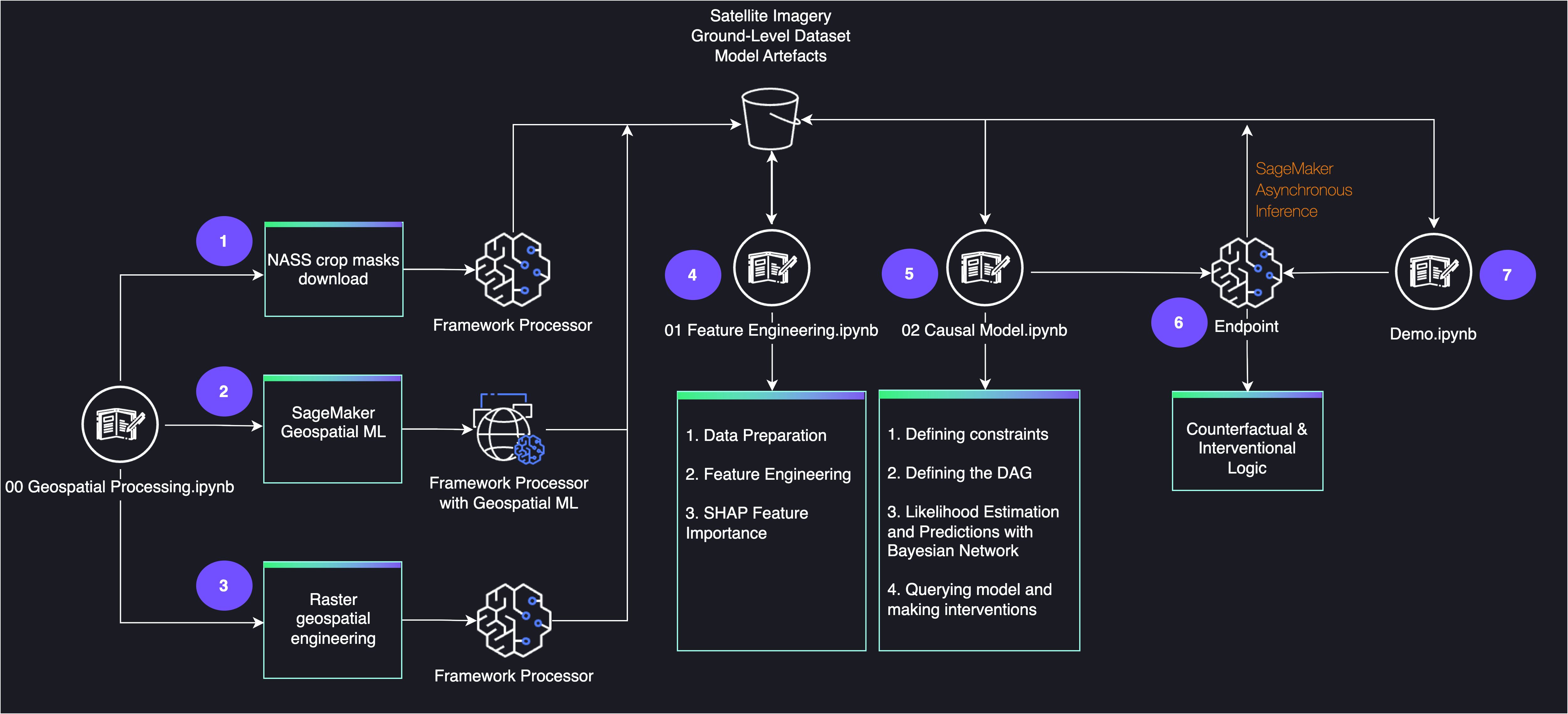
Prerequisites
You need an AWS account to use this solution.
To run this JumpStart 1P Solution and have the infrastructure deployed to your AWS account, you need to create an active Amazon SageMaker Studio instance (refer to Onboard to Amazon SageMaker Domain). When your Studio instance is ready, follow the instructions in SageMaker JumpStart to launch the Crop Yield Counterfactuals solution.
Note that this solution is currently available in the US West (Oregon) Region only.
Causal inference
Causality is all about understanding change, but how to formalize this in statistics and machine learning (ML) is not a trivial exercise.
