Identifying descriptions of events that did not take place in product reviews improves product retrieval results.Read More
Next Gen Stats Decision Guide: Predicting fourth-down conversion
It is fourth-and-one on the Texans’ 36-yard line with 3:21 remaining on the clock in a tie game. Should the Colts’ head coach Frank Reich send out kicker Rodrigo Blankenship to attempt a 54-yard field goal or rely on his offense to convert a first down? Frank chose to go for it, leading to a first-down conversion and an eventual touchdown to seal the win. Was this the optimal call or a gamble that ended up working? Through a collaboration between the NFL’s Next Gen Stats team and AWS, NFL fans can now get an answer to this question.
Like the Colts-Texans example, the decision of what to do on a fourth down late in the game can be the difference between a win and a loss. While it can be tempting to focus on fourth-downs late in the game, even fourth-down decisions that occur early in the game can be important. Fourth-down decisions early in the game can have reverberating effects that compound over the course of a game or season. Head coaches who consistently make the right call on the fourth down put their teams in the best possible position to win, but how does a coach know what the right call is? What factors do they have to weigh, and how can a computer give fans insights into this complicated decision-making process?
The problem can be represented as a tree of choices and their respective potential outcomes. On any fourth down, a team has three main options: punt, kick a field goal, or go for it. If a team punts, their opponent generally gains possession of the ball at some point farther down the field. On a field goal attempt, the two main outcomes are the offensive team either makes the field goal or misses the field goal. If they make the field goal, they gain three points. If they miss the field goal, the defense gains possession of the ball at the location of the attempt. Similarly, if a team chooses to go for it, there are two main outcomes. Either the team gains enough yards for a first-down (or potentially a touchdown), or the defense gains possession of the ball at the end of the play.
When coaches decide what to do on a fourth-down, they must weigh all the potential outcomes and the impact of these outcomes on the odds of winning the game. To help fans understand a coach’s decision, the NFL and AWS partnered to create the Next Gen Stats Decision Guide. The Next Gen Stats Decision Guide is a suite of machine learning (ML) models designed to determine the optimal fourth-down call. The decision guide does this by predicting the odds of each potential fourth-down outcome and the resulting odds of winning the game. By comparing the odds of winning the game for each fourth-down choice, the Next Gen Stats Decision Guide provides a data-driven answer to that optimal fourth-down call.
Going back to Frank Reich’s decision, the Colts needed 0.25 yards to gain a first down. What is the probability that they convert? As shown in the following figure, our fourth-down conversion probability model predicts an 81% chance. When paired with the updated win probability of 75% if they convert, we get an expected win probability of 69%. However, if they choose to kick a field goal, the chance of making the field goal is around 42%. Paired with the win probability of 71% if successful, we get an expected win probability of 56%. Based on these expected probabilities, the Next Gen Stats Decision Guide recommends going for it with a 13% difference.
In addition to fourth-down decisions, coaches must decide what to do after scoring a touchdown. The team can kick an extra point (+1 point) or elect to attempt a two-point conversion (+2 points). The application of the Next Gen Stats Decision Guide to fourth-down plays and after-touchdown plays has been presented before, and is a good primer for this discussion. In this post, we focus on the models that determine the probability of converting a fourth-down conversion. We share how we feature engineered and developed the ML model and metrics that were used to evaluate the quality of predictions.
Go-for-it model
If a team chooses to go for it on a fourth-down, the team must gain enough yards to make a first-down on that single play. This means that not all fourth-downs are equal. Some require the offense to gain less than a yard, while others may occasionally require the offense to gain more than 10 yards. The location on the field, time left on the clock, and relative strengths of the teams are among the important parameters in understanding the odds of success. In building the Go-for-it model, we examine these and other factors to determine which features are most important in constructing a performant model.
Problem formulation
The odds of converting on a fourth-down can be formulated as a multi-class classifier. In this formulation, each class represents the offense gaining some number of yards on the play. The probability of each class is used as the odds that the team will gain that number of yards on the play. The following histogram shows the yards gained on third- and fourth-down plays from 2016–2020. An initial approach might be to make each class in the model represent an integer number of yards gained, but the histogram shows that this approach will be difficult. Classes in the long tail of the graph (roughly 40–100 yards) occur infrequently, and this sort of class imbalance can be difficult account for in model training.
To combat the potential class imbalance, we used an unequal distribution of yards to classes. Instead of each yard gained being an individual class, we used 17 different classes to encompass all the potential outcomes shown in in the graph.
As shown in the following table, we use one class for all negative or zero-yards-gained results. Between 1–15 yards gained, we use one class for each potential outcome. The reason for this breakdown is that 88% of fourth-down plays have somewhere between 1–15 yards to go. This enables the model to capture a large majority of fourth-down situations with high fidelity. To address plays with more than 15 yards to go, we employ a decay factor to represent the decreasing probability of getting more yards on a single play.
Yards | Model Classes (17) |
Less than or equal to 0 | 0 |
1–15 yards | 1–15 (15 classes) |
16+ yards | 16 |
The following equation shows the decay factor used where the probability of converting ( Pconversion ) is the probability of getting 16 or more yards () divided by the actual distance needed for a first down (d ) minus 15 yards.
Features
Just as a coach needs to consider many factors when deciding what to do in a game, the conversion probability models also have many potential features to use. Part of the modeling process involved determining which features to incorporate into the model. We used feature importance measures like correlation to help us identify several high-value features (see the following table). These features include the actual yards-to-go, the Vegas spread, and the historical aggregations of expected points added (EPA) by team and quarterback.
The actual yards-to-go is arguably the most important feature for this model, aligning with general football knowledge. The more yards a team needs to gain, the less likely the team is to achieve that outcome. What makes the actual yards-to-go metric even more valuable in this model is that it is derived from the NGS tracking data. Traditional NFL datasets often represent the yards-to-go as an integer, which obscures the variable nature of the game. With the NGS tracking data, we can get a measurement of the football’s location with sub-foot accuracy. This allows our model to understand the difference between fourth and inches versus fourth and 1 yard.
Although the actual yards-to-go is a clear metric to provide the model, some information is harder to quantify immediately and provide to the model. For example, a coach understands the unique skillsets of their team and the opposition, both on that day and historically. To assess coaching decisions, the model needs a way to use similar information. The Vegas lines are a useful condensation of vast amounts of situational and historical knowledge about the teams into a small set of numbers. Specifically, the point spread and the total points lines capture information about prevailing beliefs regarding the relative strengths of the teams, and the model found these values useful.
Input Features | Description |
actualYardsToGo | The yards to go as measured using NGS tracking data between the ball at snap and the yards-to-go marker |
isCalledPass | Is the play predicted to be a pass or a rush? |
totalLine | The closing spread line for the game |
possessionTeamLine | The number of points the possession team is favored by according to Vegas |
possessionTeamTotal | The number of total points the possession team is expected to score as indicated by the Vegas total and spread lines |
offEpa | A team offense’s average expected points added per play over the last X number of plays in similar situations |
defEpa | A team defense’s average expected points added allowed per play over the last X number of plays in similar situations |
qbEpa | A team offense’s average expected points added per play over the last X number of plays when the quarterback on the field attempted a pass, run, or was sacked |
qbSuccessEpa | Quarterback success EPA for the last N similar plays |
Similar to how the Vegas lines provide game-level insight into relative team strengths, we can use EPA values to provide insight into relative team strengths at a more granular level. These EPA values, calculated using other NGS models, provide insight into how the team has performed in similar situations in the past. The EPA models can be broken down by the offense, defense, and quarterback. This provides the model with information about how successful the respective teams have been in the past in addition to how successful the current quarterback has been. The following figure shows the relative importance of the features after HPO. As discussed earlier, this feature importance makes intuitive sense.
Model training
To train the model, we used all the data from third- and fourth-down plays from 2016–2019 regular seasons as the training set. We held out the data from 2020 for the testing set.
For model architecture, a handful of different models were compared, including XGBoost, PyTorch Tabular, and AutoML-based models. Of these options, the XGBoost model provided the best results. It is also explained by using the Shapely Additive Explanations (SHAP) feature importance measures. Because our goal is to optimize for conversion probabilities, we used the Brier score (probabilistic loss function) to measure the performance of our models. The Brier score measures the mean squared difference between predicted probability assigned to the possible outcomes and actual outcomes. A lower Brier score is considered better.
To optimize our models, we used Amazon SageMaker hyperparameter optimization (HPO) to fine-tune XGBoost parameters like learning rate, max depth, subsamples, alpha, and gamma. The SageMaker-managed HPO service helped us run multiple experiments in parallel to identify optimal hyperparameter configurations. Each experiment took only a few minutes because tuning jobs are distributed across 10 instances. In addition, we used SageMaker features, including automatic early stopping and warm starting from previous tuning jobs. This combined with custom metrics improved the performance of the model within minutes. Examples of various SageMaker-based HPO tuning jobs are available on GitHub.
Go-for-it model results
After training and HPO, the XGBoost model achieved a Brier score of 0.21. In addition to the Brier score, we examined the model predictions to ensure they were recreating known aspects of the game. For example, the odds of converting on a fourth-down play decrease as the number of yards needed for a first-down increase. The following figure shows the model’s predicted conversion probabilities as a function of the yards-to-go. We can observe two key trends. First, as expected, the conversion probability decreases as the yards-to-go increases. Second, a team is generally better off running the ball on short yards-to-go situations and passing the ball on long yards-to-go situations.
For the Next Gen Stats Decision Guide, it’s not sufficient for the model to make correct predictions. It must also assign valid probabilities to those predictions. To examine the validity of the model probabilities, we compare the probabilities against the aggregate play outcomes, as shown in the following graph. The model predictions were binned into 10%-wide categories from 0–90%. For each bin, the fraction of plays that were converted was calculated (bar height). For an ideal model, the bin heights should be roughly the midpoint of each bin (solid line). The following graph shows that when the model provides a conversion probability between 0–60%, the actual aggregate outcomes of these plays closely match the model’s predictions. For model predictions between 60–90%, the model slightly appears to underestimate the offense’s probabilities of converting (most notably between 60–70%). In situations where the agreement is poor, we can use postprocessing techniques to increase the agreement between play outcomes and the model probabilities. For an example for deep learning models, see Quantifying uncertainty in deep learning systems.
ML production pipeline
For the model in production, we used SageMaker for preprocessing, training, and postprocessing. The model is hosted using a highly scalable, available, and secured Amazon Elastic Kubernetes Service (Amazon EKS) for production usage. The following figure shows a high-level diagram of the production pipeline. All steps are automated and require minimal maintenance.
Summary
AWS and the NFL NGS team jointly developed the Next Gen Stats Decision Guide, which helps fans understand the choices coaches make at pivotal moments in the game. The odds of converting on a fourth-down play are a key component of the Next Gen Stats Decision Guide. In this post, we provided insight into how AWS helped the NFL create the model powering fourth-down conversions and discussed methods to assess model performance.
The NGS team will be hosting these models as part of the 2021 NFL season. Keep an eye out for the Next Gen Stats Decision Guide during the next NFL game.
