Industrial companies have been collecting a massive amount of time-series data about operating processes, manufacturing production lines, and industrial equipment. You might store years of data in historian systems or in your factory information system at large. Whether you’re looking to prevent equipment breakdown that would stop a production line, avoid catastrophic failures in a power generation facility, or improve end product quality by adjusting your process parameters, having the ability to process time-series data is a challenge that modern cloud technologies are up to. However, everything is not about the cloud itself: your factory edge capability must also allow you to stream the appropriate data to the cloud (bandwidth, connectivity, protocol compatibility, putting data in context, and more).
What if you had a frugal way to qualify your equipment health with little data? This can definitely help leverage robust and easier-to-maintain edge-to-cloud blueprints. In this post, we focus on a tactical approach industrial companies can use to help reduce the impact of machine breakdowns by reducing how unpredictable they are.
Machine failures are often addressed by either reactive action (stop the line and repair) or costly preventive maintenance where you have to build the proper replacement parts inventory and schedule regular maintenance activities. Skilled machine operators are the most valuable assets in such settings: years of experience allow them to develop a fine knowledge of how the machinery should operate. They become expert listeners, and can to detect unusual behavior and sounds in rotating and moving machines. However, production lines are becoming more and more automated, and augmenting these machine operators with AI-generated insights is a way to maintain and develop the fine expertise needed to prevent reactive-only postures when dealing with machine breakdowns.
In this post, we compare and contrast two different approaches to identify a malfunctioning machine, providing you have sound recordings from its operation. We start by building a neural network based on an autoencoder architecture and then use an image-based approach where we feed images of sound (namely spectrograms) to an image-based automated machine learning (ML) classification feature.
Services overview
Amazon SageMaker is a fully managed service that provides every developer and data scientist with the ability to build, train, and deploy ML models quickly. SageMaker removes the heavy lifting from each step of the ML process to make it easier to develop high-quality models.
Amazon Rekognition Custom Labels is an automated ML service that enables you to quickly train your own custom models for detecting business-specific objects from images. For example, you can train a custom model to classify unique machine parts in an assembly line or to support visual inspection at quality gates to detect surface defects.
Amazon Rekognition Custom Labels builds off the existing capabilities of Amazon Rekognition, which is already trained on tens of millions of images across many categories. Instead of thousands of images, you simply need to upload a small set of training images (typically a few hundred images) that are specific to your use case. If you already labeled your images, Amazon Rekognition Custom Labels can begin training in just a few clicks. If not, you can label them directly within the labeling tool provided by the service or use Amazon SageMaker Ground Truth.
After Amazon Rekognition trains from your image set, it can produce a custom image analysis model for you in just a few hours. Amazon Rekognition Custom Labels automatically loads and inspects the training data, selects the right ML algorithms, trains a model, and provides model performance metrics. You can then use your custom model via the Amazon Rekognition Custom Labels API and integrate it into your applications.
Solution overview
In this use case, we use sounds recorded in an industrial environment to perform anomaly detection on industrial equipment. After the dataset is downloaded, it takes roughly an hour and a half to go through this project from start to finish.
To achieve this, we explore and leverage the Malfunctioning Industrial Machine Investigation and Inspection (MIMII) dataset for anomaly detection purposes. It contains sounds from several types of industrial machines (valves, pumps, fans, and slide rails). For this post, we focus on the fans. For more information about the sound capture procedure, see MIMII Dataset: Sound Dataset for Malfunctioning Industrial Machine Investigation and Inspection.
In this post, we implement the area in red of the following architecture. This is a simplified extract of the Connected Factory Solution with AWS IoT. For more information, see Connected Factory Solution based on AWS IoT for Industry 4.0 success.
