Hear Breen discuss his work leading research teams in speech synthesis and text-to-speech technologies, the science behind Alexa’s enhanced voice styles, and more.Read More
Optimizing applications with EagleDream in Amazon CodeGuru Profiler
This is a guest post by Dustin Potter at EagleDream Technologies. In their own words, “EagleDream Technologies educates, enables, and empowers the world’s greatest companies to use cloud-native technology to transform their business. With extensive experience architecting workloads on the cloud, as well as a full suite of skills in application modernization, data engineering, data lake design, and analytics, EagleDream has built a growing practice in helping businesses redefine what’s possible with technology.”
EagleDream Technologies is a trusted cloud-native transformation company and APN Premier Consulting Partner for businesses using AWS. EagleDream is unique in using its cloud-native software engineering and application modernization expertise to guide you through your journey to the cloud, optimize your operations, and transform how you do business using AWS. Our team of highly trained professionals helps accelerate projects at every stage of the cloud journey. This post shares our experience using Amazon CodeGuru Profiler to help one of our customers optimize their application under tight deadlines.
Project overview
Our team received a unique opportunity to work with one of the industry’s most disruptive airline technology leaders, who uses their expertise to build custom integrated airline booking, loyalty management, and ecommerce platforms. This customer reached out to our team to help optimize their new application. They already had a few clients using the system, but they recently signed a deal with a major airline that would represent a load increase to their platform five times in size. It was critical that they prepare for this significant increase in activity. The customer was running a traditional three-tier application written in Java that used Amazon Aurora for the data layer. They had already implemented autoscaling for the web servers and database but realized something was wrong when they started running load tests. During the first load test, the web tier expanded to over 80 servers and Aurora reached the max number of read replicas.
Our team knew we had to dive deep and investigate the application code. We had previously used other application profiling tools and realized how invaluable they can be when diagnosing these types of issues. Also, AWS recently announced Amazon CodeGuru and we were eager to try it out. On top of that, the price and ease of setup was a driving factor for us. We had looked at an existing commercial application performance monitoring tool, but it required more invasive changes to utilize. To automate the install of these tools, we would have needed to make changes to the customer’s deployment and infrastructure setup. We had to move quickly with as little disruption to their ongoing feature development as possible, which contributed to our final decision to use CodeGuru.
CodeGuru workflow
After we decided on CodeGuru, it was easy to get CodeGuru Profiler installed and start capturing metrics. There are two ways to profile an application. The first is to reference the profiler agent during the start of the application by using the standard -javaagent
parameter. This is useful if the group performing the profiling isn’t the development team, for example in an organization with more traditional development and operation silos. This is easy to set up because all that’s needed is to download the .jar published in the documentation and alter any startup scripts to include the agent and the name of the profiling group to use.
The second way to profile the application is to include the profiler code via a dependency in your build system and instantiate a profiling thread somewhere at the entry point of the program. This option is great if the development team is handling the profiling. For this particular use case, we fell into the second group, so including it in the code was the quickest and easiest approach. We added the library as a Maven dependency and added a single line of application code. After the code was committed, we used the customer’s existing Jenkins setup to deploy the latest build to an integration environment. The final step of the pipeline was to run load tests against the new build. After the tests completed, we had a flame graph that we used to start identifying any issues.
The workflow includes the following steps:
- Developers check in code.
- The check-in triggers a Jenkins job.
- Maven compiles the code.
- Jenkins deploys the artifact to the development environment.
- Load tests run against the newly deployed code.
- CodeGuru Profiler monitors the environment and generates a flame graph and a recommendation report.
The following diagram illustrates the workflow.
Flame graphs group together stack traces and highlight which part of the code consumes the most resources. The following screenshot is a sample flame graph from an AWS demo application for reference.
After CodeGuru generated the flame graphs and recommendations report, we took an iterative approach and tackled the biggest offenders first. The flame graphs provided perceptive guidance for actionable recommendations that it discovers and made it easy to identify which execution paths were taking the longest to complete. By looking at the longest frames first, we identified that the customer faced challenges around thread safety, which was leading to locking issues. To resolve issues collaboratively with the client, we created a Slack channel to review the latest graphs and provide recommendations directly to the developers. After the developers implemented the suggested changes, we deployed a new build and had a corresponding graph in a few minutes.
Results
After just one week, our team successfully alleviated their scaling challenges at the web service layer. When we ran the load tests, we saw expected results of a few servers instead of the more than 80 servers previously. Additionally, because we optimized the code, we reduced the existing application footprint, which saved our customer 30% of compute load.
Cost savings aside, one of the most notable benefits of this project was developer education. With CodeGuru Profiler pinpointing where the bottlenecks were, the developers could recognize inefficient patterns in the code that might lead to severe performance hits down the road. This helped them better understand the features of the language they’re using and armed them with increased efficiency in future development and debugging.
Conclusion
With the web service layer better optimized, our next step is to use CodeGuru and other AWS tools like Performance Insights to tackle the database layer. Even if you aren’t experiencing extreme performance challenges, CodeGuru Profiler can provide valuable insights to the health of your application in any environment, from development all the way to production, with minimal CPU utilization. Integrating these results as part of the SDLC or DevOps process leads to better efficiency and gives you and your developers the tools you need to be successful. To learn more about how to get started with CodeGuru Profiler and CodeGuru Reviewer, check the documentation found here.
About the Author
Dustin Potter is a Principal Cloud Solutions Architect at EagleDream Technologies.
Amazon Translate ranked as #1 machine translation provider by Intento
Customer obsession, one of the key Amazon Leadership principles that guides everything we do at Amazon, has helped Amazon Translate be recognized as an industry leading neural machine translation provider. This year, Intento ranked Amazon Translate #1 on the list of top-performing machine translation providers in its The State of Machine Translation 2020 report. We are excited to be recognized for pursuing our passion—designing the best customer experience in machine translation.
Amazon Translate is a neural machine translation service that delivers fast, high-quality, and affordable language translation. Neural machine translation is a form of machine translation that uses deep learning models to deliver more accurate and more natural sounding translation than traditional statistical and rule-based translation algorithms. Amazon Translate’s development has been fueled by customer feedback, leading to a steady stream of rich features that help you reach more people in more places—without breaking your translation services budget.
Intento is one of the leading organizations helping global companies procure and utilize the best-fit cognitive AI services. In this independent study, Intento evaluated 15 of the most prominent MT providers used by language service providers and localization services. The data used included examples from across 16 industry sectors, with 8 content types, including topics such as financial documentation, patents, sales and marketing material. These inputs were translated between 14 common language pairs to determine the best engine for a given translation scenario. It ranked the results of each MT engine based on how they compared to a reference human translation.
The results showed that no MT service is best in all language pairs across all industry sectors and content types. However, Amazon Translate had the highest number of instances in which it was rated “best.”
At Amazon, we strive to bring the most value to our customers and deliver the world’s best machine translation service! If your company is looking for machine translation, please contact us. We’d love to show you what Amazon Translate can do.
More information on the features and capabilities that Intento considered in its analysis of the top MT providers is available in the full report (registration is required).
About the Author
Greg Rushing is a US Air Force Fellow in Amazon’s BRIDGE program. He is currently working with the Amazon Translate Product Management team, where he focuses on coordinating the activities required to bring Amazon Translate features to market. Outside of work, you can find him spending time exploring the outdoors with his family, doing auto repair, or woodworking.
Building a medical image search platform on AWS
Improving radiologist efficiency and preventing burnout is a primary goal for healthcare providers. A nationwide study published in Mayo Clinic Proceedings in 2015 showed radiologist burnout percentage at a concerning 61% [1]. In additon, the report concludes that “burnout and satisfaction with work-life balance in US physicians worsened from 2011 to 2014. More than half of US physicians are now experiencing professional burnout.”[2] As technologists, we’re looking for ways to put new and innovative solutions in the hands of physicians to make them more efficient, reduce burnout, and improve care quality.
To reduce burnout and improve value-based care through data-driven decision-making, Artificial Intelligence (AI) can be used to unlock the information trapped in the vast amount of unstructured data (e.g. images, texts, and voice) and create clinically actionable knowledge base. AWS AI services can derive insights and relationships from free-form medical reports, automate the knowledge sharing process, and eventually improve personalized care experience.
In this post, we use Convolutional Neural Networks (CNN) as a feature extractor to convert medical images into a one-dimensional feature vector with a size of 1024. We call this process medical image embedding. Then we index the image feature vector using the K-nearest neighbors (KNN) algorithm in Amazon Elasticsearch Service (Amazon ES) to build a similarity-based image retrieval system. Additionally, we use the AWS managed natural language processing (NLP) service Amazon Comprehend Medical to perform named entity recognition (NER) against free text clinical reports. The detected named entities are also linked to medical ontology, ICD-10-CM, to enable simple aggregation and distribution analysis. The presented solution also includes a front-end React web application and backend GraphQL API managed by AWS Amplify and AWS AppSync, and authentication is handled by Amazon Cognito.
After deploying this working solution, the end-users (healthcare providers) can search through a repository of unstructured free text and medical images, conduct analytical operations, and use it in medical training and clinical decision support. This eliminates the need to manually analyze all the images and reports and get to the most relevant ones. Using a system like this improves the provider’s efficiency. The following graphic shows an example end result of the deployed application.
Dataset and architecture
We use the MIMIC CXR dataset to demonstrate how this working solution can benefit healthcare providers, in particular, radiologists. MIMIC CXR is a publicly available database of chest X-ray images in DICOM format and the associated radiology reports as free text files[3]. The methods for data collection and the data structures in this dataset have been well documented and are very detailed [3]. Also, this is a restricted-access resource. To access the files, you must be a registered user and sign the data use agreement. The following sections provide more details on the components of the architecture.
The following diagram illustrates the solution architecture.
The architecture is comprised of the offline data transformation and online query components. The offline data transformation step, the unstructured data, including free texts and image files, is converted into structured data.
Electronic Heath Record (EHR) radiology reports as free text are processed using Amazon Comprehend Medical, an NLP service that uses machine learning to extract relevant medical information from unstructured text, such as medical conditions including clinical signs, diagnosis, and symptoms. The named entities are identified and mapped to structured vocabularies, such as ICD-10 Clinical Modifications (CMs) ontology. The unstructured text plus structured named entities are stored in Amazon ES to enable free text search and term aggregations.
The medical images from Picture Archiving and Communication System (PACS) are converted into vector representations using a pretrained deep learning model deployed in an Amazon Elastic Container Service (Amazon ECS) AWS Fargate cluster. Similar visual search on AWS has been published previously for online retail product image search. It used an Amazon SageMaker built-in KNN algorithm for similarity search, which supports different index types and distance metrics.
We took advantage of the KNN for Amazon ES to find the k closest images from a feature space as demonstrated on the GitHub repo. KNN search is supported in Amazon ES version 7.4+. The container running on the ECS Fargate cluster reads medical images in DICOM format, carries out image embedding using a pretrained model, and saves a PNG thumbnail in an Amazon Simple Storage Service (Amazon S3) bucket, which serves as the storage for AWS Amplify React web application. It also parses out the DICOM image metadata and saves them in Amazon DynamoDB. The image vectors are saved in an Elasticsearch cluster and are used for the KNN visual search, which is implemented in an AWS Lambda function.
