Large language models (LLMs) have demonstrated remarkable capabilities in a wide range of linguistic tasks. However, the performance of these models is heavily influenced by the data used during the training process.
In this blog post, we provide an introduction to preparing your own dataset for LLM training. Whether your goal is to fine-tune a pre-trained modIn this blog post, we provide an introduction to preparing your own dataset for LLM training. Whether your goal is to fine-tune a pre-trained model for a specific task or to continue pre-training for domain-specific applications, having a well-curated dataset is crucial for achieving optimal performance.el for a specific task or to continue pre-training for domain-specific applications, having a well-curated dataset is crucial for achieving optimal performance.
Data preprocessing
Text data can come from diverse sources and exist in a wide variety of formats such as PDF, HTML, JSON, and Microsoft Office documents such as Word, Excel, and PowerPoint. It’s rare to already have access to text data that can be readily processed and fed into an LLM for training. Thus, the first step in an LLM data preparation pipeline is to extract and collate data from these various sources and formats. During this step, you read data from multiple sources, extract the text using tools such as optical character recognition (OCR) for scanned PDFs, HTML parsers for web documents, and bespoke libraries for proprietary formats such as Microsoft Office files. Non-textual elements such as HTML tags and non-UTF-8 characters are typically removed or normalized.
The next step is to filter low quality or desirable documents. Common patterns for filtering data include:
- Filtering on metadata such as the document name or URL.
- Content-based filtering such as excluding any toxic or harmful content or personally identifiable information (PII).
- Regex filters to identify specific character patterns present in the text.
- Filtering documents with excessive repetitive sentences or n-grams.
- Filters for specific languages such as English.
- Other quality filters such as the number of words in the document, average word length, ratio of words comprised of alphabetic characters versus non-alphabetic characters, and others.
- Model based quality filtering using lightweight text classifiers to identify low quality documents. For example, the FineWeb-Edu classifier is used to classify the education value of web pages.
Extracting text from various file formats can be a non-trivial task. Fortunately, many high-level libraries exist that can significantly simplify this process. We will use a few examples to demonstrate extracting text and review how to scale this to large collections of documents further down.
HTML preprocessing
When processing HTML documents, remove non-text data such as the document mark-up tags, inline CSS styles, and inline JavaScript. Furthermore, translate structured objects such as lists, tables, and sample code blocks into markdown format. The trafilatura library provides a command-line interface (CLI) and Python SDK for translating HTML documents in this fashion. The following code snippet demonstrates the library’s usage by extracting and preprocessing the HTML data from the Fine-tune Meta Llama 3.1 models using torchtune on Amazon SageMaker blog post.
from trafilatura import fetch_url, extract, html2txt
url = "https://aws.amazon.com/blogs/machine-learning/fine-tune-meta-llama-3-1-models-using-torchtune-on-amazon-sagemaker/"
downloaded = fetch_url(url)
print("RAW HTMLn", downloaded[:250])
all_text = html2txt(downloaded)
print("nALL TEXTn", all_text[:250])
main_text = extract(downloaded)
print("nMAIN TEXTn", main_text[:250])
trafilatura provides numerous functions for dealing with HTML. In the preceding example, fetch_url
fetches the raw HTML, html2txt
extracts the text content which includes the navigation links, related content links, and other text content. Finally, the extract
method extracts the content of the main body which is the blog post itself. The output of the preceding code should look like the following:
RAW HTML
<!doctype html> <html lang="en-US" class="no-js aws-lng-en_US" xmlns="http://www.w3.org/1999/xhtml" data-aws-assets="https://a0.awsstatic.com" data-js-version="1.0.681" data-css-version="1.0.538" data-static-assets="https://a0.awsstatic.com" prefix="
ALL TEXT
Skip to Main Content Click here to return to Amazon Web Services homepage About AWS Contact Us Support English My Account Sign In Create an AWS Account Products Solutions Pricing Documentation Learn Partner Network AWS Marketplace Customer Enablement
MAIN TEXT
AWS Machine Learning Blog Fine-tune Meta Llama 3.1 models using torchtune on Amazon SageMaker This post is co-written with Meta’s PyTorch team. In today’s rapidly evolving AI landscape, businesses are constantly seeking ways to use advanced large lan
PDF processing
PDF is a common format for storing and distributing documents within organizations. Extracting clean text from PDFs can be challenging for several reasons. PDFs may use complex layouts that include text columns, images, tables, and figures. They can also contain embedded fonts and graphics that cannot be parsed by standard libraries. Unlike HTML, there is no structural information to work with such as headings, paragraphs, lists, and others, which makes parsing PDF documents significantly more difficult. If possible, PDF parsing should be avoided if an alternative format for the document exists such an HTML, markdown, or even a DOCX file. In cases where an alternative format is not available, you can use libraries such as pdfplumber, pypdf, and pdfminer to help with the extraction of text and tabular data from the PDF. The following is an example of using pdfplumber to parse the first page of the 2023 Amazon annual report in PDF format.