In this crop yield study, the nitrogen added as fertilizer and the yield outcomes might be confounded. Similarly, the nitrogen added as a fertilizer and the nitrogen leaching outcomes could be confounded as well, in the sense that a common cause can explain their association. However, association is not causation. If we know which observed factors confound the association, we account for them, but what if there are other hidden variables responsible for confounding? Reducing the amount of fertilizer won’t necessarily reduce residual nitrogen; similarly, it might not drastically diminish the yield, whereas the soil and climatic conditions could be the observed factors that confound the association. How to handle confounding is the central problem of causal inference. A technique introduced by R. A. Fisher called randomized controlled trial aims to break possible confounding.
However, in the absence of randomized control trials, there is a need for causal inference purely from observational data. There are ways to connect the causal questions to data in observational studies by writing the causal graphical model on what we postulate as how things happen. This involves claiming the corresponding traverses will capture the corresponding dependencies, while satisfying the graphical criterion for conditional ignorability (to what extent we can treat causation as association based on the causal assumptions). After we have postulated the structure, we can use the implied invariances to learn from observational data and plug in causal questions, inferring causal claims without randomized control trials.
This solution uses both data from simulated randomized control trials (RCTs) as well as observational data from satellite imagery. A series of simulations conducted over thousands of fields and multiple years in Illinois (United States) are used to study the corn response to increasing nitrogen rates for a broad combination of weather and soil variation seen in the region. It addresses the limitation of using trial data limited in the number of soils and years it can explore by using crop simulations of various farming scenarios and geographies. The database was calibrated and validated using data from more than 400 trials in the region. Initial nitrogen concentration in the soil was set randomly among a reasonable range.
Additionally, the database is enhanced with observations from satellite imagery, whereas zonal statistics are derived from spectral indices in order to represent spatio-temporal changes in vegetation, seen across geographies and phenological phases.
Causal inference with Bayesian networks
Structural causal models (SCMs) use graphical models to represent causal dependencies by incorporating both data-driven and human inputs. A particular type of structure causal model called Bayesian networks is proposed to model the crop phenology dynamics using probabilistic expressions by representing variables as nodes and relationships between variables as edges. Nodes are indicators of crop growth, soil and weather conditions, and the edges between them represent spatio-temporal causal relationships. The parent nodes are field-related parameters (including the day of sowing and area planted), and the child nodes are yield, nitrogen uptake, and nitrogen leaching metrics.
For more information, refer to the database characterization and the guide for identifying the corn growth stages.