The unstructured data from EHR and PACS needs to be transferred to Amazon S3 to trigger the serverless data processing pipeline through the Lambda functions. You can achieve this data transfer by using AWS Storage Gateway or AWS DataSync, which is out of the scope of this post. The online query API, including the GraphQL schemas and resolvers, was developed in AWS AppSync. The front-end web application was developed using the Amplify React framework, which can be deployed using the Amplify CLI. The detailed AWS CloudFormation templates and sample code are available in the Github repo.
Solution overview
To deploy the solution, you complete the following steps:
- Deploy the Amplify React web application for online search.
- Deploy the image-embedding container to AWS Fargate.
- Deploy the data-processing pipeline and AWS AppSync API.
Deploying the Amplify React web application
The first step creates the Amplify React web application, as shown in the following diagram.
- Install and configure the AWS Command Line Interface (AWS CLI).
- Install the AWS Amplify CLI.
- Clone the code base with stepwise instructions.
- Go to your code base folder and initialize the Amplify app using the command
amplify init
. You must answer a series of questions, like the name of the Amplify app.
After this step, you have the following changes in your local and cloud environments:
- A new folder named
amplify
is created in your local environment - A file named
aws-exports.js
is created in local thesrc
folder - A new Amplify app is created on the AWS Cloud with the name provided during deployment (for example,
medical-image-search
) - A CloudFormation stack is created on the AWS Cloud with the prefix
amplify-<AppName>
You create authentication and storage services for your Amplify app afterwards using the following commands:
amplify add auth
amplify add storage
amplify push
When the CloudFormation nested stacks for authentication and storage are successfully deployed, you can see the new Amazon Cognito user pool as the authentication backend and S3 bucket as the storage backend are created. Save the Amazon Cognito user pool ID and S3 bucket name from the Outputs tab of the corresponding CloudFormation nested stack (you use these later).
The following screenshot shows the location of the user pool ID on the Outputs tab.
The following screenshot shows the location of the bucket name on the Outputs tab.
Deploying the image-embedding container to AWS Fargate
We use the Amazon SageMaker Inference Toolkit to serve the PyTorch inference model, which converts a medical image in DICOM format into a feature vector with the size of 1024. To create a container with all the dependencies, you can either use pre-built deep learning container images or derive a Dockerfile from the Amazon Sagemaker Pytorch inference CPU container, like the one from the GitHub repo, in the container folder. You can build the Docker container and push it to Amazon ECR manually or by running the shell script build_and_push.sh. You use the repository image URI for the Docker container later to deploy the AWS Fargate cluster.
The following screenshot shows the sagemaker-pytorch-inference
repository on the Amazon ECR console.
We use Multi Model Server (MMS) to serve the inference endpoint. You need to install MMS with pip locally, use the Model archiver CLI to package model artifacts into a single model archive .mar
file, and upload it to an S3 bucket to be served by a containerized inference endpoint. The model inference handler is defined in dicom_featurization_service.py in the MMS
folder. If you have a domain-specific pretrained Pytorch model, place the model.pth
file in the MMS
folder; otherwise, the handler uses a pretrained DenseNET121[4] for image processing. See the following code:
model_file_path = os.path.join(model_dir, "model.pth")
if os.path.isfile(model_file_path):
model = torch.load(model_file_path)
else:
model = models.densenet121(pretrained=True)
model = model._modules.get('features')
model.add_module("end_relu", nn.ReLU())
model.add_module("end_globpool", nn.AdaptiveAvgPool2d((1, 1)))
model.add_module("end_flatten", nn.Flatten())
model = model.to(self.device)
model.eval()
The intermediate results of this CNN-based model is to represent images as feature vectors. In other words, the convolutional layers before the final classification layer is flattened to convert feature layers to a vector representation. Run the following command in the MMS
folder to package up the model archive file:
model-archiver -f --model-name dicom_featurization_service --model-path ./ --handler dicom_featurization_service:handle --export-path ./
The preceding code generates a package file named dicom_featurization_service.mar
. Create a new S3 bucket and upload the package file to that bucket with public read Access Control List (ACL). See the following code:
aws s3 cp ./dicom_featurization_service.mar s3://<S3bucketname>/ --acl public-read --profile <profilename>
You’re now ready to deploy the image-embedding inference model to the AWS Fargate cluster using the CloudFormation template ecsfargate.yaml in the CloudFormationTemplates
folder. You can deploy using the AWS CLI: go to the CloudFormationTemplates
folder and copy the following command:
aws cloudformation deploy --capabilities CAPABILITY_IAM --template-file ./ecsfargate.yaml --stack-name <stackname> --parameter-overrides ImageUrl=<imageURI> InferenceModelS3Location=https://<S3bucketname>.s3.amazonaws.com/dicom_featurization_service.mar --profile <profilename>
You need to replace the following placeholders:
- stackname – A unique name to refer to this CloudFormation stack
- imageURI – The image URI for the MMS Docker container uploaded in Amazon ECR
- S3bucketname – The MMS package in the S3 bucket, such as
https://
<S3bucketname>.s3.amazonaws.com/dicom_featurization_service.mar
- profilename – Your AWS CLI profile name (default if not named)
Alternatively, you can choose Launch stack for the following Regions:
- us-east-1 –
- us-west-2 –
After the CloudFormation stack creation is complete, go to the stack Outputs tab on the AWS CloudFormation console and copy the InferenceAPIUrl
for later deployment. See the following screenshot.
You can delete this stack after the offline image embedding jobs are finished to save costs, because it’s not used for online queries.
Deploying the data-processing pipeline and AWS AppSync API
You deploy the image and free text data-processing pipeline and AWS AppSync API backend through another CloudFormation template named AppSyncBackend.yaml in the CloudFormationTemplates
folder, which creates the AWS resources for this solution. See the following solution architecture.
To deploy this stack using the AWS CLI, go to the CloudFormationTemplates
folder and copy the following command:
aws cloudformation deploy --capabilities CAPABILITY_NAMED_IAM --template-file ./AppSyncBackend.yaml --stack-name <stackname> --parameter-overrides AuthorizationUserPool=<CFN_output_auth> PNGBucketName=<CFN_output_storage> InferenceEndpointURL=<inferenceAPIUrl> --profile <profilename>
Replace the following placeholders:
- stackname – A unique name to refer to this CloudFormation stack
- AuthorizationUserPool – Amazon Cognito user pool
- PNGBucketName – Amazon S3 bucket name
- InferenceEndpointURL – The inference API endpoint
- Profilename – The AWS CLI profile name (use default if not named)
Alternatively, you can choose Launch stack for the following Regions:
- us-east-1 –
- us-west-2 –
You can download the Lambda function for medical image processing, CMprocessLambdaFunction.py, and its dependency layer separately if you deploy this stack in AWS Regions other than us-east-1
and us-west-2
. Because their file size exceeds the CloudFormation template limit, you need to upload them to your own S3 bucket (either create a new S3 bucket or use the existing one, like the aforementioned S3 bucket for hosting the MMS model package file) and override the LambdaBucket mapping parameter using your own bucket name.
Save the AWS AppySync API URL and AWS Region from the settings on the AWS AppSync console.
Edit the src/aws-exports.js
file in your local environment and replace the placeholders with those values:
const awsmobile = {
"aws_appsync_graphqlEndpoint": "<AppSync API URL>",
"aws_appsync_region": "<AWS AppSync Region>",
"aws_appsync_authenticationType": "AMAZON_COGNITO_USER_POOLS"
};
After this stack is successfully deployed, you’re ready to use this solution. If you have in-house EHR and PACS databases, you can set up the AWS Storage Gateway to transfer data to the S3 bucket to trigger the transformation jobs.
Alternatively, you can use the public dataset MIMIC CXR: download the MIMIC CXR dataset from PhysioNet (to access the files, you must be a credentialed user and sign the data use agreement for the project) and upload the DICOM files to the S3 bucket mimic-cxr-dicom-
and the free text radiology report to the S3 bucket mimic-cxr-report-
. If everything works as expected, you should see the new records created in the DynamoDB table medical-image-metadata
and the Amazon ES domain medical-image-search
.
You can test the Amplify React web application locally by running the following command:
npm install && npm start
Or you can publish the React web app by deploying it in Amazon S3 with AWS CloudFront distribution, by first entering the following code:
amplify hosting add
Then, enter the following code:
amplify publish
You can see the hosting endpoint for the Amplify React web application after deployment.
Conclusion
We have demonstrated how to deploy, index and search medical images on AWS, which segregates the offline data ingestion and online search query functions. You can use AWS AI services to transform unstructured data, for example the medical images and radiology reports, into structured ones.
By default, the solution uses a general-purpose model trained on ImageNET to extract features from images. However, this default model may not be accurate enough to extract medical image features because there are fundamental differences in appearance, size, and features between medical images in its raw form. Such differences make it hard to train commonly adopted triplet-based learning networks [5], where semantically relevant images or objects can be easily defined or ranked.
To improve search relevancy, we performed an experiment by using the same MIMIC CXR dataset and the derived diagnosis labels to train a weakly supervised disease classification network similar to Wang et. Al [6]. We found this domain-specific pretrained model yielded qualitatively better visual search results. So it’s recommended to bring your own model (BYOM) to this search platform for real-world implementation.
The methods presented here enable you to perform indexing, searching and aggregation against unstructured images in addition to free text. It sets the stage for future work that can combine these features for multimodal medical image search engine. Information retrieval from unstructured corpuses of clinical notes and images is a time-consuming and tedious task. Our solution allows radiologists to become more efficient and help them reduce potential burnout.
To find the latest development to this solution, check out medical image search on GitHub.
Reference:
- https://www.radiologybusiness.com/topics/leadership/radiologist-burnout-are-we-done-yet
- https://www.mayoclinicproceedings.org/article/S0025-6196(15)00716-8/abstract#secsectitle0010
- Johnson, Alistair EW, et al. “MIMIC-CXR, a de-identified publicly available database of chest radiographs with free-text reports.” Scientific Data 6, 2019.
- Huang, Gao, et al. “Densely connected convolutional networks.” Proceedings of the IEEE Conference on Computer Vision and Pattern Recognition, 2017.
- Wang, Jiang, et al. “Learning fine-grained image similarity with deep ranking.” Proceedings of the IEEE Conference on Computer Vision and Pattern Recognition, 2014.
- Wang, Xiaosong, et al. “Chestx-ray8: Hospital-scale chest x-ray database and benchmarks on weakly-supervised classification and localization of common thorax diseases.” Proceedings of the IEEE Conference on Computer Vision and Pattern Recognition, 2017.
About the Authors
Gang Fu is a Healthcare Solution Architect at AWS. He holds a PhD in Pharmaceutical Science from the University of Mississippi and has over ten years of technology and biomedical research experience. He is passionate about technology and the impact it can make on healthcare.
Ujjwal Ratan is a Principal Machine Learning Specialist Solution Architect in the Global Healthcare and Lifesciences team at Amazon Web Services. He works on the application of machine learning and deep learning to real world industry problems like medical imaging, unstructured clinical text, genomics, precision medicine, clinical trials and quality of care improvement. He has expertise in scaling machine learning/deep learning algorithms on the AWS cloud for accelerated training and inference. In his free time, he enjoys listening to (and playing) music and taking unplanned road trips with his family.
Erhan Bas is a Senior Applied Scientist in the AWS Rekognition team, currently developing deep learning algorithms for computer vision applications. His expertise is in machine learning and large scale image analysis techniques, especially in biomedical, life sciences and industrial inspection technologies. He enjoys playing video games, drinking coffee, and traveling with his family.
