Amazon SageMaker Studio is the first fully integrated development environment (IDE) for machine learning (ML). It provides a single, web-based visual interface where you can perform all ML development steps required to prepare data, as well as build, train, and deploy models. We recently introduced the ability to visually browse and connect to Amazon EMR clusters right from the Studio notebook. Starting today, you can now monitor and debug your Spark jobs running on Amazon EMR from Studio notebooks with just a single click. Additionally, you can now discover, connect to, create, stop, and manage EMR clusters directly from Studio.
We demonstrate these newly introduced capabilities in this two-part post.
Analyzing, transforming, and preparing large amounts of data is a foundational step of any data science and ML workflow. Data workers such as data scientists and data engineers use Apache Spark, Hive, and Presto running on Amazon EMR for fast data preparation. Until today, these data workers could easily discover and connect to EMR clusters running in the same account as Studio but were unable to do so across accounts—a configuration common among several customer setups. Furthermore, when data workers needed to create EMR clusters tailored to their specific interactive workloads on demand, they had to switch interfaces to either request their administrator to create one or use detailed technical knowledge of DevOps to create it by themselves. This process was not only difficult and disruptive to their workflow, but also distracted data workers from focusing on their data preparation tasks. Consequently, although uneconomical, many customers kept persistent clusters running in anticipation of incoming workload regardless of active usage. Finally, monitoring and debugging Spark jobs running on Amazon EMR required setting up complex security rules and web proxies, adding significant friction to the data workers’ workflow.
Starting today, data workers can easily discover and connect to EMR clusters in single-account and cross-account configurations directly from Studio. Furthermore, you now have one-click access to the Spark UI to monitor and debug Spark jobs running on Amazon EMR right from Studio notebooks, which greatly simplifies your Spark debugging workflow. Finally, you can use the AWS Service Catalog to define and roll out preconfigured templates to select data workers to enable them to create EMR clusters right from Studio. You can fully control the organizational, security, compute, and networking guardrails to be adhered to when data workers use these templates. Data workers can visually browse through a set of templates made available to them, customize them for their specific workloads, create EMR clusters on demand, and stop them with just a few clicks in Studio. This feature considerably simplifies the data preparation workflow and enables you to more optimally use EMR clusters for interactive workloads from Studio.
In Part 1 of our series, we dive into the details of how DevOps administrators can use the AWS Service Catalog to define parameterized templates that data workers can use to create EMR clusters directly from the Studio interface. We provide an AWS CloudFormation template to create an AWS Service Catalog product for creating EMR clusters within an existing Amazon SageMaker domain, as well as a new CloudFormation template to stand up a SageMaker domain, Studio user profile, and Service Catalog product shared with that user so you can get started from scratch. As part of the solution, we utilize a single-click Spark UI interface to debug and monitor our ETL jobs. We use the transformed data to train and deploy an ML model using SageMaker training and hosting services.
As a follow-up, Part 2 provides a deep dive into cross-account setups. These multi-account setups are common amongst customers and are a best practice for many enterprise account setups, as mentioned in our AWS Well-Architected Framework.
Solution overview
We first describe how to communicate with Amazon EMR from Studio, as shown in the post Perform interactive data engineering and data science workflows from Amazon SageMaker Studio notebooks. In our solution, we utilize a SageMaker domain that has been configured with an elastic network interface through private VPC mode. That connected VPC is where we spin up our EMR clusters for this demo. For more information about the prerequisites, see our documentation.
The following diagram shows the complete user journey. A DevOps persona creates the Service Catalog product within a portfolio that is accessible to the Studio execution roles.
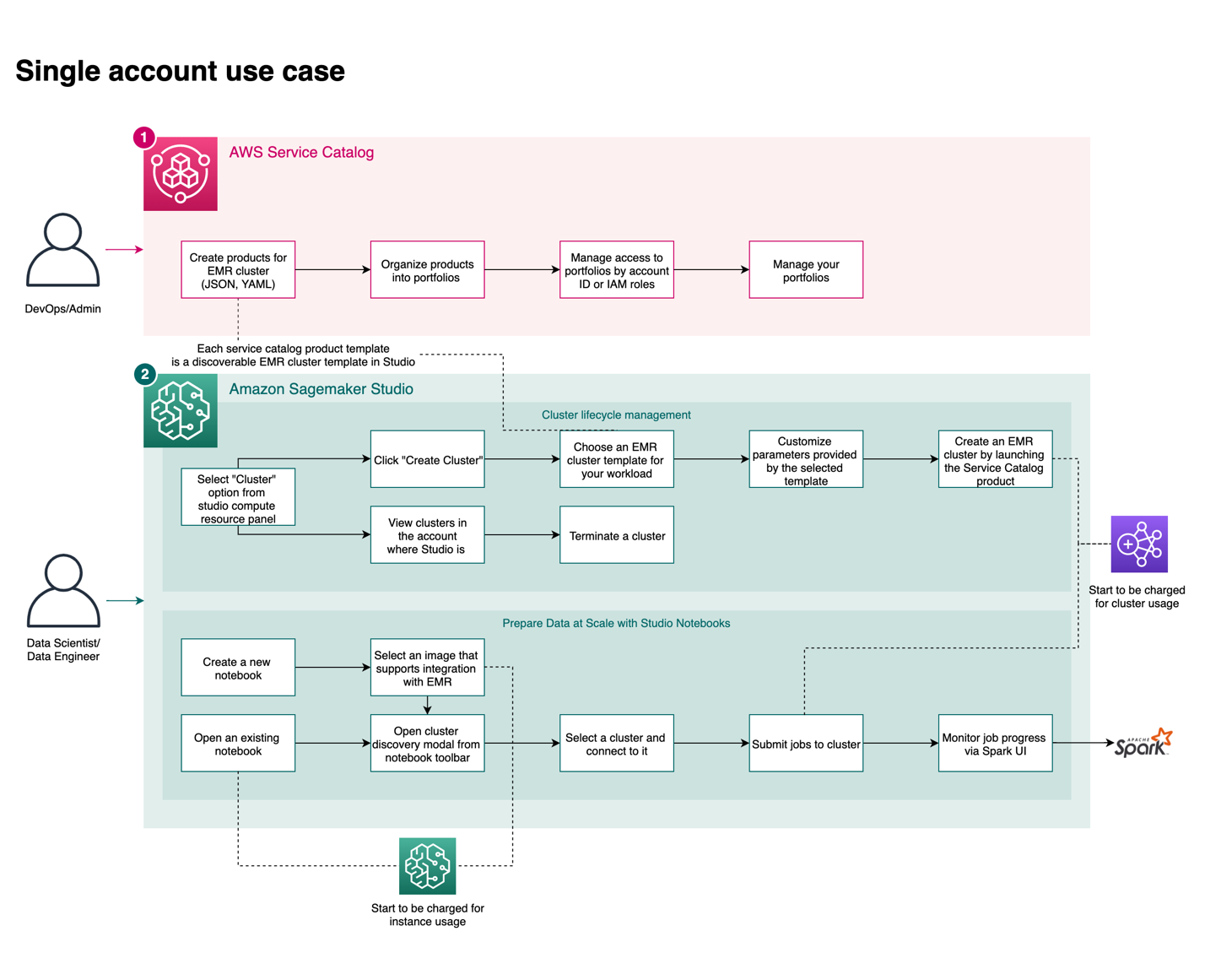
It’s important to note that you can use the full set of CloudFormation properties for Amazon EMR when creating templates that can be deployed though Studio. This means that you can enable Spot, auto scaling, and other popular configurations through your Service Catalog product.
You can parameterize the preset CloudFormation template (which creates the EMR cluster) so that end users can modify different aspects of the cluster to match their workloads. For example, the data scientist or data engineer may want to specify the number of core nodes on the cluster, and the creator of the template can specify AllowedValues
to set guardrails.
