Generative AI has revolutionized technology through generating content and solving complex problems. To fully take advantage of this potential, seamless integration with existing business systems and efficient access to data are crucial. Amazon Bedrock Agents provides the integration capabilities to connect generative AI models with the wealth of information and workflows already in place within an organization, enabling the creation of efficient and impactful generative AI applications.
Amazon Bedrock is a fully managed service that enables the development and deployment of generative AI applications using high-performance foundation models (FMs) from leading AI companies through a single API. Amazon Bedrock Agents allows you to streamline workflows and automate repetitive tasks across your company systems and data sources, while maintaining security, privacy, and responsible AI practices. Using these agents, you can enable generative AI applications to execute multiple tasks across your company systems and data sources. Businesses can now unlock the power of generative AI to automate tasks, generate content, and solve complex problems—all while maintaining connectivity to critical enterprise systems and data sources.
The post showcases how generative AI can be used to logic, reason, and orchestrate integrations using a fictitious business process. It demonstrates strategies and techniques for orchestrating Amazon Bedrock agents and action groups to seamlessly integrate generative AI with existing business systems, enabling efficient data access and unlocking the full potential of generative AI.
This solution also integrates with Appian Case Management Studio. Cases are a vital part of case management applications and represent a series of tasks to complete or a multi-step problem to solve. Appian Case Management Studio is an out-of-the box suite of applications that facilitates rapid development of case management apps. The fictitious business process used in this post creates a case in Appian for further review.
Business workflow
The following workflow shows the fictitious business process.
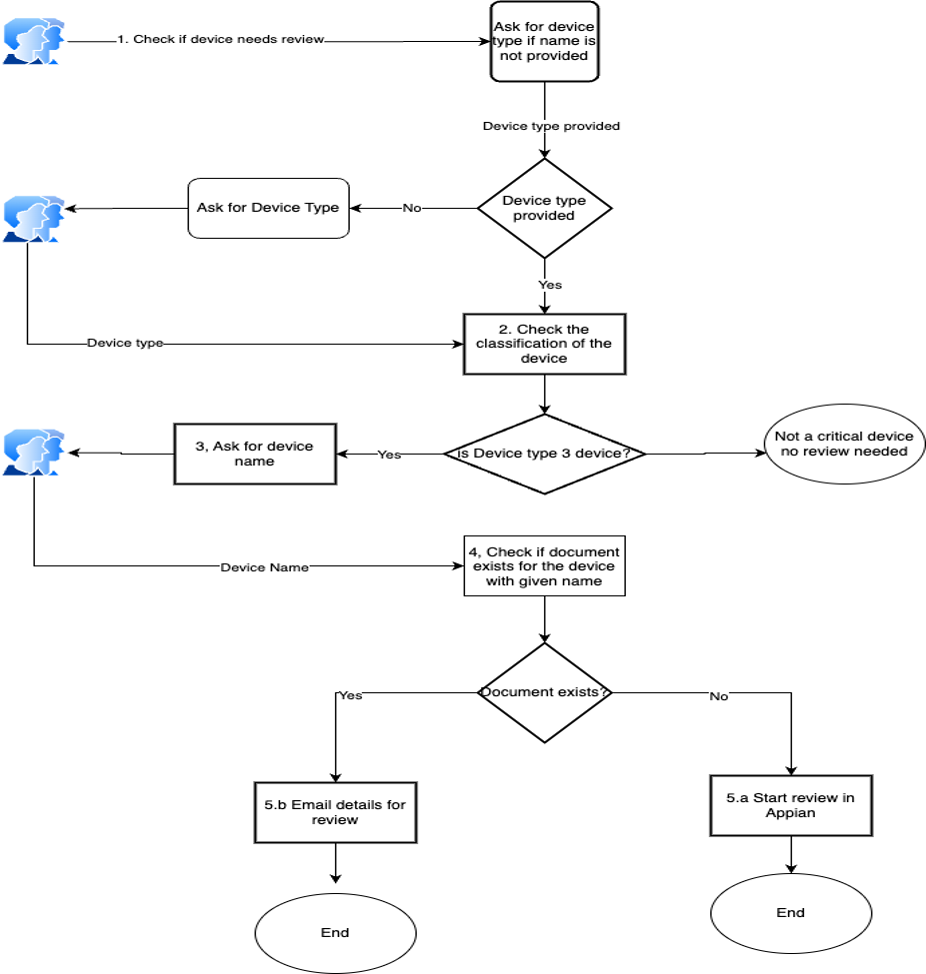
The workflow consists of the following steps:
- The user asks the generative AI assistant to determine if a device needs review.
