Students will receive funding to pursue independent research projects in robotics and adjacent areas in AI.Read More
Improve data extraction and document processing with Amazon Textract
Intelligent document processing (IDP) has seen widespread adoption across enterprise and government organizations. Gartner estimates the IDP market will grow more than 100% year over year, and is projected to reach $4.8 billion in 2022.
IDP helps transform structured, semi-structured, and unstructured data from a variety of document formats into actionable information. Processing unstructured data has become much easier with the advancements in optical character recognition (OCR), machine learning (ML), and natural language processing (NLP).
IDP techniques have grown tremendously, allowing us to extract, classify, identify, and process unstructured data. With AI/ML powered services such as Amazon Textract, Amazon Transcribe, and Amazon Comprehend, building an IDP solution has become much easier and doesn’t require specialized AI/ML skills.
In this post, we demonstrate how to use Amazon Textract to extract meaningful, actionable data from a wide range of complex multi-format PDF files. PDF files are challenging; they can have a variety of data elements like headers, footers, tables with data in multiple columns, images, graphs, and sentences and paragraphs in different formats. We explore the data extraction phase of IDP, and how it connects to the steps involved in a document process, such as ingestion, extraction, and postprocessing.
Solution overview
Amazon Textract provides various options for data extraction, based on your use case. You can use forms, tables, query-based extractions, handwriting recognition, invoices and receipts, identity documents, and more. All the extracted data is returned with bounding box coordinates. This solution uses Amazon Textract IDP CDK constructs to build the document processing workflow that handles Amazon Textract asynchronous invocation, raw response extraction, and persistence in Amazon Simple Storage Service (Amazon S3). This solution adds an Amazon Textract postprocessing component to the base workflow to handle paragraph-based text extraction.
The following diagram shows the document processing flow.
The document processing flow contains the following steps:
- The document extraction flow is initiated when a user uploads a PDF document to Amazon S3.
- An S3 object notification event triggered by new the S3 object with an
uploads/
prefix, which triggers the AWS Step Functions asynchronous workflow. - The AWS Lambda function
SimpleAsyncWorkflow
Decider validates the PDF document. This step prevents processing invalid documents. - TextractAsync is an IDP CDK construct that abstracts the invocation of the Amazon Textract
Async
API, handling Amazon Simple Notification Service (Amazon SNS) messages and workflow processing. The following are some high-level steps:- The construct invokes the asynchronous Amazon Textract StartDocumentTextDetection API.
- Amazon Textract processes the PDF file and publishes a completion status event to an Amazon SNS topic.
- Amazon Textract stores the paginated results in Amazon S3.
- Construct handles the Amazon Textract completion event, returns the paginated results output prefix to the main workflow.
- The Textract Postprocessor Lambda function uses the extracted content in the results Amazon S3 bucket to retrieve the document data. This function iterates through all the files, and extracts data using bounding boxes and other metadata. It performs various postprocessing optimizations to aggregate paragraph data, identify and ignore headers and footers, combine sentences spread across pages, process data in multiple columns, and more.
- The Textract Postprocessor Lambda function persists the aggregated paragraph data as a CSV file in Amazon S3.
Deploy the solution with the AWS CDK
To deploy the solution, launch the AWS Cloud Development Kit (AWS CDK) using AWS Cloud9 or from your local system. If you’re launching from your local system, you need to have the AWS CDK and Docker installed. Follow the instructions in the GitHub repo for deployment.
The stack creates the key components depicted in the architecture diagram.
Test the solution
The GitHub repo contains the following sample files:
- sample_climate_change.pdf – Contains headers, footers, and sentences flowing across pages
- sample_multicolumn.pdf – Contains data in two columns, headers, footers, and sentences flowing across pages
To test the solution, complete the following steps:
- Upload the sample PDF files to the S3 bucket created by the stack: The file upload triggers the Step Functions workflow via S3 event notification.
- Open the Step Functions console to view the workflow status. You should find one workflow instance per document.
- Wait for all three steps to complete.
- On the Amazon S3 console, browse to the S3 prefix mentioned in the JSON path
TextractTempOutputJsonPath
. The below screenshot of the Amazon S3 console shows the Amazon Textract paginated results (in this case objects 1 and 2) created by Amazon Textract. The postprocessing task stores the extracted paragraphs from the sample PDF asextracted-text.csv.
- Download the
extracted-text.csv
file to view the extracted content.
The sample_climate_change.pdf
file has sentences flowing across pages, as shown in the following screenshot.
The postprocessor identifies and ignores the header and footer, and combines the text across pages into one paragraph. The extracted text for the combined paragraph should look like:
“Impacts on this scale could spill over national borders, exacerbating the damage further. Rising sea levels and other climate-driven changes could drive millions of people to migrate: more than a fifth of Bangladesh could be under water with a 1m rise in sea levels, which is a possibility by the end of the century. Climate-related shocks have sparked violent conflict in the past, and conflict is a serious risk in areas such as West Africa, the Nile Basin and Central Asia.”
The sample_multi_column.pdf
file has two columns of text with headers and footers, as shown in the following screenshot.
The postprocessor identifies and ignores the header and footer, processes the text in the columns from left to right, and combines incomplete sentences across pages. The extracted text should construct paragraphs from text in the left column and separate paragraphs from text in the right column. The last line in the right column is incomplete on that page and continues in the left column of the next page; the postprocessor should combine them as one paragraph.
Cost
With Amazon Textract, you pay as you go based on the number of pages in the document. Refer to Amazon Textract pricing for actual costs.
Clean up
When you’re finished experimenting with this solution, clean up your resources by using the AWS CloudFormation console to delete all the resources deployed in this example. This helps you avoid continuing costs in your account.
Conclusion
You can use the solution presented in this post to build an efficient document extraction workflow and process the extracted document according to your needs. If you’re building an intelligent document processing system, you can further process the extracted document using Amazon Comprehend to get more insights about the document.
For more information about Amazon Textract, visit Amazon Textract resources to find video resources and blog posts, and refer to Amazon Textract FAQs. For more information about the IDP reference architecture, refer to Intelligent Document Processing. Please share your thoughts with us in the comments section, or in the issues section of the project’s GitHub repository.
About the Author
Sathya Balakrishnan is a Sr. Customer Delivery Architect in the Professional Services team at AWS, specializing in data and ML solutions. He works with US federal financial clients. He is passionate about building pragmatic solutions to solve customers’ business problems. In his spare time, he enjoys watching movies and hiking with his family.
Method enables better control of GAN image generators’ output
By plotting nonlinear trajectories through a GAN’s latent space, the method enables certain image attributes to vary while others are held fixed.Read More
Automated exploratory data analysis and model operationalization framework with a human in the loop
Identifying, collecting, and transforming data is the foundation for machine learning (ML). According to a Forbes survey, there is widespread consensus among ML practitioners that data preparation accounts for approximately 80% of the time spent in developing a viable ML model.
In addition, many of our customers face several challenges during the model operationalization phase to accelerate the journey from model conceptualization to productionization. Quite often, models are built and deployed using poor-quality, under-representative data samples, which leads to more iterations and more manual effort in data inspection, which in turn makes the process more time consuming and cumbersome.
Because your models are only as good as your training data, expert data scientists and practitioners spend an enormous time understanding the data and generating valuable insights prior to building the models. If we view our ML models as an analogy to cooking a meal, the importance of high-quality data for an advanced ML system is similar to the relationship between high-quality ingredients and a successful meal. Therefore, before rushing into building the models, make sure you’re spending enough time getting high-quality data and extracting relevant insights.
The tools and technologies to assist with data preprocessing have been growing over the years. Now we have low-code and no-code tools like Amazon SageMaker Data Wrangler, AWS Glue DataBrew, and Amazon SageMaker Canvas to assist with data feature engineering.
However, a lot of these processes are still currently done manually by a data engineer or analyst who analyzes the data using these tools. If their the knowledge of the tools is limited, the insights generated prior to building the models won’t do justice to all the steps that can be performed. Additionally, we won’t be able to make an informed decision post-analysis of those insights prior to building the ML models. For instance, the models can turn out to be biased due to lack of detailed insights that you received using AWS Glue or Canvas, and you end up spending a lot of time and resources building the model training pipeline, to eventually receive an unsatisfactory prediction.
In this post, we introduce a novel intelligent framework for data and model operationalization that provides automated data transformations and optimal model deployment. This solution can accelerate accurate and timely inspection of data and model quality checks, and facilitate the productivity of distinguished data and ML teams across your organization.
Overview of solution
Our solution demonstrates an automated end-to-end approach to perform exploratory data analysis (EDA) with a human in the loop to determine the model quality thresholds and approve the optimal and qualified data to be pushed into Amazon SageMaker Pipelines in order to push the final data into Amazon SageMaker Feature Store, thereby speeding up the executional framework.
Furthermore, the approach includes deploying the best candidate model and creating the model endpoint on the transformed dataset that was automatically processed as new data arrives in the framework.
The following diagram illustrates the initial setup for the data preprocessing step prior to automating the workflow.
This step comprises the data flow initiation to process the raw data stored in an Amazon Simple Storage Service (Amazon S3) bucket. A sequence of steps in the Data Wrangler UI are created to perform feature engineering on the data (also referred to as a recipe). The data flow recipe consists of preprocessing steps along with a bias report, multicollinearity report, and model quality analysis.
Then, an Amazon SageMaker Processing job is run to save the flow to Amazon S3 and store the transformed features into Feature Store for reusable purposes.
After the flow has been created, which includes the recipe of instructions to be run on the data pertaining to the use case, the goal is to automate the process of creating the flow on any new incoming data, and initiate the process of extracting model quality insights using Data Wrangler. Then, the information regarding the transformations performed on the new data is parsed to an authorized user to inspect the data quality, and the pipeline waits for approval to run the model building and deployment step automatically.
The following architecture showcases the end-to-end automation of data transformation followed by human in the loop approval to facilitate the steps of model training and deployment.
The steps consist of an end-to-end orchestration for automated data transformation and optimal model deployment (with a human in the loop) using the following sequence of steps:
- A new object is uploaded into the S3 bucket (in our case, our training data).
- An AWS Lambda function is triggered when the object is uploaded in Amazon S3, which invokes AWS Step Functions and notifies the authorized user via a registered email.The following steps occur within the Step Functions orchestration:
- The Data Wrangler Flow Creation Lambda function fetches the Data Wrangler flow and processes the new data to be ingested into the Data Wrangler flow. It creates a new flow, which, when imported into the Data Wrangler UI, includes all the transformations, along with a model quality report and bias report. The function saves this latest flow in a new destination bucket.
- The User Callback Approval Lambda function sends a trigger notification via Amazon Simple Notification Service (Amazon SNS) to the registered persona via email to review the analyzed flow created on new unseen data information. In the email, the user has the option to accept or reject the data quality outcome and feature engineering flow.