The following template parameters give some examples of commonly used parameters:
"Parameters": {
"EmrClusterName": {
"Type": "String",
"Description": "EMR cluster Name."
},
"CoreInstanceType": {
"Type": "String",
"Description": "Instance type of the EMR core nodes.",
"Default": "m5.xlarge",
"AllowedValues": [
"m5.xlarge",
"m3.2xlarge"
]
},
"CoreInstanceCount": {
"Type": "String",
"Description": "Number of core instances in the EMR cluster.",
"Default": "2",
"AllowedValues": [
"2",
"5",
"10"
]
},
"EmrReleaseVersion": {
"Type": "String",
"Description": "The release version of EMR to launch.",
"Default": "emr-5.33.1",
"AllowedValues": [
"emr-5.33.1",
"emr-6.4.0"
]
}
}
For the product to be visible within the Studio interface, we need to set the following tags on the Service Catalog product:
sagemaker:studio-visibility:emr true
Lastly, the CloudFormation template in the Service Catalog product must have the following mandatory stack parameters:
```
SageMakerProjectName:
Type: String
Description: Name of the project
SageMakerProjectId:
Type: String
Description: Service generated Id of the project
````
Both values for these parameters are automatically injected when the stack is launched, so you don’t need to fill them in. They’re part of the template because SageMaker projects are utilized as part of the integration between the Service Catalog and Studio.
The second part of the single-account user journey (as shown in the architecture diagram) is from the data worker’s perspective within Studio. As shown in the post Perform interactive data engineering and data science workflows from Amazon SageMaker Studio notebooks, Studio users can browse existing EMR clusters and seamlessly connect to them using Kerberos, LDAP, HTTP, or no-auth mechanisms. Now, you can also create new EMR clusters through provisioning of templates, as shown in the following architecture diagram.
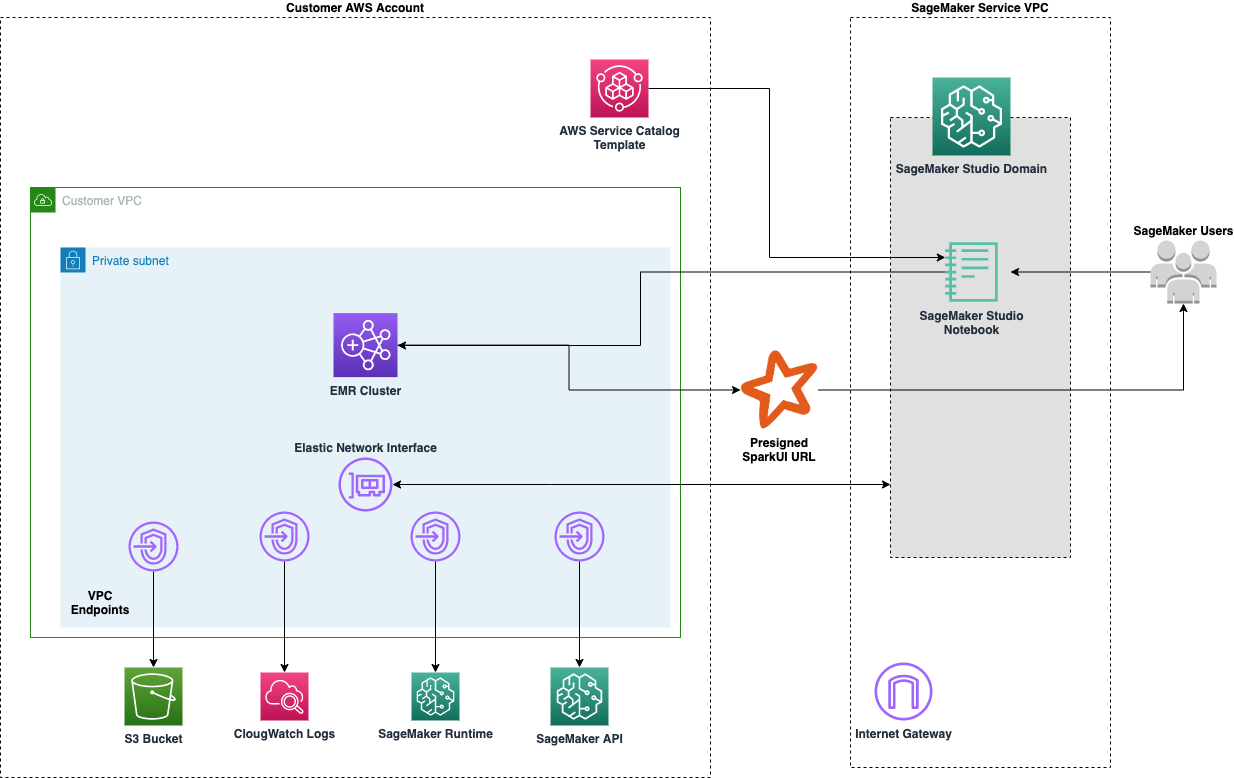
For Studio users to browse the available clusters, we need to attach an AWS Identity and Access Management (IAM) policy that permits Amazon EMR discoverability. For more information, see our existing documentation.
Deploy resources with AWS CloudFormation
For this post, we’ve provided two CloudFormation stacks to demonstrate the Studio and EMR capabilities found in our GitHub repository.
The first stack provides an end-to-end CloudFormation template that stands up a private VPC, a SageMaker domain attached to that VPC, and a SageMaker user with visibility to the pre-created Service Catalog product.
The second stack is intended for users with existing Studio private VPC setups who want to utilize a CloudFormation stack to deploy a Service Catalog product and make it visible to an existing SageMaker user.
You will be charged for Studio and Amazon EMR resources used when you launch the following stacks. For more information, see Amazon SageMaker Pricing and Amazon EMR pricing.
Follow the instructions in the cleanup sections at the end of this post to make sure that you don’t continue to be charged for these resources.
To launch the end-to-end stack, choose the stack for your desired Region.
ap-northeast-1 |
 |
ap-northeast-2 |
 |
ap-south-1 |
 |
ap-southeast-1 |
 |
ca-central-1 |
 |
eu-central-1 |
 |
eu-north-1 |
 |
eu-west-1 |
 |
eu-west-2 |
 |
eu-west-3 |
 |
sa-east-1 |
 |
us-east-1 |
 |
us-east-2 |
 |
us-west-1 |
 |
us-west-2 |
 |
This stack is intended to be a from-scratch setup and therefore the admin doesn’t need to launch this stack to input specific parameters related to their account. However, because our subsequent Amazon EMR stack uses the outputs of this stack, we need to provide a deterministic stack name so that it can be referenced. The preceding link provides the stack name as expected by this demo and it should not be modified.