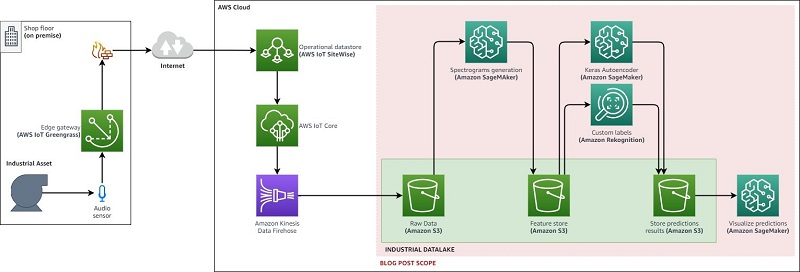
We walk you through the following steps using the Jupyter notebooks provided with this post:
- We first focus on data exploration to get familiar with sound data. This data is particular time-series data, and exploring it requires specific approaches.
- We then use SageMaker to build an autoencoder that we use as a classifier to discriminate between normal and abnormal sounds.
- We take on a more novel approach in the last part of this post: we transform the sound files into spectrogram images and feed them directly to an image classifier. We use Amazon Rekognition Custom Labels to perform this classification task and leverage SageMaker for the data preprocessing and to drive the Amazon Rekognition Custom Labels training and evaluation process.
Both approaches require an equal amount of effort to complete. Although the models obtained in the end aren’t comparable, this gives you an idea of how much of a kick-start you may get when using an applied AI service.
Introducing the machine sound dataset
You can use the data exploration work available in the first companion notebook from the GitHub repo. The first thing we do is plot the waveforms of normal and abnormal signals (see the following screenshot).
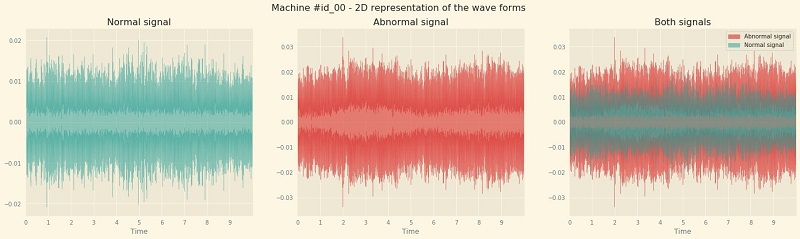
From there, you see how to leverage the short Fourier transformation to build a spectrogram of these signals.
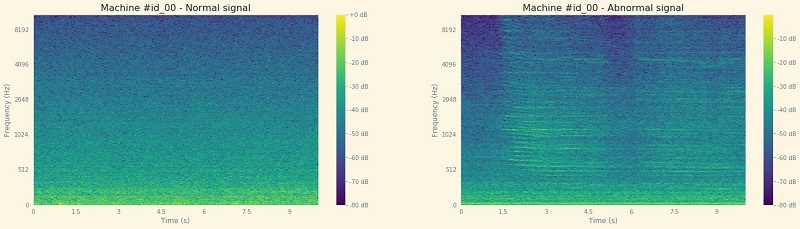
These images have interesting features; this is exactly the kind of features that a neural network can try to uncover and structure. We now build two types of feature extractors based on this data exploration work and feed them to different types of architectures.
Building a custom autoencoder architecture
The autoencoder architecture is a neural network with the same number of neurons in the input and the output layers. This kind of architecture learns to generate the identity transformation between inputs and outputs. The second notebook of our series goes through these different steps:
- To feed the spectrogram to an autoencoder, build a tabular dataset and upload it to Amazon Simple Storage Service (Amazon S3).
- Create a TensorFlow autoencoder model and train it in script mode by using the TensorFlow/Keras existing container.
- Evaluate the model to obtain a confusion matrix highlighting the classification performance between normal and abnormal sounds.
Building the dataset
For this post, we use the librosa library, which is a Python package for audio analysis. A features extraction function based on the steps to generate the spectrogram described earlier is central to the dataset generation process. This feature extraction function is in the sound_tools.py library.
We train our autoencoder only on the normal signals: we want our model to learn how to reconstruct these signals (learning the identity transformation). The main idea is to leverage this for classification later; when we feed this trained model with abnormal sounds, the reconstruction error is a lot higher than when trying to reconstruct normal sounds. We use an error threshold to discriminate abnormal and normal sounds.