Streamlining data labeling for YOLO object detection in Amazon SageMaker Ground Truth
Object detection is a common task in computer vision (CV), and the YOLOv3 model is state-of-the-art in terms of accuracy and speed. In transfer learning, you obtain a model trained on a large but generic dataset and retrain the model on your custom dataset. One of the most time-consuming parts in transfer learning is collecting and labeling image data to generate a custom training dataset. This post explores how to do this in Amazon SageMaker Ground Truth.
Ground Truth offers a comprehensive platform for annotating the most common data labeling jobs in CV: image classification, object detection, semantic segmentation, and instance segmentation. You can perform labeling using Amazon Mechanical Turk or create your own private team to label collaboratively. You can also use one of the third-party data labeling service providers listed on the AWS Marketplace. Ground Truth offers an intuitive interface that is easy to work with. You can communicate with labelers about specific needs for your particular task using examples and notes through the interface.
Labeling data is already hard work. Creating training data for a CV modeling task requires data collection and storage, setting up labeling jobs, and post-processing the labeled data. Moreover, not all object detection models expect the data in the same format. For example, the Faster RCNN model expects the data in the popular Pascal VOC format, which the YOLO models can’t work with. These associated steps are part of any machine learning pipeline for CV. You sometimes need to run the pipeline multiple times to improve the model incrementally. This post shows how to perform these steps efficiently by using Python scripts and get to model training as quickly as possible. This post uses the YOLO format for its use case, but the steps are mostly independent of the data format.
The image labeling step of a training data generation task is inherently manual. This post shows how to create a reusable framework to create training data for model building efficiently. Specifically, you can do the following:
- Create the required directory structure in Amazon S3 before starting a Ground Truth job
- Create a private team of annotators and start a Ground Truth job
- Collect the annotations when labeling is complete and save it in a pandas dataframe
- Post-process the dataset for model training
You can download the code presented in this post from this GitHub repo. This post demonstrates how to run the code from the AWS CLI on a local machine that can access an AWS account. For more information about setting up AWS CLI, see What Is the AWS Command Line Interface? Make sure that you configure it to access the S3 buckets in this post. Alternatively, you can run it in AWS Cloud9 or by spinning up an Amazon EC2 instance. You can also run the code blocks in an Amazon SageMaker notebook.
If you’re using an Amazon SageMaker notebook, you can still access the Linux shell of the underlying EC2 instance and follow along by opening a new terminal from the Jupyter main page and running the scripts from the /home/ec2-user/SageMaker
folder.
Setting up your S3 bucket
The first thing you need to do is to upload the training images to an S3 bucket. Name the bucket ground-truth-data-labeling
. You want each labeling task to have its own self-contained folder under this bucket. If you start labeling a small set of images that you keep in the first folder, but find that the model performed poorly after the first round because the data was insufficient, you can upload more images to a different folder under the same bucket and start another labeling task.
For the first labeling task, create the folder bounding_box
and the following three subfolders under it:
- images – You upload all the images in the Ground Truth labeling job to this subfolder.
- ground_truth_annots – This subfolder starts empty; the Ground Truth job populates it automatically, and you retrieve the final annotations from here.
- yolo_annot_files – This subfolder also starts empty, but eventually holds the annotation files ready for model training. The script populates it automatically.
If your images are in .jpeg format and available in the current working directory, you can upload the images with the following code:
aws s3 sync . s3://ground-truth-data-labeling/bounding_box/images/ --exclude "*" --include "*.jpg"
For this use case, you use five images. There are two types of objects in the images—pencil and pen. You need to draw bounding boxes around each object in the images. The following images are examples of what you need to label. All images are available in the GitHub repo.
Creating the manifest file
A Ground Truth job requires a manifest file in JSON format that contains the Amazon S3 paths of all the images to label. You need to create this file before you can start the first Ground Truth job. The format of this file is simple:
{"source-ref": < S3 path to image1 >}
{"source-ref": < S3 path to image2 >}
...
However, creating the manifest file by hand would be tedious for a large number of images. Therefore, you can automate the process by running a script. You first need to create a file holding the parameters required for the scripts. Create a file input.json
in your local file system with the following content:
{
"s3_bucket":"ground-truth-data-labeling",
"job_id":"bounding_box",
"ground_truth_job_name":"yolo-bbox",
"yolo_output_dir":"yolo_annot_files"
}
Save the following code block in a file called prep_gt_job.py
:
import boto3
import json
def create_manifest(job_path):
"""
Creates the manifest file for the Ground Truth job
Input:
job_path: Full path of the folder in S3 for GT job
Returns:
manifest_file: The manifest file required for GT job
"""
s3_rec = boto3.resource("s3")
s3_bucket = job_path.split("/")[0]
prefix = job_path.replace(s3_bucket, "")[1:]
image_folder = f"{prefix}/images"
print(f"using images from ... {image_folder} n")
bucket = s3_rec.Bucket(s3_bucket)
objs = list(bucket.objects.filter(Prefix=image_folder))
img_files = objs[1:] # first item is the folder name
n_imgs = len(img_files)
print(f"there are {n_imgs} images n")
TOKEN = "source-ref"
manifest_file = "/tmp/manifest.json"
with open(manifest_file, "w") as fout:
for img_file in img_files:
fname = f"s3://{s3_bucket}/{img_file.key}"
fout.write(f'{{"{TOKEN}": "{fname}"}}n')
return manifest_file
def upload_manifest(job_path, manifest_file):
"""
Uploads the manifest file into S3
Input:
job_path: Full path of the folder in S3 for GT job
manifest_file: Path to the local copy of the manifest file
"""
s3_rec = boto3.resource("s3")
s3_bucket = job_path.split("/")[0]
source = manifest_file.split("/")[-1]
prefix = job_path.replace(s3_bucket, "")[1:]
destination = f"{prefix}/{source}"
print(f"uploading manifest file to {destination} n")
s3_rec.meta.client.upload_file(manifest_file, s3_bucket, destination)
def main():
"""
Performs the following tasks:
1. Reads input from 'input.json'
2. Collects image names from S3 and creates the manifest file for GT
3. Uploads the manifest file to S3
"""
with open("input.json") as fjson:
input_dict = json.load(fjson)
s3_bucket = input_dict["s3_bucket"]
job_id = input_dict["job_id"]
gt_job_path = f"{s3_bucket}/{job_id}"
man_file = create_manifest(gt_job_path)
upload_manifest(gt_job_path, man_file)
if __name__ == "__main__":
main()
Run the following script:
python prep_gt_job.py
This script reads the S3 bucket and job names from the input file, creates a list of images available in the images folder, creates the manifest.json
file, and uploads the manifest file to the S3 bucket at s3://ground-truth-data-labeling/bounding_box/
.
This method illustrates a programmatic control of the process, but you can also create the file from the Ground Truth API. For instructions, see Create a Manifest File.
At this point, the folder structure in the S3 bucket should look like the following:
ground-truth-data-labeling
|-- bounding_box
|-- ground_truth_annots
|-- images
|-- yolo_annot_files
|-- manifest.json
Creating the Ground Truth job
You’re now ready to create your Ground Truth job. You need to specify the job details and task type, and create your team of labelers and labeling task details. Then you can sign in to begin the labeling job.
Specifying the job details
To specify the job details, complete the following steps:
- On the Amazon SageMaker console, under Ground Truth, choose Labeling jobs.
- On the Labeling jobs page, choose Create labeling job.
- In the Job overview section, for Job name, enter
yolo-bbox
. It should be the name you defined in theinput.json
file earlier. - Pick Manual Data Setup under Input Data Setup.
- For Input dataset location, enter
s3://ground-truth-data-labeling/bounding_box/manifest.json
. - For Output dataset location, enter
s3://ground-truth-data-labeling/bounding_box/ground_truth_annots
.
- In the Create an IAM role section, first select Create a new role from the drop down menu and then select Specific S3 buckets.
- Enter ground-truth-data-labeling.
- Choose Create.
Specifying the task type
To specify the task type, complete the following steps:
- In the Task selection section, from the Task Category drop-down menu, choose Image.
- Select Bounding box.
- Don’t change Enable enhanced image access, which is selected by default. It enables Cross-Origin Resource Sharing (CORS) that may be required for some workers to complete the annotation task.
- Choose Next.
Creating a team of labelers
To create your team of labelers, complete the following steps:
- In the Workers section, select Private.
- Follow the instructions to create a new team.
Each member of the team receives a notification email titled, “You’re invited to work on a labeling project” that has initial sign-in credentials. For this use case, create a team with just yourself as a member.
Specifying labeling task details
In the Bounding box labeling tool section, you should see the images you uploaded to Amazon S3. You should check that the paths are correct in the previous steps. To specify your task details, complete the following steps:
- In the text box, enter a brief description of the task.
This is critical if the data labeling team has more than one members and you want to make sure everyone follows the same rule when drawing the boxes. Any inconsistency in bounding box creation may end up confusing your object detection model. For example, if you’re labeling beverage cans and want to create a tight bounding box only around the visible logo, instead of the entire can, you should specify that to get consistent labeling from all the workers. For this use case, you can enter Please enter a tight bounding box around the entire object
.
- Optionally, you can upload examples of a good and a bad bounding box.
You can make sure your team is consistent in their labels by providing good and bad examples.
- Under Labels, enter the names of the labels you’re using to identify each bounding box; in this case,
pencil
andpen
.
A color is assigned to each label automatically, which helps to visualize the boxes created for overlapping objects.
- To run a final sanity check, choose Preview.
- Choose Create job.
Job creation can take up to a few minutes. When it’s complete, you should see a job titled yolo-bbox on the Ground Truth Labeling jobs page with In progress as the status.
- To view the job details, select the job.
This is a good time to verify the paths are correct; the scripts don’t run if there’s any inconsistency in names.
For more information about providing labeling instructions, see Create high-quality instructions for Amazon SageMaker Ground Truth labeling jobs.
Sign in and start labeling
After you receive the initial credentials to register as a labeler for this job, follow the link to reset the password and start labeling.
If you need to interrupt your labeling session, you can resume labeling by choosing Labeling workforces under Ground Truth on the SageMaker console.
You can find the link to the labeling portal on the Private tab. The page also lists the teams and individuals involved in this private labeling task.
After you sign in, start labeling by choosing Start working.
Because you only have five images in the dataset to label, you can finish the entire task in a single session. For larger datasets, you can pause the task by choosing Stop working and return to the task later to finish it.
Checking job status
After the labeling is complete, the status of the labeling job changes to Complete and a new JSON file called output.manifest
containing the annotations appears at s3://ground-truth-data-labeling/bounding_box/ground_truth_annots/yolo-bbox/manifests/output /output.manifest
.