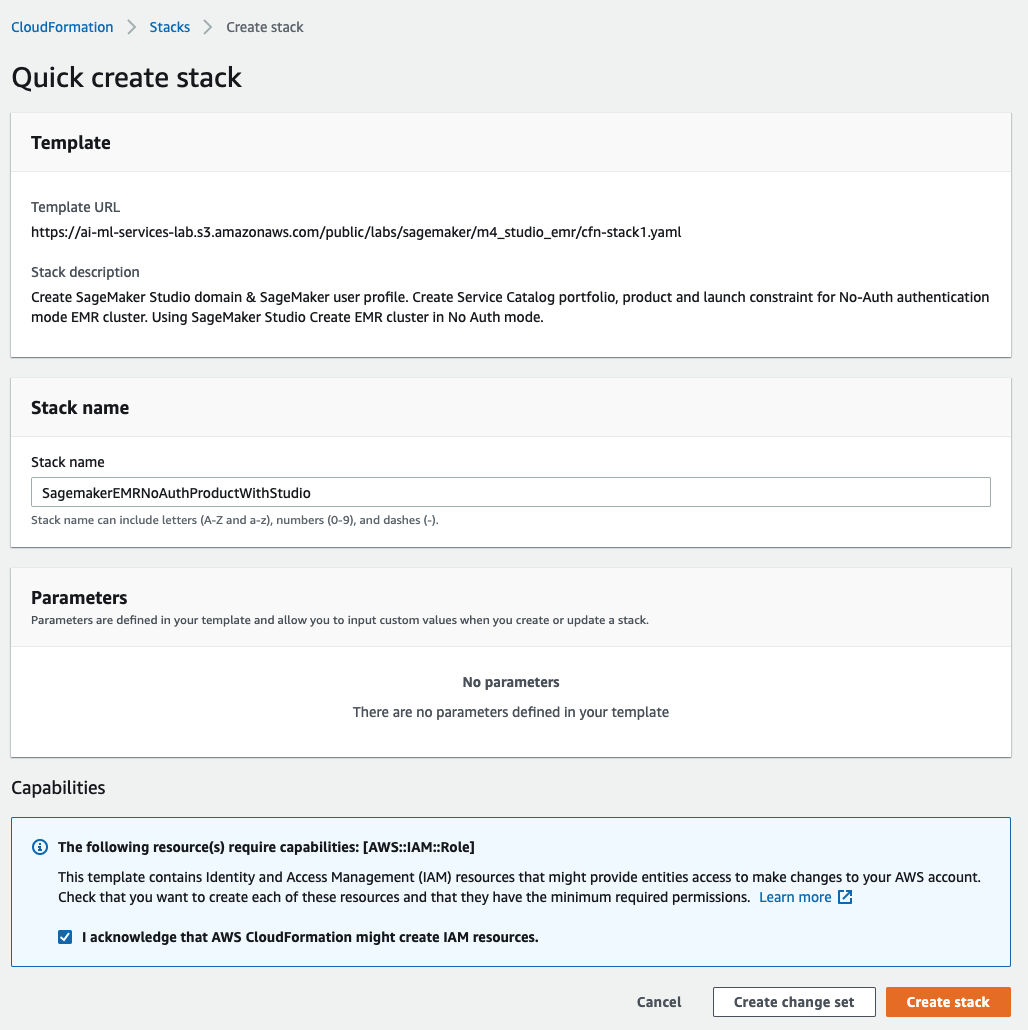
After we launch the stack, we can see that our Studio domain has been created, and studio-user
is attached to an execution role that was created with visibility to our Service Catalog product.
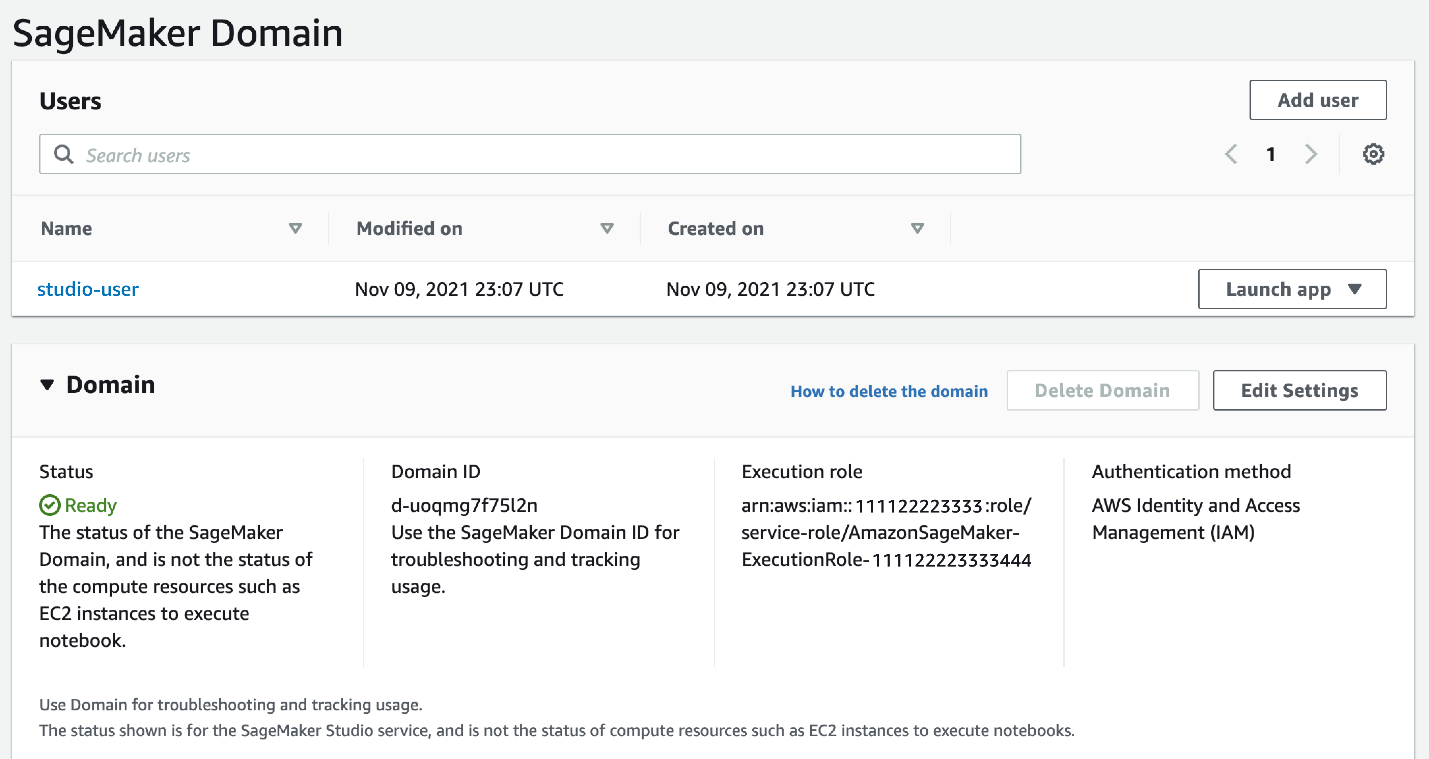
If you choose to run the end-to-end stack, skip the following existing domain information.
If you have an existing domain stack, launch the following stack in your preferred Region.
ap-northeast-1 |
 |
ap-northeast-2 |
 |
ap-south-1 |
 |
ap-southeast-1 |
 |
ca-central-1 |
 |
eu-central-1 |
 |
eu-north-1 |
 |
eu-west-1 |
 |
eu-west-2 |
 |
eu-west-3 |
 |
sa-east-1 |
 |
us-east-1 |
 |
us-east-2 |
 |
us-west-1 |
 |
us-west-2 |
 |
Because this stack is intended for accounts with existing domains that are attached to a private subnet, the admin fills in the required parameters during the stack launch. This is intended to simplify the experience for downstream data workers, and we abstract this networking information away from them.
Again, because the subsequent Amazon EMR stack utilizes the parameters the admin inputs here, we need to provide a deterministic stack name so that they can be referenced. The preceding stack link provides the stack name as expected by this demo.
