With the growth in adoption of online applications and the rising number of internet users, digital fraud is on the rise year over year. Amazon Fraud Detector provides a fully managed service to help you better identify potentially fraudulent online activities using advanced machine learning (ML) techniques, and more than 20 years of fraud detection expertise from Amazon.
To help you catch fraud faster across multiple use cases, Amazon Fraud Detector offers specific models with tailored algorithms, enrichments, and feature transformations. The model training is fully automated and hassle-free, and you can follow the instructions in the user guide or related blog posts to get started. However, with trained models, you need to decide whether the model is ready for deployment. This requires certain knowledge in ML, statistics, and fraud detection, and it may be helpful to know some typical approaches.
This post will help you to diagnose model performance and pick the right model for deployment. We walk through the metrics provided by Amazon Fraud Detector, help you diagnose potential issues, and provide suggestions to improve model performance. The approaches are applicable to both Online Fraud Insights (OFI) and Transaction Fraud Insights (TFI) model templates.
Solution overview
This post provides an end-to-end process to diagnose your model performance. It first introduces all the model metrics shown on the Amazon Fraud Detector console, including AUC, score distribution, confusion matrix, ROC curve, and model variable importance. Then we present a three-step approach to diagnose model performance using different metrics. Finally, we provide suggestions to improve model performance for typical issues.
Prerequisites
Before diving deep into your Amazon Fraud Detector model, you need to complete the following prerequisites:
- Create an AWS account.
-
Create an event dataset for model training.
-
Upload your data to Amazon Simple Storage Service (Amazon S3) or ingest your event data into Amazon Fraud Detector.
-
Build an Amazon Fraud Detector model.
Interpret model metrics
After model training is complete, Amazon Fraud Detector evaluates your model using part of the modeling data that wasn’t used in model training. It returns the evaluation metrics on the Model version page for that model. Those metrics reflect the model performance you can expect on real data after deploying to production.
The following screenshot shows example model performance returned by Amazon Fraud Detector. You can choose different thresholds on score distribution (left), and the confusion matrix (right) is updated accordingly.
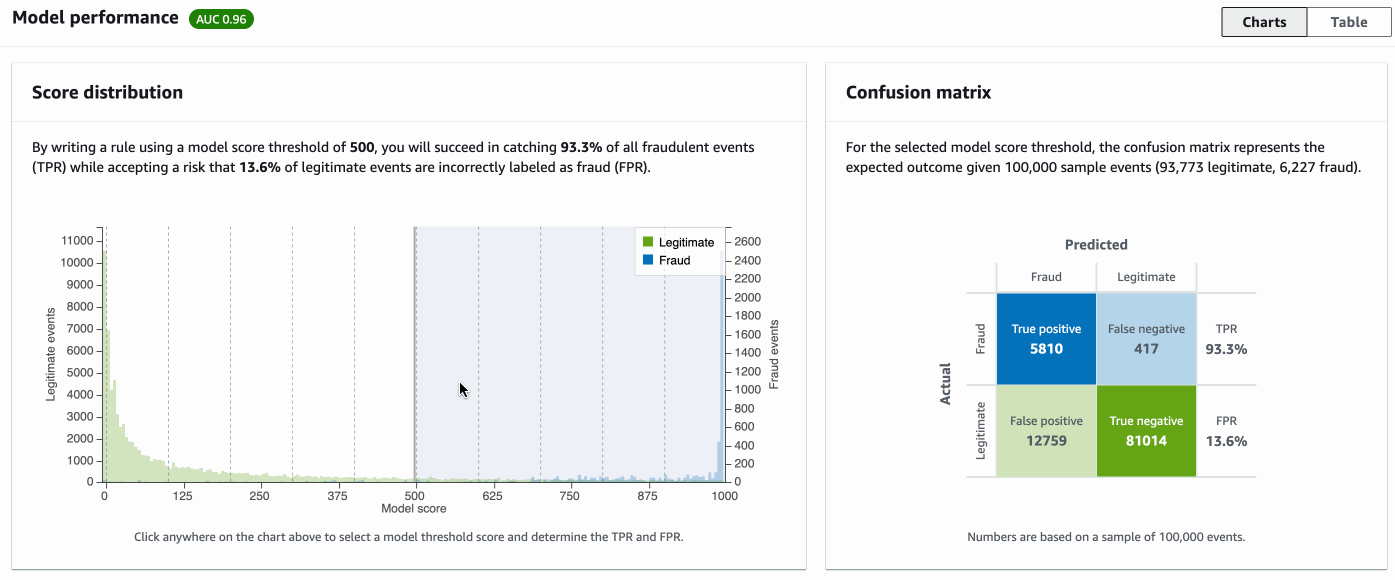
You can use the following findings to check performance and decide on strategy rules:
-
AUC (area under the curve) – The overall performance of this model. A model with AUC of 0.50 is no better than a coin flip because it represents random chance, whereas a “perfect” model will have a score of 1.0. The higher AUC, the better your model can distinguish between frauds and legitimates.