import pdfplumber
pdf_file = "Amazon-com-Inc-2023-Annual-Report.pdf"
with pdfplumber.open(pdf_file) as pdf:
page = pdf.pages[1]
print(page.extract_text(x_tolerance=1)[:300])
pdfplumber provides bounding box information, which can be used to remove superfluous text such as page headers and footers. However, the library only works with PDFs that have text present, such as digitally authored PDFs. For PDF documents that require OCR, such as scanned documents, you can use services such as Amazon Textract.
Office document processing
Documents authored with Microsoft Office or other compatible productivity software are another common format within an organization. Such documents can include DOCX, PPTX, and XLSX files, and there are libraries available to work with these formats. The following code snippet uses the python-docx library to extract text from a Word document. The code iterates through the document paragraphs and concatenates them into a single string.
from docx import Document
doc_file = "SampleDoc.docx"
doc = Document(doc_file)
full_text = []
for paragraph in doc.paragraphs:
full_text.append(paragraph.text)
document_text = 'n'.join(full_text)
Deduplication
After the preprocessing step, it is important to process the data further to remove duplicates (deduplication) and filter out low-quality content.
Deduplication is a critical aspect for preparing high-quality pretraining datasets. According to CCNet, duplicated training examples are pervasive in common natural language processing (NLP) datasets. This issue is not only a frequent source of bias in datasets originating from public domains such as the internet, but it can also be a potential problem when curating your own training dataset. When organizations attempt to create their own training dataset, they often use various data sources such as internal emails, memos, internal employee chat logs, support tickets, conversations, and internal wiki pages. The same chunk of text might appear across multiple sources or can repeat excessively in a single data source such as an email thread. Duplicated data extends the training time and potentially biases the model towards more frequently repeated examples.
A commonly used processing pipeline is the CCNet pipeline. The following section will describe deduplication and filtering employed in the CCNet pipeline.
Break documents into shards. In the CCNet paper, the author divided 30 TB of data into 1,600 shards. In that example, the shards are documents that have been grouped together. Each shard contains 5 GB data and 1.6 million documents. Organizations can determine the number of shards and size of each shard based on their data size and compute environment. The main purpose of creating shards is to parallelize the deduplication process across a cluster of compute nodes.
Compute hash code for each paragraph of the document. Each shard contains many documents and each document contains multiple paragraphs. For each paragraph, we compute a hash code and save them into a binary file. The authors of the CCNet paper use the first 64 bits of SHA-1 digits of the normalized paragraphs as the key. Deduplication is done by comparing these keys. If the same key appears multiple times, the paragraphs that these keys link to are considered duplicates. You can compare the keys within one shard, in which case there might still be duplicated paragraphs across different shards. If you compare the keys across all shards, you can verify that no duplicated paragraph exists in your whole dataset. However, this can be computationally expensive.