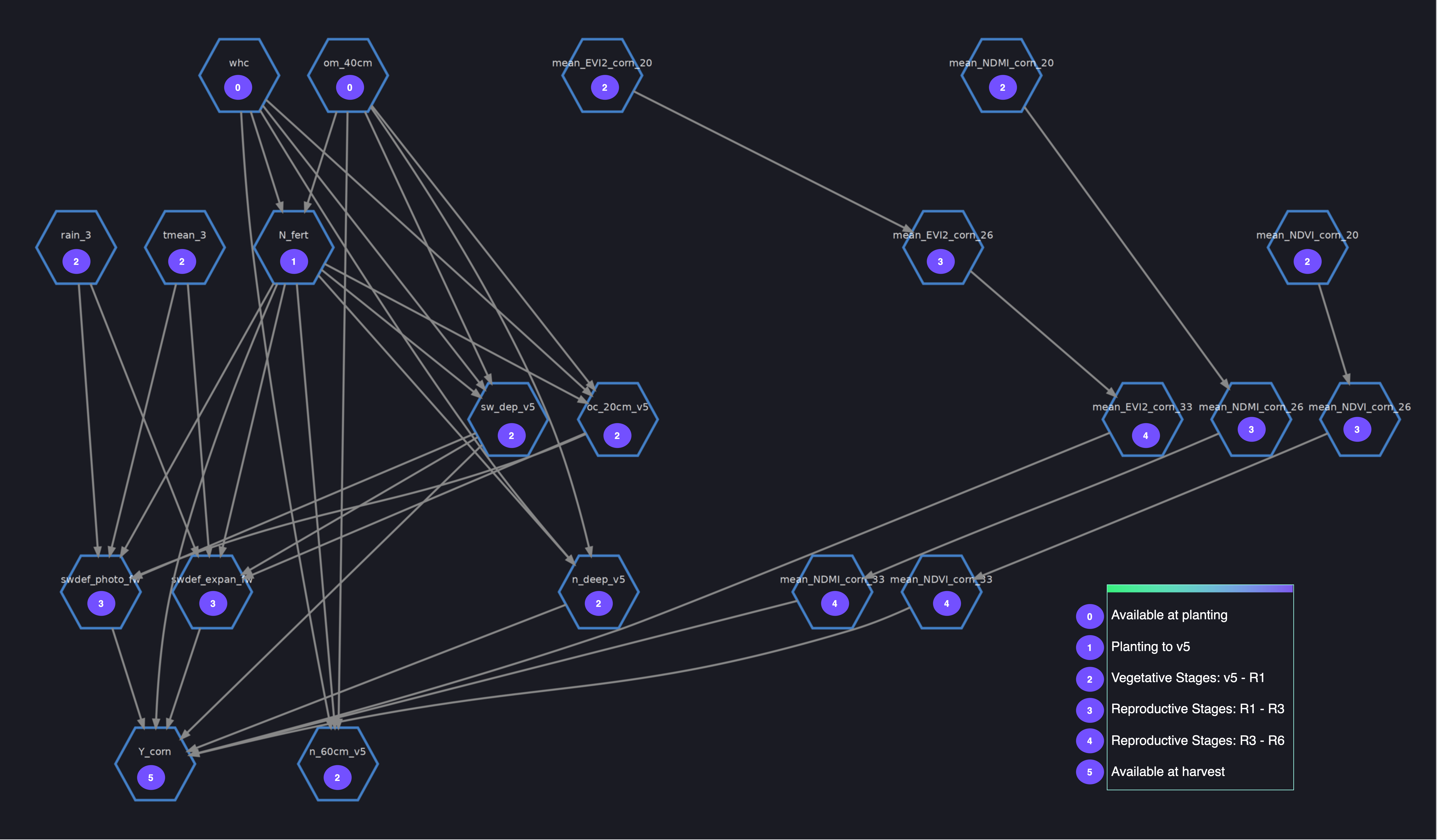
A few steps are required to build a Bayesian networks model (with CausalNex) before we can use it for counterfactual and interventional analysis. The structure of the causal model is initially learned from data, whereas subject matter expertise (trusted literature or empirical beliefs) is used to postulate additional dependencies and independencies between random variables and intervention variables, as well as asserting the structure is causal.
Using NO TEARS, a continuous optimization algorithm for structure learning, the graph structure describing conditional dependencies between variables is learned from data, with a set of constraints imposed on edges, parent nodes, and child nodes that are not allowed in the causal model. This preserves the temporal dependencies between variables. See the following code:
"""
tabu_edges: Imposing edges that are not allowed in the causal model
tabu_parents: Imposing parent nodes that are not allowed in the causal model
tabu_child: Imposing child nodes that are not allowed in the causal model
"""
from causalnex.structure.notears import from_pandas
g_learned = from_pandas(
X,
tabu_edges=tabu_edges,
tabu_parent_nodes=tabu_parents,
tabu_child_nodes=tabu_child,
max_iter=100,
)
The next step encodes domain knowledge in models and captures phenology dynamics, while avoiding spurious relationships. Multicollinearity analysis, variation inflation factor analysis, and global feature importance using SHAP analysis are conducted to extract insights and constraints on water stress variables (expansion, phenology, and photosynthesis around flowering), weather and soil variables, spectral indices, and the nitrogen-based indicators:
"""
edges: Modifying the structure by imposing constraints on edges
"""
from causalnex.structure import StructureModel
g = StructureModel()
g.add_edges_from(
edges,
origin="expert"
)
Bayesian networks in CausalNex support only discrete distributions. Any continuous features, or features with a large number of categories, are discretized prior to fitting the Bayesian network:
from causalnex.discretiser.discretiser_strategy import (
DecisionTreeSupervisedDiscretiserMethod,
MDLPSupervisedDiscretiserMethod
)
discretiser = DecisionTreeSupervisedDiscretiserMethod(
mode="single",
tree_params={"max_depth": 2, "random_state": 2022},
)
discretiser.fit(
feat_names=features,
dataframe=df,
target_continuous=True,
target=target,
)
After the structure is reviewed, the conditional probability distribution of each variable given its parents can be learned from data, in a step called likelihood estimation:
from causalnex.network import BayesianNetwork
bn = BayesianNetwork(g)
bn = bn.fit_node_states(discretised_data)
bn = bn.fit_cpds(
train,
method="BayesianEstimator",
bayes_prior="K2",
)
Finally, the structure and likelihoods are used to perform observational inference on the fly, following a deterministic Junction Tree algorithm (JTA), and making interventions using do-calculus. SageMaker Asynchronous Inference allows queuing incoming requests and processes them asynchronously. This option is ideal for both observational and counterfactual inference scenarios, where the process can’t be parallelized, thereby taking significant time to update the probabilities throughout the network, although multiple queries can be run in parallel. See the following code:
"""
Query the marginal likelihood of states in the graph given some observations.