-
Score distribution – A histogram of model score distributions assuming an example population of 100,000 events. Amazon Fraud Detector generates model scores between 0–1000, where the lower the score, the lower the fraud risk. Better separation between legitimate (green) and fraud (blue) populations typically indicates a better model. For more details, see Model scores.
-
Confusion matrix – A table that describes model performance for the selected given score threshold, including true positive, true negative, false positive, false negative, true positive rate (TPR), and false positive rate (FPR). The count on the table assumes an example population of 100,0000 events. For more details, see Model performance metrics.
-
ROC (Receiver Operator Characteristic) curve – A plot that illustrates the diagnostic ability of the model, as shown in the following screenshot. It plots the true positive rate as a function of false positive rate over all possible model score thresholds. View this chart by choosing Advanced Metrics. If you have trained multiple versions of one model, you can select different FPR thresholds to check the performance change.
-
Model variable importance – The rank of model variables based on their contribution to the generated model, as shown in the following screenshot. The model variable with the highest value is more important to the model than the other model variables in the dataset for that model version, and is listed at the top by default. For more details, see Model variable importance.
Diagnose model performance
Before deploying your model into production, you should use the metrics Amazon Fraud Detector returned to understand the model performance and diagnose the possible issues. The common problems of ML models can be divided into two main categories: data-related issues and model-related issues. Amazon Fraud Detector has taken care of the model-related issues by carefully using validation and testing sets to evaluate and tune your model on the backend. You can complete the following steps to validate if your model is ready for deployment or has possible data-related issues:
- Check overall model performance (AUC and score distribution).
- Review business requirements (confusion matrix and table).
- Check model variable importance.
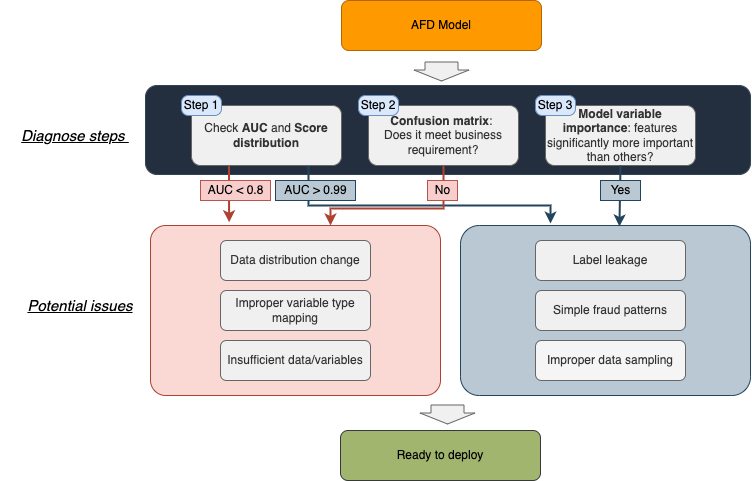
Check overall model performance: AUC and score distribution
More accurate prediction of future events is always the primary goal of a predictive model. The AUC returned by Amazon Fraud Detector is calculated on a properly sampled test set not used in training. In general, a model with an AUC greater than 0.9 is considered to be a good model.
If you observe a model with performance less than 0.8, it usually means the model has room for improvement (we discuss common issues for low model performance later in this post). Note that the definition of “good” performance highly depends on your business and the baseline model. You can still follow the steps in this post to improve your Amazon Fraud Detector model even though its AUC is greater than 0.8.
On the other hand, if the AUC is over 0.99, it means the model can almost perfectly separate the fraud and legitimate events on the test set. This is sometimes a “too good to be true” scenario (we discuss common issues for very high model performance later in this post).
Besides the overall AUC, the score distribution can also tell you how well the model is fitted. Ideally, you should see the bulk of legitimate and fraud located on the two ends of the scale, which indicates the model score can accurately rank the events on the test set.