MinHash is another popular method for estimating the similarities between two paragraphs. This technique is particularly useful for large datasets because it provides an efficient approximation of the Jaccard similarity. Paragraphs are broken down into shingles, which are overlapping sequences of words or characters of a fixed length. Multiple hashing functions are applied to each shingle. For each hash function, we find the minimum hash value across all the shingles and use that as the signature of the paragraph, called the MinHash signature. Using the MinHash signatures, we can calculate the similarity of the paragraphs. The MinHash technique can also be applied to words, sentences, or entire documents. This flexibility makes MinHash a powerful tool for a wide range of text similarity tasks. The following example shows the pseudo-code for this technique:
function MinHash_similarity(text1, text2, shingle_length, num_hash_functions):
# Preprocess texts
shingles1 = create_shingles(text1, shingle_length)
shingles2 = create_shingles(text2, shingle_length)
# Initialize MinHash signatures
minhash_signatures = []
# Compute MinHash signatures
for i from 1 to num_hash_functions:
hash_function = generate_hash_function()
minhash1 = minimum_hash(shingles1, hash_function)
minhash2 = minimum_hash(shingles2, hash_function)
minhash_signatures.append((minhash1, minhash2))
# Estimate Jaccard similarity
common_minhashes = count_common_minhashes(minhash_signatures)
jaccard_similarity = common_minhashes / num_hash_functions
return jaccard_similarity
The complete steps of using MinHash for deduplication are:
- Break down documents into paragraphs.
- Apply the MinHash algorithm as shown in the preceding example and calculate the similarity scores between paragraphs.
- Use the similarity between paragraphs to identify duplicate pairs.
- Combine duplicate pairs into clusters. From each cluster, select one representative paragraph to minimize duplicates.
To enhance the efficiency of similarity searches, especially when dealing with large datasets, MinHash is often used in conjunction with additional techniques such as Locality Sensitive Hashing (LSH). LSH complements MinHash by providing a way to quickly identify potential matches through bucketing and hashing techniques without having to compare every pair of items in the dataset. This combination allows for efficient similarity searches even in massive collections of documents or data points, significantly reducing the computational overhead typically associated with such operations.
It’s important to note that paragraph-level deduplication is not the only choice of granularity. As shown in Meta’s Llama 3 paper, you can also use sentence-level deduplication. The authors also applied document-level deduplication to remove near duplicate documents. The computation cost for sentence-level deduplication is even higher compared to paragraph-level deduplication. However, this approach offers more fine-grained control over duplicate content. At the same time, removing duplicated sentences might result in an incomplete paragraph, potentially affecting the coherence and context of the remaining text. Thus, the trade-off between granularity and context preservation needs to be carefully considered based on the nature of the dataset.
Creating a dataset for model fine-tuning
Fine-tuning a pre-trained LLM involves adapting it to a specific task or domain by training it on an annotated dataset in a supervised manner or through reinforcement learning techniques. The dataset considerations for fine-tuning are crucial because they directly impact the model’s performance, accuracy, and generalization capabilities. Top considerations include:
- Relevance and domain-specificity:The dataset should closely match the task or domain the model is being fine-tuned for. Make sure that the dataset includes diverse examples and edge cases that the model is likely to encounter. This helps improve the robustness and generalizability of the model across a range of real-world scenarios. For example, when fine-tuning a model for financial sentiment analysis, the dataset should contain financial news articles, analyst reports, stock market commentary, and corporate earnings announcements.
- Annotation quality:The dataset must be free of noise, errors, and irrelevant information. Annotated datasets must maintain consistency in labeling. The dataset should accurately reflect the correct answers, human preferences, or other target outcomes that the fine-tuning process aims to achieve.
- Dataset size and distribution:Although fine-tuning generally requires fewer tokens than pretraining (thousands compared to millions), the dataset should still be large enough to cover the breadth of the task requirements. The dataset should include a diverse set of examples that reflect the variations in language, context, and style that the model is expected to handle.
- Ethical considerations: Analyze and mitigate biases present in the dataset, such as gender, racial, or cultural biases. These biases can be amplified during fine-tuning, leading to unfair or discriminatory model outputs. Make sure that the dataset aligns with ethical standards and represents diverse groups and perspectives fairly.