- If a device type is provided, the assistant checks if it’s a Type 3 device.
- If it’s a Type 3 device, the assistant asks the user for the device name.
- The assistant checks if a document exists with the provided name.
- If the document exists, the assistant creates a case in Appian to start a review.
- If the document doesn’t exist, the assistant sends an email for review.
Solution overview
The following diagram illustrates the architecture of the solution.
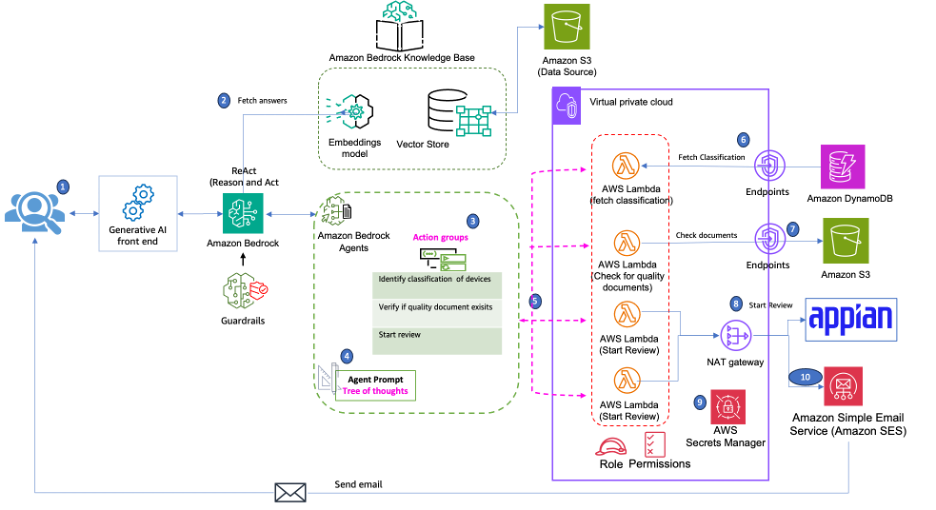
The system workflow includes the following steps:
- The user interacts with the generative AI application, which connects to Amazon Bedrock Agents.
- The application uses Amazon Bedrock Knowledge Bases to answer the user questions. These knowledge bases are created with Amazon Simple Storage Service (Amazon S3) as the data source and Amazon Titan (or another model of your choice) as the embedding model.
- Amazon Bedrock Agents uses action groups to integrate with different systems.
- The action groups call different AWS Lambda functions within private subnet of a virtual private cloud (VPC).
- The agent uses a tree-of-thought (ToT) prompt to execute different actions from the action groups.
- A Lambda function fetches the classification of the device from Amazon DynamoDB. The function invokes DynamoDB using a gateway endpoint.
- A Lambda function checks if quality documents exist in Amazon S3. The function invokes Amazon S3 using interface endpoints.
- A Lambda function calls the Appian REST API using a NAT gateway in a public subnet.
- The Appian key is stored in AWS Secrets Manager.
- A Lambda function uses AWS Identity and Access Management (IAM) permissions to make an SDK call to Amazon Simple Email Service (Amazon SES). Amazon SES sends an email using SMTP to verified emails provided by the user.
Prerequisites
You will need the following prerequisites before you can build the solution:
- A valid AWS account.
- Access to Anthropic’s Claude 3 Sonnet or the model you intend to use (for more information, see Access Amazon Bedrock foundation models). For this post, we use Anthropic’s Claude 3 Sonnet, and all instructions are pertaining to that model. If you want to use another FM, update the prompts accordingly.
- An IAM role in the account that has sufficient permissions to create the necessary resources.
- AWS CloudTrail logging enabled for operational and risk auditing. For more details, see Creating a trail for your AWS account.
- AWS Budgets policy notifications enabled to protect you from unwanted billing. For more details, see Enable Budget policy.
- Two email addresses to send and receive emails. Do not use existing verified identities in Amazon SES for these email addresses. The AWS CloudFormation template will fail otherwise.
This solution is supported only in the us-east-1
AWS Region. You can make the necessary changes to the CloudFormation template to deploy to other Regions.
Create an Appian account
Depending on your needs, follow the corresponding steps to create an Appian account.
Sign up for Appian Community Edition for personal use
The Appian Community Edition provides a personal environment for learning and exploration at no additional cost. To sign up for Apian Community Edition, complete the following steps:
- Visit the Appian Community Edition page.
- Enter your email address and choose Submit to receive confirmation and login details.