In the following example, the score distribution has an AUC of 0.96.
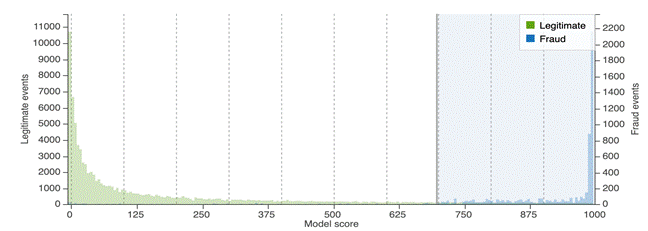
If the legitimate and fraud distribution overlapped or concentrated in the center, it probably means the model doesn’t perform well on distinguishing fraud events from legitimate events, which might indicate historical data distribution changed or that you need more data or features.
The following is an example of score distribution with an AUC of 0.64.
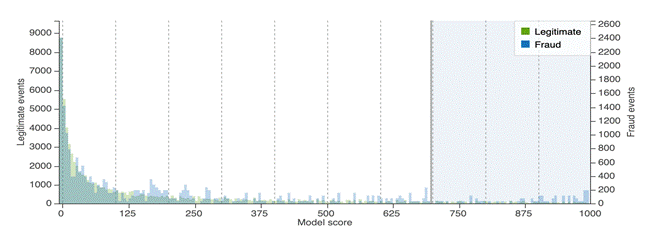
If you can find a split point that can almost perfectly split fraud and legitimate events, there is a high chance that the model has a label leakage issue or the fraud patterns are too easy to detect, which should catch your attention.
In the following example, the score distribution has an AUC of 1.0.
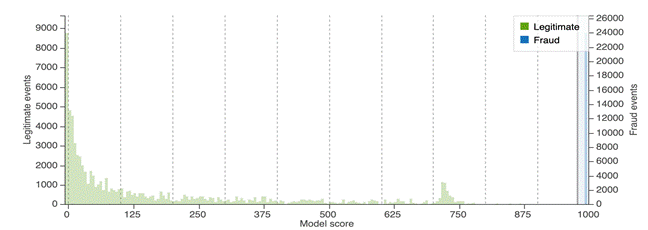
Review business requirements: Confusion matrix and table
Although AUC is a convenient indicator of model performance, it may not directly translate to your business requirement. Amazon Fraud Detector also provides metrics such as fraud capture rate (true positive rate), percentage of legitimate events that are incorrectly predicted as fraud (false positive rate), and more, which are more commonly used as business requirements. After you train a model with a reasonably good AUC, you need to compare the model with your business requirement with those metrics.
The confusion matrix and table provide you with an interface to review the impact and check if it meets your business needs. Note that the numbers depend on the model threshold, where events with scores larger than then threshold are classified as fraud and events with scores lower than the threshold are classified as legit. You can choose which threshold to use depending on your business requirements.
For example, if your goal is to capture 73% of frauds, then (as shown in the example below) you can choose a threshold such as 855, which allows you to capture 73% of all fraud. However, the model will also mis-classify 3% legitimate events to be fraudulent. If this FPR is acceptable for your business, then the model is good for deployment. Otherwise, you need to improve the model performance.
Another example is if the cost for blocking or challenging a legitimate customer is extremely high, then you want a low FPR and high precision. In that case, you can choose a threshold of 950, as shown in the following example, which will miss-classify 1% of legitimate customers as fraud, and 80% of identified fraud will actually be fraudulent.
In addition, you can choose multiple thresholds and assign different outcomes, such as block, investigate, pass. If you can’t find proper thresholds and rules that satisfy all your business requirements, you should consider training your model with more data and attributes.