- The next step is based on the approver’s decision:
- If the human in the loop approved the changes, the Lambda function initiates the SageMaker pipeline in the next state.
- If the human in the loop rejected the changes, the Lambda function doesn’t initiate the pipeline, and allows the user to look into the steps within the flow to perform additional feature engineering.
- The SageMaker Pipeline Execution Lambda function runs the SageMaker pipeline to create a SageMaker Processing job, which stores the feature engineered data in Feature Store. Another pipeline is created in parallel to save the transformed data to Amazon S3 as a CSV file.
- The AutoML Model Job Creation and Deployment Lambda function initiates an Amazon SageMaker Autopilot job to build and deploy the best candidate model and create a model endpoint, which authorized users can invoke for inference.
A Data Wrangler flow is available in our code repository that includes a sequence of steps to run on the dataset. We use Data Wrangler within our Amazon SageMaker Studio IDE, which can simplify the process of data preparation and feature engineering, and complete each step of the data preparation workflow, including data selection, cleansing, exploration, and visualization from a single visual interface.
Dataset
To demonstrate the orchestrated workflow, we use an example dataset regarding diabetic patient readmission. This data contains historical representations of patient and hospital outcomes, wherein the goal involves building an ML model to predict hospital readmission. The model has to predict whether the high-risk diabetic patients are likely to be readmitted to the hospital after a previous encounter within 30 days or after 30 days. Because this use case deals with multiple outcomes, this is a multi-class classification ML problem. You can try out the approach with this example and experiment with additional data transformations following similar steps with your own datasets.
The sample dataset we use in this post is a sampled version of the Diabetes 130-US hospitals for years 1999-2008 Data Set (Beata Strack, Jonathan P. DeShazo, Chris Gennings, Juan L. Olmo, Sebastian Ventura, Krzysztof J. Cios, and John N. Clore, “Impact of HbA1c Measurement on Hospital Readmission Rates: Analysis of 70,000 Clinical Database Patient Records,” BioMed Research International, vol. 2014, Article ID 781670, 11 pages, 2014.). It contains historical data including over 15 features with patient and hospital outcomes. The dataset contains approximately 69,500 rows. The following table summarizes the data schema.
Column Name | Data Type | Data Description |
race |
STRING | Caucasian, Asian, African American, or Hispanic. |
time_in_hospital |
INT | Number of days between admission and discharge (length of stay). |
number_outpatient |
INT | Number of outpatient visits of the patient in a given year before the encounter. |
number_inpatient |
INT | Number of inpatient visits of the patient in a given year before the encounter. |
number_emergency |
INT | Number of emergency visits of the patient in a given year before the encounter. |
number_diagnoses |
INT | Number of diagnoses entered in the system. |
num_procedures |
INT | Number of procedures (other than lab tests) performed during the encounter. |
num_medications |
INT | Number of distinct generic medicines administrated during the encounter. |
num_lab_procedures |
INT | Number of lab tests performed during the encounter. |
max_glu_serum |
STRING | The range of result or if the test wasn’t taken. Values include >200, >300, normal, and none (if not measured). |
gender |
STRING | Values include Male, Female and Unknown/Invalid. |
diabetes_med |
INT | Indicates if any diabetes medication was prescribed. |
change |
STRING | Indicates if there was a change in diabetes medications (ether dosage or generic name). Values are change or no change. |
age |
INT | Age of patient at the time of encounter. |
a1c_result |
STRING | Indicates the range of the result of blood sugar levels. Values include >8, >7, normal, and none. |
readmitted |
STRING | Days to inpatient readmission. Values include <30 if patient was readmitted in less than 30 days, >30 if patient was readmitted after 30 days of encounter, and no for no record of readmission. |
Prerequisites
This walkthrough includes the following prerequisites:
- An AWS account
- A Studio domain managed policy attached to the AWS Identity and Access Management (IAM) execution role. For instructions on assigning permissions to the role, refer to Amazon SageMaker API Permissions: Actions, Permissions, and Resources Reference. In this case, you need to assign permissions as allocated to Amazon Augmented AI (Amazon A2I). For more information, refer to Amazon SageMaker Identity-Based Policy Examples.
- An S3 bucket. For instructions, refer to Creating a bucket.
- For this post, you use the AWS Cloud Development Kit (AWS CDK) using Python. Follow the instructions in Getting Started for AWS CDK to set up your local environment setup and bootstrap your development account.
Upload the historical dataset to Amazon S3
The first step is to download the sample dataset and upload it into an S3 bucket. In our case, our training data (diabetic-readmission.csv) is uploaded.
Data Wrangler initial flow
Prior to automating the Step Functions workflow, we need to perform a sequence of data transformations to create a data flow.
If you want to create the Data Wrangler steps manually, refer to the readme in the GitHub repo.
To import the flow to automate the Data Wrangler steps, complete the following steps:
- Download the flow from the GitHub repo and save it in your system.
- Open Studio and import the Data Wrangler flow.You need to update the location of where it needs to import the latest dataset. In your case, this is the bucket you defined with the respective prefix.
- Choose the plus sign next to Source and choose Edit dataset.
- Point to the S3 location of the dataset you downloaded.
- Inspect all the steps in the transformation and make sure they align with the sequence steps.
Save data flow to Feature Store
To save the data flow to Feature Store, complete the following steps:
- Choose the plus sign next to Steps and choose Export to.
- Choose SageMaker Feature Store (via Jupyter Notebook).
SageMaker generates a Jupyter notebook for you and opens it in a new tab in Studio. This notebook contains everything you need to run the transformations over our historical dataset and ingest the resulting features into Feature Store.This notebook uses Feature Store to create a feature group, runs your Data Wrangler flow on the entire dataset using a SageMaker processing job, and ingests the processed data to Feature Store. - Choose the kernel Python 3 (Data Science) on the newly opened notebook tab.
- Read through and explore the Jupyter notebook.
- In the Create Feature Group section of the generated notebook, update the following fields for the event time and record identifier with the column names we created in the previous Data Wrangler step:
- Choose Run and then choose Run All Cells.
- Enter
flow_name = "HealthCareUncleanWrangler"
. - Run the following cells to create your feature group name.
After running a few more cells in the code, the feature group is successfully created.
- Now that the feature group is created, you use a processing job to process your data at scale and ingest the transformed data into this feature group.
If we keep the default bucket location, the flow will be saved in a SageMaker bucket located in the specific Region where you launched your SageMaker domain.With
Feature_store_offline_S3_uri
, Feature Store writes the data in theOfflineStore
of aFeatureGroup
to an Amazon S3 location owned by you.Wait for the processing job to finish. If it finishes successfully, your feature group should be populated with the transformed feature values. In addition, the raw parameters used by the processing job are printed.It takes 10–15 minutes to run the processing job to create and run the Data Wrangler flow on the entire dataset and save the output flow in the respective bucket within the SageMaker session. - Next, run the
FeatureStoreAutomation.ipynb
notebook by importing it in Studio from GitHub and running all the cells. Follow the instructions in the notebook. - Copy the following variables from the Data Wrangler generated output from the previous step and add them to the cell in the notebook:
- Run the rest of the code following the instructions in the notebook to create a SageMaker pipeline to automate the storing of features to Feature Store in the feature group that you created.
- Next, similar to the previous step in the Data Wrangler export option, choose the plus sign and choose Export to.
- Choose SageMaker Pipelines (via Jupyter Notebook).
- Run all the cells to create a CSV flow as an output to be stored to Amazon S3.That pipeline name is invoked in a Lambda function later to automate the pipeline on a new flow.
- Within the code, whenever you see the following instance count, change
instance_count
to 1: - Otherwise, your account may hit the service quota limits of running an m5.4x large instance for processing jobs being run within the notebook. You have to request an increase in service quota if you want more instances to run the job.
- As you walk through the pipeline code, navigate to Create SageMaker Pipeline, where you define the pipeline steps.
- In the Output Amazon S3 settings cell, change the location of the Amazon S3 output path to the following code (commenting the output prefix):
- Locate the following code:
- Replace it with the following:
- Remove the following cell:
- Continue running the next steps until you reach the Define a Pipeline of Parameters section with the following code. Append the last line
input_flow
to the code segment: - Also, add the
input_flow
as an additional parameter to the next cell: - In the section Submit the pipeline to SageMaker and start execution, locate the following cell:
- Replace it with the following code:
- Copy the name of the pipeline you just saved.
This will be yourS3_Pipeline_Name
value that is added as the environment variable stored inDataWrangler Flow Creation
Lambda Function. - Replace
S3_Pipeline_Name
with the name of the pipeline that you just created after running the preceding notebook.
Now, when a new object is uploaded in Amazon S3, a SageMaker pipeline runs the processing job of creating the Data Wrangler flow on the entire dataset and stores the transformed dataset in Amazon S3 as a CSV file. This object is used in the next step (the Step Functions workflow) for model training and endpoint deployment.We have created and stored a transformed dataset in Amazon S3 by running the preceding notebook. We also created a feature group in Feature Store for storing the respective transformed features for later reuse. - Update both pipeline names in the Data Wrangler Flow Creation Lambda function (created with the AWS CDK) for the Amazon S3 pipeline and Feature Store pipeline.
Step Functions orchestration workflow
Now that we have created the processing job, we need to run these processing jobs on any incoming data that arrives in Amazon S3. We initiate the data transformation automatically, notify the authorized user of the new flow created, and wait for the approver to approve the changes based on data and model quality insights. Then, the Step Functions callback action is triggered to initiate the SageMaker pipeline and start the model training and optimal model deployment endpoint in the environment.
The Step Functions workflow includes a series of Lambda functions to run the overall orchestration. The Step Functions state machine, S3 bucket, Amazon API Gateway resources, and Lambda function codes are stored in the GitHub repo.
The following figure illustrates our Step Function workflow.
Run the AWS CDK code located in GitHub to automatically set up the stack containing the components needed to run the automated EDA and model operationalization framework. After setting up the AWS CDK environment, run the following command in the terminal:
Create a healthcare folder in the bucket you named via your AWS CDK script. Then upload flow-healthcarediabetesunclean.csv
to the folder and let the automation happen!
In the following sections, we walk through each step in the Step Functions workflow in more detail.
Data Wrangler Flow Creation
As new data is uploaded into the S3 bucket, a Lambda function is invoked to trigger the Step Functions workflow. The Data Wrangler Flow Creation Lambda function fetches the Data Wrangler flow. It runs the processing job to create a new Data Wrangler flow (which includes data transformations, model quality report, bias report, and so on) on the ingested dataset and pushes the new flow to the designated S3 bucket.
This Lambda function parses the information to the User Callback Approval Lambda function and sends the trigger notification via Amazon SNS to the registered email with the location of the designated bucket where the flow has been saved.