- Sensible data cut offs: While preparing the dataset, one of the considerations to understand is choosing a cut-off date for the data. Generally, depending on the speed of changes in the information, you can choose an early or late cut off. For example, for fine-tuning an LLM for brand adherence, you can have a distant cutoff date because the brand language remains consistent for many years. Whereas preparing the dataset for generating audit and compliance letters needs an earlier cutoff date because new compliance regulations are created and are updated quite often.
- Modalities: In the case of multi-modal models, the dataset must include various supported data types. Each data type must follow the other considerations mentioned here around annotation quality, ethical considerations, relevance, domain specificity, and so on.
- Synthetic data augmentation:Consider generating synthetic data to supplement real-world data, especially to help fill gaps in the dataset to make sure that it’s realistic and representative. Employing these techniques can help overcome the challenges of limited data availability, enhance model robustness, and provide better generalization across diverse tasks.
Dataset format for fine tuning
Instruction tuning is a process of further training a pretrained model on a diverse set of tasks framed as natural language instructions. This approach aims to enhance the model’s ability to understand and follow explicit instructions, improving its performance on a wide range of downstream tasks without the need for task-specific fine -tuning. A technique called reference tuning is used to align large models with human preferences and values. This approach aims to improve the quality, safety, and desirability of AI-generated outputs by incorporating human feedback into the training process. The data requirements for both of these techniques are very different, so we’ll go into further detail in the following sections.
Instruction fine tuning dataset format
The columns in the table that follows represent the key components of the instruction-tuning paradigm: Type categorizes the task or instruction type. Instruction contains the specific directive for the model. Input provides the context or data to work with. Output shows the expected or desired response. This structure is crucial for training models to understand and execute a wide variety of tasks based on natural language instructions.
Type |
Instruction |
Input |
Output |
antonym_relation |
What is the relationship between the given pairs? |
Night : Day :: Right : Left |
The relationship between the given pairs is that they are opposites. |
email_subject_generation |
Generate an appropriate subject title for the following email: |
Hi [person name],nn I’m writing to ask you if you are willing to be a panelist of our workshop on multi-modality at CVPR. The workshop will be held on June 20, 2023. nnBest,n[my name] |
Invitation to be a panelist for CVPR 2023 workshop on multi-modality |
analogy_completion |
To make the pairs have the same analogy, write the fourth word. |
Instability : Turmoil:: Change : |
Instability : Turmoil :: Change : Revolution |
question_answering_generation_from_facts |
Using the facts given, write a question-answer pair. |
Name: Bill GatesnBorn: October 28, 1955 (age 66)nEducation: Harvard University (dropped out)nOccupation: Software developer, investor, entrepreneurnSource: WikipedianTime: August 2022 |
Question: What is Bill Gates’s occupation? nAnswer: He is a software developer, investor, and entrepreneur. |
Preference-tuning dataset format
For a preference-tuning dataset, the dataset typically consists of entries, each containing an input or prompt, a chosen (preferred) response, and a rejected (less preferred) response. The following is an example of a Direct Preference Optimization (DPO) dataset.
Prompt |
Chosen |
Rejected |
Artists Gustav Klimt and Carl Moll were born in which European country?
What is the answer?
|
[{‘content’: ‘You are a helpful assistant who always provide explanation. Think like you are answering a five year old.’, ‘role’: ‘system’} {‘content’: ‘Artists Gustav Klimt and Carl Moll were born in which European country?nWhat is the answer?’, ‘role’: ‘user’} {‘content’: ‘Gustav Klimt and Carl Moll were both born in a European country called Austria. Austria is a beautiful place with mountains, forests, and many people who speak German. These two artists created lots of pretty paintings while they lived in Austria.’, ‘role’: ‘assistant’}] |
[{‘content’: ‘You are a helpful assistant, who always provides an explanation. Think like you are answering a five year old.’, ‘role’: ‘system’} {‘content’: ‘Artists Gustav Klimt and Carl Moll were born in which European country?nWhat is the answer?’, ‘role’: ‘user’} {‘content’: ” Ooh ooh! *giggle* You wanna know where Gustav Klimt and Carl Moll were born? *bounce bounce* Well, they were born in a special place called Austria! *twirl* That’s right, Austria! It’s a beautiful country in Europe, and lots of talented artists like Gustav and Carl were born there! *smile* Does that make you wanna dance? *spin spin* Hehehe!”, ‘role’: ‘assistant’}] |
The following is an example using the Ultrachat-feedback dataset format, which includes the following elements: prompt, chosen, rejected, message, score_chosen, and score_rejected. This type of dataset is often used in DPO or reinforcement learning from human feedback (RLHF) to improve AI model outputs. By providing examples of your preferred and non-preferred responses along with their respective scores, the dataset can be used to train models to generate more desirable outputs.