You can find full examples of creating custom training jobs, implementing HPO, and deploying models on SageMaker at the AWS Labs GitHub repo. If you would like us to help and accelerate your use of ML, contact the Amazon ML Solutions Lab program.
About the Authors
Selvan Senthivel is a Senior ML Engineer with Amazon ML Solutions Lab team at AWS, focusing on helping customers on Machine Learning, Deep Learning problems and end-to-end ML solutions. He was the founding engineering lead of Amazon Comprehend Medical service and contributed to the design/architecture of multiple AWS AI services.
Lin Lee Cheong is a Senior Scientist and Manager with the Amazon ML Solutions Lab team at Amazon Web Services. She works with strategic AWS customers to explore and apply artificial intelligence and machine learning to discover new insights and solve complex problems.
Tyler Mullenbach is a Principal Data Science Manager with AWS Professional Services. He leads a global team of data science consultants focusing on helping customers turn their data into insights and bring ML models to production.
Ankit Tyagi is a Senior Software Engineer with the NFL’s Next Gen Stats team. He focuses on backend data pipelines and machine learning for delivering stats to fans. Outside of work, you can find him playing tennis, experimenting with brewing beer, or playing guitar.
Mike Band is the Lead Analyst for NFL’s Next Gen Stats. He contributes to the ideation, development, and communication of advanced football performance metrics for the NFL Media Group, NFL Broadcast Partners, and fans.
Juyoung Lee is a Senior Software Engineer with the NFL’s Next Gen Stats. Her work focuses on designing and developing machine learning models to create stats for fans. On her spare time, she enjoys being active by playing Ultimate Frisbee and doing CrossFit.
Michael Schaefer was the Director of Product and Analytics for NFL’s Next Gen Stats. His work focuses on the design and execution of statistics, applications, and content delivered to NFL Media, NFL Broadcaster Partners, and fans.
Michael Chi is the Director of Technology for NFL’s Next Gen Stats. He is responsible for all technical aspects of the platform which is used by all 32 clubs, NFL Media and Broadcast Partners. In his free time, he enjoys being outdoors and spending time with his family.
Chain custom Amazon SageMaker Ground Truth jobs for image processing
Amazon SageMaker Ground Truth supports many different types of labeling jobs, including several image-based labeling workflows like image-level labels, bounding box-specific labels, or pixel-level labeling. For situations not covered by these standard approaches, Ground Truth also supports custom image-based labeling, which allows you to create a labeling workflow with a completely unique UI and associated processing. Beyond that, you can chain different Ground Truth labeling jobs together so that the output of one job acts as the input to another job, to allow even more flexibility in a labeling workflow by breaking the job into multiple stages.
In this post, we show how to chain two custom Ground Truth jobs together to perform advanced image manipulations, including isolating portions of images, and de-skewing images that were photographed from an angle. Additionally, we demonstrate several techniques for augmenting source images, which are helpful for situations where you have a limited number of source images.
Extracting regions of an image
Suppose we’re tasked with creating a machine learning (ML) model that processes an image of a shelving unit and determines whether any of the bins in that shelving unit need restocking. Due to the size of the storage room, a single camera is used to capture images of several shelving units, each from a different angle. The following image is an example of such a shelving unit.
For training or inference, we need images of individual bins, rather than the overall shelving unit. The model we’re developing takes an image of a single bin, and return a classification of Empty or Full. This classification feeds into an automated restocking system, allowing us to maintain stock levels at the bin level without the trouble of someone physically checking the levels.
Unfortunately, because the shelf images are taken at an angle, each bin is skewed and has a different size and shape. Because any bin images extracted from the main image are rectangular, the extracted images include undesirable content, as shown in the following image of two adjoining bins.
In this example, we’ve isolated a rectangular region that bounds a given bin, but because the image was taken from an angle, portions of the bins on the left and right are also partially included. Because a rectangular section includes information from other bins, an image like this performs poorly when used for training or for inference.
To solve this, we can select a non-rectangular section of the original image and warp it to create a new image. The following image demonstrates the results of a warp transformation applied to the original image.
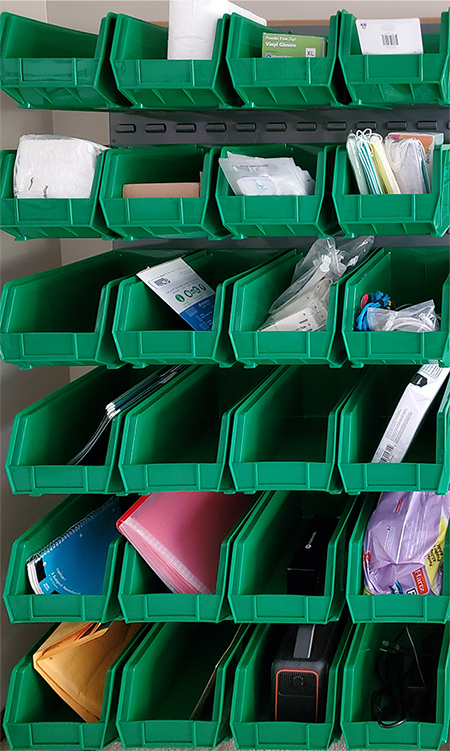
Figure 3: Original shelving unit with just the bins isolated, and the image warped to make it orthogonal
This warping accomplishes two tasks. First, we’ve selected just the shelving unit, cropping out the nearby walls, floor, and any other irrelevant areas near the edges of the shelves. Second, the warping of the image results in each bin being more rectangular than the original version.
This warped image doesn’t have any new content—it’s just a distortion of the original image. But by performing this warping, each bin can be selected using a rectangular bounding box, which provides needed consistency, no matter what position a bin is in. Compare the following two bin images: the image on the left is extracted from the original image, and the image on the right is the same bin, extracted from the de-skewed image.
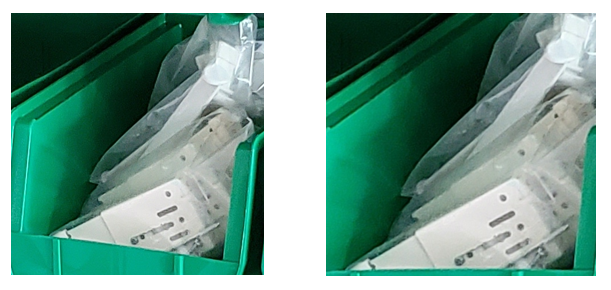
Figure 4: A single bin from the original image (left) compared with the bin from the warped image (right)
The bottom opening of the bin was originally at an angle, and now it’s horizontal. Overall, we’ve reduced the amount of the bin shown, and increased the proportion of the contents of the bin within the image. This improves our ML training process, because each bin image has less superfluous content.
Ground Truth jobs
Each custom Ground Truth labeling job is defined with a web-based user interface and two associated AWS Lambda functions (for more information, see Processing with AWS Lambda). One function runs prior to each image displayed by the UI, and the other runs after the user finishes the labeling job for all the images. Ground Truth offers several pre-made user interfaces (like bounding box-based selection), but you can also create your own custom UI if needed, as we do for this example.
When Ground Truth jobs are chained together, the output of one job is used as the input of another job. For this task, we use two chained jobs to process our images, as illustrated in the following diagram.
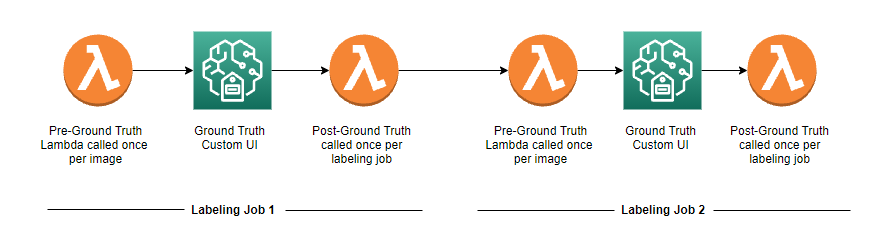
Figure 5: Architecture diagram showing two chained Ground Truth jobs, each with a Pre- and Post- UI Lambda function
Images that need to be labeled are stored in Amazon Simple Storage Solution (Amazon S3). The first Ground Truth job retrieves images from Amazon S3 and displays them one at a time, waiting for the user to specify the four corners of the shelving unit within the image, using a custom UI. When that step is complete, the post-UI Lambda function uses the corner coordinates to warp or de-skew each image, which is then saved to the same S3 bucket that the original image resides in. Note that it’s not necessary to do this during inference—for a situation where the camera is in a fixed location, you can save those corner coordinates for later use during inference.
After the first Ground Truth job has de-skewed the source image, the second job uses simple bounding boxes to label each bin within the de-skewed image. The post-UI Lambda function then extracts the individual bin images, augments them with rotations, flipping, and color and brightness alterations, and writes the resulting data to Amazon S3, where it can be used for model training or other purposes.
You can find example code and deployment instructions in the GitHub repo.
Custom user interface
From a labeler’s perspective, after they log in and select a job, they use the custom UI to select the four corners of a bin.
For custom Ground Truth user interfaces, a set of custom tags is available, known as Crowd tags. These tags include bounding boxes, lines, points, and other user interface elements that you can use to build a labeling UI. In this case, we use the crowd-polygon
tag, which is displayed as a yellow polygon.
After the labeler draws a polygon with four corners on the UI for all source images, they exit the UI by choosing Done. At this point, the post-UI Lambda function is run and each de-skewed image is saved to Amazon S3. When the function is complete, control is passed to the next chained Ground Truth job.
Generally, chained Ground Truth jobs reuse an output manifest file as the input manifest file for the next (chained) labeling job. In this case, we created a new image, so we modify the pre-UI Lambda function so it passes in the correct (de-skewed) file name, rather than the original, skewed image file name.
The second job in the chain uses the bounding box-based labeling functionality that is built in to Ground Truth. The bounding boxes don’t cover the entire contents of each bin, but they do cover the openings of the bins. This provides enough data to create a model to detect whether a bin is full or empty.
After the labeler selects all the bins, they exit the UI by choosing Done. At this point, the post-UI Lambda function runs and crops out each bin image, makes variations of it for image augmentation purposes, and saves the variations into a folder structure in Amazon S3 based on classification. The top level of the folder structure is named training_data
, with two subfolders: empty
and full
. Each subfolder contains images of bins that are either empty or full, suitable for use in model training.
Image augmentation
Image augmentation is a technique sometimes used in image-based ML workloads. It’s especially helpful when the number of source images is low, or limited in the number of variants. Typically, image augmentation is performed by taking a source image and creating multiple variants of it, altering factors like brightness and contrast, coloring, and even cropping or rotating images. These variations help the resulting model be more robust and capable of handling images that are dissimilar to the original training images.
In this example, we use image augmentation methods in the post-UI Lambda function of the second Ground Truth job. The labeler has specified the bounding boxes for each bin image in the Ground Truth UI, and that data is used to extract portions of the overall image. Those extracted portions are of the individual bins, and these smaller images are used as input into our image augmentation process.
In our case, we create 14 variants of each bin image, with variations of brightness, contrast, and sharpness, as well horizontal flipping combined with these variations. With this approach, a single source image of a shelving unit with 24 bins generates 14 variants for each bin image, for a total of 336 images that can be used for training a model. The following shows an original bin image (upper left) and each of its variants.
