We’re living in a world of everything-as-an-online-service. Service providers from almost every industry are in the race to feature the best user experience for their online channels like web portals and mobile applications. This raises a new challenge. How do we stop illegal and fraudulent behaviors without impacting typical legitimate interactions? This challenge is even greater for organizations that offer paid services. These organizations need to validate payment transactions against fraudulent behaviors in your customer-facing applications. Although subsequent checks are performed by financial entities such as card networks and banks that run the payment transaction, the service providers remain responsible for the end-to-end payment process.
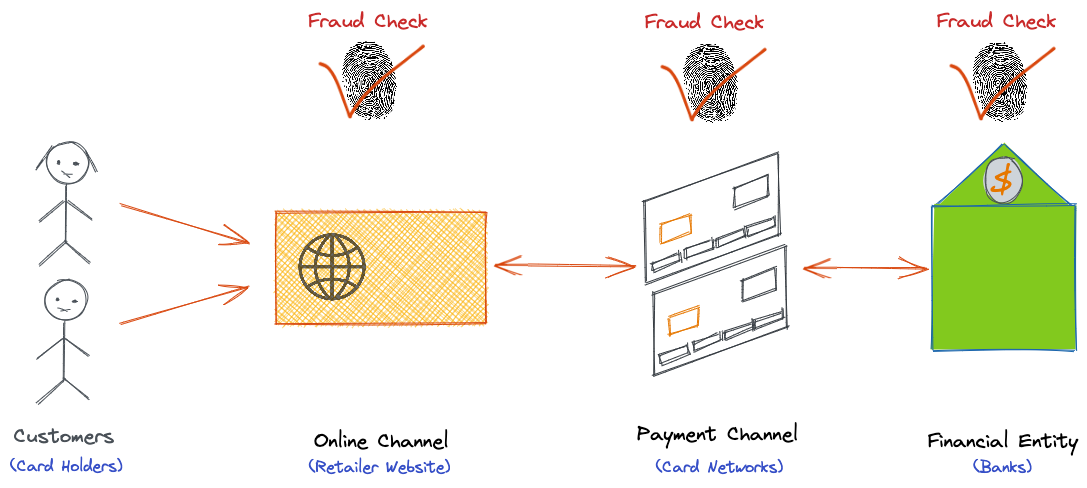
Organizations from all around the world have long implemented rule-based fraud detection systems. The following is an example of a sample rule:
if IP_ADDRESS_LOCATION is ’Japan’
and CUST_ADDRESS_COUNTRY is ‘Japan’
and CUSTOMER_PHONE_LOC is ‘Spain’
then
Investigate
Although these systems are easy to implement, they’re not scalable for everyday new fraud trends, because fraudsters are constantly looking for new loopholes to exploit and ways to hijack those static rules. As a result, new rules must be added every day. This can lead to thousands of rules, making the system difficult to maintain.
More advanced ways are needed to detect and stop losses from fraud that may be damaging organizations’ revenue and brand reputation. In this post, we discuss how to create a real-time fraud prevention system using Amazon Fraud Detector.
Solution overview
Emerging technologies like AI and machine learning (ML) can provide a solution that shifts from enforcing rule-based validations to using validations based on learning from examples and trends directly found in the transaction data. By specifying the key features that may contribute to fraudulent behavior, such as customer-related information (card number, email, IP address, and location) and transaction-related information (time, amount, and currency). An ML model can utilize statistical algorithms to identify trends such as the customer’s frequency of purchases, spending patterns, points of interest, and how long their account has been active.
AWS offers AI and ML services to help you achieve this. Amazon Fraud Detector is a scalable, fully managed service that makes it easy to use ML to detect online fraud in real time. It helps you build, deploy, and manage fraud detection models that can also combine ML and rules to ensure successful onboarding for your existing rules that can effectively stop fraudulent scenarios.
Although Amazon Fraud Detector helps you detect fraudulent behaviors, we still need to make sure this is happening without impacting legitimate interactions. To do so, we need two additional components to reduce the processing latency and handle failures: an event store and event processor.
The first component that we need to introduce is an event store to centrally manage and exchange event messages. Apache Kafka is a scalable, durable, and highly available event store for mission-critical applications. It’s designed to support high throughput of thousands of messages per second while providing milliseconds latency. It also decouples the transaction’s producers from consumers by buffering the data so that each consumer can consume the data at their own pace. This is useful if we experience a sudden increase in traffic. For example, let’s assume that on average, your website has tens of payment transactions per second. Then you release a new product that becomes very popular. You start having thousands of checkouts per second. If you’re not using a buffer like Apache Kafka, this traffic spike can overwhelm your backend applications, and potentially lead to downtime.