Creating the autoencoder
To build our autoencoder, we use Keras and assemble a simple autoencoder architecture with three hidden layers:
from tensorflow.keras import Input
from tensorflow.keras.models import Model
from tensorflow.keras.layers import Dense
def autoencoder_model(input_dims):
inputLayer = Input(shape=(input_dims,))
h = Dense(64, activation="relu")(inputLayer)
h = Dense(64, activation="relu")(h)
h = Dense(8, activation="relu")(h)
h = Dense(64, activation="relu")(h)
h = Dense(64, activation="relu")(h)
h = Dense(input_dims, activation=None)(h)
return Model(inputs=inputLayer, outputs=h)
We put this in a training script (model.py) and use the SageMaker TensorFlow estimator to configure our training job and launch the training:
tf_estimator = TensorFlow(
base_job_name='sound-anomaly',
entry_point='model.py',
source_dir='./autoencoder/',
role=role,
instance_count=1,
instance_type='ml.p3.2xlarge',
framework_version='2.2',
py_version='py37',
hyperparameters={
'epochs': 30,
'batch-size': 512,
'learning-rate': 1e-3,
'n_mels': n_mels,
'frame': frames
},
debugger_hook_config=False
)
tf_estimator.fit({'training': training_input_path})
Training over 30 epochs takes a few minutes on a p3.2xlarge instance. At this stage, this costs you a few cents. If you plan to use a similar approach on the whole MIMII dataset or use hyperparameter tuning, you can further reduce this training cost by using Managed Spot Training. For more information, see Amazon SageMaker Spot Training Examples.
Evaluating the model
We now deploy the autoencoder behind a SageMaker endpoint:
tf_endpoint_name = 'sound-anomaly-'+time.strftime("%Y-%m-%d-%H-%M-%S", time.gmtime())
tf_predictor = tf_estimator.deploy(
initial_instance_count=1,
instance_type='ml.c5.large',
endpoint_name=tf_endpoint_name
)
This operation creates a SageMaker endpoint that continues to incur costs as long as it’s active. Don’t forget to shut it down at the end of this experiment.
Our test dataset has an equal share of normal and abnormal sounds. We loop through this dataset and send each test file to this endpoint. Because our model is an autoencoder, we evaluate how good the model is at reconstructing the input. The higher the reconstruction error, the greater the chance that we have identified an anomaly. See the following code:
y_true = test_labels
reconstruction_errors = []
for index, eval_filename in tqdm(enumerate(test_files), total=len(test_files)):
# Load signal
signal, sr = sound_tools.load_sound_file(eval_filename)
# Extract features from this signal:
eval_features = sound_tools.extract_signal_features(
signal,
sr,
n_mels=n_mels,
frames=frames,
n_fft=n_fft,
hop_length=hop_length
)
# Get predictions from our autoencoder:
prediction = tf_predictor.predict(eval_features)['predictions']
# Estimate the reconstruction error:
mse = np.mean(np.mean(np.square(eval_features - prediction), axis=1))
reconstruction_errors.append(mse)
The following plot shows that the distribution of the reconstruction error for normal and abnormal signals differs significantly. The overlap between these histograms means we have to compromise between the metrics we want to optimize for (fewer false positives or fewer false negatives).
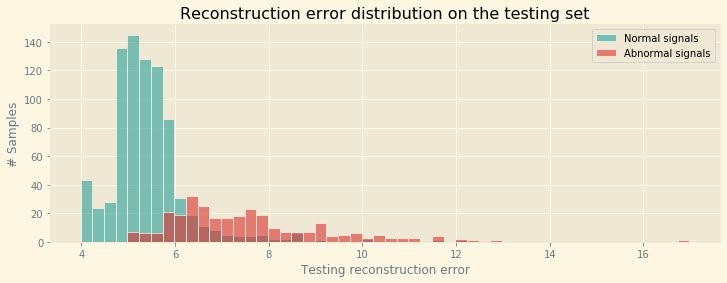
Let’s explore the recall-precision tradeoff for a reconstruction error threshold varying between 5.0–10.0 (this encompasses most of the overlap we can see in the preceding plot). First, let’s visualize how this threshold range separates our signals on a scatter plot of all the testing samples.