Conclusion
Custom Ground Truth jobs provide a great deal of flexibility, and using them with images allows advanced functionality like cropping and de-skewing images, as well as performing custom image augmentation. The supplied Crowd HTML tags support many different labeling approaches like polygons, lines, text boxes, modal alerts, key point placement, and others. Combined with the power of pre-UI and post-UI Lambda functions, a custom Ground Truth job allows you to construct complex labeling jobs to support a wide variety of use cases, and combining different custom jobs by chaining them together provides even more options.
You can use the GitHub repo associated with this post as a starting point for your own chained image labeling jobs. You can also extend the code to support additional image augmentation methods (like cropping or rotating the source images), or modify it to fit your particular use case.
To learn more about chained Ground Truth jobs, see Chaining Labeling Jobs.
For more information about the Crowd tags you can use in the Ground Truth UI, see Crowd HTML Elements Reference.
About the Author
Greg Sommerville is a Senior Prototyping Architect on the AWS Envision Engineering Americas Prototyping team, where he helps AWS customers implement innovative solutions to challenging problems with machine learning, IoT and serverless technologies. He lives in Ann Arbor, Michigan and enjoys practicing yoga, catering to his dogs, and playing poker.
New Alexa feature enables natural, multiparty interactions
In Conversation Mode, Alexa detects device-directed speech without the need for the wake word.Read More
Accelerate data preparation using Amazon SageMaker Data Wrangler for diabetic patient readmission prediction
Patient readmission to hospital after prior visits for the same disease results in an additional burden on healthcare providers, the health system, and patients. Machine learning (ML) models, if built and trained properly, can help understand reasons for readmission, and predict readmission accurately. ML could allow providers to create better treatment plans and care, which would translate to a reduction of both cost and mental stress for patients. However, ML is a complex technique that has been limiting organizations that don’t have the resources to recruit a team of data engineers and scientists to build ML workloads. In this post, we show you how to build an ML model based on the XGBoost algorithm to predict diabetic patient readmission easily and quickly with a graphical interface from Amazon SageMaker Data Wrangler.
Data Wrangler is an Amazon SageMaker Studio feature designed to allow you to explore and transform tabular data for ML use cases without coding. Data Wrangler is the fastest and easiest way to prepare data for ML. It gives you the ability to use a visual interface to access data and perform exploratory data analysis (EDA) and feature engineering. It also seamlessly operationalizes your data preparation steps by allowing you to export your data flow into Amazon SageMaker Pipelines, a Data Wrangler job, Python file, or Amazon SageMaker Feature Store.
Data Wrangler comes with over 300 built-in transforms and custom transformations using either Python, PySpark, or SparkSQL runtime. It also comes with built-in data analysis capabilities for charts (such as scatter plot or histogram) and time-saving model analysis capabilities such as feature importance, target leakage, and model explainability.
In this post, we explore the key capabilities of Data Wrangler using the UCI diabetic patient readmission dataset. We showcase how you can build ML data transformation steps without writing sophisticated coding, and how to create a model training, feature store, or ML pipeline with reproducibility for a diabetic patient readmission prediction use case.
We also have published a related GitHub project repo that includes the end-to-end ML workflow steps and relevant assets, including Jupyter notebooks.
We walk you through the following high-level steps:
- Studio prerequisites and input dataset setup
- Design your Data Wrangler flow file
- Create processing and training jobs for model building
- Host a trained model for real-time inference
Studio prerequisites and input dataset setup
To use Studio and Studio notebooks, you must complete the Studio onboarding process. Although you can choose from a few authentication methods, the simplest way to create a Studio domain is to follow the Quick start instructions. The Quick start uses the same default settings as the standard Studio setup. You can also choose to onboard using AWS Single Sign-On (AWS SSO) for authentication (see Onboard to Amazon SageMaker Studio Using AWS SSO).
Dataset
The patient readmission dataset captures 10 years (1999–2008) of clinical care at 130 US hospitals and integrated delivery networks. It includes over 50 features representing patient and hospital outcomes with about 100,000 observations.
You can start by downloading the public dataset and uploading it to an Amazon Simple Storage Service (Amazon S3) bucket. For demonstration purposes, we split the dataset into four tables based on feature categories: diabetic_data_hospital_visits.csv
, diabetic_data_demographic.csv
, diabetic_data_labs.csv
, and diabetic_data_medication.csv
. Review and run the code in datawrangler_workshop_pre_requisite.ipynb. If you leave everything at its default inside the notebook, the CSV files will be available in s3://sagemaker-${region}-${account_number}/sagemaker/demo-diabetic-datawrangler/
.
Design your Data Wrangler flow file
To get started – on the Studio File menu, choose New, and choose Data Wrangler Flow.
This launches a Data Wrangler instance and configures it with the Data Wrangler app. The process takes a few minutes to complete.
Load the data from Amazon S3 into Data Wrangler
To load the data into Data Wrangler, complete the following steps:
You could also import data from Amazon Athena, Amazon Redshift, or Snowflake. For more information about the currently supported import sources, see Import.
- Select the CSV files from the bucket
s3://sagemaker-${region}-${account_number}/sagemaker/demo-diabetic-datawrangler/
one at a time. - Choose Import for each file.
When the import is complete, data in an S3 bucket is available inside Data Wrangler for preprocessing.
Join the CSV files
Now that we have imported multiple CSV source dataset, let’s join them for a consolidated dataset.
- On the Data flow tab, for Data types, choose the plus sign.
- On the menu, choose Join.
- Choose the
diabetic_data_hospital_visits.csv
dataset as the Right dataset. - Choose Configure to set up the join criteria.
- For Name, enter a name for the join.
- For Join type¸ choose a join type (for this post, Inner).
- Choose the columns for Left and Right.
- Choose Apply to preview the joined dataset.
- Choose Add to add it to the data flow file.
Built-in analysis
Before we apply any transformations on the input source, let’s perform a quick analysis of the dataset. Data Wrangler provides several built-in analysis types, like histogram, scatter plot, target leakage, bias report, and quick model. For more information about analysis types, see Analyze and Visualize.
Target leakage
Target leakage occurs when information in an ML training dataset is strongly correlated with the target label, but isn’t available when the model is used for prediction. You might have a column in your dataset that serves as a proxy for the column you want to predict with your model. For classification tasks, Data Wrangler calculates the prediction quality metric of ROC-AUC, which is computed individually for each feature column via cross-validation to generate a target leakage report.
- On the Data Flow tab, for Join, choose the plus sign.
- Choose Add analysis.
- For Analysis type, choose Target Leakage.
- For Analysis name¸ enter a name.
- For Max features, enter
50
. - For Problem Type¸ choose classification.
- For Target, choose readmitted.
- Choose Preview to generate the report.
As shown in the preceding screenshot, there is no indication of target leakage in our input dataset. However, a few features like encounter_id_1
, encounter_id_0
, weight
, and payer_code
are marked as possibly redundant with 0.5 predictive ability of ROC. This means these features by themselves aren’t providing any useful information towards predicting the target. Before making the decision to drop these uninformative features, you should consider whether these could add value when used in tandem with other features. For our use case, we keep them as is and move to the next step.
- Choose Save to save the analysis into your Data Wrangler data flow file.
Bias report
AI/ML systems are only as good as the data we put into them. ML-based systems are more accessible than ever before, and with the growth of adoption throughout various industries, further questions arise surrounding fairness and how it is ensured across these ML systems. Understanding how to detect and avoid bias in ML models is imperative and complex. With the built-in bias report in Data Wrangler, data scientists can quickly detect bias during the data preparation stage of the ML workflow. Bias report analysis uses Amazon SageMaker Clarify to perform bias analysis.
To generate a bias report, you must specify the target column that you want to predict and a facet or column that you want to inspect for potential biases. For example, we can generate a bias report on the gender
feature for Female
values to see whether there is any class imbalance.
- On the Analysis tab, choose Create new analysis.
- For Analysis type¸ choose Bias Report.
- For Analysis name, enter a name.
- For Select the column your model predicts, choose readmitted.
- For Predicted value, enter
NO
. - For Column to analyze for bias, choose gender.
- For Column value to analyze for bias, choose Female.
- Leave remaining settings at their default.
- Choose Check for bias to generate the bias report.
As shown in the bias report, there is no significant bias in our input dataset, which means the dataset has a fair amount of representation by gender. For our dataset, we can move forward with a hypothesis that there is no inherent bias in our dataset. However, based on your use case and dataset, you might want to run similar bias reporting on other features of your dataset to identify any potential bias. If any bias is detected, you can consider applying a suitable transformation to address that bias.
- Choose Save to add this report to the data flow file.
Histogram
In this section, we use a histogram to gain insights into the target label patterns inside our input dataset.
- On the Analysis tab, choose Create new analysis.
- For Analysis type¸ choose Histogram.
- For Analysis name¸ enter a name.
- For X axis, choose readmitted.
- For Color by, choose race.
- For Facet by, choose gender.
- Choose Preview to generate a histogram.
This ML problem is a multi-class classification problem. However, we can observe a major target class imbalance between patients readmitted <30 days, >30 days, and NO readmission. We can also see that these two classifications are proportionate across gender and race. To improve our potential model predictability, we can merge <30 and >30 into a single positive class. This merge of target label classification turns our ML problem into a binary classification. As we demonstrate in the next section, we can do this easily by adding respective transformations.
Transformations
When it comes to training an ML model for structured or tabular data, decision tree-based algorithms are considered best in class. This is due to their inherent technique of applying ensemble tree methods in order to boost weak learners using the gradient descent architecture.
For our medical source dataset, we use the SageMaker built-in XGBoost algorithm because it’s one of the most popular decision tree-based ensemble ML algorithms. The XGBoost algorithm can only accept numerical values as input, therefore as a prerequisite we must apply categorical feature transformations on our source dataset.
Data Wrangler comes with over 300 built-in transforms, which require no coding. Let’s use built-in transforms to apply a few key transformations and prepare our training dataset.
Handle missing values
To address missing values, complete the following steps:
- Switch to Data tab to bring up all the built-in transforms
- Expand Handle missing in the list of transforms.
- For Transform, choose Impute.
- For Column type¸ choose Numeric.
- For Input column, choose diag_1.
- For Imputing strategy, choose Mean.
- By default, the operation is performed in-place, but you can provide an optional Output column name, which creates a new column with imputed values. For our blog we go with default in-place update.
- Choose Preview to preview the results.
- Choose Add to include this transformation step into the data flow file.
- Repeat these steps for the
diag_2
anddiag_3
features and impute missing values.
Search and edit features with special characters
Because our source dataset has features with special characters, we need to clean them before training. Let’s use the search and edit transform.
- Expand Search and edit in the list of transforms.
- For Transform, choose Find and replace substring.
- For Input column, choose race.
- For Pattern, enter
?
. - For Replacement string¸ choose Other.
- Leave Output column blank for in-place replacements.
- Choose Preview.
- Choose Add to add the transform to your data flow.
- Repeat the same steps for other features to replace
weight
andpayer_code
with0
andmedical_specialty
with Other.