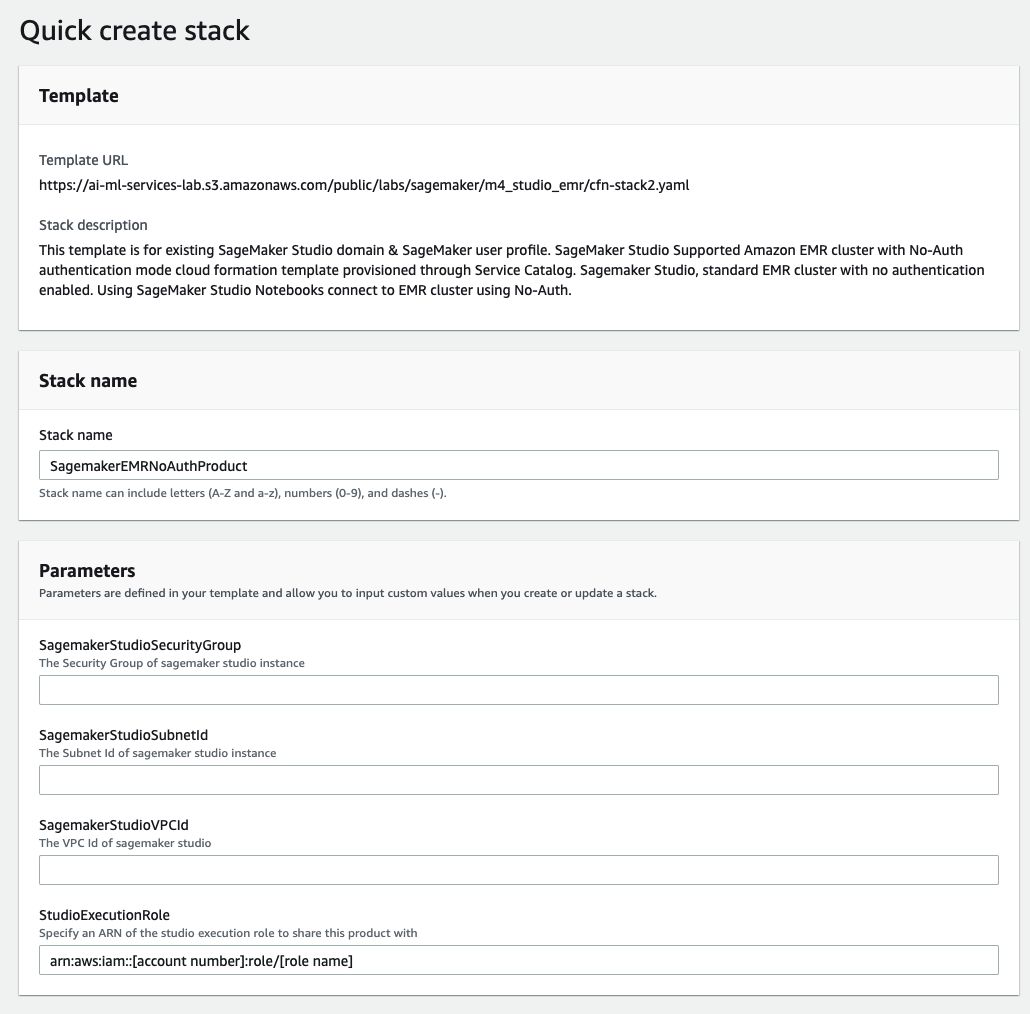
If you’re using the second stack with an existing domain and users, you need to complete one additional step to make sure the Spark UI functionality is available and that your user can browse EMR clusters and spin them up and down. Simply attach the following policy to the SageMaker execution role that you input as a parameter, providing the Region and account ID as needed:
{
"Version": "2012-10-17",
"Statement": [
{
"Sid": "AllowPresignedUrl",
"Effect": "Allow",
"Action": [
"elasticmapreduce:DescribeCluster",
"elasticmapreduce:ListInstanceGroups",
"elasticmapreduce:CreatePersistentAppUI",
"elasticmapreduce:DescribePersistentAppUI",
"elasticmapreduce:GetPersistentAppUIPresignedURL",
"elasticmapreduce:GetOnClusterAppUIPresignedURL"
],
"Resource": [
"arn:aws:elasticmapreduce:<region>:<account-id>:cluster/*"
]
},
{
"Sid": "AllowClusterDetailsDiscovery",
"Effect": "Allow",
"Action": [
"elasticmapreduce:DescribeCluster",
"elasticmapreduce:ListInstanceGroups"
],
"Resource": [
"arn:aws:elasticmapreduce:<region>:<account-id>:cluster/*"
]
},
{
"Sid": "AllowClusterDiscovery",
"Effect": "Allow",
"Action": [
"elasticmapreduce:ListClusters"
],
"Resource": "*"
},
{
"Sid": "AllowSagemakerProjectManagement",
"Effect": "Allow",
"Action": [
"sagemaker:CreateProject",
"sagemaker:DeleteProject"
],
"Resource": "arn:aws:sagemaker:<region>:<account-id>:project/*"
},
{
"Sid": "AllowEMRTemplateDiscovery",
"Effect": "Allow",
"Action": [
"servicecatalog:SearchProducts"
],
"Resource": "*"
}
]
}
Review the AWS Service Catalog product
After you launch your stack, you can see that an IAM role was created as a launch constraint, which provisions our EMR cluster. Both stacks also generated the AWS Service Catalog product and the association to our Studio execution role.
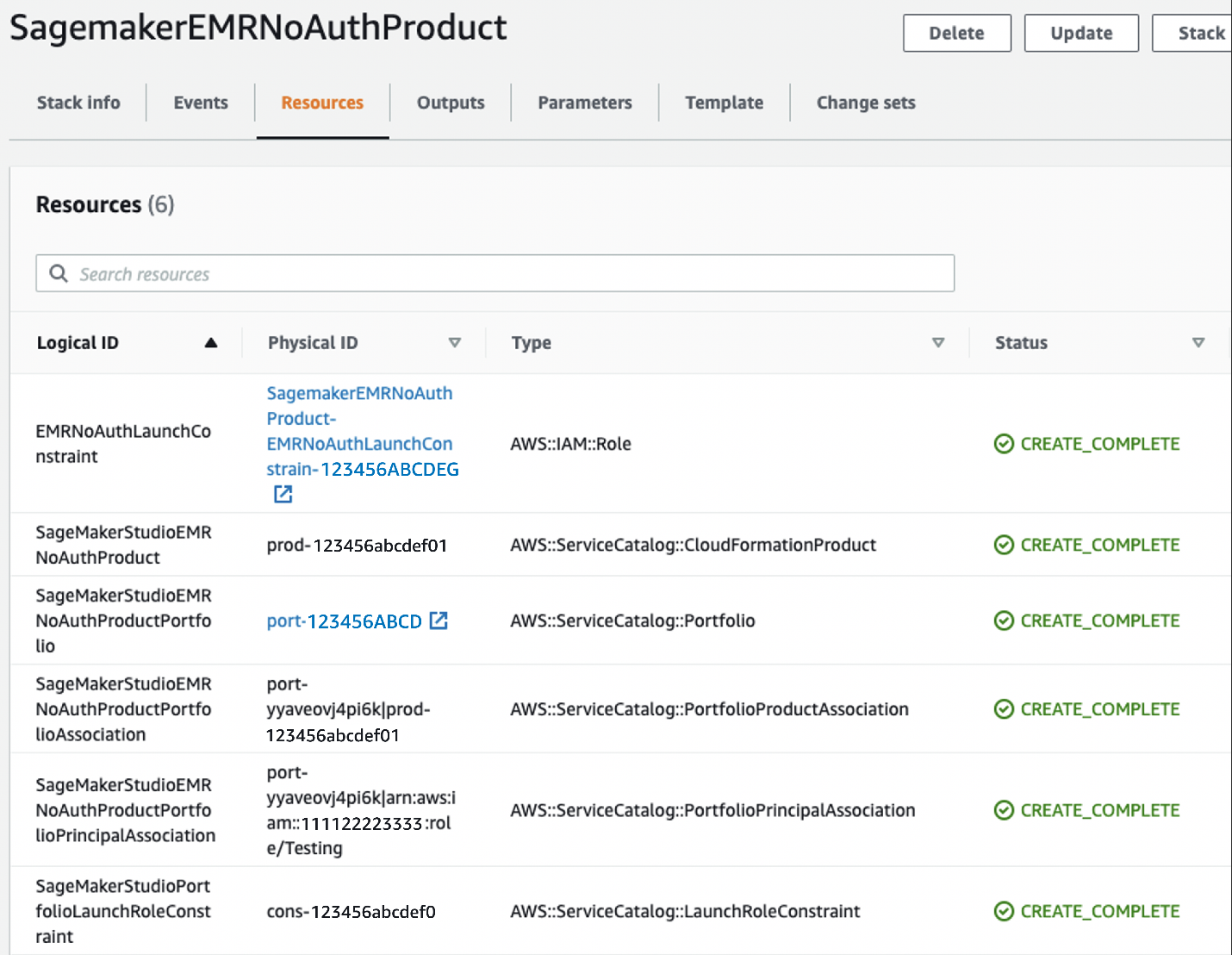
On the list of AWS Service Catalog products, we see the product name, which is later visible from the Studio interface.