Parsing Ground Truth annotations
You can now parse through the annotations and perform the necessary post-processing steps to make it ready for model training. Start by running the following code block:
from io import StringIO
import json
import s3fs
import boto3
import pandas as pd
def parse_gt_output(manifest_path, job_name):
"""
Captures the json Ground Truth bounding box annotations into a pandas dataframe
Input:
manifest_path: S3 path to the annotation file
job_name: name of the Ground Truth job
Returns:
df_bbox: pandas dataframe with bounding box coordinates
for each item in every image
"""
filesys = s3fs.S3FileSystem()
with filesys.open(manifest_path) as fin:
annot_list = []
for line in fin.readlines():
record = json.loads(line)
if job_name in record.keys(): # is it necessary?
image_file_path = record["source-ref"]
image_file_name = image_file_path.split("/")[-1]
class_maps = record[f"{job_name}-metadata"]["class-map"]
imsize_list = record[job_name]["image_size"]
assert len(imsize_list) == 1
image_width = imsize_list[0]["width"]
image_height = imsize_list[0]["height"]
for annot in record[job_name]["annotations"]:
left = annot["left"]
top = annot["top"]
height = annot["height"]
width = annot["width"]
class_name = class_maps[f'{annot["class_id"]}']
annot_list.append(
[
image_file_name,
class_name,
left,
top,
height,
width,
image_width,
image_height,
]
)
df_bbox = pd.DataFrame(
annot_list,
columns=[
"img_file",
"category",
"box_left",
"box_top",
"box_height",
"box_width",
"img_width",
"img_height",
],
)
return df_bbox
def save_df_to_s3(df_local, s3_bucket, destination):
"""
Saves a pandas dataframe to S3
Input:
df_local: Dataframe to save
s3_bucket: Bucket name
destination: Prefix
"""
csv_buffer = StringIO()
s3_resource = boto3.resource("s3")
df_local.to_csv(csv_buffer, index=False)
s3_resource.Object(s3_bucket, destination).put(Body=csv_buffer.getvalue())
def main():
"""
Performs the following tasks:
1. Reads input from 'input.json'
2. Parses the Ground Truth annotations and creates a dataframe
3. Saves the dataframe to S3
"""
with open("input.json") as fjson:
input_dict = json.load(fjson)
s3_bucket = input_dict["s3_bucket"]
job_id = input_dict["job_id"]
gt_job_name = input_dict["ground_truth_job_name"]
mani_path = f"s3://{s3_bucket}/{job_id}/ground_truth_annots/{gt_job_name}/manifests/output/output.manifest"
df_annot = parse_gt_output(mani_path, gt_job_name)
dest = f"{job_id}/ground_truth_annots/{gt_job_name}/annot.csv"
save_df_to_s3(df_annot, s3_bucket, dest)
if __name__ == "__main__":
main()
From the AWS CLI, save the preceding code block in the file parse_annot.py and run:
python parse_annot.py
Ground Truth returns the bounding box information using the following four numbers: x and y coordinates, and its height and width. The procedure parse_gt_output
scans through the output.manifest
file and stores the information for every bounding box for each image in a pandas dataframe. The procedure save_df_to_s3
saves it in a tabular format as annot.csv
to the S3 bucket for further processing.
The creation of the dataframe is useful for a few reasons. JSON files are hard to read and the output.manifest
file contains more information, like label metadata, than you need for the next step. The dataframe contains only the relevant information and you can visualize it easily to make sure everything looks fine.
To grab the annot.csv
file from Amazon S3 and save a local copy, run the following:
aws s3 cp s3://ground-truth-data-labeling/bounding_box/ground_truth_annots/yolo-bbox/annot.csv
You can read it back into a pandas dataframe and inspect the first few lines. See the following code:
import pandas as pd
df_ann = pd.read_csv('annot.csv')
df_ann.head()
The following screenshot shows the results.
You also capture the size of the image through img_width
and img_height
. This is necessary because the object detection models need to know the location of each bounding box within the image. In this case, you can see that images in the dataset were captured with a 4608×3456 pixel resolution.
There are quite a few reasons why it is a good idea to save the annotation information into a dataframe:
- In a subsequent step, you need to rescale the bounding box coordinates into a YOLO-readable format. You can do this operation easily in a dataframe.
- If you decide to capture and label more images in the future to augment the existing dataset, all you need to do is join the newly created dataframe with the existing one. Again, you can perform this easily using a dataframe.
- As of this writing, Ground Truth doesn’t allow through the console more than 30 different categories to label in the same job. If you have more categories in your dataset, you have to label them under multiple Ground Truth jobs and combine them. Ground Truth associates each bounding box to an integer index in the
output.manifest
file. Therefore, the integer labels are different across multiple Ground Truth jobs if you have more than 30 categories. Having the annotations as dataframes makes the task of combining them easier and takes care of the conflict of category names across multiple jobs. In the preceding screenshot, you can see that you used the actual names under the category column instead of the integer index.
Generating YOLO annotations
You’re now ready to reformat the bounding box coordinates Ground Truth provided into a format the YOLO model accepts.
In the YOLO format, each bounding box is described by the center coordinates of the box and its width and height. Each number is scaled by the dimensions of the image; therefore, they all range between 0 and 1. Instead of category names, YOLO models expect the corresponding integer categories.
Therefore, you need to map each name in the category column of the dataframe into a unique integer. Moreover, the official Darknet implementation of YOLOv3 needs to have the name of the image match the annotation text file name. For example, if the image file is pic01.jpg
, the corresponding annotation file should be named pic01.txt
.
The following code block performs all these tasks:
import os
import json
from io import StringIO
import boto3
import s3fs
import pandas as pd
def annot_yolo(annot_file, cats):
"""
Prepares the annotation in YOLO format
Input:
annot_file: csv file containing Ground Truth annotations
ordered_cats: List of object categories in proper order for model training
Returns:
df_ann: pandas dataframe with the following columns
img_file int_category box_center_w box_center_h box_width box_height
Note:
YOLO data format: <object-class> <x_center> <y_center> <width> <height>
"""
df_ann = pd.read_csv(annot_file)
df_ann["int_category"] = df_ann["category"].apply(lambda x: cats.index(x))
df_ann["box_center_w"] = df_ann["box_left"] + df_ann["box_width"] / 2
df_ann["box_center_h"] = df_ann["box_top"] + df_ann["box_height"] / 2
# scale box dimensions by image dimensions
df_ann["box_center_w"] = df_ann["box_center_w"] / df_ann["img_width"]
df_ann["box_center_h"] = df_ann["box_center_h"] / df_ann["img_height"]
df_ann["box_width"] = df_ann["box_width"] / df_ann["img_width"]
df_ann["box_height"] = df_ann["box_height"] / df_ann["img_height"]
return df_ann
def save_annots_to_s3(s3_bucket, prefix, df_local):
"""
For every image in the dataset, save a text file with annotation in YOLO format
Input:
s3_bucket: S3 bucket name
prefix: Folder name under s3_bucket where files will be written
df_local: pandas dataframe with the following columns
img_file int_category box_center_w box_center_h box_width box_height
"""
unique_images = df_local["img_file"].unique()
s3_resource = boto3.resource("s3")
for image_file in unique_images:
df_single_img_annots = df_local.loc[df_local.img_file == image_file]
annot_txt_file = image_file.split(".")[0] + ".txt"
destination = f"{prefix}/{annot_txt_file}"
csv_buffer = StringIO()
df_single_img_annots.to_csv(
csv_buffer,
index=False,
header=False,
sep=" ",
float_format="%.4f",
columns=[
"int_category",
"box_center_w",
"box_center_h",
"box_width",
"box_height",
],
)
s3_resource.Object(s3_bucket, destination).put(Body=csv_buffer.getvalue())
def get_cats(json_file):
"""
Makes a list of the category names in proper order
Input:
json_file: s3 path of the json file containing the category information
Returns:
cats: List of category names
"""
filesys = s3fs.S3FileSystem()
with filesys.open(json_file) as fin:
line = fin.readline()
record = json.loads(line)
labels = [item["label"] for item in record["labels"]]
return labels
def main():
"""
Performs the following tasks:
1. Reads input from 'input.json'
2. Collect the category names from the Ground Truth job
3. Creates a dataframe with annotaion in YOLO format
4. Saves a text file in S3 with YOLO annotations
for each of the labeled images
"""
with open("input.json") as fjson:
input_dict = json.load(fjson)
s3_bucket = input_dict["s3_bucket"]
job_id = input_dict["job_id"]
gt_job_name = input_dict["ground_truth_job_name"]
yolo_output = input_dict["yolo_output_dir"]
s3_path_cats = (
f"s3://{s3_bucket}/{job_id}/ground_truth_annots/{gt_job_name}/annotation-tool/data.json"
)
categories = get_cats(s3_path_cats)
print("n labels used in Ground Truth job: ")
print(categories, "n")
gt_annot_file = "annot.csv"
s3_dir = f"{job_id}/{yolo_output}"
print(f"annotation files saved in = ", s3_dir)
df_annot = annot_yolo(gt_annot_file, categories)
save_annots_to_s3(s3_bucket, s3_dir, df_annot)
if __name__ == "__main__":
main()
From the AWS CLI, save the preceding code block in a file create_annot.py
and run:
python create_annot.py
The annot_yolo
procedure transforms the dataframe you created by rescaling the box coordinates by the image size, and the save_annots_to_s3
procedure saves the annotations corresponding to each image into a text file and stores it in Amazon S3.
You can now inspect a couple of images and their corresponding annotations to make sure they’re properly formatted for model training. However, you first need to write a procedure to draw YOLO formatted bounding boxes on an image. Save the following code block in visualize.py
:
import matplotlib.pyplot as plt
import matplotlib.image as mpimg
import matplotlib.colors as mcolors
import argparse
def visualize_bbox(img_file, yolo_ann_file, label_dict, figure_size=(6, 8)):
"""
Plots bounding boxes on images
Input:
img_file : numpy.array
yolo_ann_file: Text file containing annotations in YOLO format
label_dict: Dictionary of image categories
figure_size: Figure size
"""
img = mpimg.imread(img_file)
fig, ax = plt.subplots(1, 1, figsize=figure_size)
ax.imshow(img)
im_height, im_width, _ = img.shape
palette = mcolors.TABLEAU_COLORS
colors = [c for c in palette.keys()]
with open(yolo_ann_file, "r") as fin:
for line in fin:
cat, center_w, center_h, width, height = line.split()
cat = int(cat)
category_name = label_dict[cat]
left = (float(center_w) - float(width) / 2) * im_width
top = (float(center_h) - float(height) / 2) * im_height
width = float(width) * im_width
height = float(height) * im_height
rect = plt.Rectangle(
(left, top),
width,
height,
fill=False,
linewidth=2,
edgecolor=colors[cat],
)
ax.add_patch(rect)
props = dict(boxstyle="round", facecolor=colors[cat], alpha=0.5)
ax.text(
left,
top,
category_name,
fontsize=14,
verticalalignment="top",
bbox=props,
)
plt.show()
def main():
"""
Plots bounding boxes
"""
labels = {0: "pen", 1: "pencil"}
parser = argparse.ArgumentParser()
parser.add_argument("img", help="image file")
args = parser.parse_args()
img_file = args.img
ann_file = img_file.split(".")[0] + ".txt"
visualize_bbox(img_file, ann_file, labels, figure_size=(6, 8))
if __name__ == "__main__":
main()
Download an image and the corresponding annotation file from Amazon S3. See the following code:
aws s3 cp s3://ground-truth-data-labeling/bounding_box/yolo_annot_files/IMG_20200816_205004.txt .
aws s3 cp s3://ground-truth-data-
labeling/bounding_box/images/IMG_20200816_205004.jpg .
To display the correct label of each bounding box, you need to specify the names of the objects you labeled in a dictionary and pass it to visualize_bbox
. For this use case, you only have two items in the list. However, the order of the labels is important—it should match the order you used while creating the Ground Truth labeling job. If you can’t remember the order, you can access the information from the s3://data-labeling-ground-truth/bounding_box/ground_truth_annots/bbox-yolo/annotation-tool/data.json
file in Amazon S3, which the Ground Truth job creates automatically.