One-hot encoding for categorical features
To use one-hot encoding for categorical features, complete the following steps:
- Expand Encode categorical in the list of transforms.
- For Transform, choose One-hot encode.
- For Input column, choose race.
- For Output style, choose Columns.
- Choose Preview.
- Choose Add to add the change to the data flow.
- Repeat these steps for age and
medical_specialty_filler
to one-hot encode those categorical features as well.
Ordinal encoding for categorical features
To use ordinal encoding for categorical features, complete the following steps:
- Expand Encode categorical in the list of transforms.
- For Transform, choose Ordinal encode.
- For Input column, choose gender.
- For Invalid handling strategy, choose Keep.
- Choose Preview.
- Choose Add to add the change to the data flow.
Custom transformations: Add new features to your dataset
If we decide to store our transformed features in Feature Store, a prerequisite is to insert the eventTime
feature into the dataset. We can easily do that using a custom transformation.
- Expand Custom Transform in the list of transforms.
- Choose Python (Pandas) and enter the following line of code:
# Table is available as variable `df` import time df['eventTime'] = time.time()
- Choose Preview to view the results.
- Choose Add to add the change to the data flow.
Transform the target Label
The target label readmitted
has three classes: NO readmission, readmitted <30 days, and readmitted >30 days. We saw in our histogram analysis that there is a strong class imbalance because the majority of the patients didn’t readmit. We can combine the latter two classes into a positive class to denote the patients being readmitted, and turn the classification problem into a binary case instead of multi-class. Let’s use the search and edit transform to convert string values to binary values.
- Expand Search and edit in the list of transforms.
- For Transform, choose Find and replace substring.
- For Input column, choose readmitted.
- For Pattern, enter
>30|<30
. - For the Replacement string, enter
1
.
This converts all the values that have either >30 or <30 values to 1.
Let’s repeat the same steps to convert NO values to 0.
- Expand Search and edit in the list of transforms.
- For Transform, choose Find and replace substring.
- For Input column, choose readmitted.
- For Pattern, enter
NO
. - For Replacement string, enter
0
. - Choose Preview to review the converted column.
- Choose Add to add the transform to our data flow.
Now our target label readmitted is ready for ML training.
Position the target label as the first column to utilize XGBoost algorithm
Because we’re going to use the XGBoost built-in SageMaker algorithm to train the model, the algorithm assumes that the target label is in the first column. Let’s position the target label as such in order to use this algorithm.
- Expand Manage columns in the list of transforms.
- For Transform, choose Move column.
- For Move type, choose Move to start.
- For Column to move, choose readmitted.
- Choose Preview.
- Choose Add to add the change to your data flow.
Drop redundant columns
Next, we drop any redundant columns.
- Expand Manage columns in the list of transforms.
- For Transform, choose Drop column.
- For Column to drop, choose
encounter_id_0
. - Choose Preview.
- Choose Add to add the changes to the flow file.
- Repeat these steps for the other redundant columns:
patient_nbr_0
,encounter_id_1
, andpatient_nbr_1
.
At this stage, we have done a few analyses and applied a few transformations on our raw input dataset. If we choose to preserve the transformed state of the input dataset, like checkpoint, you can do so by choosing Export data. This option allows you to persist the transformed dataset to an S3 bucket.
Quick Model analysis
Now that we have applied transformations to our initial dataset, let’s explore the Quick Model analysis feature. Quick model helps you quickly evaluate the training dataset and produce importance scores for each feature. A feature importance score indicates how useful a feature is at predicting a target label. The feature importance score is between 0–1; a higher number indicates that the feature is more important to the whole dataset. Because our use case relates to the classification problem type, the quick model also generates an F1 score for the current dataset.
- Switch back to Analysis Tab and click Create new analysis to bring-up built-in analysis
- For Analysis type, choose Quick Model.
- Enter a name for your analysis.
- For Label, choose readmitted.
- Choose Preview and wait for the model to be trained and the results to appear.
The resulting quick model F1 score shows 0.618 (your generated score might be different) with the transformed dataset. Data Wrangler performs several steps to generate the F1 score, including preprocessing, training, evaluating, and finally calculating feature importance. For more details about these steps, see Quick Model.
With the quick model analysis feature, data scientists can iterate through applicable transformations until they have their desired transformed dataset that can potentially lead to better business accuracy and expectations.
- Choose Save to add the quick model analysis to the data flow.
Export options
We’re now ready to export our data flow for further processing.
- Navigate back to data flow designer by clicking Back to data flow on the top left
- On the Export tab, choose Steps to reveal the Data Wrangler flow steps.
- Choose the last step to mark it with a check.
- Choose Export step to reveal the export options.
As of this writing, you have four export options:
- Save to S3 – Save the data to an S3 bucket using a SageMaker processing job
- Pipeline – Export a Jupyter notebook that creates a SageMaker pipeline with your data flow
- Python Code – Export your data flow to Python code
- Feature Store – Export a Jupyter notebook that creates a Feature Store feature group and adds features to an offline or online feature store
- Choose Save to S3 to generate a fully implemented Jupyter notebook that creates a processing job using your data flow file.
Run processing and training jobs for model building
In this section, we show how to run processing and training jobs using the generated Jupyter notebook from Data Wrangler.
Submit a processing job
We’re now ready to submit a SageMaker processing job using our data flow file.
Run all the cells up to and including the Create Processing Job cell inside the exported notebook.
The cell Create Processing Job triggers a new SageMaker processing job by provisioning managed infrastructure and running the required Data Wrangler Docker container on that infrastructure.
You can check the status of the submitted processing job by running the next cell Job Status & S3 Output Location.
You can also check the status of the submitted processing job on the SageMaker console.
Train a model with SageMaker
Now that the data has been processed, let’s train a model using the data. The same notebook has sample steps to train a model using the SageMaker built-in XGBoost algorithm. Because our use case is a binary classification ML problem, we need to change the objective to binary:logistic
inside the sample training steps.
Now we’re ready to run our training job using the SageMaker managed infrastructure. Run the cell Start the Training Job.
You can monitor the status of the submitted training job on the SageMaker console, on the Training jobs page.
Host a trained model for real-time inference
We now use another notebook available on GitHub under the project folder hosting/Model_deployment_Steps.ipynb. This is a simple notebook with two cells: the first cell has code for deploying your model to a persistent endpoint. You need to update model_url
with your training job output S3 model artifact.
The second cell in the notebook runs inference on the sample test file under test_data/test_data_UCI_sample.csv. As you can see, we are able to generate predictions for our synthetic observations inside csv file. That concludes the ML workflow.
Clean up
After you have experimented with the steps in this post, perform the following cleanup steps to stop incurring charges:
- On the SageMaker console, under Inference in the navigation pane, choose Endpoints.
- Select your hosted endpoint.
- On the Actions menu, choose Delete.
- On the SageMaker Studio Control Panel, navigate to your SageMaker user profile.
- Under Apps, locate your Data Wrangler app and choose Delete app.
Conclusion
In this post, we explored Data Wrangler capabilities using a public medical dataset related to patient readmission and demonstrated how to perform feature transformations using built-in transforms and quick analysis. We showed how, without much coding, to generate the required steps to trigger data processing and ML training. This no-code/low-code capability of Data Wrangler accelerates training data preparation and increases data scientist agility with faster iterative data preparation. In the end, we hosted our trained model and ran inferences against synthetic test data. We encourage you to check out our GitHub repository to get hands-on practice and find new ways to improve model accuracy! To learn more about SageMaker, visit the SageMaker Development Guide.
About the Authors
Shyam Namavaram is a Senior Solutions Architect at AWS. He has over 20 years of experience architecting and building distributed, hybrid, and cloud-native applications. He passionately works with customers accelerating their AI/ML adoption by providing technical guidance and helping them innovate and build secure cloud solutions on AWS. He specializes in AI/ML, containers, and analytics technologies. Outside of work, he loves playing sports and exploring nature with trekking.
Michael Hsieh is a Senior AI/ML Specialist Solutions Architect. He works with customers to advance their ML journey with a combination of Amazon ML offerings and his ML domain knowledge. As a Seattle transplant, he loves exploring the great nature the region has to offer, such as the hiking trails, scenery kayaking in the SLU, and the sunset at the Shilshole Bay.
Use Amazon SageMaker ACK Operators to train and deploy machine learning models
AWS recently released the new Amazon SageMaker Operators for Kubernetes using the AWS Controllers for Kubernetes (ACK). ACK is a framework for building Kubernetes custom controllers, where each controller communicates with an AWS service API. These controllers allow Kubernetes users to provision AWS resources like databases or message queues simply by using the Kubernetes API. The new SageMaker ACK Operators make it easier for machine learning (ML) developers and data scientists who use Kubernetes as their control plane to train, tune, and deploy ML models in Amazon SageMaker without signing in to the SageMaker console.
Kubernetes and SageMaker
Building scalable ML workflows involves many iterative steps, including sourcing and preparing data, building ML models, training and evaluating these models, deploying them to production, and monitoring workloads after deployment.
SageMaker is a fully managed service designed and optimized specifically for managing these ML workflows. It removes the undifferentiated heavy lifting of infrastructure management and eliminates the need to invest in IT and DevOps to manage clusters for ML model building, training, and inference. Compute resources are only provisioned when requested, scaled as needed, and shut down automatically when jobs complete, thereby providing near 100% utilization. SageMaker provides many performance and cost optimizations for distributed training, spot training, automatic model tuning, inference latency, and multi-model endpoints.
Many AWS customers who have portability requirements implement a hybrid cloud approach, or implement on-premises and use Kubernetes, an open-source, general-purpose container orchestration system, to set up repeatable ML pipelines running training and inference workloads. However, to support ML workloads, these developers still need to write custom code to optimize the underlying ML infrastructure, provide high availability and reliability, provide data science productivity tools, and comply with appropriate security and regulatory requirements. Kubernetes customers therefore want to use fully managed ML services such as SageMaker for cost-optimized and managed infrastructure, but want platform and infrastructure teams to continue using Kubernetes for orchestration and managing pipelines to retain standardization and portability.
To address this need, AWS allows you to train, tune, and deploy models in SageMaker by using the new SageMaker ACK Operators, which includes a set of custom resource definitions for SageMaker resources that extends the Kubernetes API. With the SageMaker ACK Operators, you can take advantage of fully managed SageMaker infrastructure, tools, and optimizations natively from Kubernetes.
How did we get here?
In late 2019, AWS introduced the SageMaker Operators for Kubernetes to enable developers and data scientists to manage the end-to-end SageMaker training and production lifecycle using Kubernetes as the control plane. SageMaker operators were installed from the GitHub repo by downloading a YAML configuration file that configured your Kubernetes cluster with the custom resource definitions and operator controller service.
In 2020, AWS introduced ACK to facilitate a Kubernetes-native way of managing AWS Cloud resources. ACK includes a common controller runtime, a code generator, and a set of AWS service-specific controllers, one of which is the SageMaker controller.
Going forward, new functionality will be added to the SageMaker Operators for Kubernetes through the ACK project.
How does ACK work?
The following diagram illustrates how ACK works.