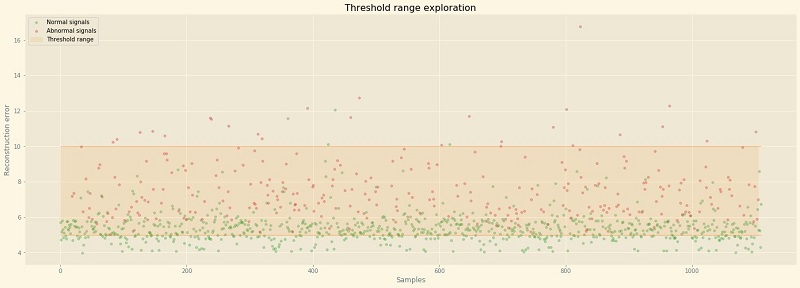
If we plot the number of samples flagged as false positives and false negatives, we can see that the best compromise is to use a threshold set around 6.3 for the reconstruction error (assuming we’re not looking at minimizing either the false positive or false negatives occurrences).
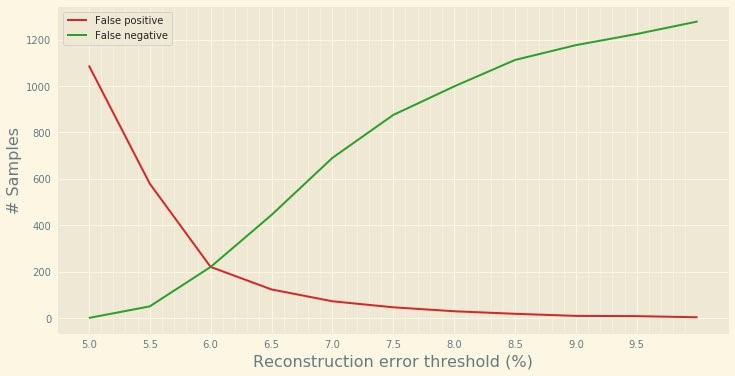
For this threshold (6.3), we obtain the following confusion matrix.
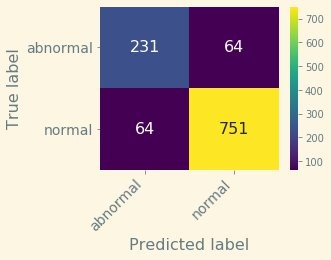
The metrics associated to this matrix are as follows:
- Precision – 92.1%
- Recall – 92.1%
- Accuracy – 88.5%
- F1 score – 92.1%
Cleaning up
Let’s not forget to delete our endpoint to prevent any additional costs by using the delete_endpoint()
API.
Autoencoder improvement and further exploration
The spectrogram approach requires defining the spectrogram square dimensions (the number of Mel cell defined in the data exploration notebook), which is a heuristic. In contrast, deep learning networks with a CNN encoder can learn the best representation to perform the task at hand (anomaly detection). The following are further steps to investigate to improve on this first result:
- Experimenting with several more or less complex autoencoder architectures, training for a longer time, performing hyperparameter tuning with different optimizers, or tuning the data preparation sequence (sound discretization parameters).
- Leveraging high-resolution spectrograms and feeding them to a CNN encoder to uncover the most appropriate representation of the sound.
- Using an end-to-end model architecture with an encoder-decoder that have been known to give good results on waveform datasets.
- Using deep learning models with multi-context temporal and channel (eight microphones) attention weights.
- Using time-distributed 2D convolution layers to encode features across the eight channels. You could feed these encoded features as sequences across time steps to an LSTM or GRU layer. From there, multiplicative sequence attention weights can be learnt on the output sequence from the RNN layer.
- Exploring the appropriate image representation for multi-variate time-series signals that aren’t waveform. You could replace spectrograms with Markov transition fields, recurrence plots, or network graphs to achieve the same goals for non-sound time-based signals.
Using Amazon Rekognition Custom Labels
For our second approach, we feed the spectrogram images directly into an image classifier. The third notebook of our series goes through these different steps:
- Build the datasets. For this use case, we just use images, so we don’t need to prepare tabular data to feed into an autoencoder. We then upload them to Amazon S3.
