Customers across all industries are experimenting with generative AI to accelerate and improve business outcomes. Generative AI is used in various use cases, such as content creation, personalization, intelligent assistants, questions and answers, summarization, automation, cost-efficiencies, productivity improvement assistants, customization, innovation, and more.
Generative AI solutions often use Retrieval Augmented Generation (RAG) architectures, which augment external knowledge sources for improving content quality, context understanding, creativity, domain-adaptability, personalization, transparency, and explainability.
This post dives deep into Amazon Bedrock Knowledge Bases, which helps with the storage and retrieval of data in vector databases for RAG-based workflows, with the objective to improve large language model (LLM) responses for inference involving an organization’s datasets.
Benefits of vector data stores
Several challenges arise when handling complex scenarios dealing with data like data volumes, multi-dimensionality, multi-modality, and other interfacing complexities. For example:
- Data such as images, text, and audio need to be represented in a structured and efficient manner
- Understanding the semantic similarity between data points is essential in generative AI tasks like natural language processing (NLP), image recognition, and recommendation systems
- As the volume of data continues to grow rapidly, scalability becomes a significant challenge
- Traditional databases may struggle to efficiently handle the computational demands of generative AI tasks, such as training complex models or performing inference on large datasets
- Generative AI applications frequently require searching and retrieving similar items or patterns within datasets, such as finding similar images or recommending relevant content
- Generative AI solutions often involve integrating multiple components and technologies, such as deep learning frameworks, data processing pipelines, and deployment environments
Vector databases serve as a foundation in addressing these data needs for generative AI solutions, enabling efficient representation, semantic understanding, scalability, interoperability, search and retrieval, and model deployment. They contribute to the effectiveness and feasibility of generative AI applications across various domains. Vector databases offer the following capabilities:
- Provide a means to represent data in a structured and efficient manner, enabling computational processing and manipulation
- Enable the measurement of semantic similarity by encoding data into vector representations, allowing for comparison and analysis
- Handle large-scale datasets efficiently, enabling processing and analysis of vast amounts of information in a scalable manner
- Provide a common interface for storing and accessing data representations, facilitating interoperability between different components of the AI system
- Support efficient search and retrieval operations, enabling quick and accurate exploration of large datasets
To help implement generative AI-based applications securely at scale, AWS provides Amazon Bedrock, a fully managed service that enables deploying generative AI applications that use high-performing LLMs from leading AI startups and Amazon. With the Amazon Bedrock serverless experience, you can experiment with and evaluate top foundation models (FMs) for your use cases, privately customize them with your data using techniques such as fine-tuning and RAG, and build agents that run tasks using enterprise systems and data sources.
In this post, we dive deep into the vector database options available as part of Amazon Bedrock Knowledge Bases and the applicable use cases, and look at working code examples. Amazon Bedrock Knowledge Bases enables faster time to market by abstracting from the heavy lifting of building pipelines and providing you with an out-of-the-box RAG solution to reduce the build time for your application.
Knowledge base systems with RAG
RAG optimizes LLM responses by referencing authoritative knowledge bases outside of its training data sources before generating a response. Out of the box, LLMs are trained on vast volumes of data and use billions of parameters to generate original output for tasks like answering questions, translating languages, and completing sentences. RAG extends the existing powerful capabilities of LLMs to specific domains or an organization’s internal knowledge base, all without the need to retrain the model. It’s a cost-effective approach to improving an LLM’s output so it remains relevant, accurate, and useful in various contexts.
The following diagram depicts the high-level steps of a RAG process to access an organization’s internal or external knowledge stores and pass the data to the LLM.
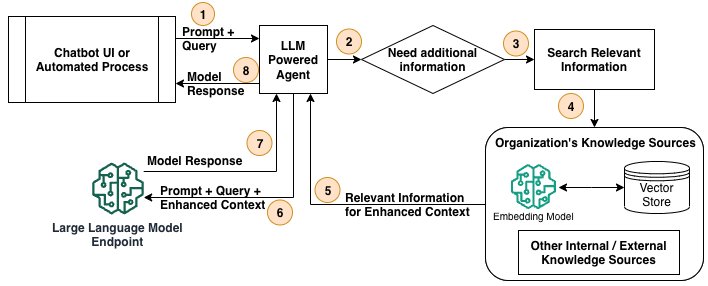
The workflow consists of the following steps:
- Either a user through a chatbot UI or an automated process issues a prompt and requests a response from the LLM-based application.
- An LLM-powered agent, which is responsible for orchestrating steps to respond to the request, checks if additional information is needed from knowledge sources.
- The agent decides which knowledge source to use.
- The agent invokes the process to retrieve information from the knowledge source.
- The relevant information (enhanced context) from the knowledge source is returned to the agent.
- The agent adds the enhanced context from the knowledge source to the prompt and passes it to the LLM endpoint for the response.
- The LLM response is passed back to the agent.
- The agent returns the LLM response to the chatbot UI or the automated process.
Use cases for vector databases for RAG
In the context of RAG architectures, the external knowledge can come from relational databases, search and document stores, or other data stores. However, simply storing and searching through this external data using traditional methods (such as keyword search or inverted indexes) can be inefficient and might not capture the true semantic relationships between data points. Vector databases are recommended for RAG use cases because they enable similarity search and dense vector representations.
The following are some scenarios where loading data into a vector database can be advantageous for RAG use cases:
- Large knowledge bases – When dealing with extensive knowledge bases containing millions or billions of documents or passages, vector databases can provide efficient similarity search capabilities.
- Unstructured or semi-structured data – Vector databases are particularly well-suited for handling unstructured or semi-structured data, such as text documents, webpages, or natural language content. By converting the textual data into dense vector representations, vector databases can effectively capture the semantic relationships between documents or passages, enabling more accurate retrieval.
- Multilingual knowledge bases – In RAG systems that need to handle knowledge bases spanning multiple languages, vector databases can be advantageous. By using multilingual language models or cross-lingual embeddings, vector databases can facilitate effective retrieval across different languages, enabling cross-lingual knowledge transfer.
- Semantic search and relevance ranking – Vector databases excel at semantic search and relevance ranking tasks. By representing documents or passages as dense vectors, the retrieval component can use vector similarity measures to identify the most semantically relevant content.
- Personalized and context-aware retrieval – Vector databases can support personalized and context-aware retrieval in RAG systems. By incorporating user profiles, preferences, or contextual information into the vector representations, the retrieval component can prioritize and surface the most relevant content for a specific user or context.
Although vector databases offer advantages in these scenarios, their implementation and effectiveness may depend on factors such as the specific vector embedding techniques used, the quality and representation of the data, and the computational resources available for indexing and retrieval operations. With Amazon Bedrock Knowledge Bases, you can give FMs and agents contextual information from your company’s private data sources for RAG to deliver more relevant, accurate, and customized responses.
Amazon Bedrock Knowledge Bases with RAG
Amazon Bedrock Knowledge Bases is a fully managed capability that helps with the implementation of the entire RAG workflow, from ingestion to retrieval and prompt augmentation, without having to build custom integrations to data sources and manage data flows. Knowledge bases are essential for various use cases, such as customer support, product documentation, internal knowledge sharing, and decision-making systems. A RAG workflow with knowledge bases has two main steps: data preprocessing and runtime execution.
The following diagram illustrates the data preprocessing workflow.