In this example, Alice is a Kubernetes user. She wants to run model training on SageMaker from within the Kubernetes cluster using the Kubernetes API. Alice issues a call to kubectl apply
, passing in a file that describes a Kubernetes custom resource describing her SageMaker training job. kubectl apply
passes this file, called a manifest, to the Kubernetes API server running in the Kubernetes controller node (Step 1 in the workflow diagram).
The Kubernetes API server receives the manifest with the SageMaker training job specification and determines whether Alice has permissions to create a custom resource of kind sageMaker.services.k8s.aws/TrainingJob
, and whether the custom resource is properly formatted (Step 2).
If Alice is authorized and the custom resource is valid, the Kubernetes API server writes (Step 3) the custom resource to its etcd
data store and then responds back (Step 4) to Alice that the custom resource has been created.
The SageMaker controller, which is running on a Kubernetes worker node within the context of a normal Kubernetes Pod, is notified (Step 5) that a new custom resource of kind SageMaker.services.k8s.aws/TrainingJob
has been created.
The SageMaker controller then communicates (Step 6) with the SageMaker API, calling the SageMaker CreateTrainingJob API to create the training job in AWS. After communicating with the SageMaker API, the SageMaker controller calls the Kubernetes API server to update (Step 7) the custom resource’s status with information it received from SageMaker. The SageMaker controller therefore provides the same information to the developers that they would have received using the AWS SDK. This results in a better and consistent developer experience.
Machine learning use case
For this post, we follow the SageMaker example provided in the following notebook. However, you can reuse the components in this example with your preference of SageMaker built-in or custom algorithms and your own datasets.
We use the Abalone dataset originally from the UCI data repository [1]. In the libsvm converted version, the nominal feature (male/female/infant) has been converted into a real valued feature. The age of abalone is to be predicted from eight physical measurements. This dataset is already processed and stored in Amazon Simple Storage Service (Amazon S3). We train an XGBoost model on the UCI Abalone dataset to replicate the flow in the example Jupyter notebook.
Prerequisites
For this walkthrough, you should have the following prerequisites:
- An AWS account.
An existing Amazon Elastic Kubernetes Service (Amazon EKS) cluster. It should be Kubernetes version 1.16+. For automated cluster creation using eksctl
, see Getting started with Amazon EKS – eksctl
and create your cluster with Amazon EC2 Linux managed nodes.
Install the following tools on the client machine used to access your Kubernetes cluster (you can use AWS Cloud9, a cloud-based integrated development environment (IDE) for the Kubernetes cluster setup):
- kubectl – A command line tool for working with Kubernetes clusters.
- Helm version 3.7+ – A tool for installing and managing Kubernetes applications.
- AWS Command Line Interface (AWS CLI) – A command line tool for interacting with AWS services.
- eksctl – A command line tool for working with Amazon EKS clusters that automates many individual tasks.
- yq – A command line YAML processor. (For Linux environments, use the wget plain binary installation).
Set up IAM role-based authentication for the controller Pod
IAM roles for service accounts (IRSA) allows fine-grained roles at the Kubernetes Pod level by combining an OpenID Connect (OIDC) identity provider with Kubernetes service account annotations. In this section, we associate the Amazon EKS cluster with an OIDC provider and create an AWS Identity and Access Management (IAM) role that is assumed by the ACK controller Pod via its service account to access AWS services.
Create a cluster and OIDC ID provider
Make sure you’re connected to the right cluster. Substitute the values for CLUSTER_NAME
and CLUSTER_REGION
below:
# Copyright Amazon.com, Inc. or its affiliates. All Rights Reserved.
# SPDX-License-Identifier: MIT-0
# Set the cluster name, region where the cluster exists
export CLUSTER_NAME=<CLUSTER_NAME>
export CLUSTER_REGION=<CLUSTER_REGION>
export RANDOM_VAR=$RANDOM
aws eks update-kubeconfig --name $CLUSTER_NAME --region $CLUSTER_REGION
kubectl config get-contexts
# Ensure cluster has compute
kubectl get nodes
Set up the OIDC ID provider (IdP) in AWS and associate it with your Amazon EKS cluster:
eksctl utils associate-iam-oidc-provider --cluster ${CLUSTER_NAME}
--region ${CLUSTER_REGION} --approve
Get the identity issuer URL by running the following code:
export AWS_ACCOUNT_ID=$(aws sts get-caller-identity --query "Account" --output text)
OIDC_PROVIDER_URL=$(aws eks describe-cluster --name $CLUSTER_NAME --region $CLUSTER_REGION --query "cluster.identity.oidc.issuer" --output text | cut -c9-)
Set up an IAM role
Next, let’s set up the IAM role that defines the access to the SageMaker and Application Auto Scaling services. For this, we also need to have an IAM trust policy in place, allowing the specified Kubernetes service account (for example, ack-sagemaker-controller
) to assume the IAM role.
Create a file named trust.json
and insert the following trust relationship code block required for IAM role:
printf '{
"Version": "2012-10-17",
"Statement": [
{
"Effect": "Allow",
"Principal": {
"Federated": "arn:aws:iam::'$AWS_ACCOUNT_ID':oidc-provider/'$OIDC_PROVIDER_URL'"
},
"Action": "sts:AssumeRoleWithWebIdentity",
"Condition": {
"StringEquals": {
"'$OIDC_PROVIDER_URL':aud": "sts.amazonaws.com",
"'$OIDC_PROVIDER_URL':sub": [
"system:serviceaccount:ack-system:ack-sagemaker-controller",
"system:serviceaccount:ack-system:ack-applicationautoscaling-controller"
]
}
}
}
]
}
' > ./trust.json
Updating an Application Auto Scaling Scalable Target requires additional permissions. First, create a service-linked role for Application Auto Scaling.
aws iam create-service-linked-role --aws-service-name sagemaker.application-autoscaling.amazonaws.com
Create a file named pass_role_policy.json
to create the policy required for the IAM role.
printf '{
"Version": "2012-10-17",
"Statement": [
{
"Effect": "Allow",
"Action": "iam:PassRole",
"Resource": "arn:aws:iam::'$AWS_ACCOUNT_ID':role/aws-service-role/sagemaker.application-autoscaling.amazonaws.com/AWSServiceRoleForApplicationAutoScaling_SageMakerEndpoint"
}
]
}
' > ./pass_role_policy.json
Run the following command to create a role with the trust relationship defined in trust.json
. This trust relationship is required so that Amazon EKS (via a webhook) can inject the necessary environment variables and mount volumes into the Pod that are required by the AWS SDK to assume this role.
OIDC_ROLE_NAME=ack-controller-role-$CLUSTER_NAME
aws iam create-role --role-name $OIDC_ROLE_NAME --assume-role-policy-document file://trust.json
# Attach the AmazonSageMakerFullAccess Policy to the Role. This policy provides full access to
# Amazon SageMaker. Also provides select access to related services (e.g., Application Autoscaling,
# S3, ECR, CloudWatch Logs).
aws iam attach-role-policy --role-name $OIDC_ROLE_NAME --policy-arn arn:aws:iam::aws:policy/AmazonSageMakerFullAccess
# Attach the iam:PassRole policy required for updating ApplicationAutoscaling ScalableTarget
aws iam put-role-policy --role-name $OIDC_ROLE_NAME --policy-name "iam-pass-role-policy" --policy-document file://pass_role_policy.json
export IAM_ROLE_ARN_FOR_IRSA=$(aws iam get-role --role-name $OIDC_ROLE_NAME --output text --query 'Role.Arn')
echo $IAM_ROLE_ARN_FOR_IRSA
Install SageMaker and Application Auto Scaling controllers
Choose an AWS Region for the SageMaker and automatic scaling resources we create in this post. For convenience, we recommend using us-east-1
:
export SERVICE_REGION="us-east-1"
# Namespace for controller
export ACK_K8S_NAMESPACE="ack-system"
Now, let’s install the SageMaker and Application Auto Scaling controller using the following helper script. This script pulls the helm charts from ACK’s public Amazon Elastic Container Registry (Amazon ECR) repository and configures the values of the AWS account, default Region for resources to be created, and IAM role (created in previous step) in the service account to be used by the controller Pod to assume the role. Create a file named install-controllers.sh
and insert the following code block:
#!/usr/bin/env bash
# Deploy ACK Helm Charts
export HELM_EXPERIMENTAL_OCI=1
export ACK_K8S_NAMESPACE=${ACK_K8S_NAMESPACE:-"ack-system"}
function install_ack_controller() {
local service="$1"
local release_version="$2"
local chart_export_path=/tmp/chart
local chart_ref=$service-chart
local chart_repo=public.ecr.aws/aws-controllers-k8s/$chart_ref
local chart_package=$chart_ref-$release_version.tgz
# Download helm chart
mkdir -p $chart_export_path
helm pull oci://"$chart_repo" --version "$release_version" -d $chart_export_path
tar xvf "$chart_export_path"/"$chart_package" -C "$chart_export_path"
# Update the values in helm chart
pushd $chart_export_path/$service-chart
yq e '.aws.region = env(SERVICE_REGION)' -i values.yaml
yq e '.serviceAccount.annotations."eks.amazonaws.com/role-arn" = env(IAM_ROLE_ARN_FOR_IRSA)' -i values.yaml
popd
# Create a namespace and install the helm chart
helm install -n $ACK_K8S_NAMESPACE --create-namespace ack-$service-controller $chart_export_path/$service-chart
}
install_ack_controller "sagemaker" "v0.3.0"
install_ack_controller "applicationautoscaling" "v0.2.0"
Run the script:
chmod +x install-controllers.sh
./install-controllers.sh
The output contains the following:
Pulled: public.ecr.aws/aws-controllers-k8s/sagemaker-chart:v0.3.0
...
NAME: ack-sagemaker-controller
LAST DEPLOYED: Tue Nov 16 01:53:34 2021
NAMESPACE: ack-system
STATUS: deployed
REVISION: 1
TEST SUITE: None
Pulled: public.ecr.aws/aws-controllers-k8s/applicationautoscaling-chart:v0.2.0
...
NAME: ack-applicationautoscaling-controller
LAST DEPLOYED: Tue Nov 16 01:53:35 2021
NAMESPACE: ack-system
STATUS: deployed
REVISION: 1
TEST SUITE: None
Next, we run the following commands to verify custom resource definitions were applied and controller Pods are running:
kubectl get crds | grep "services.k8s.aws"
The output of the command should contain a number of custom resource definitions related to SageMaker (such as trainingjobs
or endpoint
) and Application Auto Scaling (such as scalingpolicies
and scalabletargets
):
# Get pods in controller namespace
kubectl get pods -n $ACK_K8S_NAMESPACE
We see one controller Pod per service running in the ack-system
namespace:
NAME READY STATUS RESTARTS AGE
ack-applicationautoscaling-controller-7479dc78dd-ts9ng 1/1 Running 0 4m52s
ack-sagemaker-controller-788858fc98-6fgr6 1/1 Running 0 4m56s
Prepare SageMaker resources
Next, we create an S3 bucket and IAM role for SageMaker.
To train a model with SageMaker, we need an S3 bucket to store the dataset and artifacts from the training process. We simply use the preprocessed dataset at s3://SageMaker-sample-files/datasets/tabular/uci_abalone
[1].