- Create an Amazon Rekognition Custom Labels project:
- Associate the project with the training data, validation data, and output locations.
- Train a project version with these datasets.
- Start the model. This provisions an endpoint and deploys the model behind it. We can then do the following:
- Query the endpoint for inference for the validation and testing datasets.
- Evaluate the model to obtain a confusion matrix highlighting the classification performance between normal and abnormal sounds.
Building the dataset
Previously, we had to train our autoencoder on only normal signals. In this use case, we build a more traditional split of training and testing datasets. Based on the fans sound database, this yields the following:
- 4,390 signals for the training dataset, including 3,210 normal signals and 1,180 abnormal signals
- 1,110 signals for the testing dataset, including 815 normal signals and 295 abnormal signals
We generate and store the spectrogram of each signal and upload them in either a train or test bucket.
Creating an Amazon Rekognition Custom Labels model
The first step is to create a project with the Rekognition Custom Labels boto3 API:
# Initialization, get a Rekognition client:
PROJECT_NAME = 'sound-anomaly-detection'
reko_client = boto3.client("rekognition")
# Let's try to create a Rekognition project:
try:
project_arn = reko_client.create_project(ProjectName=PROJECT_NAME)['ProjectArn']
# If the project already exists, we get its ARN:
except reko_client.exceptions.ResourceInUseException:
# List all the existing project:
print('Project already exists, collecting the ARN.')
reko_project_list = reko_client.describe_projects()
# Loop through all the Rekognition projects:
for project in reko_project_list['ProjectDescriptions']:
# Get the project name (the string after the first delimiter in the ARN)
project_name = project['ProjectArn'].split('/')[1]
# Once we find it, we store the ARN and break out of the loop:
if (project_name == PROJECT_NAME):
project_arn = project['ProjectArn']
break
print(project_arn)
We need to tell Amazon Rekognition where to find the training data and testing data, and where to output its results:
TrainingData = {
'Assets': [{
'GroundTruthManifest': {
'S3Object': {
'Bucket': BUCKET_NAME,
'Name': f'{PREFIX_NAME}/manifests/train.manifest'
}
}
}]
}
TestingData = {
'AutoCreate': True
}
OutputConfig = {
'S3Bucket': BUCKET_NAME,
'S3KeyPrefix': f'{PREFIX_NAME}/output'
}
Now we can create a project version. Creating a project version builds and trains a model within this Amazon Rekognition project for the data previously configured. Project creation can fail if Amazon Rekognition can’t access the bucket you selected. Make sure the right bucket policy is applied to your bucket (check the notebooks to see the recommended policy).
The following code launches a new model training, and you have to wait approximately 1 hour (less than $1 from a cost perspective) for the model to be trained:
version = 'experiment-1'
VERSION_NAME = f'{PROJECT_NAME}.{version}'
# Let's try to create a new project version in the current project:
try:
project_version_arn = reko_client.create_project_version(
ProjectArn=project_arn, # Project ARN
VersionName=VERSION_NAME, # Name of this version
OutputConfig=OutputConfig, # S3 location for the output artefact
TrainingData=TrainingData, # S3 location of the manifest describing the training data
TestingData=TestingData # S3 location of the manifest describing the validation data
)['ProjectVersionArn']
# If a project version with this name already exists, we get its ARN:
except reko_client.exceptions.ResourceInUseException:
# List all the project versions (=models) for this project:
print('Project version already exists, collecting the ARN:', end=' ')
reko_project_versions_list = reko_client.describe_project_versions(ProjectArn=project_arn)
# Loops through them:
for project_version in reko_project_versions_list['ProjectVersionDescriptions']:
# Get the project version name (the string after the third delimiter in the ARN)
project_version_name = project_version['ProjectVersionArn'].split('/')[3]
# Once we find it, we store the ARN and break out of the loop:
if (project_version_name == VERSION_NAME):
project_version_arn = project_version['ProjectVersionArn']
break
print(project_version_arn)
status = reko_client.describe_project_versions(
ProjectArn=project_arn,
VersionNames=[project_version_arn.split('/')[3]]
)['ProjectVersionDescriptions'][0]['Status']
Deploying and evaluating the model
First, we deploy our model by using the ARN collected earlier (see the following code). This deploys an endpoint that costs you around $4 per hour. Don’t forget to decommission it when you’re done.