Amazon Managed Streaming for Apache Kafka (Amazon MSK) is a low-cost, fully managed Apache Kafka service that we use as a temporary durable store for our payment transactions
The second component that we need is a mission-critical stream processor, that we can use to apply fraud detection logic in real time within the E2E payment transaction journey. This stream processor must be scalable to deal with massive amounts of transactions, and reliable to process transactions with a very low latency, while being able to gracefully recover from a failure as if the failure had never happened.
Apache Flink is a popular open-source framework and distributed processing engine for transforming and analyzing data streams in real time. Apache Flink has been designed to perform computations at in-memory speed and at scale. Applications can run continuously with minimal downtime; it uses a recovery mechanism that is based on consistent checkpoints of an application’s state. In case of a failure, the application is restarted and its state is loaded from the latest checkpoint. Furthermore, Apache Flink provides a powerful API to transform, aggregate, and enrich events, and supports exactly-once semantics. Therefore, Apache Flink is a great fit for our stream processing requirements.
Amazon Kinesis Data Analytics is a fully managed service that provides the underlying infrastructure for your Apache Flink applications. It enables you to quickly build and run those applications with low operational overhead. For our solution, we use it to consume payment transactions stored in Amazon MSK and coordinate with Amazon Fraud Detector to detect the fraudulent transactions in real time.
Solution details
The solution in this post provides two use cases that are built on top of the Transaction Fraud Insights model created in the post Detect online transaction fraud with Amazon Fraud Detector.
The first use case demonstrates fraud prevention by identifying fraudulent transactions, flagging them to be blocked, and sending an alert notification. The second, writes all transactions in real time to Amazon OpenSearch Service (successor to Amazon Elasticsearch Service), this enables real-time transaction reporting using OpenSearch Dashboards.
The following architecture diagram illustrates the overall flow.
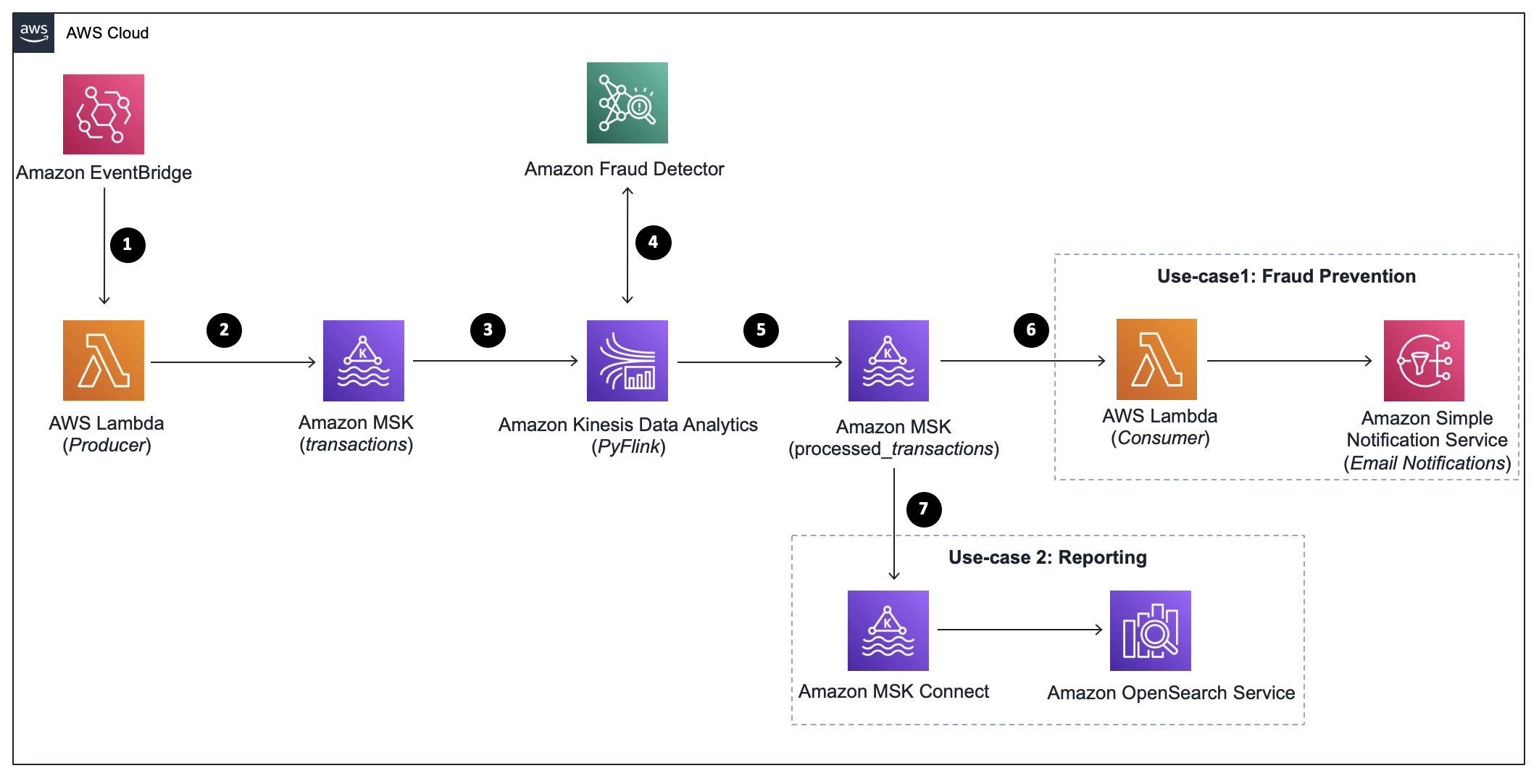
In the following subsections, we provide details about each step in the architecture and the two use cases. The steps are as follows:
- Schedule the transactions producer.