The contents of the data.json
file the task look like the following code:
{"document-version":"2018-11-28","labels":[{"label":"pencil"},{"label":"pen"}]}
Therefore, a dictionary with the labels as follows was created in visualize.py
:
labels = {0: 'pencil', 1: 'pen'}
Now run the following to visualize the image:
python visualize.py IMG_20200816_205004.jpg
The following screenshot shows the bounding boxes correctly drawn around two pens.
To plot an image with a mix of pens and pencils, get the image and the corresponding annotation text from Amazon S3. See the following code:
aws s3 cp s3://ground-truth-data-labeling/bounding_box/yolo_annot_files/IMG_20200816_205029.txt .
aws s3 cp s3://ground-truth-data-
labeling/bounding_box/images/IMG_20200816_205029.jpg .
Override the default image size in the visualize_bbox procedure to (10, 12) and run the following:
python visualize.py IMG_20200816_205029.jpg
The following screenshot shows three bounding boxes correctly drawn around two types of objects.
Conclusion
This post described how to create an efficient, end-to-end data-gathering pipeline in Amazon Ground Truth for an object detection model. Try out this process yourself next time you are creating an object detection model. You can modify the post-processing annotations to produce labeled data in the Pascal VOC format, which is required for models like Faster RCNN. You can also adopt the basic framework to other data-labeling pipelines with job-specific modifications. For example, you can rewrite the annotation post-processing procedures to adopt the framework for an instance segmentation task, in which an object is labeled at the pixel level instead of drawing a rectangle around the object. Amazon Ground Truth gets regularly updated with enhanced capabilities. Therefore, check the documentation for the most up to date features.
About the Author
Arkajyoti Misra is a Data Scientist working in AWS Professional Services. He loves to dig into Machine Learning algorithms and enjoys reading about new frontiers in Deep Learning.
How distinguished scientist Garrett van Ryzin is optimizing his time at Amazon
van Ryzin is focusing on driving innovations in areas ranging from inventory management to last-mile delivery.Read More
Using speaker diarization for streaming transcription with Amazon Transcribe and Amazon Transcribe Medical
Conversational audio data that requires transcription, such as phone calls, doctor visits, and online meetings, often has multiple speakers. In these use cases, it’s important to accurately label the speaker and associate them to the audio content delivered. For example, you can distinguish between a doctor’s questions and a patient’s responses in the transcription of a live medical consultation.
Amazon Transcribe is an automatic speech recognition (ASR) service that makes it easy for developers to add speech-to-text capability to applications. With the launch of speaker diarization for streaming transcriptions, you can use Amazon Transcribe and Amazon Transcribe Medical to label the different speakers in real-time customer service calls, conference calls, live broadcasts, or clinical visits. Speaker diarziation or speaker labeling is critical to creating accurate transcription because of its ability to distinguish what each speaker said. This is typically represented by speaker A and speaker B. Speaker identification usually refers to when the speakers are specifically identified as Sally or Alfonso. With speaker diarization, you can request Amazon Transcribe and Amazon Transcribe Medical to accurately label up to five speakers in an audio stream. Although Amazon Transcribe can label more than five speakers in a stream, the accuracy of speaker diarization decreases if you exceed that number. In some cases, the different speakers may be on different channels (e.g. Call Center). In those cases you can use Amazon Transcribe Channel Identification to separate multiple channels from within a live audio stream to generate transcripts that label each audio channel
This post uses an example application to show you how to use the AWS SDK for Java to start a stream that enables you to stream your conversational audio from your microphone to Amazon Transcribe, and receive transcripts in real time with speaker labeling. The solution is a Java application that you can use to transcribe streaming audio from multiple speakers in real time. The application labels each speaker in the transcription results, which can be exported.
You can find the application in the GitHub repo. We include detailed steps to set up and run the application in this post.
Prerequisites
You need an AWS account to proceed with the solution. Additionally, the AmazonTranscribeFullAccess
policy is attached to the AWS Identity and Access Management (IAM) role you use for this demo. To create an IAM role with the necessary permissions, complete the following steps:
- Sign in to the AWS Management Console and open the IAM console.
- On the navigation pane, under Access management, choose Roles.
- You can use an existing IAM role to create and run transcription jobs, or choose Create role.
- Under Common use cases, choose EC2. You can select any use case, but EC2 is one of the most straightforward ones.
- Choose Next: Permissions.
- For the policy name, enter
AmazonTranscribeFullAccess
. - Choose Next: Tags.
- Choose Next: Review.
- For Role name, enter a role name.
- Remove the text under Role description.
- Choose Create role.
- Choose the role you created.
- Choose Trust relationships.
- Choose Edit trust relationship.
- Replace the trust policy text in your role with the following code:
{"Version": "2012-10-17",
"Statement": [
{"Effect": "Allow",
"Principal": {"Service": "transcribe.amazonaws.com"
},
"Action": "sts:AssumeRole"
}
]
}
Solution overview
Amazon Transcribe streaming transcription enables you to send a live audio stream to Amazon Transcribe and receive a stream of text in real time. You can label different speakers in either HTTP/2 or Websocket streams. Speaker diarization works best for labeling between two and five speakers. Although Amazon Transcribe can label more than five speakers in a stream, the accuracy of speaker separation decreases if you exceed five speakers.
To start an HTTP/2 stream, we specify the ShowSpeakerLabel request parameter of the StartStreamTranscription operation in our demo solution. See the following code:
private StartStreamTranscriptionRequest getRequest(Integer mediaSampleRateHertz) {
return StartStreamTranscriptionRequest.builder()
.languageCode(LanguageCode.EN_US.toString())
.mediaEncoding(MediaEncoding.PCM)
.mediaSampleRateHertz(mediaSampleRateHertz)
.showSpeakerLabel(true)
.build();
}
Amazon Transcribe streaming returns a “result”
object as part of the transcription response element that can be used to label the speakers in the transcript. To learn more about the parameters in this result object, see Response Syntax.
"TranscriptEvent":
{ "Transcript":
{ "Results":
[ { "Alternatives":
[ { "Items":
[ { "Content": "string",
"EndTime": number,
"Speaker": "string",
"StartTime": number,
"Type": "string",
"VocabularyFilterMatch": boolean } ],
"Transcript": "string" } ],
"EndTime": number,
"IsPartial": boolean,
"ResultId": "string",
"StartTime": number }
]
}
}
Our solution demonstrates speaker diarization during transcription for real-time audio captured via the microphone. Amazon Transcribe breaks your incoming audio stream based on natural speech segments, such as a change in speaker or a pause in the audio. The transcription is returned progressively to your application, with each response containing more transcribed speech until the entire segment is transcribed. For more information, see Identifying Speakers.
Launching the application
Complete the following prerequisites to launch the Java application. If you already have JavaFX or Java and Maven installed, you can skip the first two sections (Installing JavaFX and Installing Maven). For all environment variables mentioned in the following steps, a good option is to add it to the ~/.bashrc
file and apply these variables as required by typing “source ~/.bashrc”
after you open a shell.
Installing JDK
As your first step, download and install Java SE. When the installation is complete, set the JAVA_HOME
variable (see the following code). Make sure to select the path to the correct Java version and confirm the path is valid.
export JAVA_HOME=path-to-your-install-dir/jdk-14.0.2.jdk/Contents/Home
Installing JavaFX
For instructions on downloading and installing JavaFX, see Getting Started with JavaFX. Set up the environment variable as described in the instructions or by entering for following code (replace path/to with the directory where you installed JavaFX):
export PATH_TO_FX='path/to/javafx-sdk-14/lib'
Test your JavaFX installation as shown in the sample application on GitHub.
Installing Maven
Download the latest version of Apache Maven. For installation instructions, see Installing Apache Maven.
Installing the AWS CLI (Optional)
As an optional step, you can install the AWS Command Line Interface (AWS CLI). For instructions, see Installing, updating, and uninstalling the AWS CLI version 2. You can use the AWS CLI to validate and troubleshoot the solution as needed.
Setting up AWS access
Lastly, set up your access key and secret access key required for programmatic access to AWS. For instructions, see Programmatic access. Choose a Region closest to your location. For more information, see the Amazon Transcribe Streaming section in Service Endpoints.
When you know the Region and access keys, open a terminal window in your computer and assign them to environment variables for access within our solution:
- export AWS_ACCESS_KEY_ID=<access-key>
- export AWS_SECRET_ACCESS_KEY=<secret-access-key>
- export AWS_REGION=<aws region>
Solution demonstration
The following video demonstrates how you can compile and run the Java application presented in this post. Use the following sections to walk through these steps yourself.
The quality of the transcription results depends on many factors. For example, the quality can be affected by artifacts such as background noise, speakers talking over each other, complex technical jargon, the volume disparity between speakers, and the audio recording devices you use. You can use a variety of capabilities provided by Amazon Transcribe to improve transcription quality. For example, you can use custom vocabularies to recognize out-of-lexicon terms. You can even use custom language models, which enables you to use your own data to build domain-specific models. For more information, see Improving Domain-Specific Transcription Accuracy with Custom Language Models.
Setting up the solution
To implement the solution, complete the following steps:
- Clone the solution’s GitHub repo in your local computer using the following command:
git clone https://github.com/aws-samples/aws-transcribe-speaker-identification-java
- Navigate to the main directory of the solution
aws-transcribe-streaming-example-java
with the following code:
cd aws-transcribe-streaming-example-java
- Compile the source code and build a package for running our solution:
- Enter
mvn compile
. If the compile is successful, you should aBUILD SUCCESS
message. If there are errors in compilation, it’s most likely related to JavaFX path issues. Fix the issues based on the instructions in the Installing JavaFX section in this post. - Enter
mvn clean package
. You should see aBUILD SUCCESS
message if everything went well. This command compiles the source files and creates a packaged JAR file that we use to run our solution. If you’re repeating the build exercise, you don’t need to entermvn compile
every time.
- Enter
- Run the solution by entering the following code:
--module-path $PATH_TO_FX --add-modules javafx.controls -jar target/aws-transcribe-sample-application-1.0-SNAPSHOT-jar-with-dependencies.jar
If you receive an error, it’s likely because you already had a version of Java or JavaFX and Maven installed and skipped the steps to install JDK and JavaFX in this post. In so, enter the following code:
java -jar target/aws-transcribe-sample-application-1.0-SNAPSHOT-jar-with-dependencies.jar
You should see a Java UI window open.
Running the demo solution
Follow the steps in this section to run the demo yourself. You need two to five speakers present to try out the speaker diarization functionality. This application requires that all speakers use the same audio input when speaking.
- Choose Start Microphone Transcription in the Java UI application.
- Use your computer’s microphone to stream audio of two or more people (not more than five) conversing.
- As of this writing, Amazon Transcribe speaker labeling supports real-time streams that are in US English
You should see the speaker designations and the corresponding transcript appearing in the In-Progress Transcriptions window as the conversation progresses. When the transcript is complete, it should appear in the Final Transcription window.
- Choose Save Full Transcript to store the transcript locally in your computer.
Conclusion
This post demonstrated how you can easily infuse your applications with real-time ASR capabilities using Amazon Transcribe streaming and showcased an important new feature that enables speaker diarization in real-time audio streams.
With Amazon Transcribe and Amazon Transcribe Medical, you can use speaker separation to generate real-time insights from your conversations such as in-clinic visits or customer service calls and send these to downstream applications for natural language processing, or you can send it to human loops for review using Amazon Augmented AI (Amazon A2I). For more information, see Improving speech-to-text transcripts from Amazon Transcribe using custom vocabularies and Amazon Augmented AI.