# Start the model
print('Starting model: ' + model_arn)
response = reko_client.start_project_version(ProjectVersionArn=model_arn, MinInferenceUnits=min_inference_units)
# Wait for the model to be in the running state:
project_version_running_waiter = client.get_waiter('project_version_running')
project_version_running_waiter.wait(ProjectArn=project_arn, VersionNames=[version_name])
# Get the running status
describe_response=client.describe_project_versions(ProjectArn=project_arn, VersionNames=[version_name])
for model in describe_response['ProjectVersionDescriptions']:
print("Status: " + model['Status'])
print("Message: " + model['StatusMessage'])
When the model is running, you can start querying it for predictions. The notebook contains the function get_results()
, which queries a given model with a list of pictures sitting in a given path. This takes a few minutes to run all the test samples and costs less than $1 (for approximately 3,000 test samples). See the following code:
predictions_ok = rt.get_results(project_version_arn, BUCKET, s3_path=f'{BUCKET}/{PREFIX}/test/normal', label='normal', verbose=True)
predictions_ko = rt.get_results(project_version_arn, BUCKET, s3_path=f'{BUCKET}/{PREFIX}/test/abnormal', label='abnormal', verbose=True)
def get_results(project_version_arn, bucket, s3_path, label=None, verbose=True):
"""
Sends a list of pictures located in an S3 path to
the endpoint to get the associated predictions.
"""
fs = s3fs.S3FileSystem()
data = {}
predictions = pd.DataFrame(columns=['image', 'normal', 'abnormal'])
for file in fs.ls(path=s3_path, detail=True, refresh=True):
if file['Size'] > 0:
image = '/'.join(file['Key'].split('/')[1:])
if verbose == True:
print('.', end='')
labels = show_custom_labels(project_version_arn, bucket, image, 0.0)
for L in labels:
data[L['Name']] = L['Confidence']
predictions = predictions.append(pd.Series({
'image': file['Key'].split('/')[-1],
'abnormal': data['abnormal'],
'normal': data['normal'],
'ground truth': label
}), ignore_index=True)
return predictions
def show_custom_labels(model, bucket, image, min_confidence):
# Call DetectCustomLabels from the Rekognition API: this will give us the list
# of labels detected for this picture and their associated confidence level:
reko_client = boto3.client('rekognition')
try:
response = reko_client.detect_custom_labels(
Image={'S3Object': {'Bucket': bucket, 'Name': image}},
MinConfidence=min_confidence,
ProjectVersionArn=model
)
except Exception as e:
print(f'Exception encountered when processing {image}')
print(e)
# Returns the list of custom labels for the image passed as an argument:
return response['CustomLabels']
Let’s plot the confusion matrix associated to this test set (see the following diagram).
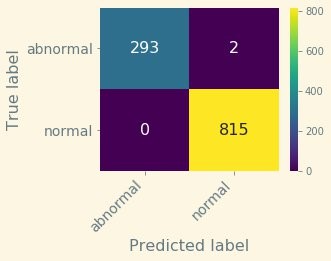
The metrics associated to this matrix are as follows:
- Precision – 100.0%
- Recall – 99.8%
- Accuracy – 99.8%
- F1 score – 99.9%
Without much effort (and no ML knowledge!), we can get impressive results. With such a low false negative rate and without any false positives, we can leverage such a model in even the most challenging industrial context.
Cleaning up
We need to stop the running model to avoid incurring costs while the endpoint is live:
response = reko_client.stop_project_version(ProjectVersionArn=model_arn)
Results comparison
Let’s display the results side by side. The following matrix shows the unsupervised custom TensorFlow model, and an F1 score of 92.1%.