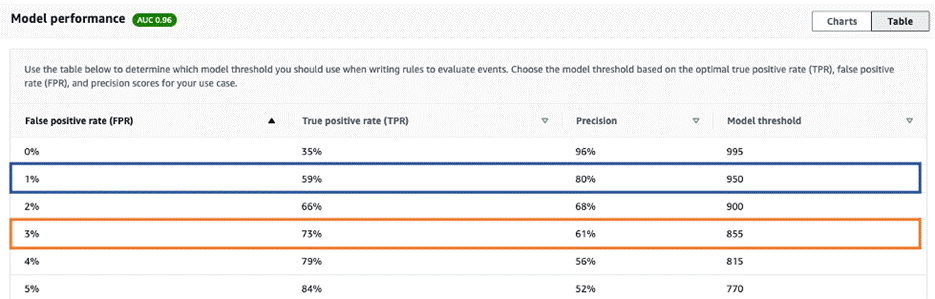
Check model variable importance
The Model variable importance pane displays how each variable contributes to your model. If one variable has a significantly higher importance value than the others, it might indicate label leakage or that the fraud patterns are too easy to detect. Note that the variable importance is aggregated back to your input variables. If you observe slightly higher importance of IP_ADDRESS
, CARD_BIN
, EMAIL_ADDRESS
, PHONE_NUMBER
, BILLING_ZIP
, or SHIPPING_ZIP
, it might because of the power of enrichment.
The following example shows model variable importance with a potential label leakage using investigation_status
.
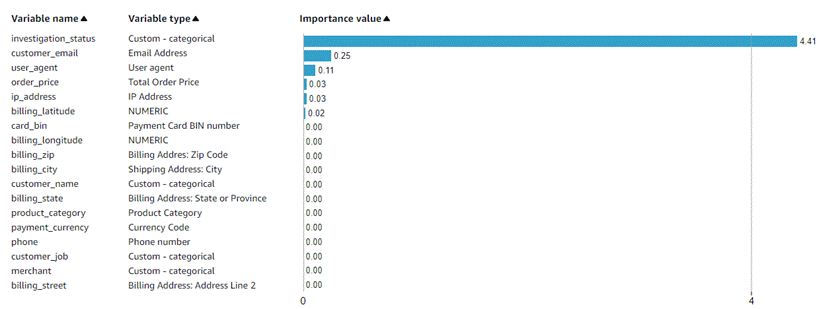
Model variable importance also gives you hints of what additional variables could potentially bring lift to the model. For example, if you observe low AUC and seller-related features show high importance, you might consider collecting more order features such as SELLER_CATEGORY
, SELLER_ADDRESS
, and SELLER_ACTIVE_YEARS
, and add those variables to your model.
Common issues for low model performance
In this section, we discuss common issues you may encounter regarding low model performance.
Historical data distribution changed
Historical data distribution drift happens when you have a big business change or a data collection issue. For example, if you recently launched your product in a new market, the IP_ADDRESS
, EMAIL
, and ADDRESS
related features could be completely different, and the fraud modus operandi could also change. Amazon Fraud Detector uses EVENT_TIMESTAMP
to split data and evaluate your model on the appropriate subset of events in your dataset. If your historical data distribution changes significantly, the evaluation set could be very different from the training data, and the reported model performance could be low.
You can check the potential data distribution change issue by exploring your historical data:
- Use the Amazon Fraud Detector Data Profiler tool to check if the fraud rate and the missing rate of the label changed over time.
- Check if the variable distribution over time changed significantly, especially for features with high variable importance.
- Check the variable distribution over time by target variables. If you observe significantly more fraud events from one category in recent data, you might want to check if the change is reasonable using your business judgments.
If you find the missing rate of the label is very high or the fraud rate consistently dropped during the most recent dates, it might be an indicator of labels not fully matured. You should exclude the most recent data or wait longer to collect the accurate labels, and then retrain your model.
If you observe a sharp spike of fraud rate and variables on specific dates, you might want to double-check if it is an outlier or data collection issue. In that case, you should delete those events and retrain the model.
If you find the outdated data can’t represent your current and future business, you should exclude the old period of data from training. If you’re using stored events in Amazon Fraud Detector, you can simply retrain a new version and select the proper date range while configuring the training job. That may also indicate that the fraud modus operandi in your business changes relatively quickly over time. After model deployment, you may need to re-train your model frequently.
Improper variable type mapping
Amazon Fraud Detector enriches and transforms the data based on the variable types. It’s important that you map your variables to the correct type so that Amazon Fraud Detector model can take the maximum value of your data. For example, if you map IP
to the CATEGORICAL
type instead of IP_ADDRESS
, you don’t get the IP-
related enrichments in the backend.
In general, Amazon Fraud Detector suggests the following actions:
- Map your variables to specific types, such as
IP_ADDRESS
, EMAIL_ADDRESS
, CARD_BIN
, and PHONE_NUMBER
, so that Amazon Fraud Detector can extract and enrich additional information.