User Callback Approval
The User Callback Approval step initiates the Lambda function that receives the updated flow information and sends a notification to the authorized user with the approval/rejection link to approve or reject the new flow. The user can review the analyzed flow created on the unseen data by downloading the flow from the S3 bucket and uploading it in the Data Wrangler UI.
After the user reviews the flow, they can go back to the email to approve the changes.
Manual Approval Choice
This Lambda function is waiting for the authorized user to approve or reject the flow.
If the answer received is yes (the user approved the flow), the SageMaker Pipeline Execution Lambda function initiates the SageMaker pipeline for storing the transformed features in Feature Store. Another SageMaker pipeline is initiated in parallel to save the transformed features CSV to Amazon S3, which is used by the next state (the AutoML Model Job Creation & Model Deployment Lambda function) for model training and deployment.
If the answer received is no (the user rejected the flow), the Lambda function doesn’t initiate the pipeline to run the flow. The user can look into the steps within the flow to perform additional feature engineering. Later, the user can rerun the entire sequence after adding additional data transformation steps in the flow.
SageMaker Pipeline Execution
This step initiates a Lambda function that runs the SageMaker pipeline to store the feature engineered data in Feature Store. Another pipeline in parallel saves the transformed data to Amazon S3.
You can monitor the two pipelines in Studio by navigating to the Pipelines page.
You can choose the graph to inspect the input, output, logs, and information.
Similarly, you can inspect the information of the other pipeline, which saves the transformed features CSV to Amazon S3.
AutoML Model Job Creation & Model Deployment
This step initiates a Lambda function that starts an Autopilot job to ingest the CSV from the previous Lambda function, and build and deploy the best candidate model. This step creates a model endpoint that can be invoked by authorized users. When the AutoML job is complete, you can navigate to Studio, choose Experiment and trials, and view the information associated with your job.
As all of these steps are run, the SageMaker dashboard reflects the processing job, batch transform job, training job, and hyperparameter tuning job that are being created in the process and the creation of the endpoint that can be invoked when the overall process is complete.
Clean up
To avoid ongoing charges, make sure to delete the SageMaker endpoint and stop all the notebooks running in Studio, including the Data Wrangler instances. Also, delete the output data in Amazon S3 you created while running the orchestration workflow via Step Functions. You have to delete the data in the S3 buckets before you can delete the buckets.
Conclusion
In this post, we demonstrated an end-to-end approach to perform automated data transformation with a human in the loop to determine model quality thresholds and approve the optimal qualified data to be pushed to a SageMaker pipeline to push the final data into Feature Store, thereby speeding up the executional framework. Furthermore, the approach includes deploying the best candidate model and creating the model endpoint on the final feature engineered data that was automatically processed when new data arrives.
References
For further information about Data Wrangler, Feature Store, SageMaker pipelines, Autopilot, and Step Functions, we recommend the following resources:
- Amazon SageMaker Pipelines Brings DevOps Capabilities to your Machine Learning Projects
- Step Functions for feature transformation
- Introducing SageMaker Data Wrangler, a Visual Interface to Prepare Data for Machine Learning
- Building machine learning workflows with Amazon SageMaker Processing jobs and AWS Step Functions
- Unified data preparation and model training with Amazon SageMaker Data Wrangler and Amazon SageMaker Autopilot
About the Author(s)
Shikhar Kwatra is an AI/ML Specialist Solutions Architect at Amazon Web Services, working with a leading Global System Integrator. He has earned the title of one of the Youngest Indian Master Inventors with over 400 patents in the AI/ML and IoT domains. He has over 8 years of industry experience from startups to large-scale enterprises, from IoT Research Engineer, Data Scientist, to Data & AI Architect. Shikhar aids in architecting, building, and maintaining cost-efficient, scalable cloud environments for organizations and supports GSI partners in building strategic industry solutions on AWS.
Sachin Thakkar is a Senior Solutions Architect at Amazon Web Services, working with a leading Global System Integrator (GSI). He brings over 22 years of experience as an IT Architect and as Technology Consultant for large institutions. His focus area is on data and analytics. Sachin provides architectural guidance and supports GSI partners in building strategic industry solutions on AWS.
Move Amazon SageMaker Autopilot ML models from experimentation to production using Amazon SageMaker Pipelines
Amazon SageMaker Autopilot automatically builds, trains, and tunes the best custom machine learning (ML) models based on your data. It’s an automated machine learning (AutoML) solution that eliminates the heavy lifting of handwritten ML models that requires ML expertise. Data scientists need to only provide a tabular dataset and select the target column to predict, and Autopilot automatically infers the problem type, performs data preprocessing and feature engineering, selects the algorithms and training mode, and explores different configurations to find the best ML model. Then you can directly deploy the model to an Amazon SageMaker endpoint or iterate on the recommended solutions to further improve the model quality.
Although Autopilot eliminates the heavy lifting of building ML models, MLOps engineers still have to create, automate, and manage end-to-end ML workflows. Amazon SageMaker Pipelines helps you automate the different steps of the ML lifecycle, including data preprocessing, training, tuning and evaluating ML models, and deploying them.
In this post, we show how to create an end-to-end ML workflow to train and evaluate an Autopilot generated ML model using Pipelines and register it in the SageMaker model registry. The ML model with the best performance can be deployed to a SageMaker endpoint.
Dataset overview
We use the publicly available hospital readmission dataset for diabetic patients to predict readmission of diabetic patients within 30 days after discharge. It is a sampled version of the “Diabetes 130-US hospitals for years 1999-2008 Data Set”. This is a multi-class classification problem because the readmission options are either < 30
if the patient is readmitted within 30 days, > 30
if the patient is readmitted after 30 days, or no for no record of readmission.
The dataset contains 50,000 rows and 15 columns. This includes demographic information about patients along with their hospital visit records and readmitted
as the target column. The following table summarizes the column details.
Column Name | Description |
Race_Caucasian | Values: 0 for no, 1 for yes |
Race_African_American | Values: 0 for no, 1 for yes |
Race_Hispanic | Values: 0 for no, 1 for yes |
Race_Asian | Values: 0 for no, 1 for yes |
Race_Other | Values: 0 for no, 1 for yes |
Age | 0–100 age range |
Time in Hospital | Number of days between admission and discharge |
Number of lab procedures | Number of lab tests performed during the encounter |
Number of medications | Number of distinct generic names administered during the encounter |
Number of emergency visits | Number of emergency visits of the patient in the year preceding the encounter |
Number of inpatient visits | Number of inpatient visits of the patient in the year preceding the encounter |
Number of diagnoses | Number of diagnoses entered to the system |
Change of medications | Indicates if there was a change in diabetic medications (either dosage or generic name); values: 0 and 1 |
Diabetic medications | Indicates if there was any diabetic medication prescribed; values: 0 for no changes in prescription and 1 for change in prescription |
Readmitted | Days to inpatient readmission; values: <30 if the patient was readmitted in less than 30 days, >30 if the patient was readmitted in more than 30 days, and no for no record of readmission |
Solution overview
We use Pipelines in Amazon SageMaker Studio to orchestrate different pipeline steps required to train an Autopilot model. An Autopilot experiment is created and run using the AWS SDKs as described in this post. Autopilot training jobs start their own dedicated SageMaker backend processes, and dedicated SageMaker API calls are required to start new training jobs, monitor training job statuses, and invoke trained Autopilot models.
The following are the steps required for this end-to-end Autopilot training process:
- Create an Autopilot training job.
- Monitor the training job status.
- Evaluate performance of the trained model on a test dataset.
- Register the model in the model registry.
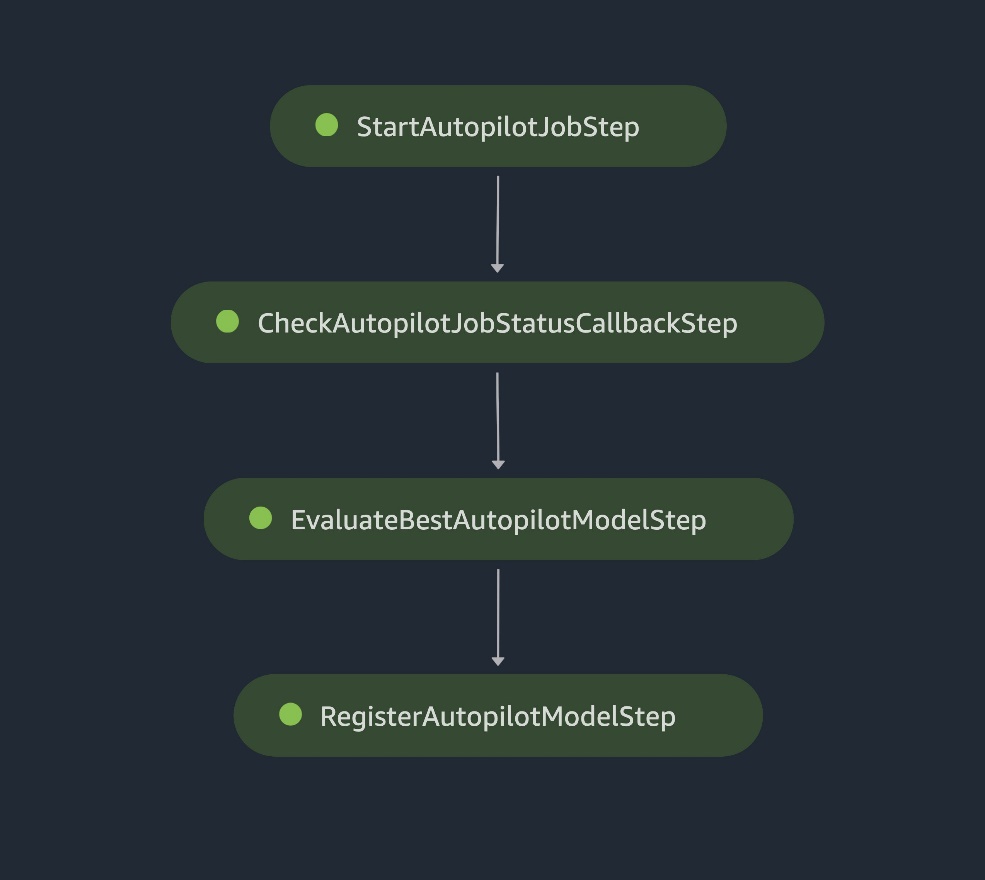
SageMaker pipeline steps
When the registered model meets the expected performance requirements after a manual review, you can deploy the model to a SageMaker endpoint using a standalone deployment script.
The following architecture diagram illustrates the different pipeline steps necessary to package all the steps in a reproducible, automated, and scalable Autopilot training pipeline. Each step is responsible for a specific task in the workflow:
- An AWS Lambda function starts the Autopilot training job.
- A Callback step continuously monitors that job status.
- When the training job status is complete, we use a SageMaker processing job to evaluate the model’s performance.