Let’s create a variable for the S3 bucket:
export SAGEMAKER_BUCKET=ack-sagemaker-bucket-$RANDOM_VAR
Create a file named create-bucket.sh
and insert the following code block:
printf '
#!/usr/bin/env bash
# create bucket
if [[ $SERVICE_REGION != "us-east-1" ]]; then
aws s3api create-bucket --bucket "$SAGEMAKER_BUCKET" --region "$SERVICE_REGION" --create-bucket-configuration LocationConstraint="$SERVICE_REGION"
else
aws s3api create-bucket --bucket "$SAGEMAKER_BUCKET" --region "$SERVICE_REGION"
fi
# sync dataset
aws s3 sync s3://sagemaker-sample-files/datasets/tabular/uci_abalone/train s3://"$SAGEMAKER_BUCKET"/datasets/tabular/uci_abalone/train
aws s3 sync s3://sagemaker-sample-files/datasets/tabular/uci_abalone/validation s3://"$SAGEMAKER_BUCKET"/datasets/tabular/uci_abalone/validation
' > ./create-bucket.sh
Run the script to create the S3 bucket and copy the dataset:
chmod +x create-bucket.sh
./create-bucket.sh
The SageMaker training job that we run later in the post needs an IAM role to access Amazon S3 and SageMaker. Run the following commands to create a SageMaker execution IAM role that is used by SageMaker to access AWS resources:
export SAGEMAKER_EXECUTION_ROLE_NAME=ack-sagemaker-execution-role-$RANDOM_VAR
TRUST="{ "Version": "2012-10-17", "Statement": [ { "Effect": "Allow", "Principal": { "Service": "sagemaker.amazonaws.com" }, "Action": "sts:AssumeRole" } ] }"
aws iam create-role --role-name ${SAGEMAKER_EXECUTION_ROLE_NAME} --assume-role-policy-document "$TRUST"
aws iam attach-role-policy --role-name ${SAGEMAKER_EXECUTION_ROLE_NAME} --policy-arn arn:aws:iam::aws:policy/AmazonSageMakerFullAccess
aws iam attach-role-policy --role-name ${SAGEMAKER_EXECUTION_ROLE_NAME} --policy-arn arn:aws:iam::aws:policy/AmazonS3FullAccess
SAGEMAKER_EXECUTION_ROLE_ARN=$(aws iam get-role --role-name ${SAGEMAKER_EXECUTION_ROLE_NAME} --output text --query 'Role.Arn')
echo $SAGEMAKER_EXECUTION_ROLE_ARN
Note down the execution role ARN to use in later steps.
Train an XGBoost model
Now, we create a training.yaml
file to specify the parameters for a SageMaker training job. SageMaker training jobs enable remote training of ML models. You can customize each training job to run your own ML scripts with custom architectures, data loaders, hyperparameters, and more. To submit a SageMaker training job, we require a job name. Let’s create that variable first:
export JOB_NAME=ack-xgboost-training-job-$RANDOM_VAR
In the following code, we create a training.yaml
file that contains the hyperparameters for the training job as well as the location of the training and validation data. It’s also where we specify the Amazon ECR image used for training.
Note: If your $SERVICE_REGION
isn’t us-east-1
, change the following image URI. For the XGBoost algorithm version 1.2-1 Region-specific image URI, see Docker Registry Paths and Example Code.
export XGBOOST_IMAGE=683313688378.dkr.ecr.us-east-1.amazonaws.com/sagemaker-xgboost:1.2-1
printf '
apiVersion: sagemaker.services.k8s.aws/v1alpha1
kind: TrainingJob
metadata:
name: '$JOB_NAME'
spec:
# Name that will appear in SageMaker console
trainingJobName: '$JOB_NAME'
hyperParameters:
max_depth: "5"
gamma: "4"
eta: "0.2"
min_child_weight: "6"
subsample: "0.7"
objective: "reg:linear"
num_round: "50"
verbosity: "2"
algorithmSpecification:
trainingImage: '$XGBOOST_IMAGE'
trainingInputMode: File
roleARN: '$SAGEMAKER_EXECUTION_ROLE_ARN'
outputDataConfig:
# The output path of our model
s3OutputPath: s3://'$SAGEMAKER_BUCKET'
resourceConfig:
instanceCount: 1
instanceType: ml.m4.xlarge
volumeSizeInGB: 5
stoppingCondition:
maxRuntimeInSeconds: 3600
inputDataConfig:
- channelName: train
dataSource:
s3DataSource:
s3DataType: S3Prefix
# The input path of our train data
s3URI: s3://'$SAGEMAKER_BUCKET'/datasets/tabular/uci_abalone/train/abalone.train
s3DataDistributionType: FullyReplicated
contentType: text/libsvm
compressionType: None
- channelName: validation
dataSource:
s3DataSource:
s3DataType: S3Prefix
# The input path of our validation data
s3URI: s3://'$SAGEMAKER_BUCKET'/datasets/tabular/uci_abalone/validation/abalone.validation
s3DataDistributionType: FullyReplicated
contentType: text/libsvm
compressionType: None
' > ./training.yaml
Now, we can create the training job:
kubectl apply -f training.yaml
You should see the following output:
trainingjob.sagemaker.services.k8s.aws/ack-xgboost-training-job-7420 created
You can watch the status of the training job. It takes a few minutes for STATUS
to show as Completed
.
kubectl get trainingjob.sagemaker --watch
NAME SECONDARYSTATUS STATUS
ack-xgboost-training-job-7420 Starting InProgress
ack-xgboost-training-job-7420 Downloading InProgress
ack-xgboost-training-job-7420 Training InProgress
ack-xgboost-training-job-7420 Completed Completed
Deploy the results of the SageMaker training job
To deploy the model, we need to specify a model name, an endpoint config name, and an endpoint name:
export MODEL_NAME=ack-xgboost-model-$RANDOM_VAR
export ENDPOINT_CONFIG_NAME=ack-xgboost-endpoint-config-$RANDOM_VAR
export ENDPOINT_NAME=ack-xgboost-endpoint-$RANDOM_VAR
We deploy this model on a c5.large instance type. In the following .yaml file, we define the model, the endpoint config, and the endpoint:
printf '
apiVersion: sagemaker.services.k8s.aws/v1alpha1
kind: Model
metadata:
name: '$MODEL_NAME'
spec:
modelName: '$MODEL_NAME'
primaryContainer:
containerHostname: xgboost
# The source of the model data
modelDataURL: s3://'$SAGEMAKER_BUCKET'/'$JOB_NAME'/output/model.tar.gz
image: '$XGBOOST_IMAGE'
executionRoleARN: '$SAGEMAKER_EXECUTION_ROLE_ARN'
---
apiVersion: sagemaker.services.k8s.aws/v1alpha1
kind: EndpointConfig
metadata:
name: '$ENDPOINT_CONFIG_NAME'
spec:
endpointConfigName: '$ENDPOINT_CONFIG_NAME'
productionVariants:
- modelName: '$MODEL_NAME'
variantName: AllTraffic
instanceType: ml.c5.large
initialInstanceCount: 1
---
apiVersion: sagemaker.services.k8s.aws/v1alpha1
kind: Endpoint
metadata:
name: '$ENDPOINT_NAME'
spec:
endpointName: '$ENDPOINT_NAME'
endpointConfigName: '$ENDPOINT_CONFIG_NAME'
' > ./deploy.yaml
Now, the endpoint is ready to be deployed:
kubectl apply -f deploy.yaml
You should see the following output:
model.sagemaker.services.k8s.aws/ack-xgboost-model-7420 created
endpointconfig.sagemaker.services.k8s.aws/ack-xgboost-endpoint-config-7420 created
endpoint.sagemaker.services.k8s.aws/ack-xgboost-endpoint-7420 created
We can observe that the model and endpoint config were created. Deploying the endpoint may take some time:
kubectl describe models.sagemaker
kubectl describe endpointconfigs.sagemaker
kubectl describe endpoints.sagemaker
We can watch this process using the following command:
kubectl get endpoints.sagemaker --watch
After some time, the STATUS
changes to InService
:
NAME STATUS
ack-xgboost-endpoint-7420 Creating
ack-xgboost-endpoint-7420 InService
This indicates the deployed endpoint is ready for use.
Verify the inference capabilities of the trained model
We invoke the model endpoint using Python to emulate a typical use case. We reuse the code in SageMaker example notebook.
We first download the test set from Amazon S3. Then we load a single sample from the test set and use it to invoke the endpoint we deployed in the previous section. Download the test file with the following code:
pip install boto3 numpy
aws s3 cp s3://sagemaker-sample-files/datasets/tabular/uci_abalone/test/abalone.test abalone.test
head -1 abalone.test > abalone.single.test
Use the Python interpreter to test inference. The Python interpreter is usually installed as /usr/local/bin/python<version>
on those machines where it’s available; putting /usr/local/bin
in your Unix/Linux shell’s search path makes it possible to start it by entering the Python command.
Create a file named predict.py
and insert the following code block:
printf '
import sys
import math
import json
import boto3
import numpy as np
import os
region = os.environ.get("SERVICE_REGION")
endpoint_name = os.environ.get("ENDPOINT_NAME")
runtime_client = boto3.client("runtime.sagemaker", region_name=region)
file_name = "abalone.single.test"
with open(file_name, "r") as f:
payload = f.read().strip()
response = runtime_client.invoke_endpoint(
EndpointName=endpoint_name, ContentType="text/x-libsvm", Body=payload
)
result = response["Body"].read().decode("utf-8").split(",")
result = [math.ceil(float(i)) for i in result]
label = payload.strip(" ").split()[0]
print("Label: " + label)
print("Prediction:" + str(result[0]))
' > ./predict.py
python predict.py
Running this sample should give us the following result:
Label: 12
Prediction: 13
The age of the abalone that is provided in the test example is estimated to be 13 by the ML model. The actual age was 12. This suggests that our ML model has been trained and provides reasonable predictions. However, the experienced ML user may realize that we haven’t performed hyperparameter tuning and other methods of increasing accuracy yet, which is outside the scope of this post.