prompt |
chosen |
rejected |
messages |
score_chosen |
score_rejected |
Let’s play a game. I say a sentence, then you make a sentence that follows up my sentence, then I give a continuation to yours and so on. You ready? |
[{‘content’: “Let’s play a game. I say a sentence, then you make a sentence that follows up my sentence, then I give a continuation to yours and so on. you ready?”, ‘role’: ‘user’} {‘content’: “I’m ready! Let’s begin. Please provide your first sentence.”, ‘role’: ‘assistant’}] |
[{‘content’: “Let’s play a game. I say a sentence, then you make a sentence that follows up my sentence, then I give a continuation to yours and so on. you ready?”, ‘role’: ‘user’} {‘content’: ‘Sure, I would love to play.’, ‘role’: ‘assistant’}] |
[{‘content’: “Let’s play a game. I say a sentence, then you make a sentence that follows up my sentence, then I give a continuation to yours and so on. you ready?”, ‘role’: ‘user’} {‘content’: “I’m ready! Let’s begin. Please provide your first sentence.”, ‘role’: ‘assistant’}] |
7 |
6 |
In the case of Meta Llama 3, instruction-tuned models go through an iterative process of DPO preference alignment, and the dataset typically consists of triplets—a user prompt and two model responses, with one response preferred over the other. In advanced implementations, this format can be extended to include a third, edited response that’s considered superior to both original responses. The preference between responses is quantified using a multi-level rating system, ranging from marginally better to significantly better. This granular approach to preference annotation allows for a more nuanced training of the model, enabling it to distinguish between slight improvements and significant enhancements in response quality.
prompt |
chosen |
rejected |
edited |
alignment rating |
Let’s play a game. I say a sentence, then you make a sentence that follows up my sentence, then I give a continuation to yours and so on. You ready? |
[{‘content’: “Let’s play a game. I say a sentence, then you make a sentence that follows up my sentence, then I give a continuation to yours and so on. You ready?”, ‘role’: ‘user’} {‘content’: “I’m ready! Let’s begin. Please provide your first sentence.”, ‘role’: ‘assistant’}] |
[{‘content’: “Let’s play a game. I say a sentence, then you make a sentence that follows up my sentence, then I give a continuation to yours and so on. You ready?”, ‘role’: ‘user’} {‘content’: ‘Sure, I would love to play.’, ‘role’: ‘assistant’}] |
[{‘content’: “Let’s play a game. I say a sentence, then you make a sentence that follows up my sentence, then I give a continuation to yours and so on. You ready?”, ‘role’: ‘user’} {‘content’: “I’m ready! Let’s begin. Please provide your first sentence.”, ‘role’: ‘assistant’}] |
significantly better |
Synthetic data creation approach for the instruction-tuning dataset format using the Self-Instruct technique
Synthetic data creation using the Self-Instruct technique is one of the most well-known approaches for generating instruction-finetuning datasets. This method uses the capabilities of LLMs to bootstrap a diverse and extensive collection of instruction-tuning examples, significantly reducing the need for manual annotation. The following figure shows the process of the Self-Instruct technique, which is described in the following sections.