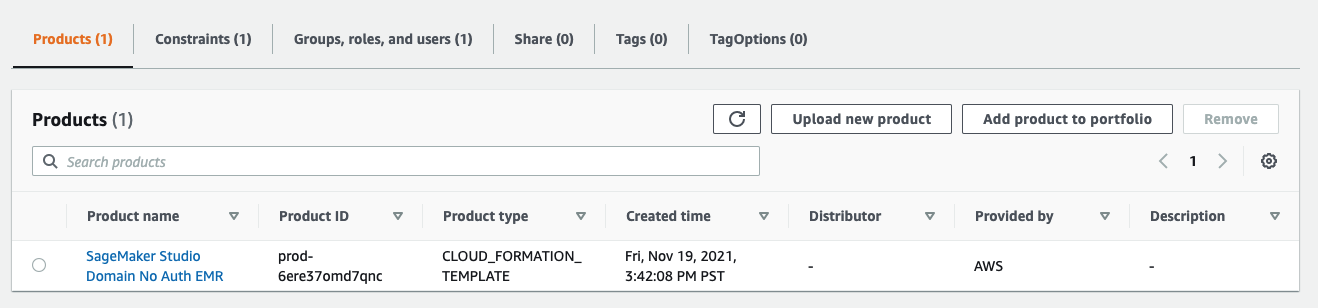
This product has a launch constraint that governs the role that creates the cluster.
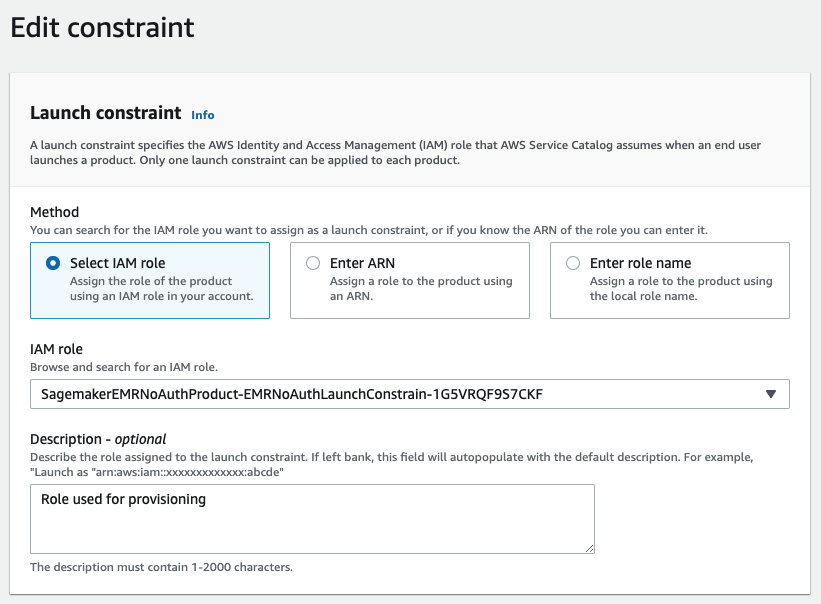
Note that our product has been tagged appropriately for visibility within the Studio interface.

If we look into the template that was provisioned, we can see the CloudFormation template that initializes our cluster, creates the Hive tables, and loads them with the demo data.
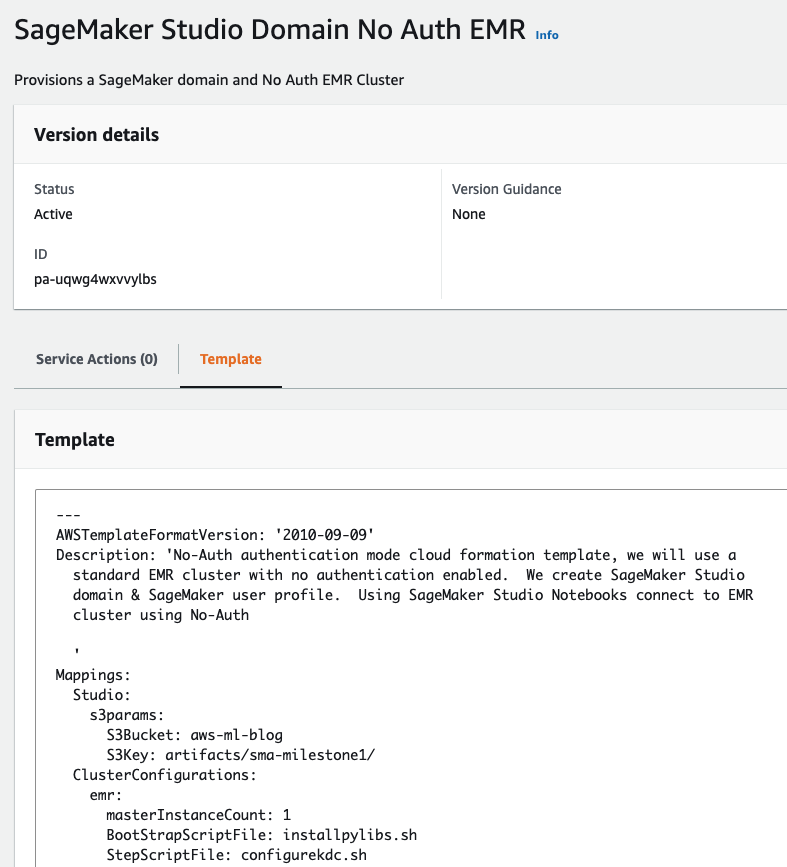
Create an EMR cluster from Studio
After the Service Catalog product has been created in your account through the stack that fits your setup, we can continue the demonstration from the data worker’s prospective.
- Launch a Studio notebook.
- Under SageMaker resources, choose Clusters on the drop-down menu.
- Choose Create cluster.
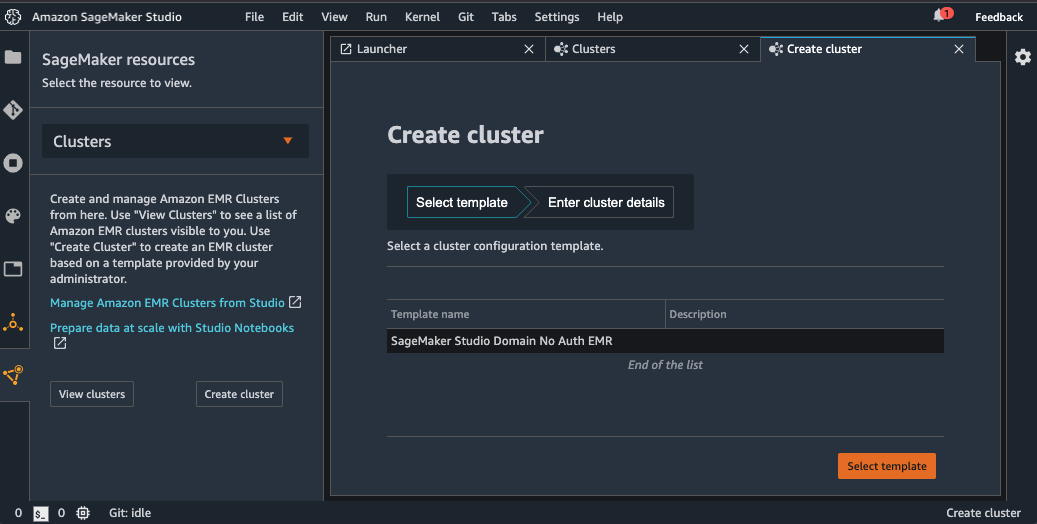
- From the available templates, choose the provisioned template SageMaker Studio Domain No Auth EMR.