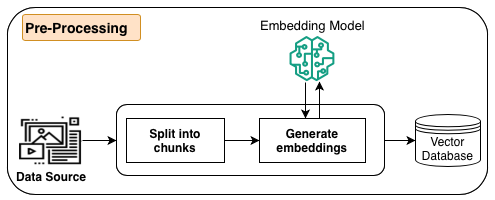
As part of preprocessing, information (structured data, unstructured data, or documents) from data sources is first split into manageable chunks. The chunks are converted to embeddings using embeddings models available in Amazon Bedrock. Lastly, the embeddings are written into a vector database index while maintaining a mapping to the original document. These embeddings are used to determine semantic similarity between queries and text from the data sources. All these steps are managed by Amazon Bedrock.
The following diagram illustrates the workflow for the runtime execution.
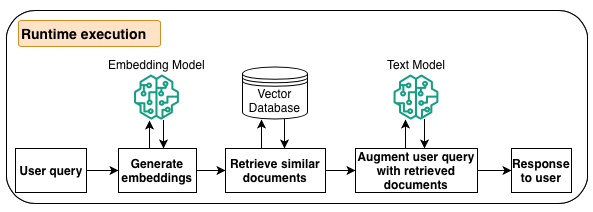
During the inference phase of the LLM, when the agent determines that it needs additional information, it reaches out to knowledge bases. The process converts the user query into vector embeddings using an Amazon Bedrock embeddings model, queries the vector database index to find semantically similar chunks to the user’s query, converts the retrieved chunks to text and augments the user query, and then responds back to the agent.
Embeddings models are needed in the preprocessing phase to store data in vector databases and during the runtime execution phase to generate embeddings for the user query to search the vector database index. Embeddings models map high-dimensional and sparse data like text into dense vector representations to be efficiently stored and processed by vector databases, and encode the semantic meaning and relationships of data into the vector space to enable meaningful similarity searches. These models support mapping different data types like text, images, audio, and video into the same vector space to enable multi-modal queries and analysis. Amazon Bedrock Knowledge Bases provides industry-leading embeddings models to enable use cases such as semantic search, RAG, classification, and clustering, to name a few, and provides multilingual support as well.
Vector database options with Amazon Bedrock Knowledge Bases
At the time of writing this post, Amazon Bedrock Knowledge Bases provides five integration options: the Vector Engine for Amazon OpenSearch Serverless, Amazon Aurora, MongoDB Atlas, Pinecone, and Redis Enterprise Cloud, with more vector database options to come. In this post, we discuss use cases, features, and steps to set up and retrieve information using these vector databases. Amazon Bedrock makes it straightforward to adopt any of these choices by providing a common set of APIs, industry-leading embedding models, security, governance, and observability.
Role of metadata while indexing data in vector databases
Metadata plays a crucial role when loading documents into a vector data store in Amazon Bedrock. It provides additional context and information about the documents, which can be used for various purposes, such as filtering, sorting, and enhancing search capabilities.
The following are some key uses of metadata when loading documents into a vector data store:
- Document identification – Metadata can include unique identifiers for each document, such as document IDs, URLs, or file names. These identifiers can be used to uniquely reference and retrieve specific documents from the vector data store.
- Content categorization – Metadata can provide information about the content or category of a document, such as the subject matter, domain, or topic. This information can be used to organize and filter documents based on specific categories or domains.
- Document attributes – Metadata can store additional attributes related to the document, such as the author, publication date, language, or other relevant information. These attributes can be used for filtering, sorting, or faceted search within the vector data store.
- Access control – Metadata can include information about access permissions or security levels associated with a document. This information can be used to control access to sensitive or restricted documents within the vector data store.
- Relevance scoring – Metadata can be used to enhance the relevance scoring of search results. For example, if a user searches for documents within a specific date range or authored by a particular individual, the metadata can be used to prioritize and rank the most relevant documents.
- Data enrichment – Metadata can be used to enrich the vector representations of documents by incorporating additional contextual information. This can potentially improve the accuracy and quality of search results.
- Data lineage and auditing – Metadata can provide information about the provenance and lineage of documents, such as the source system, data ingestion pipeline, or other transformations applied to the data. This information can be valuable for data governance, auditing, and compliance purposes.
Prerequisites
Complete the steps in this section to set up the prerequisite resources and configurations.
Configure Amazon SageMaker Studio
The first step is to set up an Amazon SageMaker Studio notebook to run the code for this post. You can set up the notebook in any AWS Region where Amazon Bedrock Knowledge Bases is available.
- Complete the prerequisites to set up Amazon SageMaker.
- Complete the quick setup or custom setup to enable your SageMaker Studio domain and user profile.
You also need an AWS Identity and Access Management (IAM) role assigned to the SageMaker Studio domain. You can identify the role on the SageMaker console. On the Domains page, open your domain. The IAM role ARN is listed on the Domain settings tab.
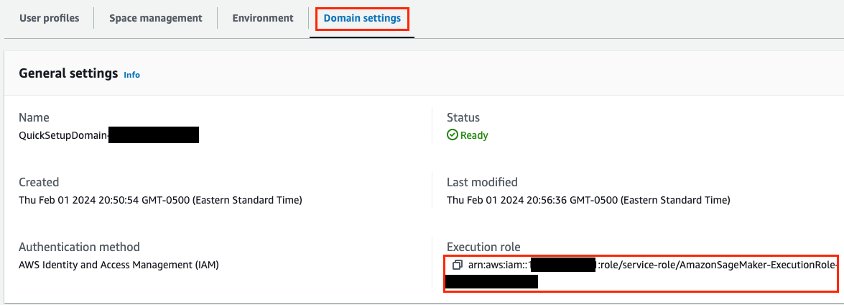
The role needs permissions for IAM, Amazon Relational Database Service (Amazon RDS), Amazon Bedrock, AWS Secrets Manager, Amazon Simple Storage Service (Amazon S3), and Amazon OpenSearch Serverless.
- Modify the role permissions to add the following policies:
IAMFullAccess
AmazonRDSFullAccess
AmazonBedrockFullAccess
SecretsManagerReadWrite
AmazonRDSDataFullAccess
AmazonS3FullAccess
- The following inline policy:
{
"Version": "2012-10-17",
"Statement": [
{
"Sid": "OpenSearchServeless",
"Effect": "Allow",
"Action": "aoss:*",
"Resource": "*"
}
]
}
- On the SageMaker console, choose Studio in the navigation pane.
- Choose your user profile and choose Open Studio.

This will open a new browser tab for SageMaker Studio Classic.
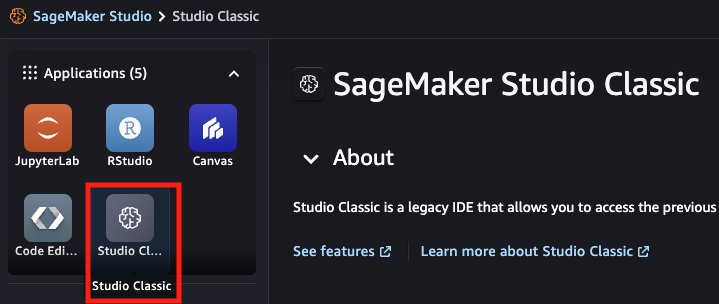
- Run the SageMaker Studio application.

- When the application is running, choose Open.

JupyterLab will open in a new tab.
- Download the notebook file to use in this post.
- Choose the file icon in the navigation pane, then choose the upload icon, and upload the notebook file.