- Generate payment transactions.
- Process the input transactions.
- Get fraud predictions.
- Sink the fraud outcome.
- Send email notifications.
- Visualize real time dashboard.
In subsequent sections, we walk through the steps to deploy the solution with AWS CloudFormation, enable the solution, and visualize the data in OpenSearch Dashboards.
Schedule the transactions producer
The transaction producer runs as an AWS Lambda function. The function is scheduled to run every minute using an Amazon EventBridge rule.
Generate payment transactions
We use a Lambda function that generates synthetic transactions. Each transaction is defined by two sets of data: entities and events.
An entity represents who is performing the transaction such as customer’s details. To enhance the accuracy of the fraud detection model, we use a reference dataset that contains entities used earlier while training the model.
An event represents the transaction-related metrics such as amount and currency. For this, we use faker and random Python libraries.
Each transaction is written into an input Amazon MSK topic called transactions
. The following is a sample transaction record:
{
"transaction_amt": 7,
"email_address": "synthetic@example.com",
"ip_address": "27.67.182.10",
"transaction_currency": "USD",
"event_id": "09a62617-a4af-40f3-926b-a0808c92015c",
"entity_id": "269-37-3393",
"event_time": "2021-11-09T22:56:43.62265",
"billing_longitude": "-80.771",
"billing_state": "VA",
"user_agent": "Opera/8.70.(Windows NT 6.0; mk-MK)",
"billing_street": "370 Synthetic Courts",
"billing_city": "Pulaski",
"card_bin": "423768",
"customer_name": "Synthetic Zamzam",
"product_category": "misc_pos",
"customer_job": "Synthetic Creator",
"phone": "412-515-4616-28430",
"billing_latitude": "37.0567",
"billing_zip": "24301"
}
Process the input transactions
To process the payment transactions in real time, Apache Flink provides the Table API, which allows intuitive processing using relational operators such as selection, filter, and join. For this post, we use the PyFlink Table API running as a Kinesis data analytics application.
The application does the following:
- Reads the transactions from the input topic
transactions
.
- Calls Amazon Fraud Detector APIs to get fraud predictions.
- Writes the results to an output topic on the same MSK cluster.
To read data from and write data into an Amazon MSK topics, we use the out-of-the-box Kafka connector provided by Apache Flink.
Get fraud predictions
The Kinesis data analytics application calls the Amazon Fraud Detector GetEventPrediction API to get the predictions in real time. Because this is considered a custom logic, we use Python user-defined functions (UDFs) to call this API.
For detection, we use a Transaction Fraud Insights model that uses feature engineering to dynamically calculate information about your customers, such as their frequency of purchases, spending patterns, and how long their account has been active. Those aggregates are calculated during training and inference. Because Amazon Fraud Detector aggregates data on entities, it’s useful if the inference data contain entities that are already known to the model. This is because in the online transactions’ context, models indicate lower fraud risk for entities with a high number of legitimate transactions.
Apart from that, to improve model accuracy in production, typically, we frequently retrain the model with a more recent dataset. By default, Amazon Fraud Detector automatically stores event data when you generate predictions. These events are available for future model trainings. We then deploy a new detector version from the newly trained model. This new detector version can be published and become the active version, and therefore all requests to GetEventPrediction
API go to this new version. To avoid any downtime in our Kinesis data analytics application, we don’t specify a detector version in our call. When the version is not specified, the detector’s active version is used. This allows us to change the detector version while being fully transparent from our Kinesis data analytics application.