About the Authors
Prem Ranga is an Enterprise Solutions Architect based out of Houston, Texas. He is part of the Machine Learning Technical Field Community and loves working with customers on their ML and AI journey. Prem is passionate about robotics, is an Autonomous Vehicles researcher, and also built the Alexa-controlled Beer Pours in Houston and other locations.
Talia Chopra is a Technical Writer in AWS specializing in machine learning and artificial intelligence. She works with multiple teams in AWS to create technical documentation and tutorials for customers using Amazon SageMaker, MxNet, and AutoGluon. In her free time, she enjoys meditating, studying machine learning, and taking walks in nature.
Parsa Shahbodaghi is a Technical Writer in AWS specializing in machine learning and artificial intelligence. He writes the technical documentation for Amazon Transcribe and Amazon Transcribe Medical. In his free time, he enjoys meditating, listening to audiobooks, weightlifting, and watching stand-up comedy. He will never be a stand-up comedian, but at least his mom thinks he’s funny.
Mahendar Gajula is a Sr. Data Architect at AWS. He works with AWS customers in their journey to the cloud with a focus on data lake, data warehouse, and AI/ML projects. In his spare time, he enjoys playing tennis and spending time with his family.
Optimizing the cost of training AWS DeepRacer reinforcement learning models
AWS DeepRacer is a cloud-based 3D racing simulator, an autonomous 1/18th scale race car driven by reinforcement learning, and a global racing league. Reinforcement learning (RL), an advanced machine learning (ML) technique, enables models to learn complex behaviors without labeled training data and make short-term decisions while optimizing for longer-term goals. But as we humans can attest, learning something well takes time—and time is money. You can build and train a simple “all-wheels-on-track” model in the AWS DeepRacer console in just a couple of hours. However, if you’re building complex models involving multiple parameters, a reward function using trigonometry, or generally diving deep into RL, there are steps you can take to optimize the cost of training.
As a Senior Solutions Architect and an AWS DeepRacer PitCrew member, you ultimately rack up a lot of training time. Recently we shared tips for keeping it frugal with Blaine Sundrud, host of DeepRacer TV News. This post discusses that advice in more detail. To see the interview, check out the August 2020 Qualifiers edition of DRTV.
Also, look out for the cost-optimization article coming soon to the AWS DeepRacer Developer Guide for step-by-step procedures on these topics.
The AWS DeepRacer console provides you with many tools to help you get the most of training and evaluating your RL models. After you build a model based on a reward function, which is the incentive plan you create for the agent, your AWS DeepRacer vehicle, you need to train it. This means you enable the agent to explore various actions in its environment, which, for your vehicle is its track. There it attempts to take actions that result in rewards. Over time it learns the behaviors that will lead to a maximum reward—training time that takes machine time and costs money. My goal is to share how avoiding overtraining, validating your model, analyzing logs, using transfer learning, and creating a budget can help keep the focus on fun, not cost.
Overview
In this post, we walk you through some strategies for training better performing and more cost-effective AWS DeepRacer models:
- Avoid overtraining your RL model
- Validate each RL model early in the process
- Analyze logs to further assess success
- Experiment with transfer learning
- Create a budget using the AWS Billing and Cost Management dashboard on the AWS Management Console
Avoid overtraining
When training an RL model, more isn’t always better. Training longer than necessary can lead to overfitting, which means a model doesn’t adapt, or generalize well, from the environment it’s trained in to a novel environment, real or online. For AWS DeepRacer, a model that is overfit may perform well on a virtual track, but conditions like gravity, shadows on the track, the friction of the wheels on the track, wear in the gears, degradation of the battery, and even smudges on the camera lens can lead to the car running slowly or veering off a replica of that track in the real world. When training and racing exclusively in the AWS DeepRacer console, a model overfitted to an oval track will not do as well on a track with s-curves. In practical terms, you can think of an email spam filter that has been overtrained on messages about window replacements, credit card programs, and rich relatives in foreign lands. It might do an excellent job detecting spam related to those topics, but a terrible job finding spam related to scam insurance plans, gutters, home food delivery, and more original get-rich-quick schemes. To learn more about overfitting, watch AWS DeepRacer League – Overfitting.
We now know overtraining that leads to overfitting isn’t a good thing, but one of the first lessons an ML practitioner learns is that undertraining isn’t good either. So how much training is enough? The key is to stop training at the point when performance begins to degrade. With AWS DeepRacer, the Training Reward graph shows the cumulative reward received per training episode. You can expect this graph to be volatile initially, but over time the graph should trend upwards and to the right, and, as your model starts converging, the average should flatten out. As you watch the reward graph, also keep an eye on the agent’s driving behavior during training. You should stop training when the percentage of the track the car completes is no longer improving. In the following image, you can see a sample reward graph with the “best model” indicated. When the model’s track completion progress per episode continuously reaches 100% and the reward levels out, more training will lead to overfitting, a poorly generalized model, and wasted training time.
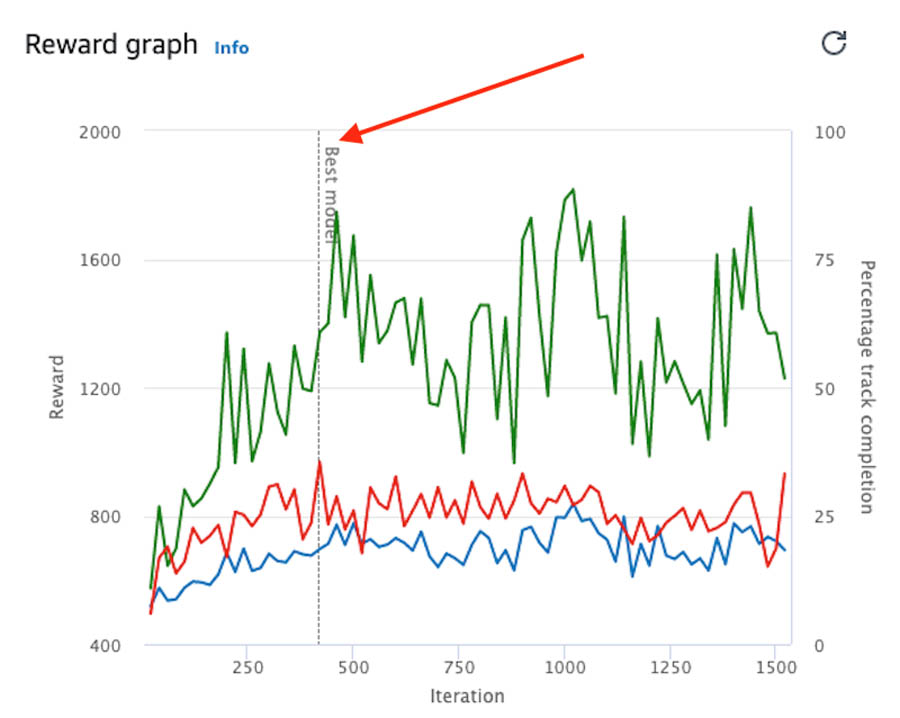
When to stop training
Validate your model
A reward function describes the immediate feedback, as a reward or penalty score, your model receives when your AWS DeepRacer vehicle moves from one position on the track to a new one. The function’s purpose is to encourage the vehicle to make moves along the track that reach a destination quickly, without incident or accident. A desirable move earns a higher score for the action, or target state, and an illegal or wasteful move earns a lower score. It may seem simple, but it’s easy to overlook errors in your code or find that your reward function unintentionally incentivizes undesirable moves. Validating your reward function both in theory and practice helps you avoid wasting time and money training a model that doesn’t do what you want it to do.
The validate function is similar to a Python lint tool. Choosing Validate checks the syntax of the reward function, and if successful, results in a “passed validation” message.
After checking the code, validate the performance of your reward function early and often. When first experimenting with a new reward function, train for a short period of time, such as 15 minutes, and observe the results to determine whether or not the reward function is performing as expected. Look at the reward results and percentage of track completion on the reward graph to see that they’re increasing (see the following example graph). If it looks like a well performing model, you can clone that model and train for additional time or start over with the same reward function. If the reward doesn’t improve, you can investigate and make adjustments without wasting training time and putting a dent in your pocketbook.
Analyze logs to improve efficiency
Focusing on the training graph alone does not give you a complete picture. Fortunately, AWS DeepRacer produces logs of actions taken during training. Log analysis involves a detailed look at the outputs produced by the AWS DeepRacer training job. Log analysis might involve an aggregation of the model’s performance at various locations on the track or at different speeds. Analysis often includes various kinds of visualization, such as plotting the agent’s behavior on the track, the reward values at various times or locations, or even plotting the racing line around the track to make sure you’re not oversteering and that your agent is taking the most efficient path. You can also include Python print() statements in your reward function to output interim results to the logs for each iteration of the reward function.
Without studying the logs, you’re likely only making guesses about where to improve. It’s better to rely on data to make these adjustments. You usually get a better model sooner by studying the logs and tweaking the reward function. When you get a decent model, try conducting log analysis before investing in further training time.
The following graph is an example of plotting the racing line around a track.
For more information about log analysis, see Using Jupyter Notebook for analysing DeepRacer’s logs.
Try transfer learning
In ML, as in life, there is no point in reinventing the wheel. Transfer learning involves relying on knowledge gained while solving one problem and applying it to a different, but related, problem. The shape of the AWS DeepRacer Convolutional Neural Network (CNN) is determined by the number of inputs (such as the cameras or LIDAR) and the outputs (such as the action space). A new model has weights set to random values, and a certain amount of training is required to converge to get a working model.
Instead of starting with random weights, you can copy an existing trained model. In the AWS DeepRacer environment, this is called cloning. Cloning works by making a deep copy of the neural network—the AWS DeepRacer CNN—including all the nodes and their weights. This can save training time and money.
The learning rate is one of the hyperparameters that controls the RL training. During each update, a portion of the new weight for each node results from the gradient-descent (or ascent) contribution, and the rest comes from the existing node weight. The learning rate controls how much a gradient-descent (or ascent) update contributes to the network weights. If you are interested in learning more about gradient descent, check out this post on optimizing deep learning.
You can use a higher learning rate to include more gradient-descent contributions for faster training, but the expected reward may not converge if the learning rate is too large. Try setting the learning rate reasonably high for the initial training. When it’s complete, clone and train the network for additional time with a reduced learning rate. This can save a significant amount of training time by allowing you to train quickly at first and then explore more slowly when you’re nearing an optimal solution.
Developers often ask why they can’t modify the action space during or after cloning. It’s because cloning a model results in a duplicate of the original network, and both the inputs and the action space are fixed. If you increase the action space, the behavior of a network with additional output nodes that had no connections to the other layers and no weights is unpredictable, and could lead to a lot more training or even a model that can’t converge at all. CNNs with node weights equal to zero are unpredictable. The nodes might even be deactivated (recall that 0 times anything is 0). Likewise, pruning one or more nodes from the output layer also drives unknown outcomes. Both situations require additional training to ensure the model works as expected, and there is no guarantee it will ever converge. Radically changing the reward function may result in a cloned model that doesn’t converge quickly or at all, which is a waste of time and money.
To try transfer learning following steps in the AWS DeepRacer Developer Guide, see Clone a Trained Model to Start a New Training Pass.