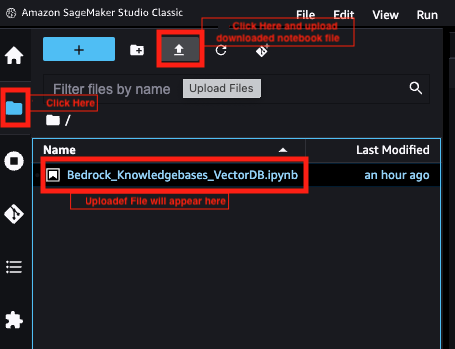
- Leave the image, kernel, and instance type as default and choose Select.
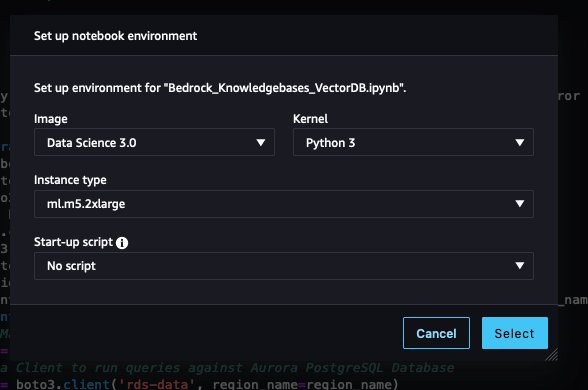
Request Amazon Bedrock model access
Complete the following steps to request access to the embeddings model in Amazon Bedrock:
- On the Amazon Bedrock console, choose Model access in the navigation pane.
- Choose Enable specific models.
- Select the Titan Text Embeddings V2 model.
- Choose Next and complete the access request.
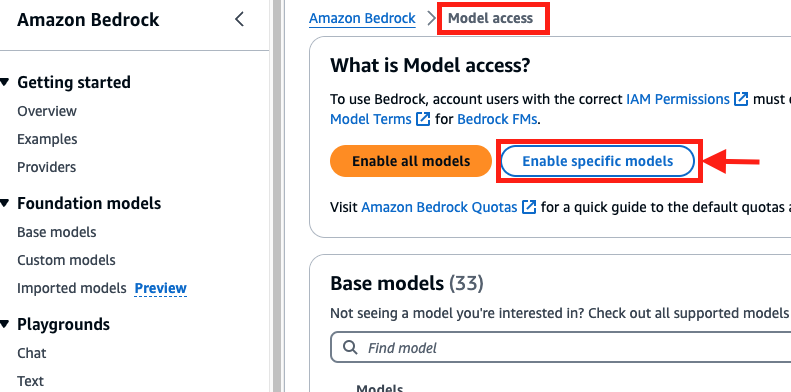
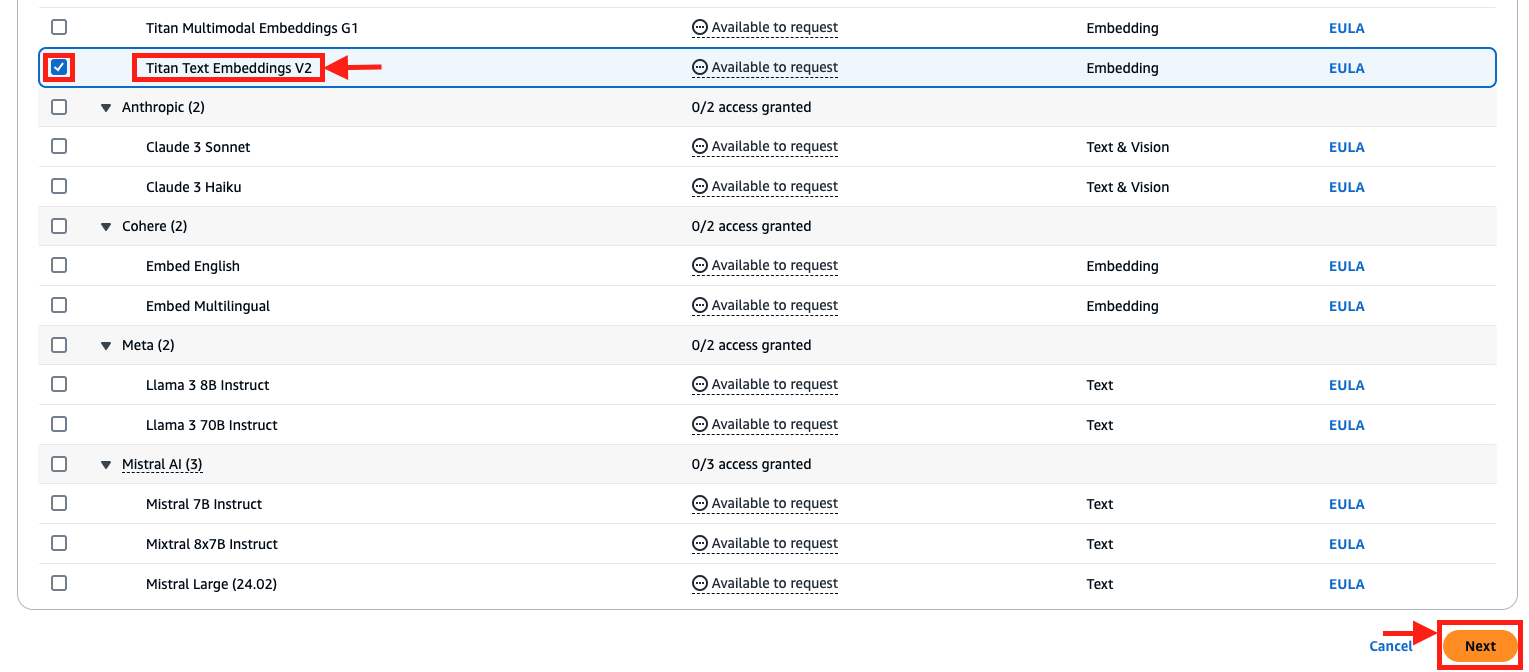
Import dependencies
Open the notebook file Bedrock_Knowledgebases_VectorDB.ipynb
and run Step 1 to import dependencies for this post and create Boto3 clients:
!pip install opensearch-py
!pip install retrying
from urllib.request import urlretrieve
import json
import os
import boto3
import random
import time
from opensearchpy import OpenSearch, RequestsHttpConnection, AWSV4SignerAuth, RequestError
credentials = boto3.Session().get_credentials()
service = 'aoss'
suffix = random.randrange(200, 900)
boto3_session = boto3.session.Session()
region_name = boto3_session.region_name
iam_client = boto3_session.client('iam')
account_number = boto3.client('sts').get_caller_identity().get('Account')
identity = boto3.client('sts').get_caller_identity()['Arn']
s3_client = boto3.client("s3", region_name=region_name)
aoss_client = boto3_session.client('opensearchserverless')
bedrock_agent_client = boto3_session.client('bedrock-agent', region_name=region_name)
bedrock_agent_runtime_client = boto3.client('bedrock-agent-runtime', region_name=region_name)
rds = boto3.client('rds', region_name=region_name)
# Create Secret Manager Client to retrieve secret values
secrets_manager = boto3.client('secretsmanager', region_name=region_name)
# Create RDS Data Client to run queries against Aurora PostgreSQL Database
rds_data_client = boto3.client('rds-data', region_name=region_name)
awsauth = auth = AWSV4SignerAuth(credentials, region_name, service)
Create an S3 bucket
You can use the following code to create an S3 bucket to store the source data for your vector database, or use an existing bucket. If you create a new bucket, make sure to follow your organization’s best practices and guidelines.