- Finally, we use another Lambda function to register the ML model and the performance metrics to the SageMaker model registry.
The data files are read from the Amazon Simple Storage Service (Amazon S3) bucket and the pipeline steps are called sequentially.
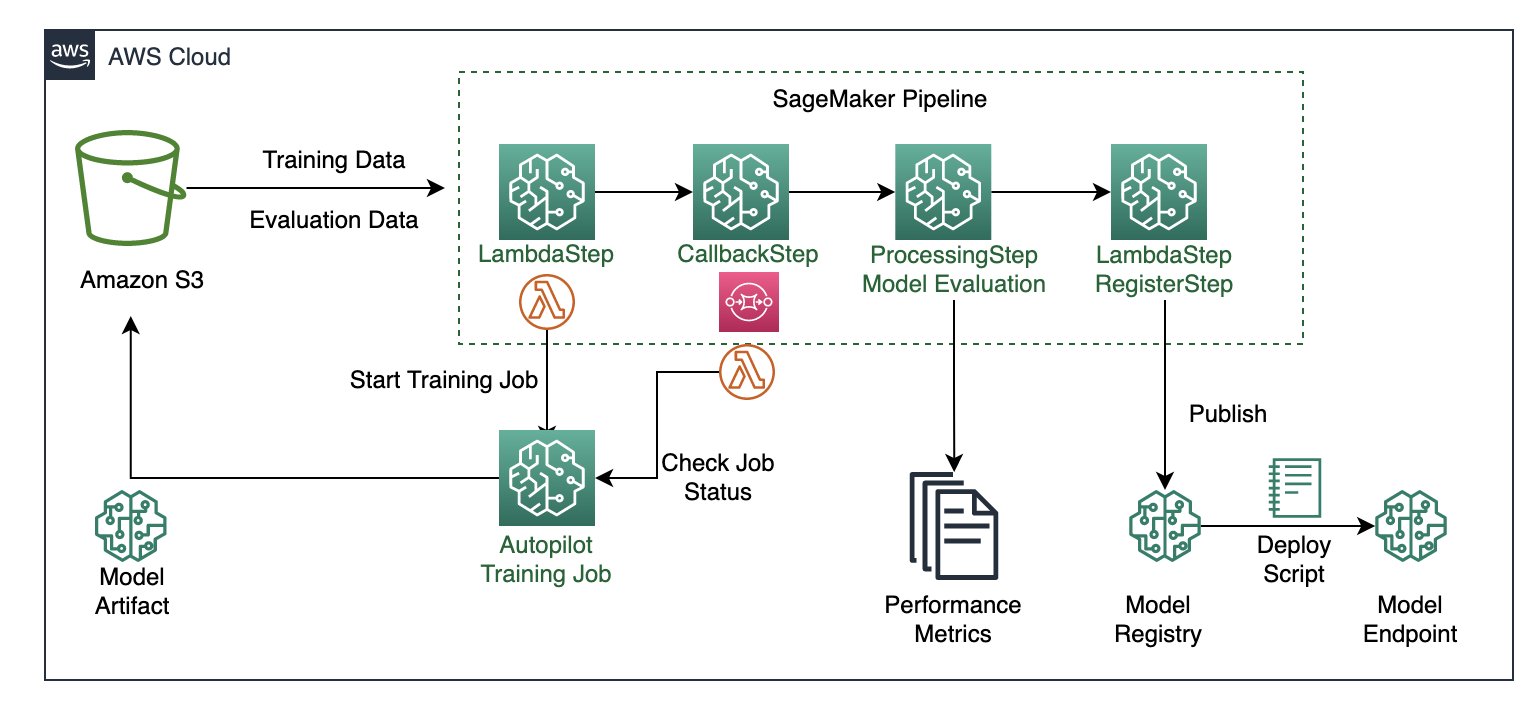
Architecture diagram of the SageMaker pipeline
In the following sections, we review the code and discuss the components of each step. To deploy the solution, reference the GitHub repo, which provides step-by-step instructions for implementing an Autopilot MLOps workflow using Pipelines.
Prerequisites
For this walkthrough, complete the following prerequisite steps:
- Set up an AWS account.
- Create a Studio environment.
- Create two AWS Identity and Access Management (IAM) roles:
LambdaExecutionRole
andSageMakerExecutionRole
, with permissions as outlined in the SageMaker notebook. The managed policies should be scoped down further for improved security. For instructions, refer to Creating a role to delegate permissions to an IAM user. - On the Studio console, upload the code from the GitHub repo.
- Open the SageMaker notebook
autopilot_pipelines_demo_notebook.ipynb
and run the cells under Get dataset to download the data and upload it to your S3 bucket.- Download the data and unzip it to a folder named data:
- Split the data into train-val and test files and upload them to your S3 bucket. The train-val file is automatically split into training and validation datasets by Autopilot. The test file is split into two separate files: one file without the target column and another file with only the target column.
When the dataset is ready to use, we can now set up Pipelines to establish a repeatable process to build and train custom ML models using Autopilot. We use Boto3 and the SageMaker SDK to launch, track, and evaluate the AutoML jobs in an automated fashion.
Define the pipeline steps
In this section, we walk you through setting up the four steps in the pipeline.
Start the Autopilot job
This pipeline step uses a Lambda step, which runs a serverless Lambda function. We use a Lambda step because the API call to Autopilot is lightweight. Lambda functions are serverless and well suited for this task. For more information about Lambda steps, refer to Use a SageMaker Pipeline Lambda step for lightweight model deployments. The Lambda function in the start_autopilot_job.py
script creates an Autopilot job.
We use the Boto3 Autopilot API call create_auto_ml_job to specify the Autopilot job configuration, with the following parameters:
- AutoMLJobName – The Autopilot job name.
- InputDataConfig – The training data, data location in Amazon S3, and S3 data type with valid values such as
S3Prefix
,ManifestFile
, andAugmentedManifestFile
. - OutputDataConfig – The S3 output path where artifacts from the AutoML job are stored.
- ProblemType – The problem type (
MulticlassClassification
for our use case). - AutoMLJobObjective –
F1macro
is our objective metric for our use case. - AutoMLJobConfig – The training mode is specified here. We use the newly released ensemble training mode powered by AutoGluon.
See the following code:
Check Autopilot job status
A Callback step helps us keep track of the status of the Autopilot training job.
The step repeatedly keeps track of the training job status by using a separate Lambda function in check_autopilot_job_status.py
until its completion.
The Callback step places a token in an Amazon Simple Queue Service (Amazon SQS) queue that triggers a Lambda function to check the training job status:
- If the job is still running, the Lambda function raises an exception and the message is placed back into the SQS queue
- If the job is complete, the Lambda function sends a success message back to the
Callback
step and the pipeline continues with the next step
We use a combination of a Callback
step and a Lambda function. There is an alternate option of using a SageMaker processing job instead.
Evaluate the best Autopilot model
The SageMaker processing step launches a SageMaker batch transform job to evaluate the trained Autopilot model against an evaluation dataset (the test set that was saved to the S3 bucket) and generates the performance metrics evaluation report and model explainability metrics. The evaluation script takes the Autopilot job name as an input argument and launches the batch transform job.
When the batch transform job is complete, we get output predictions for the test set. The output predictions are compared to the actual (ground truth) labels using Scikit-learn metrics functions. We evaluate our results based on the F1 score, precision, and recall. The performance metrics are saved to a JSON file, which is referenced when registering the model in the subsequent step.
Register the Autopilot model
We use another Lambda step, in which the Lambda function in register_autopilot_job.py
registers the Autopilot model to the SageMaker model registry using the evaluation report obtained in the previous SageMaker processing step. A Lambda step is used here for cost efficiency and latency.
At this point, we have successfully registered our new Autopilot model to the SageMaker model registry. You can view the new model on Studio by choosing Model registry on the SageMaker resources menu and opening autopilot-demo-package
. Choose any version of a training job to view the objective metrics under Model quality.
You can use the explainability report on the Explainability tab to understand your model’s predictions.
To view the experiments run for each model created, navigate to the Experiments and trials page. Choose (right-click) one of the listed experiments and choose Describe AutoML job to view the model leaderboard.
To view the pipeline steps on the Experiments and trials page, choose (right-click) the experiment and choose Open pipeline details.
Create and run the pipeline
After we define the pipeline steps, we combine them into a SageMaker pipeline. The steps are run sequentially. The pipeline runs all of the steps for an AutoML job, using Autopilot for training, model evaluation, and model registration. See the following code:
Deploy the model
After we have manually reviewed the ML model’s performance, we can deploy our newly created model to a SageMaker endpoint. For this, we can run the cell in the notebook that creates the model endpoint using the model configuration saved in the SageMaker model registry.
Note that this script is shared for demonstration purposes, but it’s recommended to follow a more robust CI/CD pipeline for production deployment. For more information, refer to Building, automating, managing, and scaling ML workflows using Amazon SageMaker Pipelines.
Conclusion
This post described an easy-to-use ML pipeline approach to automatically train tabular ML models (AutoML) using Autopilot, Pipelines, and Studio. AutoML improves ML practitioners’ efficiency, accelerating the path from ML experimentation to production without the need for extensive ML expertise. We outlined the respective pipeline steps needed for ML model creation, evaluation, and registration.
Get started by accessing the code on the GitHub repo to train and deploy your own custom AutoML models.
For more information on Pipelines and Autopilot, refer to Amazon SageMaker Pipelines and Automate model development with Amazon SageMaker Autopilot, respectively.
About the Authors
Pierre de Malliard is a Full-Stack Data Scientist for AWS and is passionate about helping customers improve their business outcomes with machine learning. He has been building AI/ML solutions across the healthcare sector. He holds multiple AWS certifications. In his free time, Pierre enjoys backcountry skiing and spearfishing.
Paavani Dua is an Applied Scientist in the AWS AI organization. At the Amazon ML Solutions Lab, she works with customers to solve their business problems using ML solutions. Outside of work, she enjoys hiking, reading, and baking.
Marcelo Aberle is an ML Engineer in the AWS AI organization. He is leading MLOps efforts at the Amazon ML Solutions Lab, helping customers design and implement scalable ML systems. His mission is to guide customers on their enterprise ML journey and accelerate their ML path to production. He is an admirer of California nature and enjoys hiking and cycling around San Francisco.
Startups across AWS Accelerators use AI and ML to solve mission-critical customer challenges
Relentless advancement in technology is improving the decision-making capacity of humans and enterprises alike. Digitization of the physical world has accelerated the three dimensions of data: velocity, variety, and volume. This has made information more widely available than before, allowing for advancements in problem-solving. Now, with cloud-enabled democratized availability, technologies like artificial intelligence (AI) and machine learning (ML) are able to increase the speed and accuracy of decision-making by humans and machines.