- Check your inbox for a verification email from Appian.
- Choose the link in the email to validate your email address and finish setting up your account by providing your first name, last name, email, and password, then accept the terms.
- Choose Register to complete the registration.
- Choose the activation link and log in with your email address and password.
- Complete your profile by entering information about your company, phone number, and learning interests, among other details.
- Choose Access Environment.
- Choose your region (USA, India, or Germany) by choosing the appropriate link.
- Navigate to Appian Designer and start exploring Appian’s features and capabilities.
Purchase Appian Platform for business use
If you’re evaluating Appian for your organization, complete the following steps:
- Visit the Appian Platform listing at AWS Marketplace.
- Choose View purchase options.
- Fill out the contract form by providing your duration, renewal settings, and contract options.
- Choose Create Contract. to submit your request.
An Appian representative will contact you to discuss your needs. They might provide access to a trial environment or schedule a personalized demo.
- Follow the instructions provided by the Appian representative to access your account.
By following these steps, you can create an Appian account suited to your personal learning or business evaluation needs. Whether you’re exploring Appian’s platform individually or assessing it for your organization, Appian provides resources and support to help you get started.
Note the following values, which we will use in the CloudFormation template below.
- AppianHostEndpoint
- AppianAPIKey
Deploy the CloudFormation template
Complete the following steps to deploy the CloudFormation template:
- Download the CloudFormation template.
- Open the AWS CloudFormation console in the
us-east-1
- Choose Stacks in the navigation pane, then choose Create stack.
- Upload the template and choose Next.
- For Stack name, enter a name, such as
QualityReviewStack
.
- In the Parameters section, provide the following information:
- For DynamoDBTableName, enter the name of the DynamoDB table.
- For Fromemailaddress, enter the email address to send emails.
- For Toemailaddress, enter the email address to receive emails.
- For AppianHostEndpoint enter the AppianHostEndpoint captured earlier.
- For AppianAPIKey enter the AppianAPIKey captured earlier.
- Leave other settings as default and choose Next.
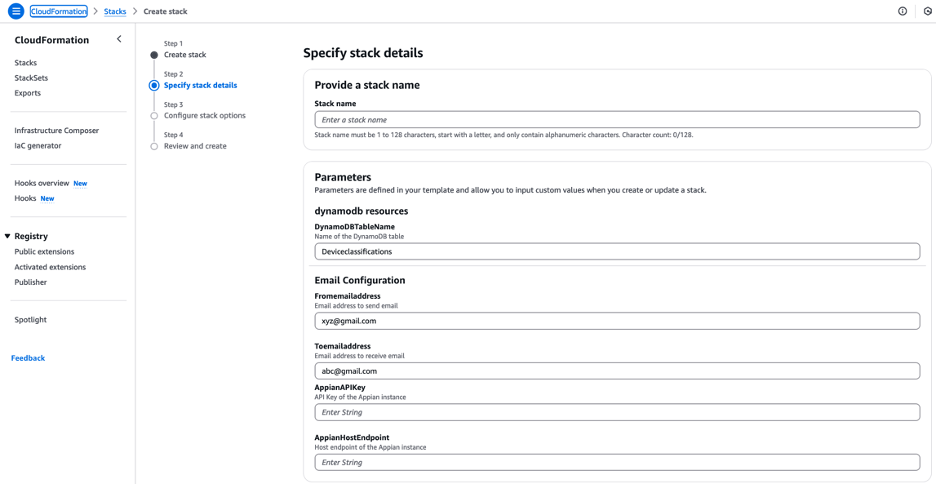
- Under Capabilities on the last page, select I acknowledge that AWS CloudFormation might create IAM resources.
- Choose Submit to create the CloudFormation stack.
After the successful deployment of the whole stack, an email will be sent to the email addresses provided earlier.
- Verify the newly created email identities by choosing link in the email.
- On the Resources tab of the CloudFormation template, make a note of the physical IDs for the following resource logical IDs. You will need them later.
OpenAPISpecsS3Bucket
QualityFormsBucket
This post does not cover auto scaling of AWS Lambda. To integrate Lambda with AWS Application Auto Scaling, see AWS Lambda and Application Auto Scaling.
Upload Open API files to the S3 bucket
Complete the following steps to upload the Open API specifications to Amazon S3:
- Download the following the Open API specifications:
- Device Classification (
deviceclassification.json
)
- Verify Quality Documents (
verifyQualityDocuments.json
)
- Email Reviewers (
emailReviewers.json
)
- Appian Case (
appian-case.json
)
- On the Amazon S3 console, navigate to the OpenAPISpecsS3Bucket captured earlier.