- Enter your desired configurable parameters and choose Create cluster.
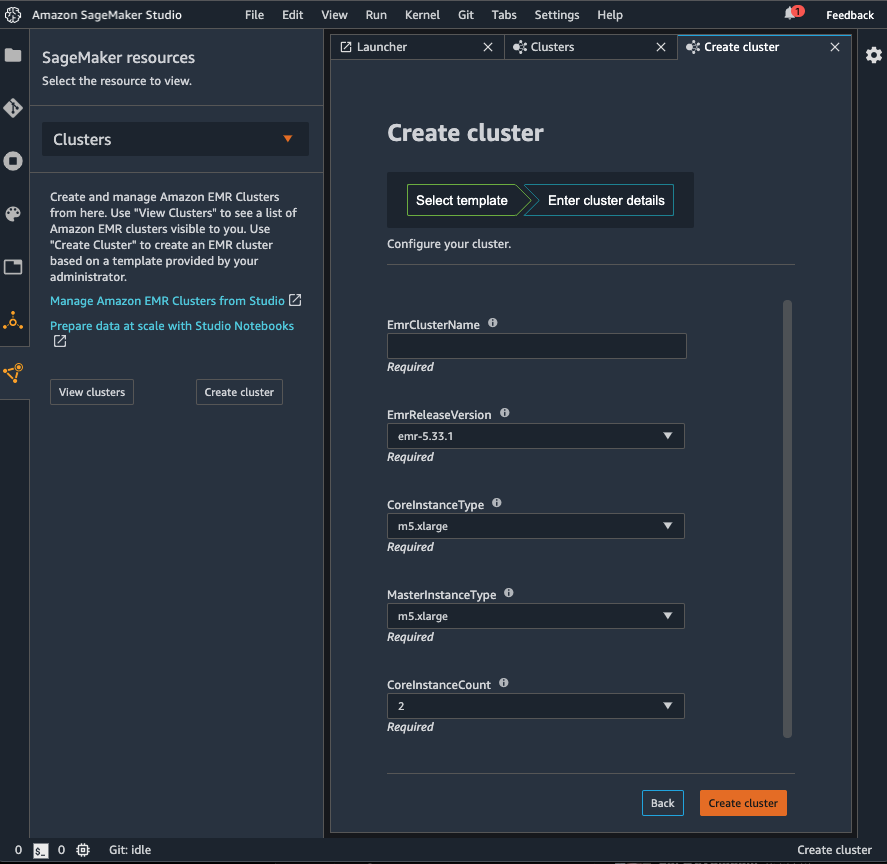
You can now monitor the deployment on the Clusters management tab. As part of the template, our cluster instantiates Hive tables with some data that we can use as part of our example.
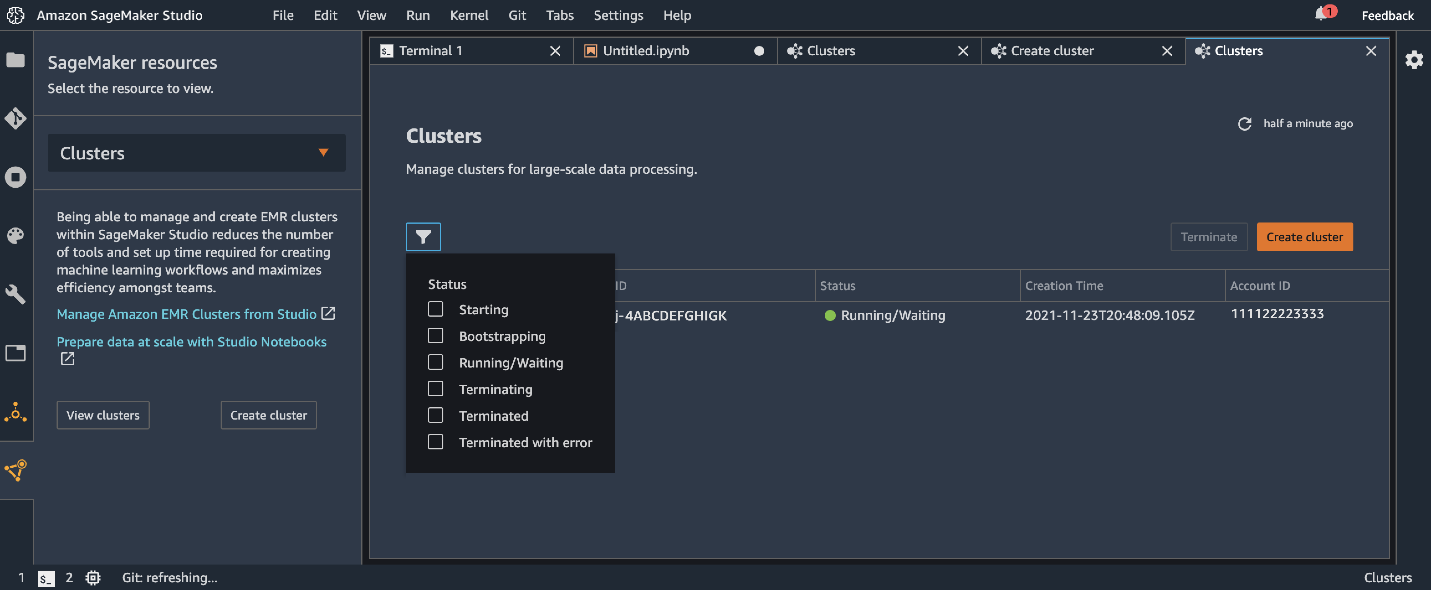
Connect to an EMR Cluster from Studio
After your cluster has entered the Running/Waiting status, you can connect to the cluster in the same way as was described in the post Perform interactive data engineering and data science workflows from Amazon SageMaker Studio notebooks.
First, we clone our GitHub repo.
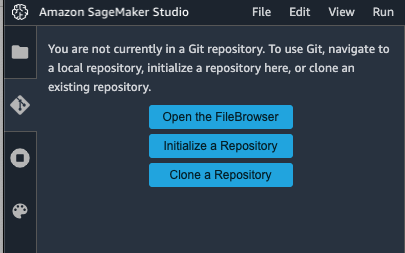
As of this writing, only a subset of kernels support connecting to an existing EMR cluster. For the full list of supported kernels, and information on building your own Studio images with connectivity capabilities; see our documentation. For this post, we use the SparkMagic kernel from the PySpark image and run the smstudio-pyspark-hive-sentiment-analysis.ipynb
notebook from the repository.
For simplicity, the template that we deploy uses a no-auth authentication mechanism, but as shown in our previous post, this works seamlessly with Kerberos, LDAP, and HTTP auth as well.