Nowhere is this speed and accuracy of decisions more important than in the public sector, where organizations across defense, healthcare, aerospace, and sustainability are solving challenges that impact citizens around the world. Many public sector customers see the benefits of using AI/ML to address these challenges, but can be overwhelmed with the range of solutions. AWS launched AWS Accelerators to find and develop startups with technologies that meet public sector customers’ unique challenges. Read on to learn more about AI/ML use cases from startups in the AWS Accelerator that are making an impact for public sector customers.
Healthcare
Pieces: Healthcare providers want to spend more time caring for patients and less time on paperwork. Pieces, an AWS Healthcare Accelerator startup, uses AWS to make it easier to input, manage, store, organize, and gain insight from Electronic Health Record (EHR) data to address social determinants of health and improve patient care. With AI, natural language processing (NLP), and clinically reviewed algorithms, Pieces can provide projected hospital discharge dates, anticipated clinical and non-clinical barriers to discharge, and risk of readmission. Pieces services also provide insights to healthcare providers in plain language and optimize clarity of patients’ clinical issues to help care teams work more efficiently. According to Pieces, the software delivers a 95% positive prediction in identifying barriers to patient discharge, and at one hospital, has shown its ability to reduce patient hospital stays on average by 2 days.
Pieces uses Amazon Elastic Compute Cloud (Amazon EC2), Amazon Relational Database Service (Amazon RDS), and Amazon Managed Streaming for Apace Kafka (Amazon MSK) for collecting and processing streamed clinical data. Pieces uses Amazon Elastic Kubernetes Service (Amazon EKS), Amazon OpenSearch Service, and Amazon Managed Workflows for Apache Airflow (Amazon MWAA) to run multiple ML models on data in production at scale.
PEP Health: Patient experience is a key priority, but gathering patient feedback can be a challenge. PEP Health, a startup in the AWS Healthcare Accelerator’s UK cohort, uses NLP technology to analyze millions of online, publicly posted patient comments, generating scores that highlight areas for celebration or concern, and identifying the reasons for improving or declining patient satisfaction. This data can be used to improve experiences, drive better outcomes, and democratize the patient voice.
PEP Health uses AWS Lambda, AWS Fargate, and Amazon EC2 to ingest information in real time from hundreds of thousands of webpages. With proprietary NLP models built and run on Amazon SageMaker, PEP Health identifies and scores themes relevant to the quality of care. These results feed PEP Health’s Patient Experience Platform and ML algorithms built and powered by Lambda, Fargate, Amazon EC2, Amazon RDS, SageMaker, and Amazon Cognito, which enable relationship analysis and uncover patterns between people, places, and things that may otherwise seem disconnected.
“Through the accelerator, PEP Health was able to scale its operations significantly with the introduction of AWS Lambda to collect more comments faster and more affordably. Additionally, we’ve been able to use Amazon SageMaker to derive further insights for customers.”
– Mark Lomax, PEP Health CEO.
Defense and space
Lunar Outpost: Lunar Outpost was part of the AWS Space Accelerator’s inaugural cohort in 2021. The company is taking part in missions to the Moon and is developing Mobile Autonomous Platform (MAP) rovers that will be capable of surviving and navigating the extreme environments of other planetary bodies. To successfully navigate in conditions that can’t be found on Earth, Lunar Outpost makes extensive use of robotic simulations to validate AI navigation algorithms.
Lunar Outpost uses AWS RoboMaker, Amazon EC2, Amazon Elastic Container Registry (Amazon ECR), Amazon Simple Storage Service (Amazon S3), Amazon Virtual Private Cloud (Amazon VPC), Lambda, AWS CodeBuild, and Amazon QuickSight to test rovers by deploying lunar simulations. As Lunar Outpost develops navigation technologies for the lunar surface, simulation instances are spun up. These simulations will be used during lunar missions to assist human operators and decrease risk. Data streamed back from the lunar surface will be imported into their simulation, giving a real-time view of the rover’s activities. Simulation of digital MAP rovers allows for trial runs of navigation trajectories without moving the physical rover, dramatically reducing the risks of moving rovers in space.
Adarga: Adarga, part of the first AWS Defense Accelerator cohort, is delivering an AI-driven intelligence platform to rapidly understand risks and opportunities for theater entry preparation and deployment. Adarga uses AI to find insights buried within large volumes of unstructured data, such as news, presentations, reports, videos, and more.
Adarga uses Amazon EC2, OpenSearch Service, Amazon Aurora, Amazon DocumentDB (with MongoDB compatibility), Amazon Translate, and SageMaker. Adarga ingests information in real time, translates foreign language documents, and transcribes audio and video files into text. In addition to SageMaker, Adarga uses proprietary NLP models to extract and classify details, like people, places, and things, deploying disambiguation techniques to contextualize the information. These details are mapped into a dynamic intelligence picture for customers. Adarga’s ML algorithms, together with AWS AI/ML services, enable relationship analysis, uncovering patterns that may otherwise seem disconnected.
“We are proud to be part of this pioneering initiative as we continue to work closely with AWS and a wider ecosystem of tech players to deliver game-changing capabilities to defence, enabled by hyperscale cloud.”
– Robert Bassett-Cross, CEO, Adarga
Sustainable cities
SmartHelio: Within the commercial solar farm industry, it is critical to determine the health of installed solar infrastructure. SmartHelio combines physics and SageMaker to construct models that determine the current health of solar assets, build predictions on which assets will fail, and determine proactively which assets to service first.
SmartHelio’s solution, built on AWS, analyzes incredibly complex photovoltaic physics and power systems. A data lake on Amazon S3 stores billions of data points streamed on a real-time basis from Supervisory Control and Data Acquisition (SCADA) servers on solar farms, Internet of Things (IoT) devices, or third-party Content Management Systems (CMS) platforms. SmartHelio uses SageMaker to run deep learning models to recognize patterns, quantify solar farm health, and predict farm losses on a real-time basis, delivering intelligent insights instantly to its customers.
After being selected for the first AWS Sustainable Cities Accelerator cohort, SmartHelio secured several pilots with new customers. In CEO Govinda Upadhyay’s words, “the AWS Accelerator gave us global exposure to markets, mentors, potential customers, and investors.”
Automotus: Automotus uses computer vision technology to give drivers the ability to view in real time if curb space is available, significantly reducing time spent searching for parking. Automotus helps cities and airports manage and monetize their curbs using a fleet of computer vision sensors powered by AWS IoT Greengrass. Automotus’s sensors upload training data to Amazon S3, where a workflow powered by Lambda indexes sample data to create complex datasets for training new models and improving existing ones.
Automotus uses SageMaker to automate and containerize its computer vision model training process, the outputs of which are deployed back to the edge using a simple, automated process. Equipped with these trained models, Automotus sensors send metadata to the cloud using AWS IoT Core, uncovering granular insights about curb activity and enabling fully automated billing and enforcement at the curb. With one customer, Automotus increased enforcement efficiency and revenue by more than 500%, resulting in a 24% increase in parking turnover and a 20% reduction in traffic.
What’s next for AI/ML and startups
Customers have embraced AI/ML to solve a wide spectrum of challenges, which is a testament to the advancement of the technology and the increased confidence customers have in using data to improve decision-making. AWS Accelerators aim to continue the acceleration and adoption of AI/ML solutions by helping customers brainstorm and share critical problem statements, and finding and connecting startups with these customers.
Interested in advancing solutions for public good through your startup? Or have a challenge in need of a disruptive solution? Connect with the AWS Worldwide Public Sector Venture Capital and Startups team today to learn more about AWS Accelerators and other resources available to drive decision-making innovations.
About the authors
Swami Sivasubramanian is Vice President of Data and Machine Learning at AWS. In this role, Swami oversees all AWS Database, Analytics, and AI & Machine Learning services. His team’s mission is to help organizations put their data to work with a complete, end-to-end data solution to store, access, analyze, and visualize, and predict.
Manpreet Mattu is the Global Head for Venture Capital and Startups Business Development for the World Wide Public Sector at Amazon Web Services (AWS). He has 15 years of experience in venture Investments and acquisitions in leading-edge technology and non-tech segments. Beyond tech, Manpreet’s interest spans history, philosophy, and economics. He is also an endurance runner.
Two Amazon Scholars named among inaugural Rousseeuw Prize winners
Prize recognizes pioneering work in statistical methodology, and aims to raise awareness of the impact of statistics on society.Read More
Cost efficient ML inference with multi-framework models on Amazon SageMaker
Machine learning (ML) has proven to be one of the most successful and widespread applications of technology, affecting a wide range of industries and impacting billions of users every day. With this rapid adoption of ML into every industry, companies are facing challenges in supporting low-latency predictions and with high availability while maximizing resource utilization and reducing associated costs. Because each ML framework has its own dependencies, and deployment steps for each framework are different, deploying models built in different frameworks in production and managing each of the endpoints becomes more and more complex.
Amazon SageMaker multi-container endpoints (MCEs) enables us to group models on different frameworks and deploy them to the same host, creating a single endpoint. You can provide containers for the different frameworks that you’re using to build the models, and SageMaker takes all of these containers and puts them behind one endpoint. For instance, you could have a PyTorch and a TensorFlow model loaded up on two dedicated endpoints serving the same or entirely different use cases, and both of these models have intermittent incoming traffic not utilizing resources to its limit. In such a scenario, you could club them together using containers into one endpoint using an MCE, improving the resource utilization while reducing the costs incurred in having both the models serving from different endpoints.
Multi-container endpoints provide a scalable and cost-effective solution to deploy up to 15 models built on different ML frameworks, model servers, and algorithms serving the same or different use case, meaning that you can have models built on diverse ML frameworks or intermediary steps across all of these containers and models. All these models can be accessed individually via direct invocation or stitched into a pipeline using serial invocation, where the output of one model is the input for the next one.
In this post, we discuss how to perform cost-efficient ML inference with multi-framework models on SageMaker.
MCE invocation patterns
SageMaker MCE direct invocation is useful in cases where you have clubbed unrelated models into an MCE endpoint or you’re running an A/B test between the models behind an MCE endpoint to gauge their performance. You can call the specific container directly in the API call and get the prediction from that model.
With serial invocation, you can stitch together 2–15 containers, and the output of one becomes the input of the next container in sequence. This is an ideal use case if, for example, you have a multi-step prediction pipeline where a Scikit-learn model is used for an intermediate prediction and the result is fed to a TensorFlow model for final inference. Instead of having them deployed as different endpoints and another application or job orchestrating them and making multiple API calls, you can deploy them as a SageMaker MCE, abstracting the logic and setting them up for serial invocation, where SageMaker manages the data transfer between one container to another automatically and emits the output of the final container to the client making the API request.