- Upload the downloaded files to the bucket.
Upload the quality forms to the S3 bucket
Complete the following steps to upload the quality form to the Amazon S3:
- Download the dummy quality form.
- On the AWS CloudFormation console, navigate to the Resources tab of the stack and choose the link next to the physical ID of
QualityFormsBucket
.
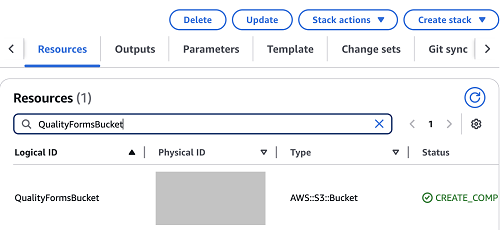
- Upload the file downloaded sample articles to the bucket.
Create an effective prompt
Before we configure the agents, we will define a prompt. Prompts are the key to unlocking the full potential of Amazon Bedrock agents. Prompts are the textual inputs that guide the agent’s behavior and responses. Crafting well-designed prompts is essential for making sure that the agent understands the context, intent, and desired output.
When creating prompts, consider the following best practices:
- Provide clear and concise instructions
- Include relevant background information and context
- Follow the model best practices to format the prompt
Amazon Bedrock Agents supports advanced prompting techniques, Chain of thought (CoT) and Tree-of-thought (ToT) prompting. CoT prompting is a technique that enhances the reasoning capabilities of FMs by breaking down complex questions or tasks into smaller, more manageable steps. ToT prompting is a technique used to improve FM reasoning capabilities by breaking down larger problem statements into a treelike format, where each problem is divided into smaller subproblems. We use Tree-of-thought (ToT) prompting and start by breaking down the business process into logical steps and then incorporate model formatting.
The following is the prompt developed for Anthropic’s Claude 3 Sonnet:
You are an agent that helps determine if device requires a quality review and you always use actions groups to answer. To verify if a review is needed, follow these steps:
1. Ask the user to provide the device type. If not provided, prompt for it.
2. Fetch the device classification from the database based on the provided device type using deviceClassification action group
3. If the classification returned from action group is Class III or 3
4. Ask the user for the specific device name.
5. Check if the device name has quality review forms using the verifyifformsExists action group
6. If a quality review document exists:
7. Prepare an email with the relevant content.
8. Ask for to email address and from email address
9. Send the email to the user.
10. If no quality review document exists, create a case.
Create an Amazon Bedrock Agent
The first step in configuring Amazon Bedrock Agents is to define their capabilities. Amazon Bedrock agents can be trained to perform a wide range of tasks, from natural language processing and generation to task completion and decision-making. When defining an agent’s capabilities, consider the specific use case and the desired outcomes.
To create an agent, complete the following steps:
- On the Amazon Bedrock console, choose Agents in the navigation pane.
- Choose Create Agent.
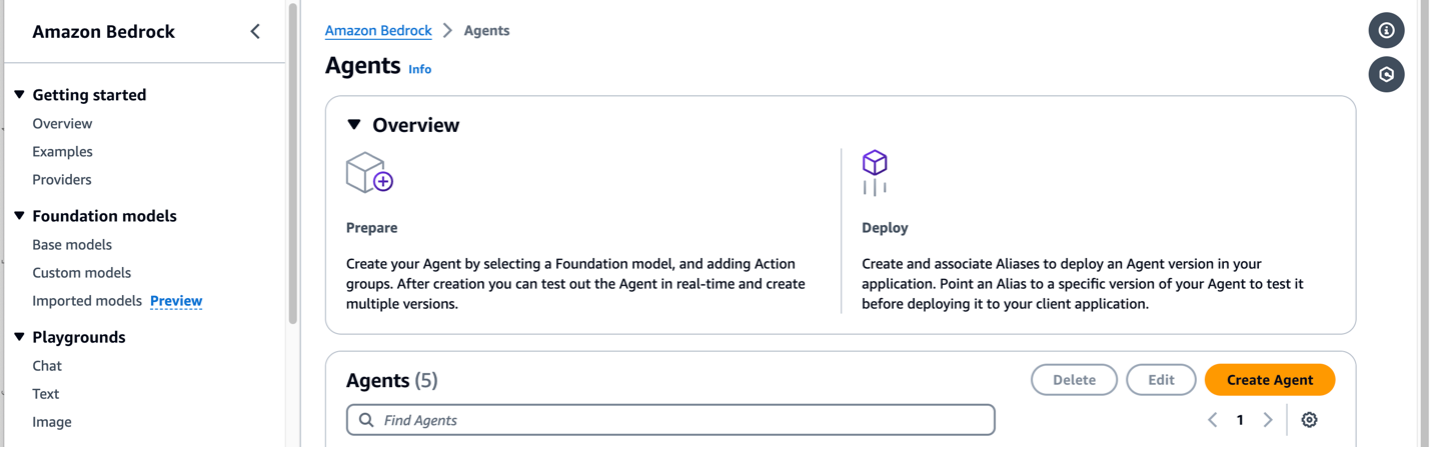
- In the Agent details section, enter a name for the agent and an optional description.