These observations can be made anywhere in the network,
and their impact will be propagated through to the node of interest.
"""
from causalnex.inference import InferenceEngine
ie = InferenceEngine(bn)
pseudo_observation = [{"day_sow":0}, {"day_sow":1}, {"day_sow":2}]
marginals_multi = ie.query(
pseudo_observation,
parallel=True,
num_cores=multiprocessing.cpu_count(),
)
# distribution before intervention
marginals_before = ie.query()["Y_corn"]
# updating a node distribution
ie.do_intervention("N_fert", 0)
# effect of do on marginals
marginals_after = ie.query()["Y_corn"]
# Resetting the node distribution
ie.reset_do("N_fert")
For further details, refer to the inference script.
The causal model notebook is a step-by-step guide on running the preceding steps.
Geospatial data processing
Earth Observation Jobs (EOJs) are chained together to acquire and transform satellite imagery, whereas purpose-built operations and pre-trained models are used for cloud removal, mosaicking, band math operations, and resampling. In this section, we discuss in more detail the geospatial processing steps.
Area of interest
In the following figure, green polygons are the selected counties, the orange grid is the database map (a grid of 10 x 10 km cells where trials are conducted in the region), and the grid of grayscale squares is the 100 km x 100 km Sentinel-2 UTM tiling grid.
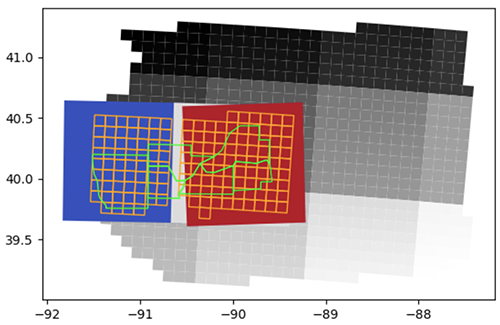
Spatial files are used to map the simulated database with corresponding satellite imagery, overlaying polygons of 10 km x 10 km cells that divide the state of Illinois (where trials are conducted in the region), counties polygons, and 100 km x 100 km Sentinel-2 UTM tiles. To optimize the geospatial data processing pipeline, a few nearby Sentinel-2 tiles are first selected. Next, the aggregated geometries of tiles and cells are overlayed in order to obtain the region of interest (RoI). The counties and the cell IDs that are fully observed within the RoI are selected to form the polygon geometry passed onto the EOJs.
Time range
For this exercise, the corn phenology cycle is divided into three stages: the vegetative stages v5 to R1 (emergence, leaf collars, and tasseling), the reproductive stages R1 to R4 (silking, blister, milk, and dough) and the reproductive stages R5 (dented) and R6 (physiological maturity). Consecutive satellite visits are acquired for each phenology stage within a time range of 2 weeks and a predefined area of interest (selected counties), enabling spatial and temporal analysis of satellite imagery. The following figure illustrates these metrics.