SageMaker MCE serial invocation is fundamentally different from a SageMaker serial inference pipeline (more details in the sections below). A serial inference pipeline is targeted more to orchestrate complex ML workflows such as data preprocessing, building a model ensemble, implementing conditional checks to determine which model to invoke, or postprocessing the prediction, involving business logic before the prediction is sent out to the downstream applications. In contrast, MCE serial invocation is designed to stitch 2–14 models into a pipeline for inference, each model taking the prediction of the previous model as input.
All the containers in an MCE are always in service and in memory, so there is no cold start while invoking the endpoint. MCEs also improve endpoint utilization and improve costs because models are deployed behind one endpoint and share the underlying compute instance, instead of each model occupying individual compute resources.
Let’s look at a few use cases and see how you can use SageMaker MCEs to optimize ML inference.
Use cases for SageMaker MCEs
Suppose you have two models for sentiment classification, one for English language and other for German language, and these models are serving different geographies with traffic coming in at different times in a day. Instead of having two endpoints running 24/7, you can deploy both of them into one endpoint using an MCE and access them using direct invocation, thereby optimizing your resource utilization and costs. See the following code:
In this example, we have two models (englishModel
and germanModel
), and we define the containers in the SageMaker create_model
construct and define the InferenceExecutionConfig
as ‘Direct’. Now we can call the endpoint for inference and define the TargetContainerHostname
as either englishModel
or germanModel
depending on the client making the API call:
You can also use direct invocation within the MCE to run A/B tests to compare the performance between the models.
The following diagram illustrates our architecture.
Similarly, in other ML use cases, when the trained model is used for processing a request, the model receives data in a format that needs to be preprocessed (for example, featurized) before it can be passed to the algorithm for inference. When ML algorithms are chained together, the output of one model serves as input for the next one before reaching the final result. In this case, you can build a SageMaker MCE serial pipeline, where the containers talk to each other in the sequence defined in the create_model
construct instead of you deploying each of the models into different endpoints and writing an independent logic to facilitate the flow of data between all these models and API calls. The following diagram illustrates this architecture.
For this use case, we use the following code:
In this example, we have two processing containers (Processing-1
and Processing-2
) for feature processing and data transformations, and two inference containers (Inference-1
and Inference-2
) to run ML model predictions on the preprocessed data. The PipelineModel
instance allows you to define the inference pipeline composed of a linear sequence of four containers that process requests for inference on data. The containers are co-located on the same instance, enabling you to run inference with low latency.
Scale multi-model endpoints for large numbers of models
The benefits of SageMaker multi-model endpoints increase based on the scale of model consolidation. You can see cost savings when hosting two models with one endpoint, and for use cases with hundreds or thousands of models, the savings are much greater.
Scaling the MCE endpoints is also straightforward using the SageMakerVariantInvocationsPerInstance
predefined metric, which gives the average number of times per minute that each instance for a model endpoint is invoked to define a TargetScaling
policy. SageMaker dynamically adjusts the number of instances provisioned for a model in response to changes in your workload. When the workload increases, autoscaling brings more instances online and loads with the target models and containers to keep up serving the requests. When the workload decreases, autoscaling removes unnecessary instances and offloads the model containers so that the containers don’t eat up the resources, and you don’t pay for instances that you aren’t using. The time to complete the first request against a given model experiences additional latency (called a cold start) to download the model from Amazon Simple Storage Service (Amazon S3) and load it into memory. Subsequent calls finish with no additional overhead because the model is already loaded. See the following code:
Following the preceding example policy configuration, we use the SageMakerVariantInvocationsPerInstance
predefined metric to adjust the number of variant instances so that each instance has an InvocationsPerInstance
metric of 70.
We can also scale SageMaker MCEs based on our own custom metric, such as CPUUtilization
, MemoryUtilization
, GPUUtilization
, GPUMemoryUtilization
, or DiskUtilization
, to scale up or down the number of instances based on utilization of a specific resource. For more information, refer to Automatically Scale Amazon SageMaker Models.
It’s recommended that the model in each container exhibits similar compute and latency requirements on each inference request, because if traffic to the MCE shifts from a high CPU utilization model to a low CPU utilization model, but the overall call volume remains the same, the endpoint doesn’t scale out and there may not be enough instances to handle all the requests to the high CPU utilization model.
Secure MCEs
For MCEs with direct invocation, multiple containers are hosted in a single instance by sharing memory and a storage volume. It’s important to secure the containers, maintain the correct mapping of requests to target containers, and provide users with the correct access to target containers. You can restrict invoke_endpoint
access to a limited set of containers inside an MCE using the sagemaker:TargetContainerHostname
AWS Identity and Access Management (IAM) condition key. SageMaker uses IAM roles to provide IAM identity-based policies that you use to specify allowed or denied actions and resources and the conditions under which actions are allowed or denied. The following policies show how to limit calls to specific containers within an endpoint:
Monitor multi-model endpoints using Amazon CloudWatch metrics
To make price and performance trade-offs, you’ll want to test multi-model endpoints with models and representative traffic from your own application. SageMaker provides additional metrics in Amazon CloudWatch for multi-model endpoints so you can determine the endpoint usage and the cache hit rate and optimize your endpoint. The metrics are as follows:
- ModelLoadingWaitTime – The interval of time that an invocation request waits for the target model to be downloaded or loaded to perform the inference.
- ModelUnloadingTime – The interval of time that it takes to unload the model through the container’s
UnloadModel
API call. - ModelDownloadingTime – The interval of time that it takes to download the model from Amazon S3.
- ModelLoadingTime – The interval of time that it takes to load the model through the container’s
LoadModel
API call. - ModelCacheHit – The number of
InvokeEndpoint
requests sent to the endpoint where the model was already loaded. Taking theAverage
statistic shows the ratio of requests in which the model was already loaded. - LoadedModelCount – The number of models loaded in the containers in the endpoint. This metric is emitted per instance. The
Average
statistic with a period of 1 minute tells you the average number of models loaded per instance, and theSum
statistic tells you the total number of models loaded across all instances in the endpoint. The models that this metric tracks aren’t necessarily unique because you can load a model in multiple containers in the endpoint.
There are also several other metrics that are used by each container running on an instance, such as Invocations
indicating the number of InvokeEndpoint
requests sent to a container inside an endpoint, ContainerLatency
giving the time an endpoint took for the target container or all the containers in a serial invocation to respond as viewed from SageMaker, and CPUUtilization
and MemoryUtilizaton
indicating the CPU units and percentage of memory.
Conclusion
In the post, we discussed how SageMaker multi-container endpoints can be helpful in optimizing costs and resource utilization. Examples of when to utilize MCEs include, but are not limited to, the following:
- Hosting models across different frameworks (such as TensorFlow, PyTorch, and Scikit-learn) that don’t have sufficient traffic to saturate the full capacity of an instance
- Hosting models from the same framework with different ML algorithms (such as recommendations, forecasting, or classification) and handler functions
- Comparisons of similar architectures running on different framework versions (such as TensorFlow 1.x vs. TensorFlow 2.x) for scenarios like A/B testing
SageMaker MCEs support deploying up to 15 containers on real-time endpoints and invoking them independently for low-latency inference and cost savings. The models can be completely heterogenous, with their own independent serving stack. You can either invoke these containers sequentially or independently for each request. Securely hosting multiple models, from different frameworks, on a single instance could save you up to 90% in cost compared to hosting models in dedicated single-instance endpoints.
About the authors
Dhawal Patel is a Principal Machine Learning Architect at AWS. He has worked with organizations ranging from large enterprises to mid-sized startups on problems related to distributed computing and artificial intelligence. He focuses on deep learning, including NLP and computer vision domains. He helps customers achieve high-performance model inference on Amazon SageMaker.
Vikram Elango is a Senior AI/ML Specialist Solutions Architect at Amazon Web Services, based in Virginia, US. Vikram helps global financial and insurance industry customers with design and thought leadership to build and deploy machine learning applications at scale. He is currently focused on natural language processing, responsible AI, inference optimization, and scaling ML across the enterprise. In his spare time, he enjoys traveling, hiking, cooking, and camping with his family.
Saurabh Trikande is a Senior Product Manager for Amazon SageMaker Inference. He is passionate about working with customers and is motivated by the goal of democratizing machine learning. He focuses on core challenges related to deploying complex ML applications, multi-tenant ML models, cost optimizations, and making deployment of deep learning models more accessible. In his spare time, Saurabh enjoys hiking, learning about innovative technologies, following TechCrunch, and spending time with his family.
Solve business problems end-to-end through machine learning in Amazon SageMaker JumpStart solutions
Amazon SageMaker JumpStart provides pre-trained, open-source models for a wide range of problem types to help you get started with machine learning (ML). JumpStart also provides solution templates that set up infrastructure for common use cases, and executable example notebooks for ML with Amazon SageMaker.
As a business user, you get to do the following with JumpStart solutions:
- Explore the solutions and evaluate which are a good match for your business needs.
- Launch solutions with a single click in Amazon SageMaker Studio. This launches an AWS CloudFormation template to create the required resources.
- Modify the solution to meet your needs with access to underlying notebook and model assets.
- Delete the acquired resources once done.
This post focuses on the five ML solutions that were recently added to address five different business challenges. As of this writing, JumpStart offers 23 business solutions varying from detecting fraud in financial transactions to recognizing handwriting. The number of solutions that are offered through JumpStart increase on a regular basis as more solutions are added to it.
Solution overview
The five new solutions are as follows:
- Price optimization – Offers customizable ML models to help you make optimal decisions for setting the price of your product or service in order to achieve your business objective, such as maximizing revenue, profit, or other custom metrics.
- Bird species prediction – Shows how you can train and fine-tune an object detection model. It demonstrates model tuning through training image augmentation, and charts the accuracy improvements that occur across the iterations (epochs) of the training job.
- Lung cancer survival prediction – Shows how you can feed 2D and 3D radiomic features and patient demographics to an ML algorithm to predict a patient’s lung cancer survival chances. The results from this prediction can help providers take appropriate proactive measures.
- Financial payment classification – Demonstrates how to train and deploy an ML model to classify financial transactions based on transaction information. You can also use this solution as an intermediate step in fraud detection, personalization, or anomaly detection.
- Churn prediction for mobile phone customers – Demonstrates how to quickly develop a churn prediction model using a mobile call transaction dataset. This is a simple example for users that are new to ML.
Prerequisites
To use these solutions, make sure that you have access to Studio with an execution role that allows you to run SageMaker functionality. For your user role within Studio, make sure that the SageMaker Projects and JumpStart option is turned on.
In the following sections, we go through each of the five new solutions and discuss how it works in detail, along with some recommendations on how you can use it for your own business needs.
Price optimization
Businesses like using various levers to fetch the best results. For example, the price of a product or a service is a lever that a business can control. The question is how to decide what price to set a product or service at, in order to maximize a business objective such as profit or revenue.