- Choose Create.
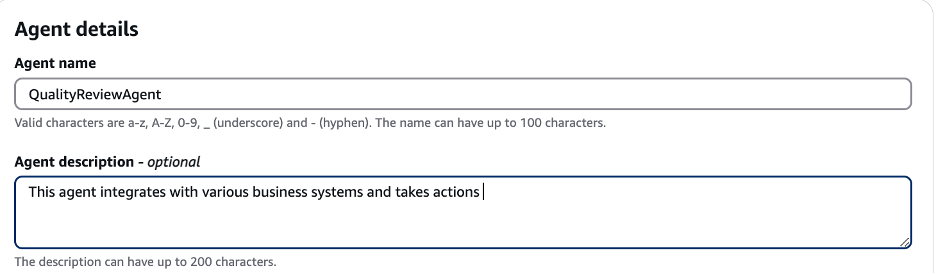
- In the agent builder, choose Create and use a new service role for the agent resource role.

- Choose Anthropic’s Claude 3 Sonnet as the model.
- In the Instructions for the Agent section, provide the prompt crafted earlier.
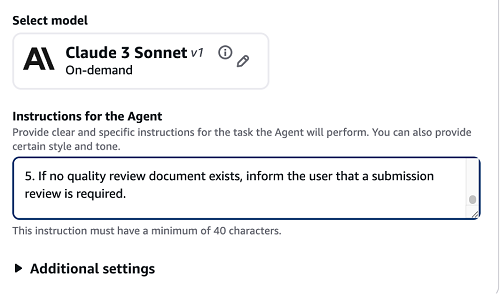
- In the Additional settings section, for User input, select Enabled.
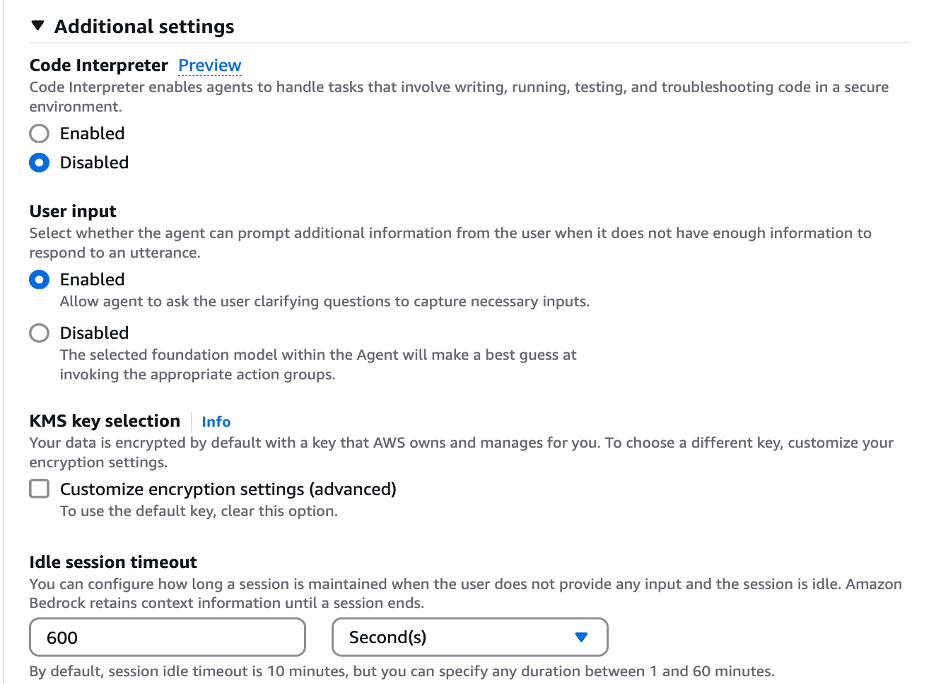
- Choose Save and exit to save the agent.