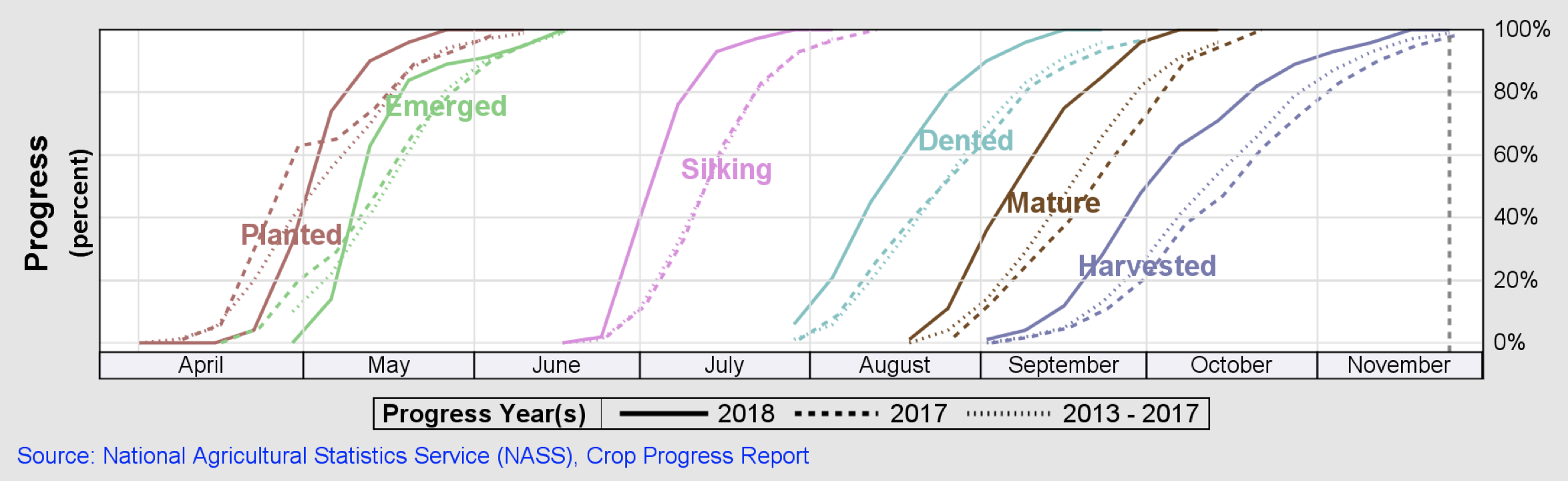
Cloud removal
Cloud removal for Sentinel-2 data uses an ML-based semantic segmentation model to identify clouds in the image, where cloudy pixels are replaced by with value -9999 (nodata value):
request_polygon_coordinates = [[(-90.571754, 39.839326), (-90.893651, 39.84092), (-90.916609, 39.845075), (-90.916071, 39.757168), (-91.147678, 39.75707), (-91.265848, 39.757258), (-91.365125, 39.758723), (-91.367962, 39.759124), (-91.365396, 39.777266), (-91.432919, 39.840554), (-91.446385, 39.870394), (-91.455887, 39.945538), (-91.460287, 39.980333), (-91.494865, 40.037421), (-91.510322, 40.127994), (-91.512974, 40.181062), (-91.510332, 40.201142), (-91.258828, 40.197299), (-90.911969, 40.193088), (-90.909756, 40.284394), (-90.450227, 40.276335), (-90.451502, 40.188892), (-90.199556, 40.183945), (-90.118966, 40.235263), (-90.033026, 40.377806), (-89.92468, 40.435921), (-89.717104, 40.435655), (-89.714927, 40.319218), (-89.602979, 40.320129), (-89.601604, 40.122432), (-89.578289, 39.976127), (-89.698259, 39.975309), (-89.701864, 39.916787), (-89.994506, 39.901925), (-89.994405, 39.87286), (-90.583534, 39.87675), (-90.582435, 39.854574), (-90.571754, 39.839326)]]
start_time = '2018-08-15T00:00:00Z'
end_time = '2018-09-15T00:00:00Z'
eoj_input_config = {
"RasterDataCollectionQuery": {
"RasterDataCollectionArn": 'arn:aws:sagemaker-geospatial:us-west-2:378778860802:raster-data-collection/public/nmqj48dcu3g7ayw8',
"AreaOfInterest": {
"AreaOfInterestGeometry": {
"PolygonGeometry": {"Coordinates": request_polygon_coordinates}
}
},
"TimeRangeFilter": {"StartTime": start_time, "EndTime": end_time},
"PropertyFilters": {
"Properties": [{"Property": {"EoCloudCover":
{"LowerBound": 0, "UpperBound": 10}}}],
"LogicalOperator": "AND",
},
}
}
eoj_config = {
"JobConfig": {
"CloudRemovalConfig": {
"AlgorithmName": "INTERPOLATION",
"InterpolationValue": "-9999",
"TargetBands": ["red", "green", "blue", "nir", "swir16"],
},
}
}
eojParams = {
"Name": "cloudremoval",
"InputConfig": eoj_input_config,
**eoj_config,
"ExecutionRoleArn": role_arn,
}
eoj_response = sg_client.start_earth_observation_job(**eojParams)
After the EOJ is created, the ARN is returned and used to perform the subsequent geomosaic operation.