# Set the bucket name
bucket_name = "<PROVIDE AMAZON S3 BUCKET NAME>"
if region_name in ('af-south-1','ap-east-1','ap-northeast-1','ap-northeast-2','ap-northeast-3','ap-south-1','ap-south-2','ap-southeast-1','ap-southeast-2','ap-southeast-3','ca-central-1','cn-north-1','cn-northwest-1','EU','eu-central-1','eu-north-1','eu-south-1','eu-south-2','eu-west-1','eu-west-2','eu-west-3','me-south-1','sa-east-1','us-east-2','us-gov-east-1','us-gov-west-1','us-west-1','us-west-2'):
# Create the bucket
response = s3_client.create_bucket(
Bucket=bucket_name,
CreateBucketConfiguration={
'LocationConstraint': region_name
}
)
# Print the response and validate that value for HTTPStatusCode is 200
print(response)
else:
# Create the bucket
response = s3_client.create_bucket(
Bucket=bucket_name
)
# Print the response and validate that value for HTTPStatusCode is 200
print(response)
Set up sample data
Use the following code to set up the sample data for this post, which will be the input for the vector database:
# Leverage Amazon Shareholder news letter as datasets for loading into vector databases for this Blogpost
urls = [
'https://s2.q4cdn.com/299287126/files/doc_financials/2023/ar/2022-Shareholder-Letter.pdf',
'https://s2.q4cdn.com/299287126/files/doc_financials/2022/ar/2021-Shareholder-Letter.pdf',
'https://s2.q4cdn.com/299287126/files/doc_financials/2021/ar/Amazon-2020-Shareholder-Letter-and-1997-Shareholder-Letter.pdf',
'https://s2.q4cdn.com/299287126/files/doc_financials/2020/ar/2019-Shareholder-Letter.pdf'
]
# Define standard file names which be leveraged while loading data to Amazon S3
filenames = [
'AMZN-2022-Shareholder-Letter.pdf',
'AMZN-2021-Shareholder-Letter.pdf',
'AMZN-2020-Shareholder-Letter.pdf',
'AMZN-2019-Shareholder-Letter.pdf'
]
# Create local temporary directory to download files, before uploading to Amazon S3
!mkdir -p ./data
# Assing local directory path to a python variable
local_data_path = "./data/"
# Assign S3 bucket name to a python variable. This was created in Step-2 above.
# This bucket will be used as source for vector databases and uploading source files.
data_s3_bucket = bucket_name
# Define S3 Prefix with in the bucket to upload files
data_s3_prefix = 'shareholder_newsletter'
# Download file to local_data_path
for idx, url in enumerate(urls):
file_path = local_data_path + filenames[idx]
urlretrieve(url, file_path)
# define metadata corresponding to Shareholder letters
metadata_2022 = {
"metadataAttributes": {
"company": "Amazon",
"document_type": "Shareholder Letter",
"year": 2022
}
}
metadata_2021 = {
"metadataAttributes": {
"company": "Amazon",
"document_type": "Shareholder Letter",
"year": 2021
}
}
metadata_2020 = {
"metadataAttributes": {
"company": "Amazon",
"document_type": "Shareholder Letter",
"year": 2020
}
}
metadata_2019 = {
"metadataAttributes": {
"company": "Amazon",
"document_type": "Shareholder Letter",
"year": 2019
}
}
# Create metadata files in local_data_path which will be uploaded to Amazon S3
# Create metadata file for 2022
metadata_2022_json = json.dumps(metadata_2022)
with open(f"{local_data_path}AMZN-2022-Shareholder-Letter.pdf.metadata.json", "w") as f:
f.write(str(metadata_2022_json))
f.close()
# Create metadata file for 2021
metadata_2021_json = json.dumps(metadata_2021)
with open(f"{local_data_path}AMZN-2021-Shareholder-Letter.pdf.metadata.json", "w") as f:
f.write(str(metadata_2021_json))
f.close()
# Create metadata file for 2020
metadata_2020_json = json.dumps(metadata_2020)
with open(f"{local_data_path}AMZN-2020-Shareholder-Letter.pdf.metadata.json", "w") as f:
f.write(str(metadata_2020_json))
f.close()
# Create metadata file for 2019
metadata_2019_json = json.dumps(metadata_2019)
with open(f"{local_data_path}AMZN-2019-Shareholder-Letter.pdf.metadata.json", "w") as f:
f.write(str(metadata_2019_json))
f.close()
# Upload files to Amazon S3
def uploadDirectory(path,bucket_name):
for root,dirs,files in os.walk(path):
for file in files:
key = data_s3_prefix + '/' + file
s3_client.upload_file(os.path.join(root,file),bucket_name,key)
uploadDirectory(local_data_path, data_s3_bucket)
# Delete files from local directory
!rm -r ./data/
Configure the IAM role for Amazon Bedrock
Use the following code to define the function to create the IAM role for Amazon Bedrock, and the functions to attach policies related to Amazon OpenSearch Service and Aurora:
encryption_policy_name = f"bedrock-sample-rag-sp-{suffix}"
network_policy_name = f"bedrock-sample-rag-np-{suffix}"
access_policy_name = f'bedrock-sample-rag-ap-{suffix}'
bedrock_execution_role_name = f'AmazonBedrockExecutionRoleForKnowledgeBase_{suffix}'
fm_policy_name = f'AmazonBedrockFoundationModelPolicyForKnowledgeBase_{suffix}'
s3_policy_name = f'AmazonBedrockS3PolicyForKnowledgeBase_{suffix}'
oss_policy_name = f'AmazonBedrockOSSPolicyForKnowledgeBase_{suffix}'
rds_policy_name = f'AmazonBedrockRDSPolicyForKnowledgeBase_{suffix}'
aurora_policy_name = f'AmazonBedrockAuroraPolicyForKnowledgeBase_{suffix}'
oss_vector_store_name = f'os-shareholder-letter-{suffix}'
oss_index_name = "os_shareholder_letter"
aurora_vector_db_cluster = f'aurora-shareholder-letter-{suffix}'
aurora_vector_db_instance = f'aurora-shareholder-letter-instance-{suffix}'
aurora_database_name = 'vectordb'
aurora_schema_name = 'bedrock_kb'
aurora_table_name = 'aurora_shareholder_letter'
def create_bedrock_execution_role(bucket_name):
foundation_model_policy_document = {
"Version": "2012-10-17",
"Statement": [
{
"Effect": "Allow",
"Action": [
"bedrock:InvokeModel",
],
"Resource": [
f"arn:aws:bedrock:{region_name}::foundation-model/amazon.titan-embed-text-v2:0"
]
}
]
}
s3_policy_document = {
"Version": "2012-10-17",
"Statement": [
{
"Effect": "Allow",
"Action": [
"s3:GetObject",
"s3:ListBucket"
],
"Resource": [f'arn:aws:s3:::{data_s3_bucket}', f'arn:aws:s3:::{data_s3_bucket}/*'],
"Condition": {
"StringEquals": {
"aws:ResourceAccount": f"{account_number}"
}
}
}
]
}
assume_role_policy_document = {
"Version": "2012-10-17",
"Statement": [
{
"Effect": "Allow",
"Principal": {
"Service": "bedrock.amazonaws.com"
},
"Action": "sts:AssumeRole"
}
]
}
# create policies based on the policy documents
fm_policy = iam_client.create_policy(
PolicyName=fm_policy_name,
PolicyDocument=json.dumps(foundation_model_policy_document),