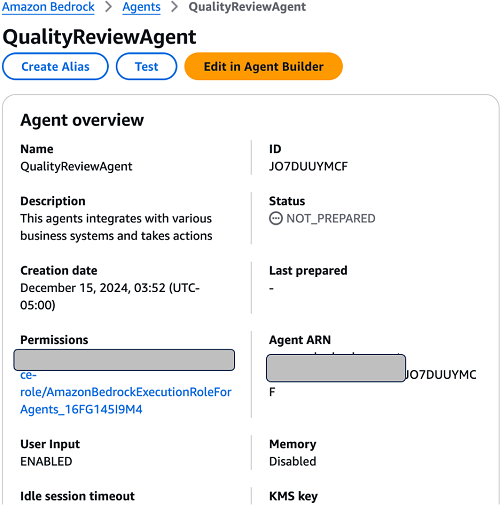
Create action groups
Complete the following steps to create the action groups for the newly created agent:
- On the Amazon Bedrock console, choose Agents in the navigation pane.
- Choose the newly created agent and choose Edit in Agent Builder.
- In the Action groups section, choose Add.
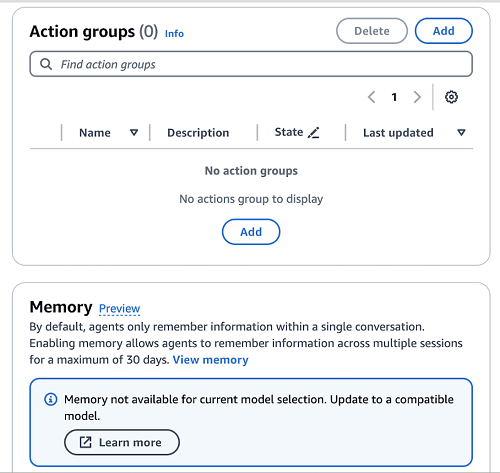
- In the Action group details section, change the automatically generated name to
checkdeviceclassification
and provide an optional description for your action group.
- In the Action group type section, select Define with API schemas to use the OpenAPI schema.
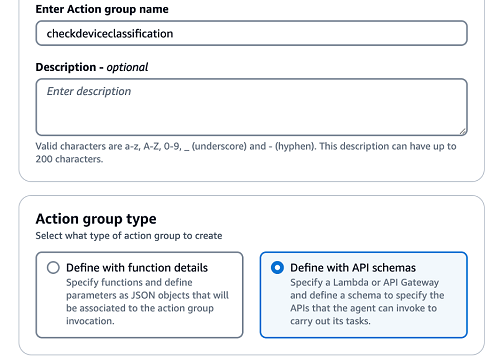
- In the Action group invocation section, select Select an existing Lambda function to use an existing Lambda function.
- On the drop-down menu, choose the Lambda function with the name containing
DeviceClassification
.
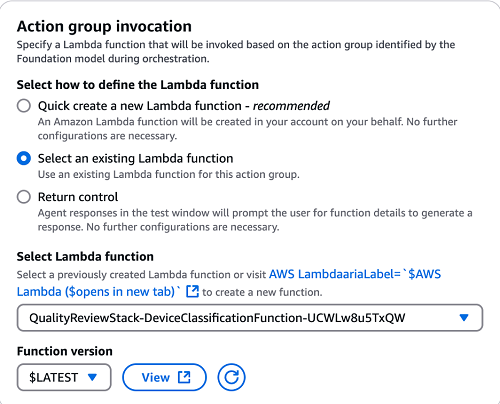
- In the Action group schema section, select Define via in-line schema editor to define the schema.
- Choose JSON on the drop-down menu next to
- Open the device classification file downloaded earlier and copy the content of the schema file.
- Enter the content in the schema editor.
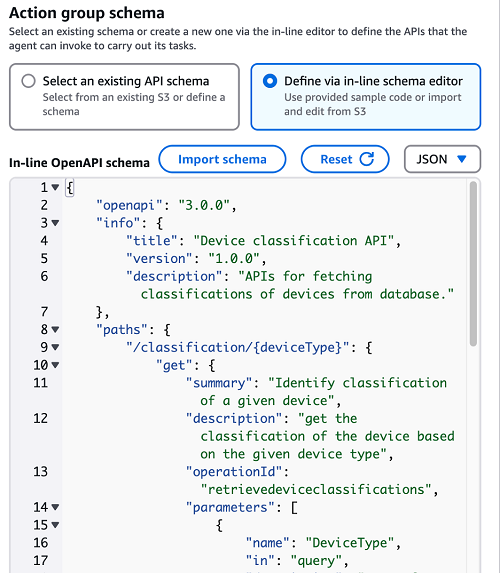
- Choose Create to create an action group.