- If you can’t find the specific variable type, map it to one of the three generic types:
NUMERIC
, CATEGORICAL
, or FREE_FORM_TEXT
.
- If a variable is in text form and has high cardinality, such as a customer review or product description, you should map it to the
FREE_FORM_TEXT
variable type so that Amazon Fraud Detector extracts text features and embeddings on the backend for you. For example, if you map url_string
to FREE_FORM_TEXT
, it’s able to tokenize the URL and extract information to feed into the downstream model, which will help it learn more hidden patterns from the URL.
If you find any of your variable types are mapped incorrectly in variable configuration, you can change your variable type and then retrain the model.
Insufficient data or features
Amazon Fraud Detector requires at least 10,000 records to train an Online Fraud Insights (OFI) or Transaction Fraud Insights (TFI) model, with at least 400 of those records identified as fraudulent. TFI also requires that both fraudulent records and legitimate records come from at least 100 different entities each to ensure the diversity of the dataset. Additionally, Amazon Fraud Detector requires the modeling data to have at least two variables. Those are the minimum data requirements to build a useful Amazon Fraud Detector model. However, using more records and variables usually helps the ML models better learn the underlying patterns from your data. When you observe a low AUC or can’t find thresholds that meet your business requirement, you should consider retraining your model with more data or add new features to your model. Usually, we find EMAIL_ADDRESS
, IP
, PAYMENT_TYPE
, BILLING_ADDRESS
, SHIPPING_ADDRESS
, and DEVICE
related variables are important in fraud detection.
Another possible cause is that some of your variables contain too many missing values. To see if that is happening, check the model training messages and refer to Troubleshoot training data issues for suggestions.
Common issues for very high model performance
In this section, we discuss common issues related to very high model performance.
Label leakage
Label leakage occurs when the training datasets use information that would not be expected to be available at prediction time. It overestimates the model’s utility when run in a production environment.
High AUC (close to 1), perfectly separated score distribution, and significantly higher variable importance of one variable could be indicators of potential label leakage issues. You can also check the correlation between the features and the label using the Data Profiler. The Feature and label correlation plot shows the correlation between each feature and the label. If one feature has over 0.99 correlation with the label, you should check if the feature is used properly based on business judgments. For example, to build a risk model to approve or decline a loan application, you shouldn’t use the features like AMOUNT_PAID
, because the payments happen after the underwriting process. If a variable isn’t available at the time you make prediction, you should remove that variable from model configuration and retrain a new model.
The following example shows the correlation between each variable and label. investigation_status
has a high correlation (close to 1) with the label, so you should double-check if there is a label leakage issue.
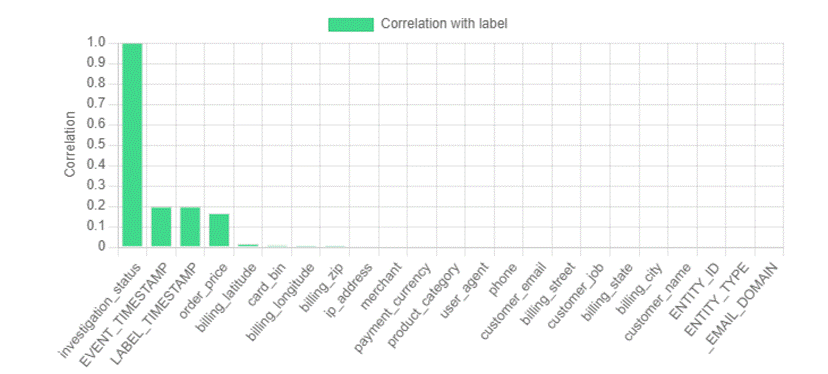
Simple fraud patterns
When the fraud patterns in your data are simple, you might also observe very high model performance. For example, suppose all the fraud events in the modeling data come through the same Internal Service Provider; it’s straightforward for the model to pick the IP-
related variables and return a “perfect” model with high importance of IP
.
Simple fraud patterns don’t always indicate a data issue. It could be true that the fraud modus operandi in your business is easy to capture. However, before making a conclusion, you need to make sure the labels used in model training are accurate, and the modeling data covers as many fraud patterns as possible. For example, if you label your fraud events based on rules, such as labeling all applications from a specific BILLING_ZIP
plus PRODUCT_CATEGORY
as fraud, the model can easily catch those frauds by simulating the rules and achieving a high AUC.