Create a budget
So far, we’ve looked at things you can do within the RL training process to save money. Aside from those I’ve discussed in the AWS DeepRacer console, there is another tool in AWS Management console that can help you keep your spend where you want it—AWS Budgets. You can set monthly, quarterly, and annual budgets for cost, usage, reservations, and savings plans.
On the Cost Management page, choose Budgets and create a budget for AWS DeepRacer.
To set a budget, sign in to the console and navigate to AWS Budgets. Then select a period, effective dates, and a budget amount. Next, configure an alert so that you receive an email notification when usage exceeds a stated percentage of that budget.
You can also configure an Amazon Simple Notification Service (Amazon SNS) topic to have chatbot alerts sent to Amazon Chime or Slack.
Clean up when done
When you’re done training, evaluating, and racing, it’s good practice to shut down unneeded resources and perform cleanup actions. Storage costs are minimal, but delete any models or log files that aren’t needed. If you used Amazon SageMaker or AWS RoboMaker, save and stop your notebooks and if they are no longer needed, delete them. Make sure you end any running training jobs in both services.
Conclusion
In this post, we covered several tips for optimizing spend for AWS DeepRacer, which you can apply to many other ML projects. Try any or all of these tips to minimize your expenses while having fun learning ML, by getting started in the AWS DeepRacer Console today!
About the Authors
Tim O’Brien brings over 30 years of experience in information technology, security, and accounting to his customers. Tim has worked as a Senior Solutions Architect at AWS since 2018 and is focused on Machine Learning and Artificial Intelligence.
Previously, as a CTO and VP of Engineering, he led product design and technical delivery for three startups. Tim has served numerous businesses in the Pacific Northwest conducting security related activities, including data center reviews, lottery security reviews, and disaster planning.
A wordsmith, futurist, and relatively fresh recruit to the position of technical writer – AI/ML at AWS, Heather Johnston-Robinson is excited to leverage her background as a maker and educator to help people of all ages and backgrounds find and foster their spark of ingenuity with AWS DeepRacer. She recently migrated from adventures in the maker world with Foxbot Industries, Makerologist, MyOpen3D, and LEGO robotics to take on her current role at AWS.
Using log analysis to drive experiments and win the AWS DeepRacer F1 ProAm Race
This is a guest post by Ray Goh, a tech executive at DBS Bank.
AWS DeepRacer is an autonomous 1/18th scale race car powered by reinforcement learning, and the AWS DeepRacer League is the world’s first global autonomous racing league. It’s a fun and easy way to get started with machine learning (ML), regardless of skill or background. For companies, it’s also a powerful platform to facilitate teaching ML to employees at the enterprise level.
As part of our digital transformation journey at DBS Bank, we’re taking innovative steps to future-proof our workforce. We’ve partnered with AWS to bring the AWS DeepRacer League to DBS to train over 3,000 employees in AI and ML by the end of 2020. Thanks to the AWS DeepRacer virtual simulation and training environment, our employees can upgrade their skills and pick up new knowledge, even when they aren’t physically in the office. The ability to run private races also allows us to create our own racing league, where our employees can put their newly learned skills to the test.
Winning the F1 ProAm Race in May 2020
As an individual racer, I’ve been active in the AWS DeepRacer League since 2019. In May 2020, racers from around the world had the unique opportunity to pit their ML skills against F1 professionals in the AWS DeepRacer F1 ProAm Race. We trained our models on a replica of the F1 Spanish Grand Prix track, and the top 10 racers from the month-long, head-to-head qualifying race faced off against F1 professional drivers Daniel Ricciardo and Tatiana Calderon in a Grand Prix-style race. Watch the AWS DeepRacer ProAm series here.
After a challenging month of racing, I emerged as the champion in the F1 ProAm Race, beating fellow racers and the pro F1 drivers to the checkered flag! Looking back now, I attribute my win to having performed many experiments throughout the month of racing. Those experiments allowed me to continuously tweak and improve my model leading up to the final race. Behind those experiments are ideas that arose from data-driven insights through log analysis.
What is log analysis?
Log analysis is using a Jupyter notebook to analyze and debug models based on log data generated from the AWS DeepRacer simulation and training environment. With snippets of Python code, you can plot and visualize your model’s training performance through various graphs and heatmaps. I created several unique visualizations that ultimately helped me train a model that was fast and stable enough to win the F1 ProAm Race.
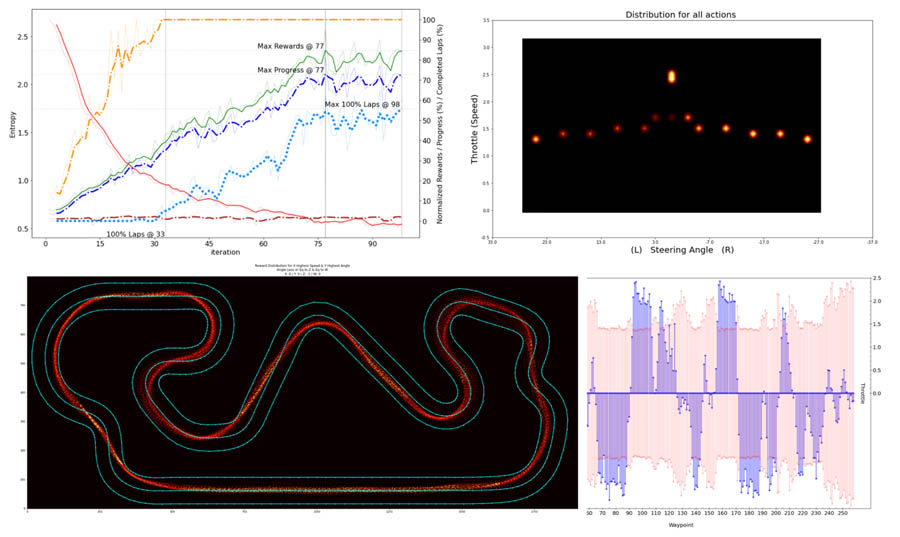
Figure 1 Log analysis visualizations
In this post, I share some of the visualizations I created and show how you can use Amazon SageMaker to spin up a notebook instance to perform log analysis using DeepRacer model training data.
If you’re already familiar with opening notebooks in a JupyterLab notebook application, you can simply clone my log analysis repository and skip directly to the log analysis section.
Amazon SageMaker notebook instances
An Amazon SageMaker notebook instance is a managed ML compute instance running the Jupyter notebook application. Amazon SageMaker manages the creation of the instance and its related resources, so we can focus on analyzing the data collected during training without worrying about provisioning Amazon Elastic Compute Cloud (Amazon EC2) or storage resources directly.
Using an Amazon SageMaker notebook instance for log analysis
One of the greatest benefits of using an Amazon SageMaker notebook instance to perform AWS DeepRacer log analysis is that Amazon SageMaker automatically installs Anaconda packages and libraries for common deep learning platforms on our behalf, including TensorFlow deep learning libraries. It also automatically attaches an ML storage volume to our notebook instance, which we can use as a persistent working storage to perform log analysis and retain our analysis artifacts.
Creating a notebook instance
To get started, create a notebook instance on the Amazon SageMaker console.
- On the Amazon SageMaker console, under Notebook, choose Notebook instances.
- Choose Create notebook instance.
- For Notebook instance name, enter a name (for example, DeepRacer-Log-Analysis).
- For Notebook instance type¸ choose your instance.
For AWS DeepRacer log analysis, the smallest instance type (ml.t2.medium) is usually sufficient.
- For Volume size in GB, enter your storage volume size. For this post, we enter 5.
When the notebook instance shows an InService status, we can open JupyterLab, the IDE for Jupyter notebooks.
- Locate your notebook instance and choose Open JupyterLab.
Cloning the log analysis repo from JupyterLab
From the JupyterLab IDE, we can easily clone a Git repository to use log analysis notebooks shared by the community. For example, I can clone my log analysis repository in seconds, using https://github.com/TheRayG/deepracer-log-analysis.git as the Clone URI.
After cloning the repository, we should see it appear in the folder structure on the left side of the JupyterLab IDE.
Downloading logs from the AWS DeepRacer console
To prepare the data that we want to analyze, we have to download our model training logs from the AWS DeepRacer console.
- On the AWS DeepRacer console, under Reinforcement learning, choose Your models.
- Choose the model to analyze.
- In the Training section, under Resources, choose Download Logs.
This downloads the training log files, which are packaged in a .tar.gz file.
Extracting the required log files for analysis
In this step, we complete the final configurations.
- Extract the RoboMaker and Amazon SageMaker log files from the .tar.gz package (found in the logs/training/ subdirectory).
- Upload the two log files into the /deepracer-log-analysis/logs folder in the JupyterLab IDE.
We’re now ready to open up our log analysis notebook to work its magic!
- Navigate to the /deepracer-log-analysis folder on the left side of the IDE and choose the .ipynb file to open the notebook.
- When opening the notebook, you may be prompted to provide a kernel. Choose a kernel that uses Python 3, such as conda_tensorflow_p36.
- Wait until the kernel status changes from Starting to Idle.
- Edit the notebook to specify the path and names of the two log files that we just uploaded.
To perform our visualizations, we use the simulation trace data from the RoboMaker log file and policy update data from the Amazon SageMaker log file. We parse the data in the notebook using pandas dataframes, which are two-dimensional labeled data structures like spreadsheets or SQL tables.
For the RoboMaker log file, we aggregate important information, such as minimum, maximum, and average progress and lap completion ratios for each iteration of training episodes.
For the Amazon SageMaker log file, we calculate the average entropy per epoch in each policy update iteration.
Performing visualizations
We can now run the notebook by choosing Run and Run All Cells in JupyterLab. My log analysis notebook contains numerous markdown descriptions and comments to explain what each cell does. In this section, I highlight some of the visualizations from that notebook and explain some of the thought processes behind them.
Visualizing the performance envelope of the model
A common question asked by beginners of AWS DeepRacer is, “If two models are trained for the same amount of time using the same reward function and hyperparameters, why do they have different lap times when I evaluate them?”
The following visualization is a great way to explain it; it shows the frequency of performance to lap time in seconds.
I use this to illustrate the performance envelope of my model. We can show the relative probability of the model achieving various lap times by plotting a histogram of lap times achieved by the model during training. We can also work out statistically the average and best-case lap times that we can expect from the model. I’ve noticed that the lap times of the model during training resembles a normal distribution, so I use the -2 and -3 Std Dev markers to show the potential best-case lap times for the model, albeit with just 2.275% (-2 SD) and 0.135% (-3 SD) chance of occurring respectively. By understanding the likelihood of the model achieving a given lap time and comparing that to leaderboard times, I can gauge if I should continue cloning and tweaking the model, or abandon it and start fresh with a different approach.
Identifying potential model checkpoints for race submission
When training many different models for a race, racers commonly ask, “Which model would give me the highest chance of winning a virtual race?”
To answer that question, I plot the top quartile (p25) lap times vs. iterations from the training data, which identifies potential models for race submission. This scatter plot also allows me to identify potential trade-offs between speed (dots with very fast lap times) and stability (dense cluster of dots for a particular iteration). From the following diagram, I would choose models from the three highlighted iterations for race submission.
Identifying convergence and gauging consistency
As racers gain experience with model training, they start paying attention to convergence in their models. Simply put, convergence in the AWS DeepRacer context is when a model is performing close to its best (in terms of average lap progress), and further training may harm its performance or make it overfit, such that it only does well for that track in a very specific simulation environment, but not in other tracks or in a physical AWS DeepRacer car. That begs the following questions: “How do I tell when the model has converged?” and “How consistent is my model after it has converged?”