- Repeat the preceding steps to create additional action groups. Use the following table to map the action groups to the respective Lambda functions and Open API schemas.
Action Group Name |
Lambda Functin Name Containing |
Open API Schema |
checkdeviceclassification |
DeviceClassification |
deviceclassification.json |
verifyqualitydocuments |
VerifyQualityDocuments |
verifyQualityDocuments.json |
emailreviewers |
EmailReviewers |
emailReviewers.json |
appiancase |
Appian |
appian-case.json |
To customize the agent’s behavior to your specific use case, you can modify the prompt templates for the preprocessing, orchestration, knowledge base response generation, and postprocessing steps. For more information, see Enhance agent’s accuracy using advanced prompt templates in Amazon Bedrock.
Create a knowledge base
You can create an Amazon Bedrock knowledge base to retrieve information from your proprietary data and generate responses to answer natural language questions. As part of creating a knowledge base, you configure a data source and a vector store of your choice.
The prompt crafted earlier provides instructions that are not dependent on a knowledge base. To use a knowledge base, modify the prompt accordingly.
Prepare the agent
Complete the following steps to prepare the agent for deployment:
- On the Amazon Bedrock console, navigate to the agent you created.
- In the agent builder, choose Save.
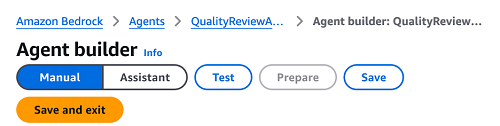
After the agent is saved, the Prepare button will be enabled.
- Choose Prepare to build the agent.
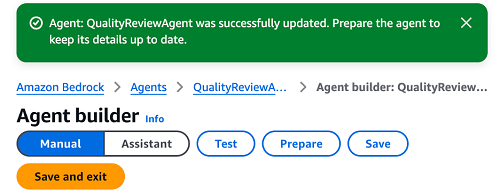
Test the agent
To test the agent, we use the Amazon Bedrock agent console. You can embed the API calls into your applications.
If you use AWS published API calls to access Amazon Bedrock through the network, the client must adhere to the following requirements.
Complete the following steps to test the agent on the Amazon Bedrock console:
- On the Test page for the agent, choose the arrows icon to enlarge the test window.
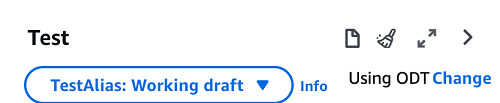
- In the message bar, enter “verify if the device requires review.”
The agent will respond by asking for the type of device.
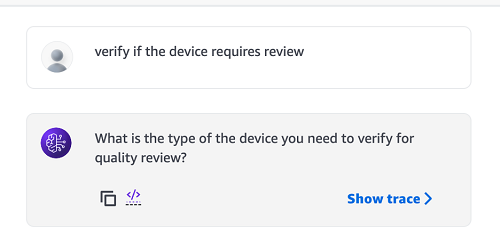
- Enter “HIV diagnostic tests.”
The CloudFormation template only deploys “HIV diagnostic tests” as a Type 3 device.
The agent fetches the classification of the device from the DynamoDB. You can update the CloudFormation template to add more values.
Because the classification of HIV diagnostic tests is Type 3, the agent will ask for the device name to verify if the quality document exists.
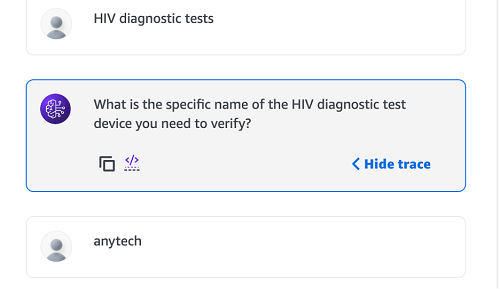
- Enter
anytech
.
The agent will verify if the document with the name anytech
exists in Amazon S3. (Earlier, you uploaded a dummy document for anytech
.)
The agent should now ask for an email address to receive the quality review request.
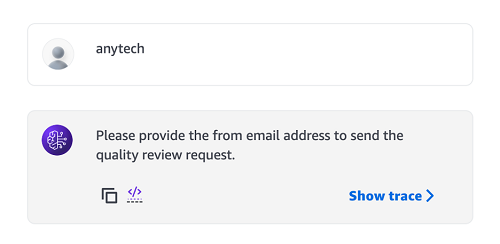
An email will be sent with the review details.