This solution provides customizable ML models to help you make optimal decisions for setting the price of your product or service in order to achieve your objective, such as maximizing revenue, profit, or other custom metrics. The solution uses ML and causal inference approaches to learn price-volume relations from historical data, and is able to make dynamic price recommendations in real time to optimize the custom objective metrics.
The following screenshot shows the sample input data.
The solution includes three parts:
- Price elasticity estimation – This is estimated by causal inference via a double ML algorithm
- Volume forecast – This is forecasted using the Prophet algorithm
- Price optimization – This is achieved by a what-if simulation through different price scenarios
The solution provides the recommended price for the next day for maximizing revenue. In addition, the outputs include the estimated price elasticity, which is a value indicating the effect of price on volume, and a forecast model, which is able to forecast the next day’s volume. The following chart shows how a causal model that incorporated the calculated price elasticity performs much better under a what-if analysis (with large deviations from behavior price) than a predictive model that uses Prophet for forecasting volume using time series data.
You could apply this solution to your business for the following use cases:
- Determine the optimal price of goods for a retail store
- Estimate the effect of discount coupons on customer purchases
- Predict the effect of various incentive methods in any business
Bird species prediction
There are several computer vision (CV) applications for businesses today. One of those applications is object detection, where an ML algorithm detects the location of an object in an image by drawing a bounding box around it, and identifies the type of object it is. Learning how to apply an object detection model and fine-tune it can be of great value to an organization that has CV needs.
This solution provides an example of how to translate bounding box specifications when providing images to the SageMaker algorithm. This solution also demonstrates how to improve an object detection model by adding training images that are flipped horizontally (mirror images).
A notebook is provided for experimenting with object detection challenges when there are a large number of classes (200 bird species). The notebook also shows how to chart the accuracy improvements that occur across the epochs of the training job. The following image shows example images from the birds dataset.
This solution contains five steps:
- Prepare the data, including download and
RecordIO
file generation. - Create and train an object detection model.
- Deploy an endpoint and evaluate model performance.
- Create and train an object detection model again with the expanded dataset.
- Deploy an endpoint and evaluate the expanded model performance.
You get the following as output:
- Object detection results with bonding boxes against your test image
- A trained object detection model
- A trained object detection model with an additional expanded (flipped) dataset
- Two separate endpoints deployed with one of each model
The following chart shows model improvement against model iterations (epochs) during training.
The following examples are output from two test images.
You could apply this solution to your business for the following use cases:
- Detect objects on a conveyer belt in a packaging industry
- Detect toppings on a pizza
- Implement supply chain operational applications that involve object detection
Lung cancer survival prediction
COVID-19 brought a lot more attention to lung-related medical challenges. It has also put a lot of pressure on hospitals, doctors, nurses, and radiologists. Imagine a possibility where you can apply ML as a powerful tool to assist medical practitioners and help them speed up their work. In this solution, we show how 2D and 3D radiomic features and patient demographics can be fed to an ML algorithm to predict a patient’s lung cancer survival chances. Results from this prediction can help providers take appropriate proactive measures.
This solution demonstrates how to build a scalable ML pipeline for the Non-Small Cell Lung Cancer (NSCLC) Radiogenomics dataset, which consists of RNA sequencing data, clinical data (reflective of EHR data), and medical images. Using multiple types of data to create a machine model is referred to as multi-modal ML. This solution predicts survival outcome of patients diagnosed with non-small cell lung cancer.
The following image shows an example of the input data from the Non-Small Cell Lung Cancer (NSCLC) Radiogenomics dataset.
As part of the solution, total RNA was extracted from the tumor tissue and analyzed with RNA sequencing technology. Although the original data contains more than 22,000 genes, we keep 21 genes from 10 highly coexpressed gene clusters (metagenes) that were identified, validated in publicly available gene-expression cohorts, and correlated with prognosis.
The clinical records are stored in CSV format. Each row corresponds to a patient, and the columns contain information about the patients, including demographics, tumor stage, and survival status.
For genomic data, we keep 21 genes from 10 highly coexpressed gene clusters (metagenes) that were identified, validated in publicly available gene-expression cohorts, and correlated with prognosis.
For medical imaging data, we create patient-level 3D radiomic features that explain the size, shape, and visual attributes of the tumors observed in the CT scans. For each patient study, the following steps are performed:
- Read the 2D DICOM slice files for both the CT scan and tumor segmentation, combine them to 3D volumes, save the volumes in NIfTI format.
- Align CT volume and tumor segmentation so we can focus the computation inside the tumor.
- Compute radiomic features describing the tumor region using the pyradiomics library.
- Extract 120 radiomic features of eight classes, such as statistical representations of the distribution and co-occurrence of the intensity within tumorous region of interest, and shape-based measurements describing the tumor morphologically.
To create a multi-modal view of a patient for model training, we join the feature vectors from three modalities. We then process the data. First, we normalize the range of independent features using feature scaling. Then we perform principal component analysis (PCA) on the features to reduce the dimensionality and identify the most discriminative features that contribute 95% variance in the data.
This results in a dimensionality reduction from 215 features down to 45 principal components, which constitute features for the supervised learner.
The solution produces an ML model that predicts NSCLC patients’ survival status (dead or alive) in a form of probability. Besides the model and prediction, we also generate reports to explain the model. The medical imaging pipeline produces 3D lung CT volumes and tumor segmentation for visualization purposes.
You can apply this solution to healthcare and life sciences use cases.
Financial payment classification
Taking all financial transactions of a business or a consumer and organizing them into various categories can be quite helpful. It can help the user learn how much they have spent in which category, and it can also raise alerts when transactions or spending in a given category goes up or down unexpectedly.
This solution demonstrates how to train and deploy an ML model to classify financial transactions based on transaction information. Many banks provide this as a service to give their end-users an overview of their spending habits. You can also use this solution as an intermediate step in fraud detection, personalization, or anomaly detection. We use SageMaker to train and deploy an XGBoost model with the required underlying infrastructure.
The synthetic dataset that we to demonstrate this solution has the following features:
- transaction_category – The category of the transaction, out of the following 19 options:
Uncategorized
,Entertainment
,Education
,Shopping
,Personal Care
,Health and Fitness
,Food and Dining
,Gifts and Donations
,Investments
,Bills and Utilities
,Auto and Transport
,Travel
,Fees and Charges
,Business Services
,Personal Services
,Taxes
,Gambling
,Home
, andPension and insurances
. - receiver_id – An identifier for the receiving party. The identifier consists of 16 numbers.
- sender_id – An identifier for the sending party. The identifier consists of 16 numbers.
- amount – The amount that is transferred.
- timestamp – The timestamp of the transaction in YYYY-MM-DD HH:MM:SS format.
The first five observations of the dataset are as follows:
For this solution, we use XGBoost, a popular and efficient open-source implementation of the gradient boosted trees algorithm. Gradient boosting is a supervised learning algorithm that attempts to accurately predict a target variable by combining an ensemble of estimates from a set of simpler and weaker models. Its implementation is available in the SageMaker built-in algorithms.
The financial payment classification solution contains four steps:
- Prepare the data.
- Build a feature store.
- Create and train an XGBoost model.
- Deploy an endpoint and evaluate model performance.
We get the following output:
- A trained XGBoost model based on our example dataset
- A SageMaker endpoint that can predict the transaction category
After running this solution, you should see a classification report similar to the following.
Possible applications for your business include the following:
- Various financial applications in retail and investment banking
- When transactions need to be classified in any use case (not just financial)
Churn prediction for mobile phone customers
Predicting customer churn is a very common business need. Numerous studies show that the cost of retaining an existing customer is much less than acquiring a new customer. The challenge often comes from businesses having a tough time understanding why a customer is churning, or building a model that predicts churning.
In this example, users that are new to ML can experience how a churn prediction model can be quickly developed using a mobile call transaction dataset. This solution uses SageMaker to train and deploy an XGBoost model on a customer profile dataset to predict whether a customer is likely to leave a mobile phone operator.
The dataset this solution uses is publicly available and is mentioned in the book Discovering Knowledge in Data by Daniel T. Larose. It is attributed by the author to the University of California Irvine Repository of Machine Learning Datasets.
This dataset uses the following 21 attributes to describe the profile of a customer of an unknown US mobile operator.
- State: the US state in which the customer resides, indicated by a two-letter abbreviation; for example, OH or NJ
- Account Length: the number of days that this account has been active
- Area Code: the three-digit area code of the corresponding customer’s phone number
- Phone: the remaining seven-digit phone number
- Int’l Plan: whether the customer has an international calling plan: yes/no
- VMail Plan: whether the customer has a voice mail feature: yes/no
- VMail Message: the average number of voice mail messages per month
- Day Mins: the total number of calling minutes used during the day
- Day Calls: the total number of calls placed during the day
- Day Charge: the billed cost of daytime calls
- Eve Mins, Eve Calls, Eve Charge: the billed cost for calls placed during the evening
- Night Mins, Night Calls, Night Charge: the billed cost for calls placed during nighttime
- Intl Mins, Intl Calls, Intl Charge: the billed cost for international calls
- CustServ Calls: the number of calls placed to Customer Service
- Churn?: whether the customer left the service: true/false
This solution contains three stages:
- Prepare the data.
- Create and train an XGBoost model.
- Deploy an endpoint and evaluate model performance.
We get the following output:
- A trained XGBoost model based on our example dataset to predict user churn
- A SageMaker endpoint that can predict user churn
This model helps estimate how many of the 5,000 mobile phone customers are likely to stop using their current mobile phone operator.
The following chart shows a probability distribution of the churn as an output from the model.
You could apply this to your business for the following use cases:
- Predict customer churn in your own business
- Classify which customers may open your marketing email and who will not (binary classification)
- Predict which students are likely to drop out from a course
Clean up resources
After you’re done running a solution in JumpStart, make sure to choose Delete all resources so all the resources that you have created in the process are deleted and your billing is stopped.
Summary
This post showed you how to solve various business problems by applying ML, based on JumpStart solutions. Although this post focused on the five new solutions that were recently added to JumpStart, there are a total of 23 available solutions. We encourage you to log in to Studio and look at the JumpStart solutions yourselves and start deriving immediate value out of them. For more information, refer to Amazon SageMaker Studio and SageMaker JumpStart.
Note: If you don’t see all of the above five solutions in the JumpStart console of your AWS region, please wait for a week and check again. We are releasing them to various regions in a phased manner.
About the Authors
Dr. Raju Penmatcha is an AI/ML Specialist Solutions Architect in AI Platforms at AWS. He works on the low-code/no-code suite of services in SageMaker that help customers easily build and deploy machine learning models and solutions. When not helping customers, he likes traveling to new places.
Manan Shah is a Software Development Manager at Amazon Web Services. He is an ML enthusiast and focuses on building no-code/low-code AI/ML products. He strives to empower other talented, technical people to build great software.