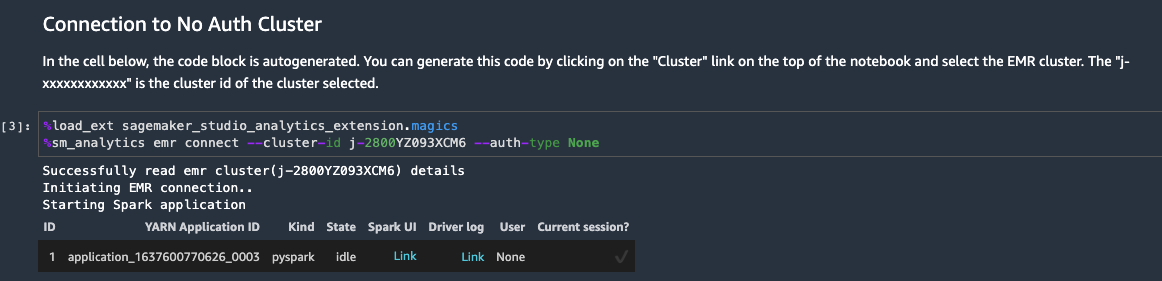
After a connection is made, there is a hyperlink for the Spark UI, which we use to debug and monitor our demonstration. We dive into the technical details later in the post, but you can open this in a new tab now.
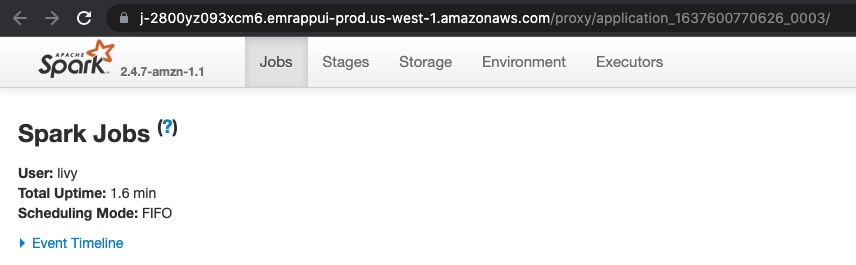
Next, we show the functionality from our previous post where we can query the newly instantiated tables using PySpark, write transformed data to Amazon Simple Storage Service (Amazon S3), and launch SageMaker training and hosting jobs all from the same smstudio-pyspark-hive-sentiment-analysis.ipynb
notebook.
The following screenshots demonstrate preprocessing the data.
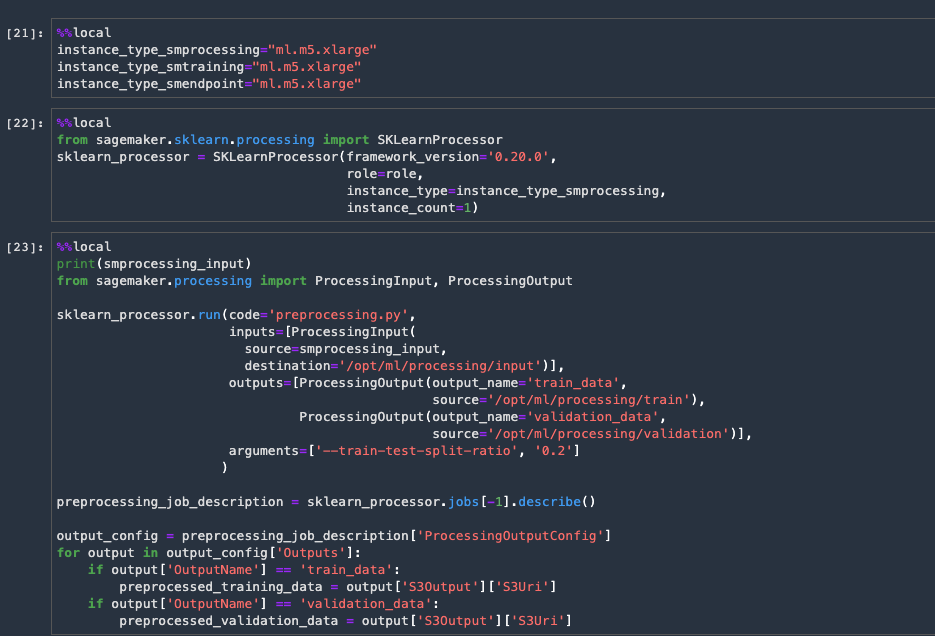
The following screenshots show the process of training the model.
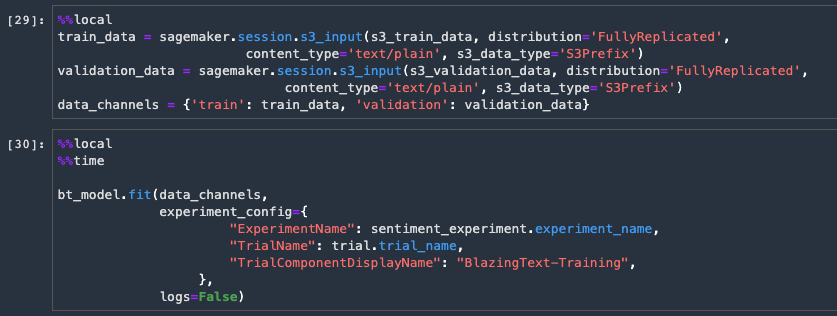
The following screenshots demonstrate deploying the model.
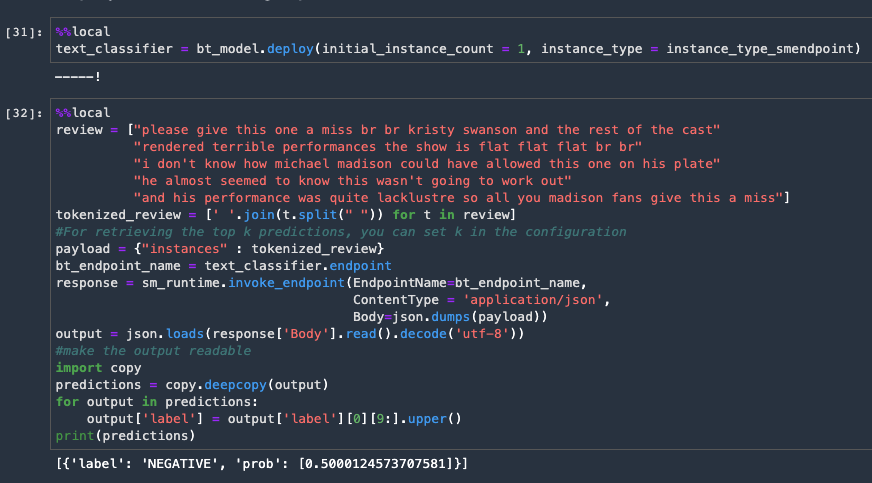
Monitor and debug with the Spark UI
As mentioned before, the process for viewing the Spark UI has been greatly simplified, and a presigned URL is generated at the time of connection to your cluster. Each pre-signed URL has a time to live of 5 minutes.
You can use this UI for monitoring your Spark run and shuffling, among other things. For more information, see the documentation.