- Repeat the preceding steps but this time, enter
anytechorg
as the document name.
We did not upload a document named anytechorg
, so the agent will create a case by asking for the following information:
- First name
- Last name
- Mobile phone number
- Description
- Title of the case
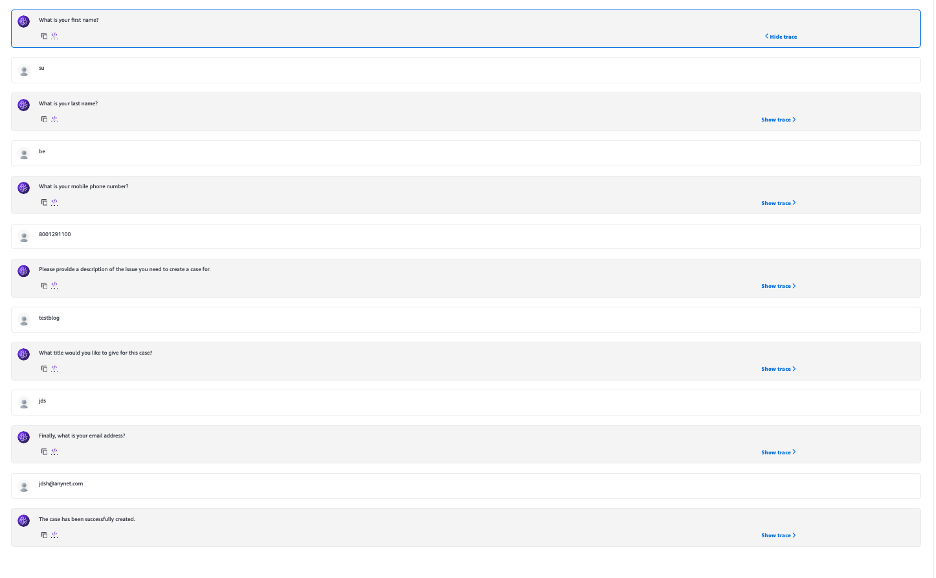
- Provide the required information to the agent.
The agent now creates a case.
Best practices
Consider the following best practices for building efficient and well-architected generative AI applications:
Clean up
To avoid incurring future charges, delete the resources you created. To clean up the AWS environment, complete the following steps:
- Empty the contents of the S3 buckets you created as part of the CloudFormation stack.
- Delete the agent from Amazon Bedrock.
- Delete the CloudFormation stack you created.
Conclusion
Integrating generative AI with existing systems is crucial to unlocking its transformative potential. By using tools like Amazon Bedrock Agents, organizations can seamlessly connect generative AI to core data and workflows, enabling automation, content generation, and problem-solving while maintaining connectivity. The strategies and techniques showcased in this post demonstrate how generative AI can be orchestrated to drive maximum value across a wide range of use cases, from extracting intelligence from regulatory submissions to providing prescriptive guidance to industry. As generative AI continues to evolve, the ability to integrate it with existing infrastructure will be paramount to realizing its true business impact.
To get started with integrating generative AI into your business, explore How Amazon Bedrock Agents works and discover how you can unlock the transformative potential of this technology across your organization.
Stay up to date with the latest advancements in generative AI and start building on AWS. If you’re seeking assistance on how to begin, check out the Generative AI Innovation Center.
About the Authors
Sujatha Dantuluri is a seasoned Senior Solutions Architect in the US federal civilian team at AWS, with over two decades of experience supporting commercial and federal government clients. Her expertise lies in architecting mission-critical solutions and working closely with customers to ensure their success. Sujatha is an accomplished public speaker, frequently sharing her insights and knowledge at industry events and conferences.
Arianna Burgman is a Solutions Architect at AWS based in NYC, supporting state and local government agencies. She is a data and AI enthusiast with experience collaborating with organizations to architect technical solutions that further their missions for continuous innovation and positive, lasting impact.
Annie Cimack is an Associate Solutions Architect based in Arlington, VA, supporting public sector customers across the federal government as well as higher education. Her area of focus is data analytics, and she works closely with customers of all sizes to support projects ranging from storage to intelligent document processing.
Sunil Bemarkar is a Sr. Partner Solutions Architect at AWS based out of San Francisco with over 20 years of experience in the information technology field. He works with various independent software vendors and AWS partners specialized in cloud management tools and DevOps segments to develop joint solutions and accelerate cloud adoption on AWS.
Marcelo Silva is a Principal Product Manager at Amazon Web Services, leading strategy and growth for Amazon Bedrock Knowledge Bases and Amazon Lex.
Read More