Sink the fraud outcome
The Kinesis data analytics application writes the output containing the transaction outcome (fraud prediction) into an output Amazon MSK topic called processed_transactions
. Writing the output back to Kafka gives us the benefits we discussed earlier. Moreover, it enables us to consume the same output by different use cases concurrently.
Apache Flink supports different guarantee models: exactly-once, at-most-once, and at-least-once. In our solution, we use Flink’s Kafka sink connector to sink the results to the output topic. This connector supports at-least-once (default) or exactly-once. For this post, we use at-least-once, but you can easily enable exactly-once using the connector options. However, setting the consistency guarantees to exactly-once has an impact on latency because Flink uses two-phase commits and Kafka transactions to guarantee exactly-once. For more information, see An Overview of End-to-End Exactly-Once Processing in Apache Flink.
Send email notifications
To notify downstream services about suspicious transactions, the solution uses a Lambda function to consume records from the processed_transactions
topic. The function evaluates the outcome of each transaction and if the outcome is block, it triggers an Amazon Simple Notification Service (Amazon SNS) notification to notify you by email.
Visualize real-time dashboard
To power real-time dashboards, the solution uses Kafka Connect to sink the data in real time to an Amazon OpenSearch Service domain. This makes the data available for visualization as soon as it is indexed in OpenSearch. Kafka Connect is a scalable and reliable framework to stream data between a Kafka cluster and external systems such as databases, Amazon Simple Storage Service (Amazon S3), and OpenSearch.
Amazon MSK Connect, is a feature of Amazon MSK, enables you to run fully managed Apache Kafka Connect workloads on AWS. MSK Connect is fully compatible with Kafka Connect, enabling you to lift and shift your Kafka Connect applications with zero code changes.
The connector used simply creates an index in Amazon OpenSearch Service with the same name as the output topic in Amazon MSK. If throughput is very high, you need to roll over your indices periodically to stay within the recommended shard size (10–50 GB). Alternatively, you can write the data into an OpenSearch data stream by creating an index template and then configuring the connector to use it. Data streams simplify this process and enforce a setup that best suits append-only time-series data. Because our use case doesn’t have the volume you would normally get with time-series data, we write the output to an index instead.
Each event is indexed into a different document in OpenSearch. The document ID is set to topic+partition+offset
. Therefore, if the same Kafka record is written twice to OpenSearch, the same document will be updated because the document ID will have the same offset. This ensures exactly-once delivery.
Prerequisites
The solution builds on top of the post Detect online transaction fraud with new Amazon Fraud Detector features. We use the same schema as the sample dataset used in the post.
The solution code is available in our GitHub repo. Before proceeding, complete the following prerequisites:
- Create a Transaction Fraud Insights model and a publish a detector as per the steps in that post.
- Follow the instruction on GitHub to package and upload the solution artifacts to an Amazon S3 bucket. The newly created S3 bucket should have 4 artifacts,
- Lambda functions code –
lambda-functions.zip
- Flink code –
RealTimeFraudPrevention.zip
- Kafka connector –
confluentinc-kafka-connect-elasticsearch-11.1.3.zip
- Pre-created OpenSearch dashboard NDJSON file –
dashboard.ndjson
Deploy the solution using AWS CloudFormation
You use CloudFormation templates to create all the necessary resources for the data pipeline. Complete the following steps:
- Choose Launch Stack and navigate to the Region where the Amazon Fraud Detector model is deployed.
- Choose Next.
- For Stack name, enter a name for your stack. The stack name must satisfy the regular expression pattern: [a-z][a-z0-9-]+ and must be fewer than 15 characters long. The default is fraud-prevention.
- Enter the following parameters:
- For BucketName, enter the bucket name where the solution artifacts are stored.
- For S3SourceCodePath, enter the S3 key for the Lambda functions .zip file, the default is
lambda-functions.zip
- For S3connectorPath, enter the S3 key for the Kafka connector .zip file, the default is
confluentinc-kafka-connect-elasticsearch-11.1.6.zip
- For YourEmail, enter the email that receives Amazon SNS notifications.