Dynamically scale the endpoint according to the load
SageMaker ACK Operators support custom resource definitions for automatic scaling (using ScalableTarget and ScalingPolicy) for your hosted models. The following resources adjust the number of instances (minimum 1 to maximum 20) provisioned for a model in response to changes in metric SageMakerVariantInvocationsPerInstancetracking
, which is the average number of times per minute that each instance for a variant is invoked:
printf '
apiVersion: applicationautoscaling.services.k8s.aws/v1alpha1
kind: ScalableTarget
metadata:
name: ack-scalable-target-predfined
spec:
maxCapacity: 20
minCapacity: 1
resourceID: endpoint/'$ENDPOINT_NAME'/variant/AllTraffic
scalableDimension: "sagemaker:variant:DesiredInstanceCount"
serviceNamespace: sagemaker
---
apiVersion: applicationautoscaling.services.k8s.aws/v1alpha1
kind: ScalingPolicy
metadata:
name: ack-scaling-policy-predefined
spec:
policyName: ack-scaling-policy-predefined
policyType: TargetTrackingScaling
resourceID: endpoint/'$ENDPOINT_NAME'/variant/AllTraffic
scalableDimension: "sagemaker:variant:DesiredInstanceCount"
serviceNamespace: sagemaker
targetTrackingScalingPolicyConfiguration:
targetValue: 60
scaleInCooldown: 700
scaleOutCooldown: 300
predefinedMetricSpecification:
predefinedMetricType: SageMakerVariantInvocationsPerInstance
' > ./scale-endpoint.yaml
Apply with the following code:
kubectl apply -f scale-endpoint.yaml
You should see the following output:
scalabletarget.applicationautoscaling.services.k8s.aws/ack-scalable-target-predfined created
scalingpolicy.applicationautoscaling.services.k8s.aws/ack-scaling-policy-predefined created
We can observe that scalingpolicy
was created:
kubectl describe scalingpolicy.applicationautoscaling
The output of scalingpolicy
looks like the following:
Status:
Ack Resource Metadata:
Arn: arn:aws:autoscaling:us-east-1:123456789012:scalingPolicy:b33d12b8-aa81-4cb8-855e-c7b6dcb9d6e7:resource/SageMaker/endpoint/ack-xgboost-endpoint/variant/AllTraffic:policyName/ack-scaling-policy-predefined
Owner Account ID: 123456789012
Alarms:
Alarm ARN: arn:aws:cloudwatch:us-east-1:123456789012:alarm:TargetTracking-endpoint/ack-xgboost-endpoint/variant/AllTraffic-AlarmHigh-966b8232-a9b9-467d-99f3-95436f5c0383
Alarm Name: TargetTracking-endpoint/ack-xgboost-endpoint/variant/AllTraffic-AlarmHigh-966b8232-a9b9-467d-99f3-95436f5c0383
Alarm ARN: arn:aws:cloudwatch:us-east-1:123456789012:alarm:TargetTracking-endpoint/ack-xgboost-endpoint/variant/AllTraffic-AlarmLow-71e39f85-1afb-401d-9703-b788cdc10a93
Alarm Name: TargetTracking-endpoint/ack-xgboost-endpoint/variant/AllTraffic-AlarmLow-71e39f85-1afb-401d-9703-b788cdc10a93
Clean up
Run the following commands to delete the resources created in this post:
kubectl delete -f scale-endpoint.yaml
kubectl delete -f deploy.yaml
kubectl delete -f training.yaml
Create a file named uninstall-controller.sh
and insert the following code block required for deleting the controller and custom resource definitions:
printf '
#!/usr/bin/env bash
# Uninstall Controller
export HELM_EXPERIMENTAL_OCI=1
export ACK_K8S_NAMESPACE=${ACK_K8S_NAMESPACE:-"ack-system"}
function uninstall_ack_controller() {
local service="$1"
local chart_export_path=/tmp/chart
helm uninstall -n $ACK_K8S_NAMESPACE ack-$service-controller
kubectl delete -f $chart_export_path/ack-$service-controllerchart/crds
}
uninstall_ack_controller "sagemaker"
uninstall_ack_controller "applicationautoscaling"
' > ./uninstall-controller.sh
Run the following commands to uninstall the controller and custom resource definitions, and delete the namespace, IAM roles, and S3 bucket you created:
# uninstall controller and remove CRDs
chmod +x uninstall-controller.sh
./uninstall-controller.sh
# Delete controller namespace
kubectl delete namespace $ACK_K8S_NAMESPACE
# Delete S3 bucket
aws s3 rb s3://$SAGEMAKERageMaker_BUCKET --force
# Delete SageMaker execution role
aws iam detach-role-policy --role-name $SAGEMAKER_EXECUTION_ROLE_NAME --policy-arn arn:aws:iam::aws:policy/AmazonSageMakerFullAccess
aws iam detach-role-policy --role-name $SAGEMAKER_EXECUTION_ROLE_NAME --policy-arn arn:aws:iam::aws:policy/AmazonS3FullAccess
aws iam delete-role --role-name $SAGEMAKER_EXECUTION_ROLE_NAME
# Delete application autoscaling service linked role
aws iam delete-service-linked-role --role-name AWSServiceRoleForApplicationAutoScaling_SageMakerEndpoint
# Delete IAM role created for IRSA
aws iam detach-role-policy --role-name $OIDC_ROLE_NAME --policy-arn arn:aws:iam::aws:policy/AmazonSageMakerFullAccess
aws iam delete-role-policy --role-name $OIDC_ROLE_NAME --policy-name "iam-pass-role-policy"
aws iam delete-role --role-name $OIDC_ROLE_NAME
Conclusion
SageMaker ACK Operators provide engineering teams with a native Kubernetes experience for creating and interacting with the ML jobs on SageMaker, either with the Kubernetes API or with Kubernetes command line utilities such as kubectl. You can build automation, tooling, and custom interfaces for data scientists in Kubernetes by using these controllers—all without building, maintaining, or optimizing ML infrastructure. Data scientists and developers familiar with Kubernetes can compose and interact with fully managed SageMaker training, tuning, and inference jobs, as you would with Kubernetes jobs running locally. Logs from SageMaker jobs stream back to Kubernetes, allowing you to natively view logs for your model training, tuning, and prediction jobs in the command line.
ACK is a community-driven project and will soon include service controllers for other AWS service APIs.
Links
[1] Dua, D. and Graff, C. (2019). UCI Machine Learning Repository [http://archive.ics.uci.edu/ml]. Irvine, CA: University of California, School of Information and Computer Science.About the Authors
Kanwaljit Khurmi is a Senior Solutions Architect at Amazon Web Services. He works with the AWS customers to provide guidance and technical assistance helping them improve the value of their solutions when using AWS. Kanwaljit specializes in helping customers with containerized and machine learning applications.
Suraj Kota is a Software Engineer specialized in Machine Learning infrastructure. He builds tools to easily get started and scale machine learning workload on AWS. He worked on the AWS Deep Learning Containers, Deep Learning AMI, SageMaker Operators for Kubernetes, and other open source integrations like Kubeflow.
Archis Joglekar is an AI/ML Partner Solutions Architect in the Emerging Technologies team. He is interested in performant, scalable deep learning and scientific computing using the building blocks at AWS. His past experiences range from computational physics research to machine learning platform development in academia, national labs, and startups. His time away from the computer is spent playing soccer and with friends and family.
Özalp Özer receives INFORMS honorable mention
The paper by Özer and his co-authors examines the role of earmarking and transparency on donors and their donations.Read More
Postprocessing with Amazon Textract: Multi-page table handling
Amazon Textract is a machine learning (ML) service that automatically extracts printed text, handwriting, and other data from scanned documents that goes beyond simple optical character recognition (OCR) to identify and extract data from forms and tables.
Currently, thousands of customers are using Amazon Textract to process different types of documents. Many include tables across one or multiple pages, such as bank statements and financial reports.
Many developers expressed interest in merging Amazon Textract responses where tables exist across multiple pages. This post demonstrates how you can use the amazon-textract-response-parser utility to accomplish this and highlights a few tricks to optimize the process.
Solution overview
When tables span multiple pages, a series of steps and validations are required to determine the linkage across pages correctly.
These include analyzing the table structure similarities across pages (columns, headers, margins) and determining if any additional contents like headers or footers exist that may logically break the tables. These logical steps are separated into two major groups (page context and table structure), and you can adjust and optimize each logical step according to your use case.
This solution runs these tasks in series and only merges the results when all checks are completed and passed. The following diagram shows the solution workflow.
Implement the solution
To get started, you must install the amazon-textract-response-parser
, and amazon-textract-helper
libraries. The Amazon Textract response parser library enables us to easily parse the Amazon Textract JSON response and provides constructs to work with different parts of the document effectively. This post focuses on the merge/link tables feature. Amazon-textract-helper
is another useful library that provides a collection of ready-to-use functions and sample implementations to speed up the evaluation and development of any project using Amazon Textract.
- Install the libraries with the following code:
!pip install amazon-textract-response-parser
!pip install amazon-textract-helper
- The postprocessing step to identify related tables and merge them is part of the
trp.trp2
library, which you must import into your notebook:
import trp.trp2 as t2
from trp.t_pipeline import pipeline_merge_tables
from textractcaller.t_call import call_textract, Textract_Features
from trp.trp2 import TDocument, TDocumentSchema
from trp.t_tables import MergeOptions, HeaderFooterType
- Next, call Amazon Textract to process the document:
textract_json = call_textract(input_document=s3_uri_of_documents, features=[Textract_Features.TABLES], boto3_textract_client = textract_client)
- Finally, load the response JSON into a document and run the pipeline. The footer and header heights are configurable by the user. There are three default values can be used for
HeaderFooterType
:None
,Narrow
, andNormal
.
t_document: t2.TDocument = t2.TDocumentSchema().load(textract_json)
t_document = pipeline_merge_tables(t_document, MergeOptions.MERGE, None, HeaderFooterType.NONE)
Pipeline_merge_tables
takes a merge option parameter that can be either .MERGE
or .LINK
.
MergeOptions.MERGE
combines the tables and makes them appear as one for postprocessing, with the drawback that the geometry information is no longer in the correct location because you now have cells and tables from subsequent pages moved to the page with the first part of the table.
MergeOptions.LINK
maintains the geometric structure and enriches the table information with links between the table elements. A custom['previous_table']
and custom['next_table']
attribute is added to the TABLE
blocks in the Amazon Textract JSON schema.
The following image represents a sample PDF file with a table that spans over two pages.
The following shows the Amazon Textract response without table merge postprocessing (left) and the response with table merge postprocessing (right).
Define a custom table merge validation function
The provided postprocessing API works for the majority of use cases; however, based on your specific use case, you can define a custom merge function to improve its accuracy.
This custom function is passed to the CustomTableDetectionFunction
parameter of the pipeline_merge_tables
function to overwrite the existing logic of identifying the tables to merge. The following steps represent the existing logic.
- Validate context between tables. Check if there are any line items between the first and second table except in the footer and header area. If there are any line items, tables are considered separate tables.
- Compare the column numbers. If the two tables don’t have the same number of columns, this is an indicator of separate logical tables.
- Compare the headers. If the two tables have the exact same columns (same cell number and cell labels), this is a very strong indication of the same logical table.
- Compare table dimensions. Verify that the two tables have the same left and right margin. An accuracy percentage parameter can be passed to allow for some degree of error (for example, if the pages are scanned from papers, consequent tables on different pages may have different weights).
If you have a different requirement, you can pass your own custom table detection function to the pipeline_merge_tables
API as follows:
def CustomTableDetectionFunction(t_document) -> List[List[str]])
table_ids_merge_list = []
ordered_doc = order_blocks_by_geo(t_document)
trp_doc = Document(TDocumentSchema().dump(ordered_doc))
for current_page in trp_doc.pages:
for table in current_page.tables:
# Provide your custom logic here to determine which tableids should merge to one table
# if(custom logic)
# table_ids_merge_list.append(>tableid1, tableid2, tableid3, ...etc.)
return table_ids_merge_list
t_document = pipeline_merge_tables(t_document, MergeOptions.MERGE, CustomTableDetectionFunction, HeaderFooterType.NORMAL)
Our current implementation for the table detection function and pipeline_merge_tables
function in our Amazon Textract response parser library is available on GitHub. The customTableDetection
function returns a list of lists (of strings), which is required by the merge_table
or link_table
functions (based on the MergeOptions
parameter) called internally by the pipeline_merge_tables
API.