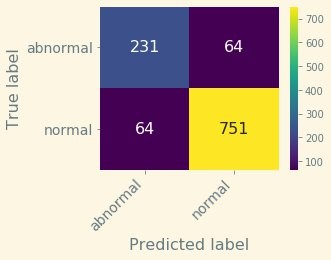
The data preparation effort includes the following:
- No need to collect abnormal signals; only the normal signal is used to build the training dataset
- Generating spectrograms
- Building sequences of sound frames
- Building training and testing datasets
- Uploading datasets to the S3 bucket
The modeling and improvement effort include the following:
- Designing the autoencoder architecture
- Writing a custom training script
- Writing the distribution code (to ensure scalability)
- Performing hyperparameter tuning
The following matrix shows the supervised custom Amazon Rekognition Custom Labels model, and an F1 score of 99.9%.
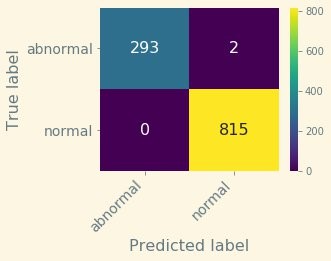
The data preparation effort includes the following:
- Collecting a balanced dataset with enough abnormal signals
- Generating spectrograms
- Making the train and test split
- Uploading spectrograms to the respective S3 training and testing buckets
The modeling and improvement effort include the following:
Determining which approach to use
Which approach should you use? You might use both! As expected, using a supervised approach yields better results. However, the unsupervised approach is perfect to start curating your collected data to easily identify abnormal situations. When you have enough abnormal signals to build a more balanced dataset, you can switch to the supervised approach. Your overall process looks something like the following:
- Start collecting the sound data.
- When you have enough data, train an unsupervised model and use the results to start issuing warnings to a pilot team, who annotates (confirms) abnormal conditions and sets them aside.
- When you have enough data characterizing abnormal conditions, train a supervised model.
- Deploy the supervised approach to a larger scale (especially if you can tune it to limit the undesired false negative to a minimum number).
- Continue collecting sound signals for normal and abnormal conditions, and monitor potential drift between the recent data and the one used for training. Optionally, you can also further detail the anomalies to detect different types of abnormal conditions.
Conclusion
A major challenge factory managers have in order to take advantage of the most recent progress in AI and ML is the amount of customization needed. Training anomaly detection models that can be adapted to many different industrial machineries in order to reduce the maintenance effort, reduce rework or waste, increase product quality, or improve overall equipment efficiency (OEE) or product lines is a massive amount of work.
SageMaker and Amazon Applied AI services such as Amazon Rekognition Custom Labels enables manufacturers to build AI models without having access to a versatile team of data scientists sitting next to each production line. These services allow you to focus on collecting good quality data to augment your factory and provide machine operators, process engineers, and lean manufacturing practioners with high quality insights.
Building upon this solution, you could record 10 seconds sound snippets of your machines and send them to the cloud every 5 minutes, for instance. After you train a model, you can use its predictions to feed custom notifications that you can send back to the supervision screens sitting in the factory.
Can you apply the same process to actual time series as captured by machine sensors? In these cases, spectrograms might not be the best visual representation for these. What about multivariate time series? How can we generalize this approach? Stay tuned for future posts and samples on this impactful topic!
If you’re an ML practitioner passionate about industrial use cases, head over to the Performing anomaly detection on industrial equipment using audio signals GitHub repo for more examples. The solution in this post features an industrial use case, but you can use sound classification ML models in a variety of other settings, for example to analyze animal behavior in agriculture, or to detect anomalous urban sounds such as gunshots, accidents, or dangerous driving. Don’t hesitate to test these services, and let us know what you built!
About the Author
Michaël Hoarau is an AI/ML specialist solution architect at AWS who alternates between a data scientist and machine learning architect, depending on the moment. He is passionate about bringing the power of AI/ML to the shop floors of his industrial customers and has worked on a wide range of ML use cases, ranging from anomaly detection to predictive product quality or manufacturing optimization. When not helping customers develop the next best machine learning experiences, he enjoys observing the stars, traveling, or playing the piano.
Read More