Description='Policy for accessing foundation model',
)
s3_policy = iam_client.create_policy(
PolicyName=s3_policy_name,
PolicyDocument=json.dumps(s3_policy_document),
Description='Policy for reading documents from s3')
# create bedrock execution role
bedrock_kb_execution_role = iam_client.create_role(
RoleName=bedrock_execution_role_name,
AssumeRolePolicyDocument=json.dumps(assume_role_policy_document),
Description='Amazon Bedrock Knowledge Base Execution Role for accessing OSS and S3',
MaxSessionDuration=3600
)
# fetch arn of the policies and role created above
bedrock_kb_execution_role_arn = bedrock_kb_execution_role['Role']['Arn']
s3_policy_arn = s3_policy["Policy"]["Arn"]
fm_policy_arn = fm_policy["Policy"]["Arn"]
# attach policies to Amazon Bedrock execution role
iam_client.attach_role_policy(
RoleName=bedrock_kb_execution_role["Role"]["RoleName"],
PolicyArn=fm_policy_arn
)
iam_client.attach_role_policy(
RoleName=bedrock_kb_execution_role["Role"]["RoleName"],
PolicyArn=s3_policy_arn
)
return bedrock_kb_execution_role
def create_oss_policy_attach_bedrock_execution_role(collection_id, bedrock_kb_execution_role):
# define oss policy document
oss_policy_document = {
"Version": "2012-10-17",
"Statement": [
{
"Effect": "Allow",
"Action": [
"aoss:APIAccessAll"
],
"Resource": [
f"arn:aws:aoss:{region_name}:{account_number}:collection/{collection_id}"
]
}
]
}
oss_policy = iam_client.create_policy(
PolicyName=oss_policy_name,
PolicyDocument=json.dumps(oss_policy_document),
Description='Policy for accessing opensearch serverless',
)
oss_policy_arn = oss_policy["Policy"]["Arn"]
print("Opensearch serverless arn: ", oss_policy_arn)
iam_client.attach_role_policy(
RoleName=bedrock_kb_execution_role["Role"]["RoleName"],
PolicyArn=oss_policy_arn
)
return None
def create_policies_in_oss(vector_store_name, aoss_client, bedrock_kb_execution_role_arn):
encryption_policy = aoss_client.create_security_policy(
name=encryption_policy_name,
policy=json.dumps(
{
'Rules': [{'Resource': ['collection/' + vector_store_name],
'ResourceType': 'collection'}],
'AWSOwnedKey': True
}),
type='encryption'
)
network_policy = aoss_client.create_security_policy(
name=network_policy_name,
policy=json.dumps(
[
{'Rules': [{'Resource': ['collection/' + vector_store_name],
'ResourceType': 'collection'}],
'AllowFromPublic': True}
]),
type='network'
)
access_policy = aoss_client.create_access_policy(
name=access_policy_name,
policy=json.dumps(
[
{
'Rules': [
{
'Resource': ['collection/' + vector_store_name],
'Permission': [
'aoss:CreateCollectionItems',
'aoss:DeleteCollectionItems',
'aoss:UpdateCollectionItems',
'aoss:DescribeCollectionItems'],
'ResourceType': 'collection'
},
{
'Resource': ['index/' + vector_store_name + '/*'],
'Permission': [
'aoss:CreateIndex',
'aoss:DeleteIndex',
'aoss:UpdateIndex',
'aoss:DescribeIndex',
'aoss:ReadDocument',
'aoss:WriteDocument'],
'ResourceType': 'index'
}],
'Principal': [identity, bedrock_kb_execution_role_arn],
'Description': 'Easy data policy'}
]),
type='data'
)
return encryption_policy, network_policy, access_policy
def create_rds_policy_attach_bedrock_execution_role(db_cluster_arn, aurora_db_secret_arn, bedrock_kb_execution_role):
# define rds policy document
rds_policy_document = {
"Version": "2012-10-17",
"Statement": [
{
"Effect": "Allow",
"Action": [
"rds-data:ExecuteStatement",
"rds:DescribeDBClusters",
"rds-data:BatchExecuteStatement"
],
"Resource": [
db_cluster_arn
]
},
{
"Effect": "Allow",
"Action": [
"secretsmanager:GetSecretValue",
"secretsmanager:DescribeSecret"
],
"Resource": [
aurora_db_secret_arn
]
}
]
}
rds_policy = iam_client.create_policy(
PolicyName=rds_policy_name,
PolicyDocument=json.dumps(rds_policy_document),
Description='Policy for accessing RDS Aurora Database',
)
rds_policy_arn = rds_policy["Policy"]["Arn"]
print("RDS Aurora Policy arn: ", rds_policy_arn)
iam_client.attach_role_policy(
RoleName=bedrock_kb_execution_role["Role"]["RoleName"],
PolicyArn=rds_policy_arn
)
return None
Use the following code to create the IAM role for Amazon Bedrock, which you’ll use while creating the knowledge base:
bedrock_kb_execution_role = create_bedrock_execution_role(bucket_name=data_s3_bucket)
bedrock_kb_execution_role_arn = bedrock_kb_execution_role['Role']['Arn']
Integrate with OpenSearch Serverless
The Vector Engine for Amazon OpenSearch Serverless is an on-demand serverless configuration for OpenSearch Service. Because it’s serverless, it removes the operational complexities of provisioning, configuring, and tuning your OpenSearch clusters. With OpenSearch Serverless, you can search and analyze a large volume of data without having to worry about the underlying infrastructure and data management.
The following diagram illustrates the OpenSearch Serverless architecture. OpenSearch Serverless compute capacity for data ingestion, searching, and querying is measured in OpenSearch Compute Units (OCUs).
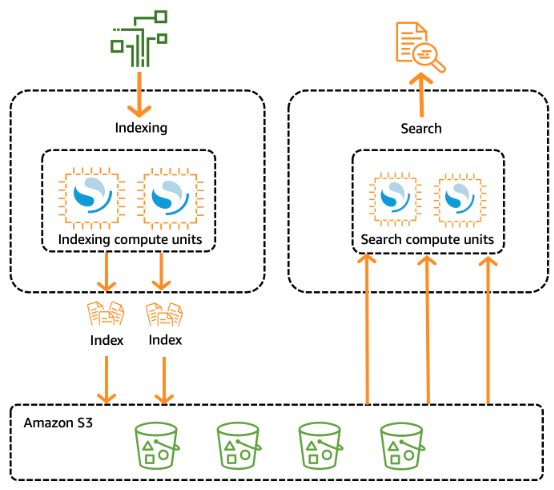
The vector search collection type in OpenSearch Serverless provides a similarity search capability that is scalable and high performing. This makes it a popular option for a vector database when using Amazon Bedrock Knowledge Bases, because it makes it straightforward to build modern machine learning (ML) augmented search experiences and generative AI applications without having to manage the underlying vector database infrastructure. Use cases for OpenSearch Serverless vector search collections include image searches, document searches, music retrieval, product recommendations, video searches, location-based searches, fraud detection, and anomaly detection. The vector engine provides distance metrics such as Euclidean distance, cosine similarity, and dot product similarity. You can store fields with various data types for metadata, such as numbers, Booleans, dates, keywords, and geopoints. You can also store fields with text for descriptive information to add more context to stored vectors. Collocating the data types reduces complexity, increases maintainability, and avoids data duplication, version compatibility challenges, and licensing issues.
The following code snippets set up an OpenSearch Serverless vector database and integrate it with a knowledge base in Amazon Bedrock:
- Create an OpenSearch Serverless vector collection.