Train gigantic models with near-linear scaling using sharded data parallelism on Amazon SageMaker
In the pursuit of superior accuracy, deep learning models in areas such as natural language processing and computer vision have significantly grown in size in the past few years, frequently counted in tens to hundreds of billions of parameters. Training these gigantic models is challenging and requires complex distribution strategies. Data scientists and machine learning engineers are constantly looking for the best way to optimize their training compute, yet are struggling with the communication overhead that can increase along with the overall cluster size.
This is why we recently launched sharded data parallelism on Amazon SageMaker, a new memory-saving distributed training technique in the SageMaker model parallel (SMP) library. Sharded data parallelism is purpose-built for extreme-scale models and uses Amazon in-house MiCS technology under the hood, a science effort to minimize the communication scale by bringing down expensive communication overhead rooted in parameter gathering and gradient synchronization. With a 30B parameter GPT-2 model with sequence length 2048, this new feature achieved 141 TFLOPs, a 39.7% speed up compared to DeepSpeed ZeRO-3. For a 10B GPT-2 model with sequence length 512, this new feature also achieved 564 samples per second, a 13.9% speed up compared to PyTorch’s Fully Sharded Data Parallel (FSDP). Remember that in gigantic model training, every percentage of speedup translates to dollars saved and productivity gained in your team.
In this blog post, we’ll first take a closer look at the key differentiators of sharded data parallelism and when to use it. Then, you’ll learn how to train a 30B parameter GPT-2 model on SageMaker with ease with this new feature. Finally we’ll compare the performance with other open source options, notably outperforming DeepSpeed ZeRO by up to 39.7% on 256 GPUs.
How sharded data parallelism works and when to use it
Before we introduce sharded data parallelism, let’s look at its broader technique family. Recent distributed training approaches for large models have moved to a paradigm where model parameters, gradients, and optimizer states are shared across data-parallel nodes. Unlike Pipeline Parallelism which has the innate complexity of choosing layers to partition across devices especially when your framework doesn’t support automated model splitting, this paradigm elegantly preserves the simplicity of data parallelism, while removing data parallelism’s constraint where a model must fit into a single GPU.
In existing frameworks that fall under this paradigm, notably DeepSpeed ZeRO-3 and PyTorch’s FSDP upstreamed from FairScale, model states are sharded across all GPUs, a strategy that lowers the memory consumption on each GPU at the cost of incurring large communication overhead which increases with cluster size and therefore causes the scalability to significantly drop at scale. In contrast, sharded data parallelism in the SMP library partitions model states in a scale-aware manner by partitioning each replica of model states only within a subset of GPUs.
Let’s look closer at the scale-aware model partitioning in MiCS, the core technology behind sharded data parallel. The intuition behind this design is that partitioning training states across the entire data-parallel group may not be required to train a model with tens of billions of parameters. For example, 8 V100 GPUs (32GB each) are sufficient to hold the model states replica of a 10B-parameter model which needs about 200GB of memory when training with Adam optimizer using mixed-precision. By limiting a complete replica of model states in the smallest subset of GPUs, we can effectively reduce the scale of communication overhead compared to DeepSpeed and PyTorch FSDP. Sharded data parallel also leverages other techniques in MiCS such as Hierarchical Communication and 2-hop Gradient Synchronization. For more information, check out Near-linear scaling of gigantic-model training on AWS or MiCS: Near-linear Scaling for Training Gigantic Model on Public Cloud.
Now, how do you know when to choose sharded data parallel over other distributed training techniques? The general rule is that if your model has less than 1 billion parameters and can fit into GPU memory, SageMaker data parallel library or SageMaker training compiler can be sufficient for you. If you have larger language or computer vision models, our suggestion is to train it with the sharded data parallelism technique combined with activation checkpointing and activation offloading in the SageMaker model parallel library first, before other techniques such as tensor parallelism or pipeline parallelism.
Using sharded data parallelism to train GPT-2 on Amazon SageMaker
Let’s now learn how to train a GPT-2 model with sharded data parallel, with SMP encapsulating the complexity for you. This complete tutorial notebook walks you through the entire process, from data processing, defining and submitting training jobs, to monitoring training logs. What follows is a brief overview highlighting key steps for using this feature.
1. Get started
Sharded data parallelism is available in PyTorch v1.12.0+ and works with both FP16 and BF16. The easiest way to use the SMP library is through a prebuilt AWS Deep Learning Container for PyTorch. However, if you want to bring your own Docker container, you can refer to Create Your Own Docker Container with the SageMaker Distributed Model Parallel Library. To get started, follow Modify a PyTorch Training Script to adapt SMPs’ APIs in your training script. In this section, we only call out a few main steps with code snippets from the ready-to-use training script train_gpt_simple.py
. You can follow the comments in the script and API document to learn more about where SMP APIs are used.
First, import and initialize the library by calling smdistributed.modelparallel.torch.init()
at the beginning of the training script:
Second, wrap the model to be partitioned with smdistributed.modelparallel.torch.DistributedModel and use the returned DistributedModel
object going forward:
Wrap the optimizer with smdistributed.modelparallel.torch.DistributedOptimizer
for saving and loading optimizer states.
Put the forward and backward logic in a step function and decorate it with smdistributed.modelparallel.torch.step
. Any computation defined inside the smp.step-decorated
function is executed in a distributed manner.
2. Prepare the dataset
We use the openwebtext is the dataset we use in this example. The notebook uses the script data_prep_512.py
to download and preprocess the dataset. You can also train with other datasets by modifying data_pipeline.py
. When dealing with large dataset and model, you can speed up the training job by using data stored in Amazon FSx for Lustre, which provides a high-performance file system natively integrated with Amazon Simple Storage Service (S3). Please see the instructions from Configure Data Input Channel to Use Amazon FSx for Lustre for guidance on setting an FSx Lustre file system as data input channel.
3. Start the training jobs
This step assumes you have already modified your training script and prepared the dataset as mentioned in the preceding sections. To enable sharded data parallelism, simply set the sharded_data_parallel_degree
in the PyTorch Estimator. In this tutorial, we set sharded_data_parallel_degree=128
and instace_count=32
for p4d.24xlarge nodes, which indicates that the model states will be sharded across 128 GPUs among the total 256 GPUs. Based on this selected value, SMP will then automatically sets the data parallel degree to 2 (because 256/128=2), meaning we’ll have two replicas for data parallelism. A general rule for picking an ideal value for sharded_data_parallel_degree
is to add one more node to the sharing group per every 3B of model parameters. In this tutorial, our model size is 30B, so we should use at least 10 nodes for sharding. And because 16 nodes (128 GPUs) is the smallest power-of-2 above the threshold, we set sharded_data_parallel_degree=128
.
For checkpointing, we also provide a set of checkpointing utilities in sharded_data_parallel_checkpoint.py
, including a utility to reconstruct the full state_dict
for advanced use cases. Finally, we can launch a distributed training job by calling fit() on the Estimator.
4. Monitor the training jobs
You can access the training logs and track GPU and memory utilization on Amazon CloudWatch. Make sure to look at the logs of “algo-1” because that is the main node whose output stream has the training job logs from all instances.
Benchmarking performance
We benchmarked sharded data parallelism in the SMP library on both 16 and 32 p4d.24xlarge nodes for sequence length 512 and 2048, respectively. The 30B-parameter GPT2 model is configured to use a hidden width of 7168, 48 layers, and 64 heads. You can adopt the exact same configuration where sequence length is 2048 by setting model_config = "gpt2-30b"
in the tutorial notebook. With this setting, SMP achieved 73.52 samples per second, a 39.7% speed up compared to DeepSpeed ZeRO-3. If your token size is 500 billion, this speed up means nearly 367 hours of savings on p4d.24xlarge nodes, an equivalent of more than $12,000 budget saved per training! The following table summarizes our benchmark results.
Configuration | Performance | Time to train with SMP (days) | |||||||
Model/Training | Cluster | DeepSpeed | SMP | Speed (samples/sec) DeepSpeed v0.7.2 |
Speed (samples/sec) SMP v1.11 |
% Speedup of SMP | TFLOPS achieved by SMP | 100 billion tokens | 500 billion tokens |
30B GPT-2 Seq length:512 Global batch size:3072 FP16 |
16 p4d.24xlarge nodes | Activation checkpointing gradient_accumulation_steps:2 |
Activation checkpointing sharded_data_parallel_degree:64 gradient_accumulation:1 |
142 | 181.05 | 27.5 | 173.6 | 12.49 | 62.43 |
30B GPT-2 Seq length:2048 Global batch size 1536 FP16 |
32 p4d.24xlarge nodes | Activation checkpointing gradient_accumulation_steps:2 |
Activation checkpointing sharded_data_parallel_degree:128 gradient_accumulation:1 |
52.6 | 73.52 | 39.77 | 141 | 7.69 | 38.43 |
1/ For each model configuration, we tested different features, stages, and configurations in DeepSpeed ZeRO and chose the one that provides the best throughput as the DeepSpeed baseline. The benchmark was run on Amazon Elastic Compute Cloud (Amazon EC2). 2/ These results rely on improved communication collectives optimized for AWS which will be made available soon. 3/ Time to train is projected from speed based on number of tokens processed.
In summary, we observed consistently higher throughput with sharded data parallelism in SMP when compared to DeepSpeed across a range of models and configurations. This new feature also demonstrated a better memory efficiency compared to DeepSpeed, enabling SMP to fit a larger batch size and reduce the level of gradient accumulation required to fit a particular global batch size.
Conclusion
In this post, we introduced a new distributed training technique — sharded data parallelism — and how it speeds up gigantic model training with near linear-scaling on Amazon SageMaker. We also walked through how to train a GPT-2 model with the new technique following this complete example. You can follow the Amazon SageMaker Examples GitHub repo to track all SageMaker model parallel examples or attend our next distributed training workshops. To learn more about sharded data parallelism, please see the documentation.
About the authors
Emily Webber joined AWS just after SageMaker launched, and has been trying to tell the world about it ever since! Outside of building new ML experiences for customers, Emily enjoys meditating and studying Tibetan Buddhism.
Can Karakus is a Senior Applied Scientist at AWS, optimizing large-scale distributed deep learning on AWS. His research interests cover deep learning, distributed optimization, distributed systems, and information theory. Outside of work, he enjoys cycling, traveling, reading and learning.
Rahul Huilgol is a Senior Software Engineer at AWS. He works on distributed deep learning systems, towards making it easy and performant to train large deep learning models in the cloud. In his spare time, he enjoys photography, biking and gardening.
Suhit Kodgule is a Software Development Engineer with AWS Artificial Intelligence group working on deep learning frameworks. In his spare time, he enjoys hiking, traveling and cooking.
Erin Ho is a Product Manager for AWS Deep Learning. She works on products that make it easier for customers to train deep learning models on AWS. For fun outside work, she enjoys hiking and skiing.