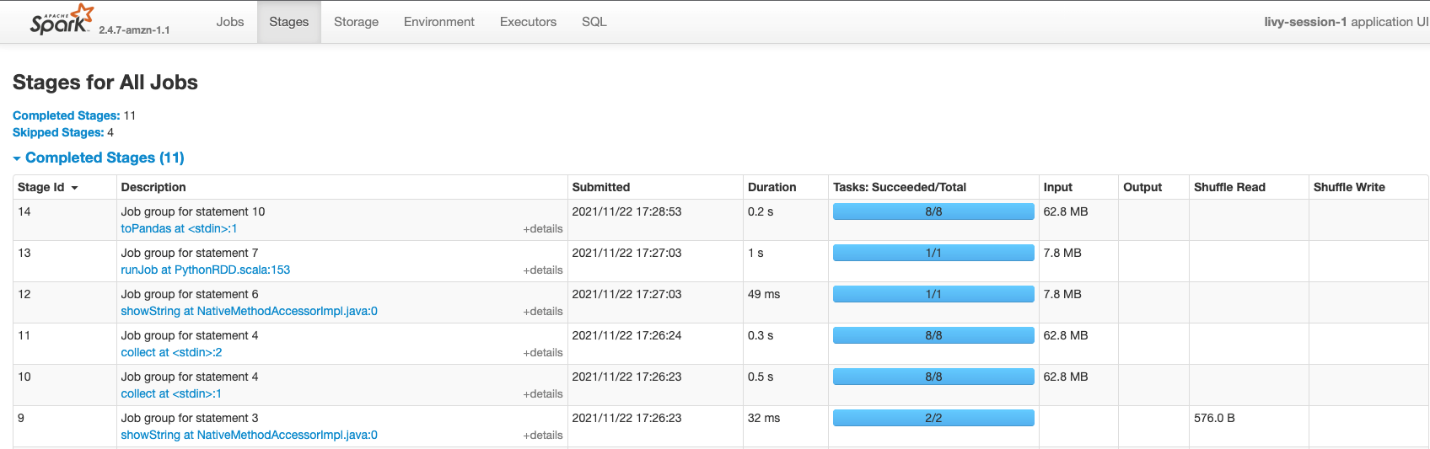
Stop an EMR cluster from Studio
After we’re done with our analysis and model building, we can use the Studio interface to stop our cluster. Because this runs DELETE STACK under the hood, users only have access to stop clusters that were launched using provisioned Service Catalog templates and can’t stop existing clusters that were created outside of Studio.
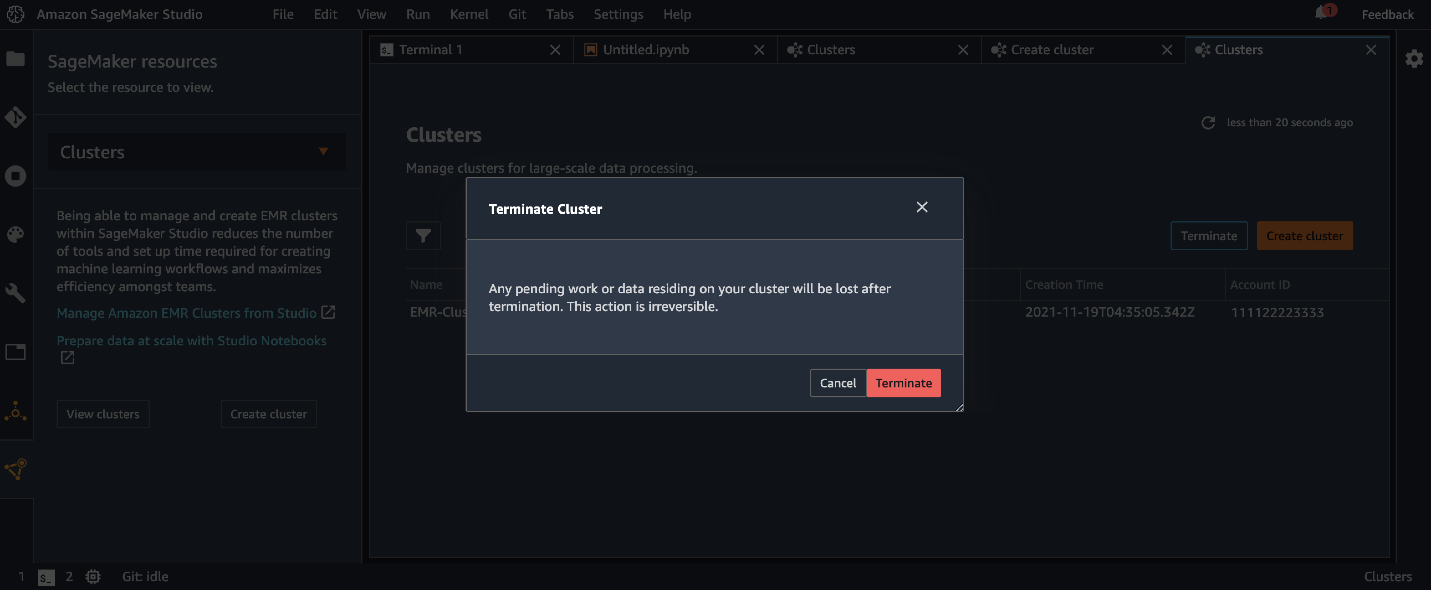
Clean up the end-to-end stack
If you deployed the end-to-end stack, complete the following steps to clean up resources deployed for this solution:
- Stop your cluster, as shown in the previous section.
This also deletes the S3 bucket, so you should copy the contents in the bucket to a backup location if you want to retain the data for later use.
- On the Studio console, choose your user name (
studio-user
).
- Delete all the apps listed under Apps by choosing Delete app.
- Wait until the status shows as
Completed
.
Next, you delete your Amazon Elastic File System (Amazon EFS) volume.
- On the Amazon EFS console, delete the file system that SageMaker created.
You can confirm it’s the correct volume by choosing the file system ID and confirming the tag is ManagedByAmazonSageMakerResource
.
Finally, you delete the CloudFormation template.
- On the AWS CloudFormation console, choose Stacks.
- Select the stack you deployed for this solution.
- Choose Delete.
Clean up the existing domain stack
The second stack has a simpler cleanup because we’re leaving the Studio resources in place as they were prior to starting this tutorial.
- Stop your cluster as shown in the previous cleanup instructions.
- Remove the attached policy you added to the SageMaker execution role that permitted Amazon EMR browsing and
PresignedURL
access.
- On the AWS CloudFormation console, choose Stacks.
- Select the stack you deployed for this solution.
- Choose Delete.
Conclusion
In this post, we demonstrated a unified notebook-centric experience to create and manage EMR clusters, run analytics on those clusters, and train and deploy SageMaker models, all from the Studio interface. We also showed a one-click interface for debugging and monitoring Amazon EMR jobs through the Spark UI. We encourage you to try out this new functionality in Studio yourself, and check out Part 2 of this post, which dives deep how data workers can discover, connect, create, and stop clusters in a multi-account setup.
About the Authors
Sumedha Swamy is a Principal Product Manager at Amazon Web Services. He leads SageMaker Studio team to build it into the IDE of choice for interactive data science and data engineering workflows. He has spent the past 15 years building customer-obsessed consumer and enterprise products using Machine Learning. In his free time, he likes photographing the amazing geology of the American Southwest.
Prateek Mehrotra is a Senior SDE working for SageMaker Studio at Amazon Web Services. He is focused on building interactive ML solutions which simplify usability by abstracting away complexity. In his spare time, Prateek enjoys spending time with his family and likes to explore the world with them.
Sriharsha M S is an AI/ML specialist solutions architect in the Strategic Specialist team at Amazon Web Services. He works with strategic AWS customers who are taking advantage of AI/ML to solve complex business problems. He provides technical guidance and design advice to implement AI/ML applications at scale. His expertise spans application architecture, big data, analytics, and machine learning.
Sean Morgan is a Senior ML Solutions Architect at AWS. He has experience in the semiconductor and academic research fields, and uses his experience to help customers reach their goals on AWS. In his free time Sean is an activate open source contributor/maintainer and is the special interest group lead for TensorFlow Addons.
Ruchir Tewari is a Senior Solutions Architect specializing in security and is a member of the ML TFC. For several years he has helped customers build secure architectures for a variety of hybrid, big data and AI/ML applications. He enjoys spending time with family, music and hikes in nature.
Luna Wang is a UX designer at AWS who has a background in computer science and interaction design. She is passionate about building customer-obsessed products and solving complex technical and business problems by using design methods. She is now working with a cross-functional team to build a set of new capabilities for interactive ML in SageMaker Studio.
Read More