# create security, network and data access policies within OSS
encryption_policy, network_policy, access_policy = create_policies_in_oss(vector_store_name=oss_vector_store_name,
aoss_client=aoss_client,
bedrock_kb_execution_role_arn=bedrock_kb_execution_role_arn)
# Create OpenSearch Serverless Vector Collection
collection = aoss_client.create_collection(name=oss_vector_store_name,type='VECTORSEARCH')
# Get the OpenSearch serverless collection URL
collection_id = collection['createCollectionDetail']['id']
host = collection_id + '.' + region_name + '.aoss.amazonaws.com'
print(host)
# wait for collection creation
# This can take couple of minutes to finish
response = aoss_client.batch_get_collection(names=[oss_vector_store_name])
# Periodically check collection status
while (response['collectionDetails'][0]['status']) == 'CREATING':
print('Creating collection...')
time.sleep(30)
response = aoss_client.batch_get_collection(names=[oss_vector_store_name])
print('nCollection successfully created:')
# create opensearch serverless access policy and attach it to Bedrock execution role
try:
create_oss_policy_attach_bedrock_execution_role(collection_id=collection_id,
bedrock_kb_execution_role=bedrock_kb_execution_role)
# It can take up to a minute for data access rules to be enforced
time.sleep(60)
except Exception as e:
print("Policy already exists")
pp.pprint(e)
- Create an index in the collection; this index will be managed by Amazon Bedrock Knowledge Bases:
body_json = {
"settings": {
"index.knn": "true",
"number_of_shards": 1,
"knn.algo_param.ef_search": 512,
"number_of_replicas": 0,
},
"mappings": {
"properties": {
"vector": {
"type": "knn_vector",
"dimension": 1024,
"method": {
"name": "hnsw",
"engine": "faiss",
"space_type": "l2"
},
},
"text": {
"type": "text"
},
"text-metadata": {
"type": "text" }
}
}
}
# Build the OpenSearch client
oss_client = OpenSearch(
hosts=[{'host': host, 'port': 443}],
http_auth=awsauth,
use_ssl=True,
verify_certs=True,
connection_class=RequestsHttpConnection,
timeout=300
)
# Create index
try:
response = oss_client.indices.create(index=oss_index_name, body=json.dumps(body_json))
print('Creating index:')
# index creation can take up to a minute
time.sleep(60)
print('Index Creation Completed:')
except RequestError as e:
# you can delete the index if its already exists
# oss_client.indices.delete(index=oss_index_name)
print(f'Error while trying to create the index, with error {e.error}nyou may unmark the delete above to delete, and recreate the index')
- Create a knowledge base in Amazon Bedrock pointing to the OpenSearch Serverless vector collection and index:
opensearchServerlessConfiguration = {
"collectionArn": collection["createCollectionDetail"]['arn'],
"vectorIndexName": oss_index_name,
"fieldMapping": {
"vectorField": "vector",
"textField": "text",
"metadataField": "text-metadata"
}
}
# The embedding model used by Bedrock to embed ingested documents, and realtime prompts
embeddingModelArn = f"arn:aws:bedrock:{region_name}::foundation-model/amazon.titan-embed-text-v2:0"
name = f"kb-os-shareholder-letter-{suffix}"
description = "Amazon shareholder letter knowledge base."
roleArn = bedrock_kb_execution_role_arn
# Create a KnowledgeBase
from retrying import retry
@retry(wait_random_min=1000, wait_random_max=2000,stop_max_attempt_number=7)
def create_knowledge_base_func():
create_kb_response = bedrock_agent_client.create_knowledge_base(
name = name,
description = description,
roleArn = roleArn,
knowledgeBaseConfiguration = {
"type": "VECTOR",
"vectorKnowledgeBaseConfiguration": {
"embeddingModelArn": embeddingModelArn
}
},
storageConfiguration = {
"type": "OPENSEARCH_SERVERLESS",
"opensearchServerlessConfiguration":opensearchServerlessConfiguration
}
)
return create_kb_response["knowledgeBase"]
try:
kb = create_knowledge_base_func()
except Exception as err:
print(f"{err=}, {type(err)=}")
# Get KnowledgeBase
get_kb_response = bedrock_agent_client.get_knowledge_base(knowledgeBaseId = kb['knowledgeBaseId'])
print(f'OpenSearch Knowledge Response: {get_kb_response}')
- Create a data source for the knowledge base:
# Ingest strategy - How to ingest data from the data source
chunkingStrategyConfiguration = {
"chunkingStrategy": "FIXED_SIZE",
"fixedSizeChunkingConfiguration": {
"maxTokens": 512,
"overlapPercentage": 20
}
}
# The data source to ingest documents from, into the OpenSearch serverless knowledge base index
s3Configuration = {
"bucketArn": f"arn:aws:s3:::{data_s3_bucket}",
"inclusionPrefixes": [f"{data_s3_prefix}"] # you can use this if you want to create a KB using data within s3 prefixes.
}
# Create a DataSource in KnowledgeBase
create_ds_response = bedrock_agent_client.create_data_source(
name = f'{name}-{bucket_name}',
description = description,
knowledgeBaseId = kb['knowledgeBaseId'],
dataSourceConfiguration = {
"type": "S3",
"s3Configuration":s3Configuration
},
vectorIngestionConfiguration = {
"chunkingConfiguration": chunkingStrategyConfiguration
}
)
ds = create_ds_response["dataSource"]
ds
- Start an ingestion job for the knowledge base pointing to OpenSearch Serverless to generate vector embeddings for data in Amazon S3:
ingest_jobs=[]
# Start an ingestion job
try:
start_job_response = bedrock_agent_client.start_ingestion_job(knowledgeBaseId = kb['knowledgeBaseId'], dataSourceId = ds["dataSourceId"])
job = start_job_response["ingestionJob"]
print(f"ingestion job started successfullyn")
while(job['status']!='COMPLETE' ):
get_job_response = bedrock_agent_client.get_ingestion_job(
knowledgeBaseId = kb['knowledgeBaseId'],
dataSourceId = ds["dataSourceId"],
ingestionJobId = job["ingestionJobId"]
)
job = get_job_response["ingestionJob"]
time.sleep(30)
print(f"job completed successfullyn")
except Exception as e:
print(f"Couldn't start job.n")
print(e)
Integrate with Aurora pgvector
Aurora provides pgvector integration, which is an open source extension for PostgreSQL that adds the ability to store and search over ML-generated vector embeddings. This enables you to use Aurora for generative AI RAG-based use cases by storing vectors with the rest of the data. The following diagram illustrates the sample architecture.