To get the status of a job, you can run sg_client.get_earth_observation_job(Arn = response['Arn']).
Geomosaic
The geomosaic EOJ is used to merge images from multiple satellite visits into a large mosaic, by overwriting nodata or transparent pixels (including the cloudy pixels) with pixels from other timestamps:
eoj_config = {"JobConfig": {"GeoMosaicConfig": {"AlgorithmName": "NEAR"}}}
eojParams = {
"Name": "geomosaic",
"InputConfig": {"PreviousEarthObservationJobArn": eoj_arn},
**eoj_config,
"ExecutionRoleArn": role_arn,
}
eoj_response = sg_client.start_earth_observation_job(**eojParams)
After the EOJ is created, the ARN is returned and used to perform the subsequent resampling operation.
Resampling
Resampling is used to downscale the resolution of the geospatial image in order to match the resolution of the crop masks (10–30 m resolution rescaling):
eoj_config = {
"JobConfig": {
"ResamplingConfig": {
"OutputResolution": {"UserDefined": {"Value": 30, "Unit": "METERS"}},
"AlgorithmName": "NEAR",
},
}
}
eojParams = {
"Name": "resample",
"InputConfig": {"PreviousEarthObservationJobArn": eoj_arn},
**eoj_config,
"ExecutionRoleArn": role_arn,
}
eoj_response = sg_client.start_earth_observation_job(**eojParams)
After the EOJ is created, the ARN is returned and used to perform the subsequent band math operation.
Band math
Band math operations are used for transforming the observations from multiple spectral bands to a single band. It includes the following spectral indices:
- EVI2 – Two-Band Enhanced Vegetation Index
- GDVI – Generalized Difference Vegetation Index
- NDMI – Normalized Difference Moisture Index
- NDVI – Normalized Difference Vegetation Index
- NDWI – Normalized Difference Water Index
See the following code:
spectral_indices = [['EVI2', ' 2.5 * ( nir - red ) / ( nir + 2.4 * red + 1.0 ) '],
['GDVI', ' ( ( nir * * 2.0 ) - ( red * * 2.0 ) ) / ( ( nir * * 2.0 ) + ( red * * 2.0 ) ) '],
['NDMI', ' ( nir - swir16 ) / ( nir + swir16 ) '],
['NDVI', ' ( nir - red ) / ( nir + red ) '],
['NDWI', ' ( green - nir ) / ( green + nir ) ']]
eoj_config = {
"JobConfig": {
"BandMathConfig": {"CustomIndices": {"Operations": []}},
}
}
for indices in spectral_indices:
eoj_config["JobConfig"]["BandMathConfig"]["CustomIndices"]["Operations"].append(
{"Name": indices[0], "Equation": indices[1][1:-1]}
)
eojParams = {
"Name": "bandmath",
"InputConfig": {"PreviousEarthObservationJobArn": eoj_arn},
**eoj_config,
"ExecutionRoleArn": role_arn,
}
eoj_response = sg_client.start_earth_observation_job(**eojParams)
Zonal statistics
The spectral indices are further enriched using Amazon SageMaker Processing, where GDAL-based custom logic is used to do the following:
- Merge the spectral indices into a single multi-channel mosaic
- Reproject the mosaic to the crop mask‘s projection
- Apply the crop mask and reproject the mosaic to the cells polygons’s CRC
- Calculate zonal statistics for selected polygons (10 km x 10 km cells)
With parallelized data distribution, manifest files (for each crop phenological stage) are distributed across several instances using the ShardedByS3Key
S3 data distribution type. For further details, refer to the feature extraction script.
The geospatial processing notebook is a step-by-step guide on running the preceding steps.
The following figure shows RGB channels of consecutive satellite visits representing the vegetative and reproductive stages of the corn phenology cycle, with (right) and without (left) crop masks (CW 20, 26 and 33, 2018 Central Illinois).