To aid in visualizing convergence, I overlay the entropy information from the Amazon SageMaker policy training logs over the usual plots for rewards and progress.
Entropy is a measure of the amount of randomness in our reinforcement learning neural network. At the beginning of model training, entropy is high, because our neural network is updated mostly based on random actions as the car explores the track.
Over time, with more experiences gained from actions and rewards at various parts of the track, the car starts to exploit this information and takes less random actions.
The thinking behind this is that, as rewards and progress increase, the entropy value should decrease. When rewards and progress plateau, the entropy loss should also flatten out. Therefore, I use entropy as an additional indicator for convergence.
To gauge the consistency of my model, I also plot the percentage of lap completions per iteration during training. When the model is capable of completing laps, the percentage of completed laps should creep up in subsequent iterations, until around the point of convergence, when the percentage value should plateau too. See the following plot.
The model training process is probabilistic because the reinforcement learning agent incorporates entropy to explore the environment. To smooth out the effects of the probabilistic model in my visualization, I use a simple moving average over three iterations for each of my plotted metrics.
Identifying inefficiencies in driving behavior
When racers have a competitive model, they may start to wonder, “Are there sections of the track where the car is driving inefficiently? What are the sections where I can encourage the car to speed up?”
In pursuit of answering these questions, I designed a visualization that shows the average speed and steering angle of the car measured at every waypoint along the track. This allows me to see how the model is negotiating the track, because from this plot, you can see the rate at which the model is speeding up or slowing down as it travels through the waypoints. The following visualization shows the deviation of the optimal racing line (orange) from the track centerline (blue).
You can also see how the model adjusts its steering angle as it negotiates turns. What I love about the following visualization is that it allows me to see clearly at which point after a long straight the model starts to brake before entering into a turn. It also helps me visualize if a model is accelerating quickly enough upon exiting a turn.
Identifying track sections to adjust actions and rewards
Although speed is the primary performance criteria in a time trial race, stability is also important in an object avoidance or head-to-head race. Because time penalties for going off-track impact race position, it’s very important to find the right balance between speed and stability. Even if the model can negotiate the track well, top racers are also asking, “Is the car over- or under-steering at any of the turns? Which turn should I focus on optimizing in subsequent experiments?”
By plotting a heatmap of rewards over the track, you can easily see how consistently we reward the model at various parts of the track. A thin band in the heatmap reflects very consistent rewards, while a sparse scattering of dots brings attention to the parts of the track where the model has trouble getting rewards. For my reward function, this usually highlights the turns at which the model is over- or under-steering.
For example, in the highlighted parts of the preceding plot, the model isn’t consistently going around those turns according to the racing line that I’m rewarding for. It’s actually over-steering as it exits Turn 3 (around waypoint 62), and under-steering around the other two highlighted turns. Tweaking the action space may help (in the case of under-steering, lowering the speed at high steering angles). Interestingly, the lap completion rate of the model can increase substantially with such minor tweaks, without sacrificing lap times!
Experiment, Experiment, Experiment
For the F1 ProAm Race that in May 2020, I planned to do two experiments per day (at least 60 experiments total) to try out different reward strategies and racing lines. I could iterate quickly while focusing on incremental improvements by using log analysis to surface insights from the training data.
For example, the following plot helped me answer the question “Is the car going to go as fast as possible through the entire lap?” by showing where the car uses 0-degree and highest speeds.
Cleaning up
To save on ML compute costs, when you’re done with log analysis, you can stop the notebook instance without deleting it. The notebook, data, and log files are still retained as long as you don’t delete the notebook instance. A stopped instance still incurs cost for the provisioned ML storage. But you can always restart the instance later to continue working on the notebook.
When you no longer need the notebook or data, you can permanently delete the instance, which also deletes the attached ML storage volume, so that you no longer incur its related ML storage cost.
For pricing details for Amazon SageMaker notebook instances, see Amazon SageMaker Pricing.
Conclusion
The visualizations I shared with you in this post helped me win the May 2020 F1 ProAm Race against other top racers and F1 pros, so it’s my hope that by sharing these ideas with the community, others can benefit and learn from them too.
Together as a community of practice, we can help to accelerate learning for everyone and raise the bar for the AI/ML community in general!
You can start training your own model and improve it through log analysis by signing in to the AWS DeepRacer console.
About the Author
Ray Goh is a Tech executive who leads Agile Teams in the delivery of FX Trading & Digital Solutions at DBS Bank. He is a passionate Cloud advocate with deep interest in Voice and Serverless technology, and has 8 AWS Certifications under his belt. He is also active in the DeepRacer (a Machine Learning autonomous model car) community. Obsessed with home automation, he owns close to 20 Alexa-enabled devices at home and in the car.
Amazon Personalize improvements reduce model training time by up to 40% and latency for generating recommendations by up to 30%
We’re excited to announce new efficiency improvements for Amazon Personalize. These improvements decrease the time required to train solutions (the machine learning models trained with your data) by up to 40% and reduce the latency for generating real-time recommendations by up to 30%.
Amazon Personalize enables you to build applications with the same machine learning (ML) technology used by Amazon.com for real-time personalized recommendations—no ML expertise required. Amazon Personalize provisions the necessary infrastructure and manages the entire ML pipeline, including processing the data, identifying features, using the best algorithms, and training, optimizing, and hosting the models.
When serving recommendations, minimizing the time your system takes to generate and serve a recommendation improves conversion. A 2017 Akamai study shows that every 100-millisecond delay in website load time can hurt conversion rates by 7%.[1] All other things being equal, lower latency is better. Our efficiency improvements have generated latency reductions of up to 30% for user recommendations across the full range of item catalogs supported in Amazon Personalize.
As your datasets grow and your users’ behavior changes, regular retraining is needed to keep your recommendations relevant. Solution training is one of the three cost drivers when using Amazon Personalize and can be a significant portion of your overall cost of ownership for Amazon Personalize. Improved training efficiency in Amazon Personalize reduces the cost of training solutions and increases the speed at which you can deploy new recommendation solutions for your users. New solution versions ensure that your Amazon Personalize model includes the most recent user events and that new items in your catalog are included in your personalized recommendations. The relative popularity of items changes as user preferences shift and when your catalog changes. Now, you can maintain the relevance of your recommendations at a lower cost and in less time.
The following sections walk you through how to use Amazon Personalize.
Creating dataset groups and datasets
When you get started with Amazon Personalize, the first step is to create a dataset group and import data about your users, your item catalog, and your users’ interaction history with those items. Each dataset group contains three distinct datasets: user-item interaction data, item, data, and user data. If you don’t have historical data or if you want to ensure you generate the most relevant recommendations based on in-session behavior, real-time user-item interactions (events) can be recorded using the putEvents
API. New items and user records can be added incrementally to your item and user datasets using the putItems
and putUsers
APIs, allowing you to update not only your model’s recent user actions but also ensure the most current item and user data is available when updating or retraining your solutions.
Creating an interaction dataset
Use the Amazon Personalize console to create an interaction dataset, with the following schema and import the file bandits-demo-interactions.csv, which is a synthetic movie rating dataset:
{
"type": "record",
"name": "Interactions",
"namespace": "com.amazonaws.personalize.schema",
"fields": [
{
"name": "USER_ID",
"type": "string"
},
{
"name": "ITEM_ID",
"type": "string"
},
{
"name": "EVENT_TYPE",
"type": "string"
},
{
"name": "EVENT_VALUE",
"type": ["null","float"]
},
{
"name": "TIMESTAMP",
"type": "long"
},
{
"name": "IMPRESSION",
"type": "string"
}
],
"version": "1.0"
}
Creating an item dataset
You follow similar steps to create an item dataset and import your data using bandits-demo-items.csv, which has metadata for each movie. We use an optional reserved keyword CREATION_TIMESTAMP
for the item dataset, which helps Amazon Personalize compute the age of the item and adjust recommendations accordingly.
If you don’t provide the CREATION_TIMESTAMP
, the model infers this information from the interaction dataset and uses the timestamp of the item’s earliest interaction as its corresponding release date. If an item doesn’t have an interaction, its release date is set as the timestamp of the latest interaction in the training set and it is considered a new item with age 0.
Our dataset for this post has 1,931 movies, of which 191 have a creation timestamp marked as the latest timestamp in the interaction dataset. These newest 191 items are considered cold items and have a label number higher than 1800 in the dataset.
Create your dataset and import the data with the following item dataset schema:
{
"type": "record",
"name": "Items",
"namespace": "com.amazonaws.personalize.schema",
"fields": [
{
"name": "ITEM_ID",
"type": "string"
},
{
"name": "GENRES",
"type": ["null","string"],
"categorical": true
},
{
"name": "TITLE",
"type": "string"
},
{
"name": "CREATION_TIMESTAMP",
"type": "long"
}
],
"version": "1.0"
}
Training a model
After the dataset import jobs are complete, you’re ready to train a model.
- On the Amazon Personalize console, in the navigation pane, choose Solutions.
- Choose Create solution.
- For Solution name, enter your name.
- For Recipe, choose aws-user-personalization.
This recipe combines deep learning models (RNNs) with bandits to provide you more accurate user modeling (high relevance) while also allowing for effective exploration of new items.
- Leave the Solution configuration section at its default values and choose Next.
- On the Create solution version page, choose Finish to start training.
When the training is complete, you can navigate to the Solution Version Overview page to see the offline metrics.
Creating a campaign
In this step, you create a campaign using the solution created in the previous step.
- On the Amazon Personalize console, choose Campaigns.
- Choose Create Campaign.
- For Campaign name, enter a name.
- For Solution, choose user-personalization-solution.
- For Solution version ID, choose the solution version that uses the
aws-user-personalization
recipe.
Retraining and updating campaigns
To update a model (solutionVersion
), you can call the createSolutionVersion
API with trainingMode
set to UPDATE
. This updates the model with the latest item information for the item in the dataset used to train the solution previously and adjusts the exploration according to implicit feedback from the users. This is not equivalent to training a model, which you can do by setting trainingMode
to FULL
. Full training should be done less frequently, typically one time every 1–5 days depending on your use case.
When the new solutionVersion
is created, you can update the campaign using the UpdateCampaign
API or on the Amazon Personalize console to get recommendations using it.
Conclusion
Product and content recommendations are only one part of an overarching personalization experience. End-to-end latency budgets require fast responses, and unnecessary latency decreases the impact and value of personalization for your users and business. The reduced latency of recommendations generated by Amazon Personalize has improved the speed at which you can generate recommendations for your users. Additionally, the improved efficiency of training Amazon Personalize ensures that your recommendations maintain relevance at a lower cost. For more information about training and deploying personalized recommendations for your users with Amazon Personalize, see What Is Amazon Personalize?
[1] https://www.akamai.com/us/en/multimedia/documents/report/akamai-state-of-online-retail-performance-2017-holiday.pdf
About the Authors
Deepesh Nathani is a Software Engineer with Amazon Personalize focused on building the next generation recommender systems. He is a Computer Science graduate from New York University. Outside of work he enjoys water sports and watching movies.
Venkatesh Sreenivas is a Senior Software Engineer at Amazon Personalize and works on building distributed data science pipelines at scale. In his spare time, he enjoys hiking and exploring new technologies.
Matt Chwastek is a Senior Product Manager for Amazon Personalize. He focuses on delivering products that make it easier to build and use machine learning solutions. In his spare time, he enjoys reading and photography.