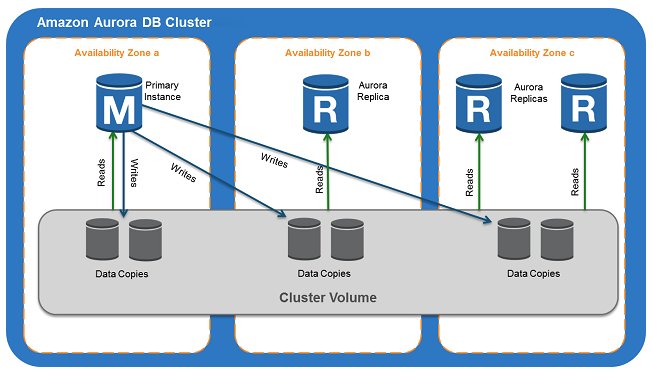
Use cases for Aurora pgvector include applications that have requirements for ACID compliance, point-in-time recovery, joins, and more. The following is a sample code snippet to configure Aurora with your knowledge base in Amazon Bedrock:
- Create an Aurora DB instance (this code creates a managed DB instance, but you can create a serverless instance as well). Identify the security group ID and subnet IDs for your VPC before running the following step and provide the appropriate values in the
vpc_security_group_ids
and SubnetIds variables:
# Define database instance parameters
db_instance_identifier = aurora_vector_db_instance
db_cluster_identifier = aurora_vector_db_cluster
engine = 'aurora-postgresql'
db_name = aurora_database_name
db_instance_class = 'db.r6g.2xlarge'
master_username = 'postgres'
# Get Security Group Id(s), for replicating Blogpost steps it can be one associated with Default VPC
vpc_security_group_ids = ['sg-XXXXXXX']
subnet_group_name = 'vectordbsubnetgroup'
response = rds.create_db_subnet_group(
DBSubnetGroupName=subnet_group_name,
DBSubnetGroupDescription='Subnet Group for Blogpost Aurora PostgreSql Database Cluster',
# Get Subnet IDs, for replicating Blogpost steps it can be one associated with Default VPC
SubnetIds=[
'subnet-XXXXXXX',
'subnet-XXXXXXX',
'subnet-XXXXXXX',
'subnet-XXXXXXX',
'subnet-XXXXXXX',
'subnet-XXXXXXX'
]
)
# Create the Aurora cluster
response = rds.create_db_cluster(
DBClusterIdentifier=db_cluster_identifier,
Engine=engine,
MasterUsername=master_username,
ManageMasterUserPassword=True,
DBSubnetGroupName=subnet_group_name,
VpcSecurityGroupIds=vpc_security_group_ids,
DatabaseName=db_name
)
# Create the Aurora instance
response = rds.create_db_instance(
DBInstanceIdentifier=db_instance_identifier,
DBInstanceClass=db_instance_class,
Engine=engine,
DBClusterIdentifier=db_cluster_identifier
)
- On the Amazon RDS console, confirm the Aurora database status shows as Available.
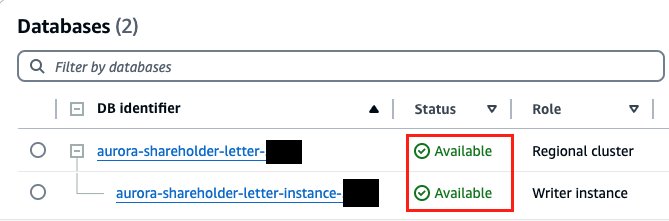
- Create the vector extension, schema, and vector table in the Aurora database:
##Get Amazon Aurora Database Secret Manager ARN created internally while creating DB Cluster and Database Cluster ARN
describe_db_clusters_response = rds.describe_db_clusters(
DBClusterIdentifier=db_cluster_identifier,
IncludeShared=False
)
aurora_db_secret_arn = describe_db_clusters_response['DBClusters'][0]['MasterUserSecret']['SecretArn']
db_cluster_arn = describe_db_clusters_response['DBClusters'][0]['DBClusterArn']
# Enable HTTP Endpoint for Amazon Aurora Database instance
response = rds.enable_http_endpoint(
ResourceArn=db_cluster_arn
)
# Create Vector Extension in Aurora PostgreSQL Database which will be used in table creation
vector_extension_create_response = rds_data_client.execute_statement(
resourceArn=db_cluster_arn,
secretArn=aurora_db_secret_arn,
sql='CREATE EXTENSION IF NOT EXISTS vector',
database=db_name
)
# Create Schema in Aurora PostgreSQL database
schema_create_response = rds_data_client.execute_statement(
resourceArn=db_cluster_arn,
secretArn=aurora_db_secret_arn,
sql='CREATE SCHEMA IF NOT EXISTS bedrock_integration',
database=db_name
)
# Create Table which store vector embedding corresponding to Shareholder letters
table_create_response = rds_data_client.execute_statement(
resourceArn=db_cluster_arn,
secretArn=aurora_db_secret_arn,
sql='CREATE TABLE IF NOT EXISTS bedrock_integration.share_holder_letter_kb(id uuid PRIMARY KEY, embedding vector(1024), chunks text, metadata json, company varchar(100), document_type varchar(100), year int)',
database=db_name
)
# Check the status of queries
vector_extension_create_status = 'Success' if vector_extension_create_response['ResponseMetadata']['HTTPStatusCode'] == 200 else 'Fail'
schema_create_status = 'Success' if schema_create_response['ResponseMetadata']['HTTPStatusCode'] == 200 else 'Fail'
table_create_response = 'Success' if table_create_response['ResponseMetadata']['HTTPStatusCode'] == 200 else 'Fail'
# Print the status of queries
print(f"Create Vector Extension Status: {vector_extension_create_status}")
print(f"Create Schema Status: {schema_create_status}")
print(f"Create Table Status: {table_create_response}")
- Create a knowledge base in Amazon Bedrock pointing to the Aurora database and table:
# Attached RDS related permissions to the Bedrock Knowledgebase role
create_rds_policy_attach_bedrock_execution_role(db_cluster_arn, aurora_db_secret_arn, bedrock_kb_execution_role)
# Define RDS Configuration for Knowledge bases
rdsConfiguration = {
'credentialsSecretArn': aurora_db_secret_arn,
'databaseName': db_name,
'fieldMapping': {
'metadataField': 'metadata',
'primaryKeyField': 'id',
'textField': 'chunks',
'vectorField': 'embedding'
},
'resourceArn': db_cluster_arn,
'tableName': 'bedrock_integration.share_holder_letter_kb'
}
# The embedding model used by Bedrock to embed ingested documents, and realtime prompts
embeddingModelArn = f"arn:aws:bedrock:{region_name}::foundation-model/amazon.titan-embed-text-v2:0"
name = f"kb-aurora-shareholder-letter-{suffix}"
description = "Amazon shareholder letter Aurora PG Vector knowledge base."
roleArn = bedrock_kb_execution_role_arn
# Create a KnowledgeBase
from retrying import retry
@retry(wait_random_min=1000, wait_random_max=2000,stop_max_attempt_number=7)
def create_knowledge_base_func():
create_rds_kb_response = bedrock_agent_client.create_knowledge_base(
name = name,
description = description,
roleArn = roleArn,
knowledgeBaseConfiguration = {
"type": "VECTOR",
"vectorKnowledgeBaseConfiguration": {
"embeddingModelArn": embeddingModelArn
}
},
storageConfiguration = {
"type": "RDS",
"rdsConfiguration":rdsConfiguration
}
)
return create_rds_kb_response["knowledgeBase"]
try:
rds_kb = create_knowledge_base_func()
except Exception as err:
print(f"{err=}, {type(err)=}")
# Get KnowledgeBase
get_rds_kb_response = bedrock_agent_client.get_knowledge_base(knowledgeBaseId = rds_kb['knowledgeBaseId'])
print(f'RDS Aurora Knowledge Response: {get_rds_kb_response}')
- Create a data source for the knowledge base:
# Ingest strategy - How to ingest data from the data source
chunkingStrategyConfiguration = {
"chunkingStrategy": "FIXED_SIZE",
"fixedSizeChunkingConfiguration": {
"maxTokens": 512,
"overlapPercentage": 20
}
}
# The data source to ingest documents from, into the OpenSearch serverless knowledge base index
s3Configuration = {
"bucketArn": f"arn:aws:s3:::{data_s3_bucket}",
"inclusionPrefixes": [f"{data_s3_prefix}"] # you can use this if you want to create a KB using data within s3 prefixes.