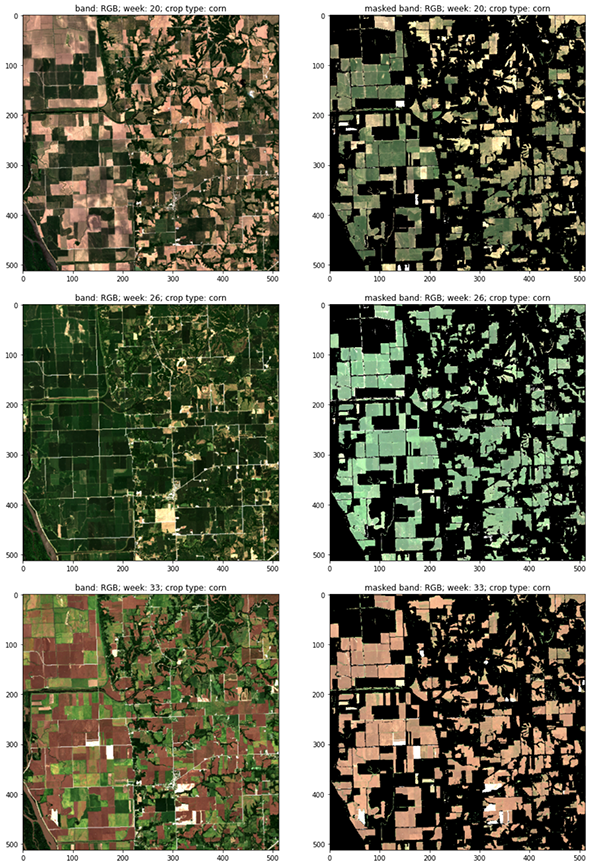
In the following figure, spectral indices (NDVI, EVI2, NDMI) of consecutive satellite visits represent the vegetative and reproductive stages of the corn phenology cycle (CW 20, 26 and 33, 2018 Central Illinois).
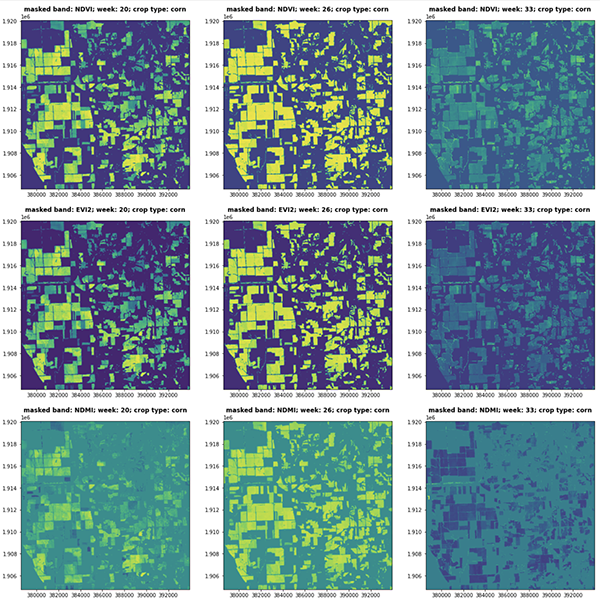
Clean up
If you no longer want to use this solution, you can delete the resources it created. After the solution is deployed in Studio, choose Delete all resources to automatically delete all standard resources that were created when launching the solution, including the S3 bucket.