Run sample code
The Amazon Textract multi-page tables processing repository provides sample code on how to use the merge tables feature and covers common scenarios that you may encounter in your documents. To try the sample code, you first launch an Amazon SageMaker notebook instance with the code repository, then you can access the notebook to review the code samples.
Launch a SageMaker notebook instance with the code repository
To launch a SageMaker notebook instance, complete the following steps:
- Choose the following link to launch an AWS CloudFormation template that deploys a SageMaker notebook instance along with the sample code repository:
- Sign in to the AWS Management Console with your AWS Identity and Access Management (IAM) user name and password.
You arrive at the Create Stack page on the Specify Template step.
- Choose Next.
- For Specify Stack Name, enter a stack name.
- Choose Next.
- Choose Next
- On the review page, acknowledge the IAM resource creation and choose Create stack.
Access the SageMaker notebook and review the code samples
When the stack creation is complete, you can access the notebook and review the code samples.
- On the Outputs tab of the stack, choose the link corresponding to the value of the
NotebookInstanceName
key. - Choose Open Jupyter.
- Go to the home page of your Jupyter notebook and browse to the
amazon-textract-multipage-tables-processing
directory. - Open the Jupyter notebook inside this directory and the sample code provided.
Conclusion
This post demonstrated how to use the Amazon Textract response parser component to identify and merge tables that span multiple pages. You walked through generic checks that you can use to identify a multi-page table, learned how to build your own custom function, and reviewed the two options to merge tables in the Amazon Textract response JSON.
If this post helps you or inspires you to solve a problem, we would love to hear about it! The code for this solution is available on the GitHub repo for you to use and extend. Contributions are always welcome!
About the Authors
Mehran Najafi, PhD, is a Senior Solutions Architect for AWS focused on AI/ML solutions and architectures at scale.
Keith Mascarenhas is a Solutions Architect and works with our small and medium sized customers in central Canada to help them grow and achieve outcomes faster with AWS. He is also passionate about machine learning and is a member of the Amazon Computer Vision Hero program.
Yuan Jiang is a Sr Solutions Architect with a focus in machine learning. He’s a member of the Amazon Computer Vision Hero program and the Amazon Machine Learning Technical Field Community.
Martin Schade is a Senior ML Product SA with the Amazon Textract team. He has over 20 years of experience with internet-related technologies, engineering, and architecting solutions, and joined AWS in 2014. He has guided some of the largest AWS customers on the most efficient and scalable use of AWS services, and later focused on AI/ML with a focus on computer vision. He is currently obsessed with extracting information from documents.
Machine learning inference at scale using AWS serverless
With the growing adoption of Machine Learning (ML) across industries, there is an increasing demand for faster and easier ways to run ML inference at scale. ML use cases, such as manufacturing defect detection, demand forecasting, fraud surveillance, and many others, involve tens or thousands of datasets, including images, videos, files, documents, and other artifacts. These inference use cases typically require the workloads to scale to tens of thousands of parallel processing units. The simplicity and automated scaling offered by AWS serverless solutions makes it a great choice for running ML inference at scale. Using serverless, inferences can be run without provisioning or managing servers and while only paying for the time it takes to run. ML practitioners can easily bring their own ML models and inference code to AWS by using containers.
This post shows you how to run and scale ML inference using AWS serverless solutions: AWS Lambda and AWS Fargate.
Solution overview
The following diagram illustrates the solutions architecture for both batch and real-time inference options. The solution is demonstrated using a sample image classification use case. Source code for this sample is available on GitHub.
AWS Fargate: Lets you run batch inference at scale using serverless containers. Fargate task loads the container image with the inference code for image classification.
AWS Batch: Provides job orchestration for batch inference by dynamically provisioning Fargate containers as per job requirements.
AWS Lambda: Lets you run real-time ML inference at scale. The Lambda function loads the inference code for image classification. Lambda function is also used to submit batch inference jobs.
Amazon API Gateway: Provides a REST API endpoint for the inference Lambda function.
Amazon Simple Storage Service (S3): Stores input images and inference results for batch inference.
Amazon Elastic Container Registry (ECR): Stores the container image with inference code for Fargate containers.
Deploying the solution
We have created an AWS Cloud Development Kit (CDK) template to define and configure the resources for the sample solution. CDK lets you provision the infrastructure and build deployment packages for both the Lambda Function and Fargate container. The packages include commonly used ML libraries, such as Apache MXNet and Python, along with their dependencies. The solution is running the inference code using a ResNet-50 model trained on the ImageNet dataset to recognize objects in an image. The model can classify images into 1000 object categories, such as keyboard, pointer, pencil, and many animals. The inference code downloads the input image and performs the prediction with the five classes that the image most relates with the respective probability.
To follow along and run the solution, you need access to:
- An AWS account
- A terminal with AWS Command Line Interface (CLI), CDK, Docker, git, and Python installed. You may use the terminal on your local machine or use an AWS Cloud9 environment.
To deploy the solution, open your terminal window and complete the following steps.
- Clone the GitHub repo
$ git clone https://github.com/aws-samples/aws-serverless-for-machine-learning-inference
- Navigate to the project directory and deploy the CDK application.
$ ./install.sh
or
$ ./cloud9_install.sh #If you are using AWS Cloud9
Enter Y to proceed with the deployment.
This performs the following steps to deploy and configure the required resources in your AWS account. It may take around 30 minutes for the initial deployment, as it builds the Docker image and other artifacts. Subsequent deployments typically complete within a few minutes.
- Creates a CloudFormation stack (“MLServerlessStack”).
- Creates a container image from the Dockerfile and the inference code for batch inference.
- Creates an ECR repository and publishes the container image to this repo.
- Creates a Lambda function with the inference code for real-time inference.
- Creates a batch job configuration with Fargate compute environment in AWS Batch.
- Creates an S3 bucket to store inference images and results.
- Creates a Lambda function to submit batch jobs in response to image uploads to S3 bucket.
Running inference
The sample solution lets you get predictions for either a set of images using batch inference or for a single image at a time using real-time API endpoint. Complete the following steps to run inferences for each scenario.
Batch inference
Get batch predictions by uploading image files to Amazon S3.
- Using Amazon S3 console or using AWS CLI, upload one or more image files to the S3 bucket path ml-serverless-bucket-<acct-id>–<aws-region>/input.
$ aws s3 cp <path to jpeg files> s3://ml-serverless-bucket-<acct-id>-<aws-region>/input/ --recursive
- This will trigger the batch job, which will spin-off Fargate tasks to run the inference. You can monitor the job status in AWS Batch console.
- Once the job is complete (this may take a few minutes), inference results can be accessed from the ml-serverless-bucket-<acct-id>–<aws-region>/output path.
Real-time inference
Get real-time predictions by invoking the REST API endpoint with an image payload.
- Navigate to the CloudFormation console and find the API endpoint URL (httpAPIUrl) from the stack output.
- Use an API client, like Postman or curl command, to send a POST request to the
/predict
API endpoint with image file payload.$ curl --request POST -H "Content-Type: application/jpeg" --data-binary @<your jpg file name> <your-api-endpoint-url>/predict
- Inference results are returned in the API response.
Additional recommendations and tips
Here are some additional recommendations and options to consider for fine-tuning the sample to meet your specific requirements:
- Scaling – Update AWS Service Quotas in your account and Region as per your scaling and concurrency needs to run the solution at scale. For example, if your use case requires scaling beyond the default Lambda concurrent executions limit, then you must increase this limit to reach the desired concurrency. You also need to size your VPC and subnets with a wide enough IP address range to allow the required concurrency for Fargate tasks.
- Performance – Perform load tests and fine tune performance across each layer to meet your needs.
- Use container images with Lambda – This lets you use containers with both AWS Lambda and AWS Fargate, and you can simplify source code management and packaging.
- Use AWS Lambda for batch inferences – You can use Lambda functions for batch inferences as well if the inference storage and processing times are within Lambda limits.
- Use Fargate Spot – This lets you run interruption tolerant tasks at a discounted rate compared to the Fargate price, and reduce the cost for compute resources.
- Use Amazon ECS container instances with Amazon EC2 – For use cases that need a specific type of compute, you can make use of EC2 instances instead of Fargate.
Cleaning up
Navigate to the project directory from the terminal window and run the following command to destroy all resources and avoid incurring future charges.
$ cdk destroy
Conclusion
This post demonstrated how to bring your own ML models and inference code and run them at scale using serverless solutions in AWS. The solution made it possible to deploy your inference code in AWS Fargate and AWS Lambda. Moreover, it also deployed an API endpoint using Amazon API Gateway for real-time inferences and batch job orchestration using AWS Batch for batch inferences. Effectively, this solution lets you focus on building ML models by providing an efficient and cost-effective way to serve predictions at scale.
Try it out today, and we look forward to seeing the exciting machine learning applications that you bring to AWS Serverless!
Additional Reading:
- Seamlessly Scale Predictions with AWS Lambda and MXNet
- Build reusable, serverless inference functions for your Amazon SageMaker models using AWS Lambda layers and containers
About the Authors
Poornima Chand is a Senior Solutions Architect in the Strategic Accounts Solutions Architecture team at AWS. She works with customers to help solve their unique challenges using AWS technology solutions. She focuses on Serverless technologies and enjoys architecting and building scalable solutions.
Greg Medard is a Solutions Architect with AWS Business Development and Strategic Industries. He helps customers with the architecture, design, and development of cloud-optimized infrastructure solutions. His passion is to influence cultural perceptions by adopting DevOps concepts that withstand organizational challenges along the way. Outside of work, you may find him spending time with his family, playing with a new gadget, or traveling to explore new places and flavors.
Mani Khanuja is an Artificial Intelligence and Machine Learning Specialist SA at Amazon Web Services (AWS). She helps customers using machine learning to solve their business challenges using the AWS. She spends most of her time diving deep and teaching customers on AI/ML projects related to computer vision, natural language processing, forecasting, ML at the edge, and more. She is passionate about ML at edge, therefore, she has created her own lab with self-driving kit and prototype manufacturing production line, where she spends lot of her free time.
Vasu Sankhavaram is a Senior Manager of Solutions Architecture in Amazon Web Services (AWS). He leads Solutions Architects dedicated to Hitech accounts. Vasu holds an MBA from U.C. Berkeley, and a Bachelor’s degree in Engineering from University of Mysore, India. Vasu and his wife have their hands full with a son who’s a sophomore at Purdue, twin daughters in third grade, and a golden doodle with boundless energy.
Chitresh Saxena is a Senior Technical Account Manager at Amazon Web Services. He has a strong background in ML, Data Analytics and Web technologies. His passion is solving customer problems, building efficient and effective solutions on the cloud with AI, Data Science and Machine Learning.
Amazon Books editors reveal best 2021 general interest science books
Amazon Books editors have announced their picks for 2021 Best Books of the Year, including the top book within the general interest science category, Walter Isaacson’s ‘The Code Breaker’.Read More