}
# Create a DataSource in KnowledgeBase
create_ds_response = bedrock_agent_client.create_data_source(
name = f'{name}-{data_s3_bucket}',
description = description,
knowledgeBaseId = rds_kb['knowledgeBaseId'],
dataSourceConfiguration = {
"type": "S3",
"s3Configuration":s3Configuration
},
vectorIngestionConfiguration = {
"chunkingConfiguration": chunkingStrategyConfiguration
}
)
ds = create_ds_response["dataSource"]
ds
- Start an ingestion job for your knowledge base pointing to the Aurora pgvector table to generate vector embeddings for data in Amazon S3:
ingest_jobs=[]
# Start an ingestion job
try:
start_job_response = bedrock_agent_client.start_ingestion_job(knowledgeBaseId = kb['knowledgeBaseId'], dataSourceId = ds["dataSourceId"])
job = start_job_response["ingestionJob"]
print(f"job started successfullyn")
while(job['status']!='COMPLETE' ):
get_job_response = bedrock_agent_client.get_ingestion_job(
knowledgeBaseId = kb['knowledgeBaseId'],
dataSourceId = ds["dataSourceId"],
ingestionJobId = job["ingestionJobId"]
)
job = get_job_response["ingestionJob"]
time.sleep(30)
print(f"job completed successfullyn")
except Exception as e:
print(f"Couldn't start job.n")
print(e)
Integrate with MongoDB Atlas
MongoDB Atlas Vector Search, when integrated with Amazon Bedrock, can serve as a robust and scalable knowledge base to build generative AI applications and implement RAG workflows. By using the flexible document data model of MongoDB Atlas, organizations can represent and query complex knowledge entities and their relationships within Amazon Bedrock. The combination of MongoDB Atlas and Amazon Bedrock provides a powerful solution for building and maintaining a centralized knowledge repository.
To use MongoDB, you can create a cluster and vector search index. The native vector search capabilities embedded in an operational database simplify building sophisticated RAG implementations. MongoDB allows you to store, index, and query vector embeddings of your data without the need for a separate bolt-on vector database.
There are three pricing options available for MongoDB Atlas through AWS Marketplace: MongoDB Atlas (pay-as-you-go), MongoDB Atlas Enterprise, and MongoDB Atlas for Government. Refer to the MongoDB Atlas Vector Search documentation to set up a MongoDB vector database and add it to your knowledge base.
Integrate with Pinecone
Pinecone is a type of vector database from Pinecone Systems Inc. With Amazon Bedrock Knowledge Bases, you can integrate your enterprise data into Amazon Bedrock using Pinecone as the fully managed vector database to build generative AI applications. Pinecone is highly performant; it can speed through data in milliseconds. You can use its metadata filters and sparse-dense index support for top-notch relevance, achieving quick, accurate, and grounded results across diverse search tasks. Pinecone is enterprise ready; you can launch and scale your AI solution without needing to maintain infrastructure, monitor services, or troubleshoot algorithms. Pinecone adheres to the security and operational requirements of enterprises.
There are two pricing options available for Pinecone in AWS Marketplace: Pinecone Vector Database – Pay As You Go Pricing (serverless) and Pinecone Vector Database – Annual Commit (managed). Refer to the Pinecone documentation to set up a Pinecone vector database and add it to your knowledge base.
Integrate with Redis Enterprise Cloud
Redis Enterprise Cloud enables you to set up, manage, and scale a distributed in-memory data store or cache environment in the cloud to help applications meet low latency requirements. Vector search is one of the solution options available in Redis Enterprise Cloud, which solves for low latency use cases related to RAG, semantic caching, document search, and more. Amazon Bedrock natively integrates with Redis Enterprise Cloud vector search.
There are two pricing options available for Redis Enterprise Cloud through AWS Marketplace: Redis Cloud Pay As You Go Pricing and Redis Cloud – Annual Commits. Refer to the Redis Enterprise Cloud documentation to set up vector search and add it to your knowledge base.
Interact with Amazon Bedrock knowledge bases
Amazon Bedrock provides a common set of APIs to interact with knowledge bases:
- Retrieve API – Queries the knowledge base and retrieves information from it. This is a Bedrock Knowledge Base specific API, it helps with use cases where only vector-based searching of documents is needed without model inferences.
- Retrieve and Generate API – Queries the knowledge base and uses an LLM to generate responses based on the retrieved results.
The following code snippets show how to use the Retrieve API from the OpenSearch Serverless vector database’s index and the Aurora pgvector table:
- Retrieve data from the OpenSearch Serverless vector database’s index:
query = "What is Amazon's doing in the field of generative AI?"
relevant_documents_os = bedrock_agent_runtime_client.retrieve(
retrievalQuery= {
'text': query
},
knowledgeBaseId=kb['knowledgeBaseId'],
retrievalConfiguration= {
'vectorSearchConfiguration': {
'numberOfResults': 3 # will fetch top 3 documents which matches closely with the query.
}
}
)
relevant_documents_os["retrievalResults"]
- Retrieve data from the Aurora pgvector table:
query = "What is Amazon's doing in the field of generative AI?"
relevant_documents_rds = bedrock_agent_runtime_client.retrieve(
retrievalQuery= {
'text': query
},
knowledgeBaseId=rds_kb['knowledgeBaseId'],
retrievalConfiguration= {
'vectorSearchConfiguration': {
'numberOfResults': 3 # will fetch top 3 documents which matches closely with the query.
}
}
)
relevant_documents_rds["retrievalResults"]
Clean up
When you’re done with this solution, clean up the resources you created:
- Amazon Bedrock knowledge bases for OpenSearch Serverless and Aurora
- OpenSearch Serverless collection
- Aurora DB instance
- S3 bucket
- SageMaker Studio domain
- Amazon Bedrock service role
- SageMaker Studio domain role
Conclusion
In this post, we provided a high-level introduction to generative AI use cases and the use of RAG workflows to augment your organization’s internal or external knowledge stores. We discussed the importance of vector databases and RAG architectures to enable similarity search and why dense vector representations are beneficial. We also went over Amazon Bedrock Knowledge Bases, which provides common APIs, industry-leading governance, observability, and security to enable vector databases using different options like AWS native and partner products through AWS Marketplace. We also dived deep into a few of the vector database options with code examples to explain the implementation steps.
Try out the code examples in this post to implement your own RAG solution using Amazon Bedrock Knowledge Bases, and share your feedback and questions in the comments section.
About the Authors
Vishwa Gupta is a Senior Data Architect with AWS Professional Services. He helps customers implement generative AI, machine learning, and analytics solutions. Outside of work, he enjoys spending time with family, traveling, and trying new foods.
Isaac Privitera is a Principal Data Scientist with the AWS Generative AI Innovation Center, where he develops bespoke generative AI-based solutions to address customers’ business problems. His primary focus lies in building responsible AI systems, using techniques such as RAG, multi-agent systems, and model fine-tuning. When not immersed in the world of AI, Isaac can be found on the golf course, enjoying a football game, or hiking trails with his loyal canine companion, Barry.
Abhishek Madan is a Senior GenAI Strategist with the AWS Generative AI Innovation Center. He helps internal teams and customers in scaling generative AI, machine learning, and analytics solutions. Outside of work, he enjoys playing adventure sports and spending time with family.
Ginni Malik is a Senior Data & ML Engineer with AWS Professional Services. She assists customers by architecting enterprise data lake and ML solutions to scale their data analytics in the cloud.
Satish Sarapuri is a Sr. Data Architect, Data Lake at AWS. He helps enterprise-level customers build high-performance, highly available, cost-effective, resilient, and secure generative AI, data mesh, data lake, and analytics platform solutions on AWS through which customers can make data-driven decisions to gain impactful outcomes for their business, and helps them on their digital and data transformation journey. In his spare time, he enjoys spending time with his family and playing tennis.
Read More