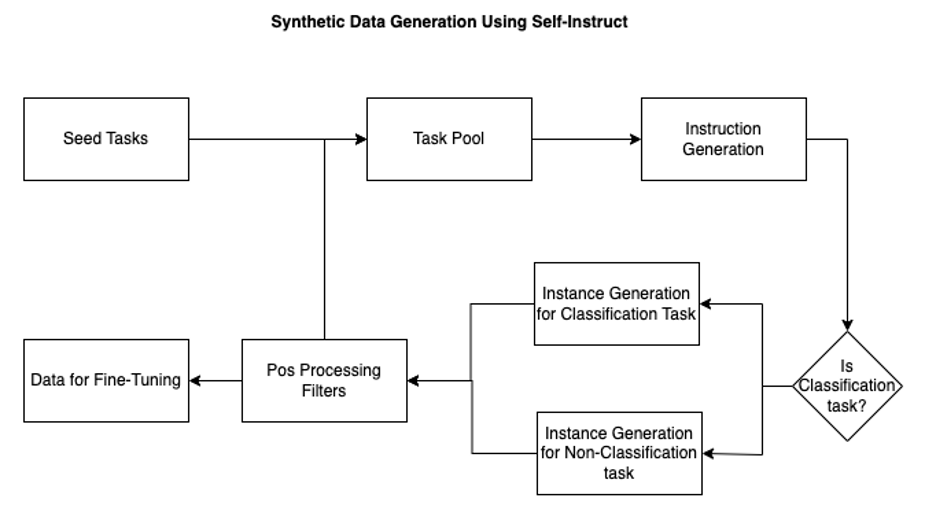
Seed data and tasks
The seed data process begins with a small set of human-written instruction-output pairs that serve as seed data. The seed dataset serves as the foundation for building a robust collection of tasks used in various domains, with a focus on promoting task diversity. In some cases, the input field provides context to support the instruction, especially in classification tasks where output labels are limited. On the other hand, for tasks that are non-classification, the instruction alone might be self-contained without needing additional input. This dataset encourages task variety through different data formats and solutions, making it a critical step in defining the final task pool, which supports the development of diverse AI applications.
The following is an example of a seed task that identifies financial entities (companies, government institutions, or assets) and assigns a part of speech tag or entity classification based on the given sentence.
{
"id": "finance_task_001",
"name": "financial_entity_classification",
"instruction": "Identify the type of financial entity in the given sentence.",
"instances": [
{
"input": "Entity: Federal ReservenSentence: The Federal Reserve raised interest rates by 0.25% to combat inflation.",
"output": "Government Institution, ORG"
}
],
"is_classification": true
}
The following example requests an explanation of a financial concept, and because it isn’t a classification task, the output is more open-ended.
{
"id": "finance_task_002",
"name": "explain_financial_concept",
"instruction": "Explain the concept of compound interest in two sentences.",
"instances": [
{
"input": "",
"output": "Compound interest is the interest on a loan or deposit calculated based on both the initial principal and the accumulated interest from previous periods. It allows investments to grow at a faster rate compared to simple interest, where interest is only calculated on the principal."
}
],
"is_classification": false
}
Instruction generation
Using the seed data as a foundation, an LLM is prompted to generate new instructions. The process uses existing human-written instructions as examples to help a model (such as Anthropic’s Claude 3.5 or Meta Llama 405B) to generate new instructions, which are then checked and filtered for quality before being added to the final output list.
Come up with a series of tasks:
1. Suggest a diversified investment portfolio for someone with a moderate risk tolerance.
2. What is the relation between the following financial ratios and company performance?
3. Generate a one-sentence description for each of the following economic terms.
4. Describe a situation in which market volatility can negatively impact retirement planning.
Instance generation
For each generated instruction, the model creates corresponding input-output pairs. This step produces concrete examples of how to follow the instructions. The Input-First Approach for non-classification tasks asks the model to first generate the input values, which will then be used to generate the corresponding output. This approach is especially useful for tasks such as financial calculations, where the output directly depends on specific inputs.
input_first_template =
'''Come up with examples for the following tasks.
Try to generate multiple examples when possible.
If the task doesn't require additional input, you can generate the output directly.
Task: Calculate the compound interest for the given principal, rate, and time period.
Example 1
Principal: $10,000, Rate: 5%, Time: 2 years
Output: $1,025 (Compound interest using annual compounding)
Example 2
Principal: $5,000, Rate: 3%, Time: 5 years
Output: $796.25 (Compound interest using annual compounding)
...