You can check the label distribution across different categories or bins of each feature using the Data Profiler. For example, if you observe that most fraud events come from one or a few product categories, it might be an indicator of simple fraud patterns, and you need to confirm that it’s not a data collection or process mistake. If the feature is like CUSTOMER_ID
, you should exclude the feature in model training.
The following example shows label distribution across different categories of product_category
. All fraud comes from two product categories.
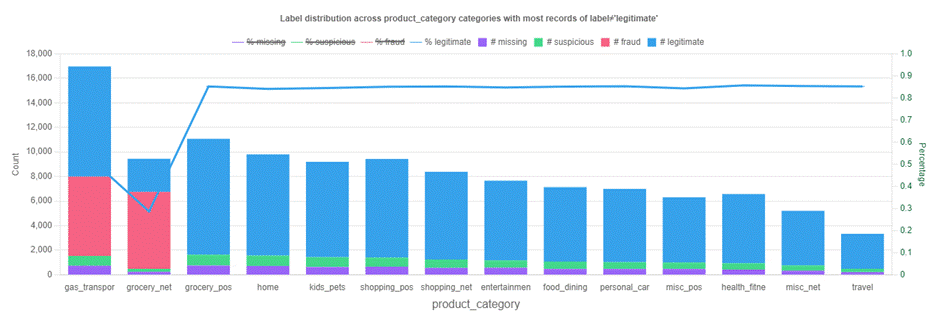
Improper data sampling
Improper data sampling may happen when you sampled and only sent part of your data to Amazon Fraud Detector. If the data isn’t sampled properly and isn’t representative of the traffic in production, the reported model performance will be inaccurate and the model could be useless for production prediction. For example, if all fraud events in the modeling data are sampled from Asia and all legit events are sampled from the US, the model might learn to separate fraud and legit based on BILLING_COUNTRY
. In that case, the model is not generic to be applied to other populations.
Usually, we suggest sending all the latest events without sampling. Based on the data size and fraud rate, Amazon Fraud Detector does sampling before model training for you. If your data is too large (over 100 GB) and you decide to sample and send only a subset, you should randomly sample your data and make sure the sample is representative of the entire population. For TFI, you should sample your data by entity, which means if one entity is sampled, you should include all its history so that the entity level aggregates are calculated correctly. Note that if you only send a subset of data to Amazon Fraud Detector, the real-time aggregates during inference might be inaccurate if the previous events of the entities aren’t sent.
Another improper data sampling could be only using a short period of data, like one day’s data, to build the model. The data might be biased, especially if your business or fraud attacks have seasonality. We usually recommend including at least two cycles’ (such as 2 weeks or 2 months) worth of data in the modeling to ensure the diversity of fraud types.
Conclusion
After diagnosing and resolving all the potential issues, you should get a useful Amazon Fraud Detector model and be confident about its performance. For the next step, you can create a detector with the model and your business rules, and be ready to deploy it to production for a shadow mode evaluation.
Appendix
How to exclude variables for model training
After the deep dive, you might identify a variable leak target information, and want to exclude it from model training. You can retrain a model version excluding the variables you don’t want by completing the following steps:
- On the Amazon Fraud Detector console, in the navigation pane, choose Models.
- On the Models page, choose the model you want to retrain.
- On the Actions menu, choose Train new version.
- Select the date range you want to use and choose Next.
- On the Configure training page, deselect the variable you don’t want to use in model training.
- Specify your fraud labels and legitimate labels and how you want Amazon Fraud Detector to use unlabeled events, then choose Next.
- Review the model configuration and choose Create and train model.
How to change event variable type
Variables represent data elements used in fraud prevention. In Amazon Fraud Detector, all variables are global and are shared across all events and models, which means one variable could be used in multiple events. For example, IP could be associated with sign-in events, and it could also be associated with transaction events. Naturally, Amazon Fraud Detector locked the variable type and data type once a variable is created. To delete an existing variable, you need to first delete all associated event types and models. You can check the resources associated with the specific variable by navigating to Amazon Fraud Detector, choosing Variables in the navigation pane, and choosing the variable name and Associated resources.
Delete the variable and all associated event types
To delete the variable, complete the following steps:
- On the Amazon Fraud Detector console, in the navigation pane, choose Variables.
- Choose the variable you want to delete.
- Choose Associated resources to view a list of all the event types used this variable.
You need to delete those associated event types before deleting the variable.
- Choose the event types in the list to go to the associated event type page.
- Choose Stored events to check if any data is stored under this event type.