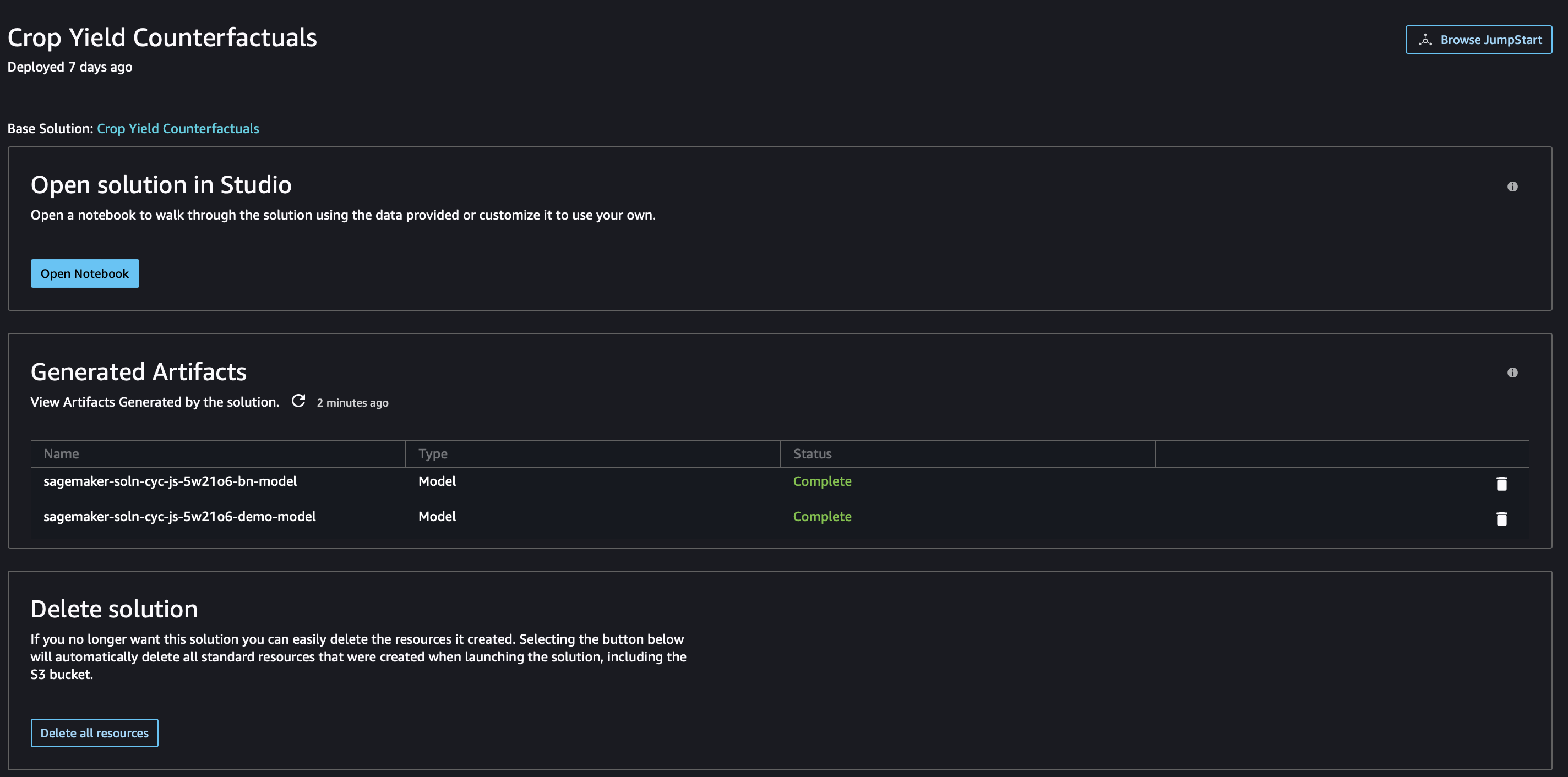
Conclusion
This solution provides a blueprint for use cases where causal inference with Bayesian networks are the preferred methodology for answering causal questions from a combination of data and human inputs. The workflow includes an efficient implementation of the inference engine, which queues incoming queries and interventions and processes them asynchronously. The modular aspect enables the reuse of various components, including geospatial processing with purpose-built operations and pre-trained models, enrichment of satellite imagery with custom-built GDAL operations, and multimodal feature engineering (spectral indices and tabular data).
In addition, you can use this solution as a template for building gridded crop models where nitrogen fertilizer management and environmental policy analysis are conducted.
For more information, refer to Solution Templates and follow the guide to launch the Crop Yield Counterfactuals solution in the US West (Oregon) Region. The code is available in the GitHub repo.
Citations
German Mandrini, Sotirios V. Archontoulis, Cameron M. Pittelkow, Taro Mieno, Nicolas F. Martin,
Simulated dataset of corn response to nitrogen over thousands of fields and multiple years in Illinois,
Data in Brief, Volume 40, 2022, 107753, ISSN 2352-3409
Useful resources
About the Authors
Paul Barna is a Senior Data Scientist with the Machine Learning Prototyping Labs at AWS.
Read More