- For KafkaInputTopic, enter the input topic name, the default is
transactions
- For KafkaOutputTopic, enter the output topic name. We recommend keeping the default value because we use it later in the pre-created OpenSearch dashboard, the default is
processed_transactions
- For FraudDetectorName, enter the detector name, the default is
transaction_fraud_detector
- For FraudDetectorEventName, enter the Amazon Fraud Detector event resource name, the default is
transaction_event
- For FraudDetectorEntityType, enter the Amazon Fraud Detector entity type resource name, the default is
customer
- For OpenSearchMasterUsername, enter the username of the OpenSearch Service domain, the default is
admin
- For OpenSearchMasterPassword, enter the password of the OpenSearch Service domain. The password must meet the following requirements:
- Minimum 8 characters long.
- Contains at least one uppercase letter, one lowercase letter, one digit, and one special character.
- Follow the wizard to create the stack.
Enable the solution
After the stack is successfully created, you can see that the status of the MSK cluster is Updating. The reason for this is that we used a custom resource in the CloudFormation template to change the configuration of the MSK cluster. For the purpose of this post, we set the auto.create.topics.enable to true
. This setting enables automatic creation of topics on the server.
After the status of the MSK cluster changes to Active
, complete the following steps to enable the solution:
- On the AWS Cloud9 console, you should see an AWS Cloud9 environment provisioned by the CloudFormation template.
- Choose Open IDE.
- On the AWS CloudFormation console, navigate to the stack you deployed and choose the Outputs tab.
- Copy the value of the
EnableEventRule
key and run it in your AWS Cloud9 terminal. It should follow the following format:
aws events enable-rule --name <Event_bridge_rule_name>
- Go back to the CloudFormation stack Outputs tab and copy the value of the
EnableEventSourceMapping
key and run it in your AWS Cloud9 terminal. It should follow the following format:
aws lambda update-event-source-mapping --uuid <UUID> --enabled
Visualize the data in OpenSearch Dashboards
Now that that data is flowing through the system, we can create a simple dashboard to visualize this data in real time. To save you development time and effort, we pre-created a sample dashboard that you can import directly into OpenSearch Dashboards. The dashboard file creates all the necessary objects required by the dashboard, including index patterns, visuals, and the dashboard.
The pre-created template uses an OpenSearch index pattern of processed_transactions
*, which is the same prefix as the default Amazon MSK output topic name. Complete the following steps to import the dashboards:
- On the AWS CloudFormation console, navigate to the stack you deployed and choose the Outputs tab.
- Take note of the OpenSearch dashboard link including the trailing
/_dashboards
.
- In the AWS Cloud9 terminal, download
dashboard.ndjson
(the Amazon OpenSearch Service dashboard object NDJSON file):
wget https://github.com/aws-samples/realtime-fraud-prevention/blob/main/Artifacts/dashboard.ndjson
- Use curl to run the following command to generate the appropriate authorization cookies needed to import the dashboards:
curl -X POST <OpenSearch_dashboard_link>/auth/login -H "osd-xsrf: true" -H "content-type:application/json" -d '{"username":"<username>", "password" : "<password>"} ' -c auth.txt
- Run the following command to import all objects defined in the NDJSON file:
curl -XPOST <OpenSearch_dashboard_link>/api/saved_objects/_import -H "osd-xsrf:true" -b auth.txt --form file=@dashboard.ndjson
Now the dashboard is immediately available in OpenSearch Dashboards. However, because the Amazon OpenSearch Service domain is provisioned in a private VPC, you must have VPN access to the VPC or use a bastion host be able to access OpenSearch Dashboards..
- Follow the instruction on GitHub to access OpenSearch Dashboards.
- After logging in to OpenSearch you will find a new sample fraud detection dashboard, which is updated in real time.
You’ve now created a sample dashboard.
Clean up
To clean up after using this solution, complete the following steps:
Conclusion
In this post, we showcased a simple, cost-effective, and efficient solution to detect and stop fraud. The solution uses open-source frameworks and tools like Apache Kafka, Apache Flink, and OpenSearch coupled with ML-based fraud detection mechanism using Amazon Fraud Detector. The solution is designed to process transactions (and identify fraud) in the range of milliseconds, and therefore has no negative impact on the experience of legitimate customers.
You can integrate this solution with your current transaction processing application to protect revenue losses that occur from fraud. This can be achieved by modifying the source code available on GitHub to replace the Lambda producer and consumer with your own application microservices.
About the Authors
Ahmed Zamzam is a Specialist Solutions Architect for Analytics AWS. He supports SMB customers in the UK in their digital transformation and cloud journey to AWS, and specializes in streaming and search. Outside of work, he loves traveling, playing tennis, and cycling.
Karim Hammouda is a Specialist Solutions Architect for Analytics at AWS with a passion for data integration, data analysis, and BI. He works with AWS customers to design and build analytics solutions that contribute to their business growth. In his free time, he likes to watch TV documentaries and play video games with his son.
Read More