- If there are events stored in Amazon Fraud Detector, choose Delete stored events to delete the stored events.
When the delete job is complete, the message “The stored events for this event type were successfully deleted” appears.
- Choose Associated resources.
If detectors and models are associated with this event type, you need to delete those resources first.
- If detectors are associated, complete the following steps to delete all associated detectors:
- Choose the detector to go to the Detector details page.
- In the Model versions pane, choose the detector’s version.
- On the detector version page, choose Actions.
- If the detector version is active, choose Deactivate, choose Deactivate this detector version without replacing it with a different version, and choose Deactivate detector version.
- After the detector version is deactivated, choose Actions and then Delete.
- Repeat these steps to delete all detector versions.
- On the Detector details page, choose Associated rules.
- Choose the rule to delete.
- Choose Actions and Delete rule version.
- Enter the rule name to confirm and choose Delete version.
- Repeat these steps to delete all associated rules.
- After all detector versions and associated rules are deleted, go to the Detector details page, choose Actions, and choose Delete detector.
- Enter the detector’s name and choose Delete detector.
- Repeat these steps to delete the next detector.
- If any models are associated with the event type, complete the following steps to delete them:
- Choose the name of the model.
- In the Model versions pane, choose the version.
- If the model status is
Active
, choose Actions and Undeploy model version.
- Enter
undeploy
to confirm and choose Undeploy model version.
The status changes to Undeploying
. The process takes a few minutes to complete.
- After the status becomes
Ready to deploy
, choose Actions and Delete.
- Repeat these steps to delete all model versions.
- On the Model details page, choose Actions and Delete model.
- Enter the name of the model and choose Delete model.
- Repeat these steps to delete the next model.
- After all associated detectors and models are deleted, choose Actions and Delete event type on the Event details page.
- Enter the name of the event type and choose Delete event type.
- In the navigation pane, choose Variables, and choose the variable you want to delete.
- Repeat the earlier steps to delete all event types associated with the variable.
- On the Variable details page, choose Actions and Delete.
- Enter the name of the variable and choose Delete variable.
Create a new variable with the correct variable type
After you have deleted the variable and all associated event types, stored events, models, and detectors from Amazon Fraud Detector, you can create a new variable of the same name and map it to the correct variable type.
- On the Amazon Fraud Detector console, in the navigation pane, choose Variables.
- Choose Create.
- Enter the variable name you want to modify (the one you deleted earlier).
- Select the correct variable type you want to change to.
- Choose Create variable.
Upload data and retrain the model
After you update the variable type, you can upload the data again and train a new model. For instructions, refer to Detect online transaction fraud with new Amazon Fraud Detector features.
How to add new variables to an existing event type
To add new variables to the existing event type, complete the following steps:
- Add the new variables to the previous training CVS file.
- Upload the new training data file to an S3 bucket. Note the Amazon S3 location of your training file (for example,
s3://bucketname/path/to/some/object.csv
) and your role name.
- On the Amazon Fraud Detector console, in the navigation pane, choose Events.
- On the Event types page, choose the name of the event type you want to add variables.
- On the Event type details page, choose Actions, then Add variables.
- Under Choose how to define this event’s variables, choose Select variables from a training dataset.
- For IAM role, select an existing IAM role or create a new role to access data in Amazon S3.
- For Data location, enter the S3 location of the new training file and choose Upload.
The new variables not present in the existing event type should show up in the list.
- Choose Add variables.
Now, the new variables have been added to the existing event type. If you’re using stored events in Amazon Fraud Detector, the new variables of the stored events are still missing. You need to import the training data with the new variables to Amazon Fraud Detector and then retrain a new model version. When uploading the new training data with the same EVENT_ID
and EVENT_TIMESTAMP
, the new event variables overwrite the previous event variables stored in Amazon Fraud Detector.
About the Authors
Julia Xu is a Research Scientist with Amazon Fraud Detector. She is passionate about solving customer challenges using Machine Learning techniques. In her free time, she enjoys hiking, painting, and exploring new coffee shops.
Hao Zhou is a Research Scientist with Amazon Fraud Detector. He holds a PhD in electrical engineering from Northwestern University, USA. He is passionate about applying machine learning techniques to combat fraud and abuse.
Abhishek Ravi is a Senior Product Manager with Amazon Fraud Detector. He is passionate about leveraging technical capabilities to build products that delight customers.
Read More