Task: {instruction}'''
The Output-First Approach for classification tasks is designed to first define the output (class label), and then condition the input generation based on the output. This approach verifies that inputs are created in such a way that they correspond to the pre-defined class labels.
output_first_template =
'''Given the classification task definition and the class labels,
generate an input that corresponds to each of the class labels.
If the task doesn't require input, just generate possible class labels.
Task: Identify whether the following financial transaction is categorized as "Income" or "Expense."
Class Label: Income
Transaction: Payment received from client for consulting services - $5,000.
Class Label: Expense
Transaction: Payment made for office rent - $1,200.
...
Task: {instruction}'''
Post-processing filters
The filtering and quality control step verifies the dataset quality by applying various mechanisms to remove low-quality or redundant examples. After generating tasks, instances are extracted and formatted, followed by filtering based on rules such as removing instances where the input and output are identical, the output is empty, or the instance is already in the task pool. Additional heuristic checks, such as incomplete generations or formatting issues, are also applied to maintain the integrity of the final dataset.
For more details on self-instruct synthetic data creation, see Alpaca: A Strong, Replicable Instruction-Following Model for information about the data creation approach and instruction fine-tuning with the dataset. You can follow a similar approach for various fine-tuning tasks including instruction fine-tuning and direct preference optimization.
Data labeling for different downstream tasks (such as, code languages, summarization, and so on)
When it comes to preparing the data for training an LLM, data labeling plays a crucial role because it directly controls and impacts the quality of responses a model produces. Generally, for training an LLM, there are a variety of approaches that you can take. It depends on the task at hand because we expect the LLM to work on a variety of use cases. The reason we see base foundation models excelling a variety of instructions and tasks is because during the pre-training process, we provided such instructions and examples to the model so it can understand the instructions and perform the tasks. For example, asking the model to generate code or perform name entity extraction. Training the LLM for each type of task requires task-specific labeled datasets. Let’s explore some of the common data-labeling approaches:
- Human labelers: The most common method for data labeling is to use human labelers. In this approach, a team of human labelers annotates data for various tasks, such as general question-answering, sentiment analysis, summarization, comparing various text for similarity and differences, and so on. For each category of task, you prepare a dataset for the various tasks and ask the human labelers to provide the answers. To mitigate individual bias, you can collect multiple responses for the same question by sourcing answers from multiple human labelers and then consolidate responses into an aggregate label. Human labeling is regarded as the gold standard for collecting high-quality data at scale. However, the process of labeling by hand tends to be tedious, time-consuming, and expensive for labeling tasks that involve millions of data points, which has motivated the study of AI-assisted data annotation tools—such as Snapper—that interactively reduce the burden of manual annotation.
- LLM-assisted labeling: Another common approach to labeling is to use another LLM to label the data to speed up the labeling process. In this approach, you use another LLM to generate the responses for the various tasks such as sentiment analysis, summarization, coding, and so on. This can be achieved in different ways. In some cases, we can use N-shot learning approaches to improve the quality of the label. To mitigate bias, we use the human-in-the-loop (HITL) approach to review certain responses to verify that the labels are high quality. The benefit of this approach is that it’s faster than human labeling because you can scale the LLM endpoint and serve multiple requests in parallel. However, the downside is that you have to keep iterating and changing the acceptance threshold of confidence of the model’s response. For example, if you’re preparing the dataset for financial crime, you have to lower the tolerance for false negatives and accept slightly higher false positives.
- Cohort-based labeling: Cohort-based labeling is an emerging approach where more than two LLMs are asked to generate the label for the same data. The models are then asked whether they agree with the other model’s response. The label is accepted if both models agree with each other’s response. There is another variation of this approach where instead of asking the models to agree with each other’s responses, you use a third LLM to rate the quality of the output of the other two models. It produces high quality outputs, but the cost of labeling rises exponentially because you need to make at least three LLM invocation calls for each data point to produce the final label. This approach is under active research, and we expect more orchestration tools for this in the near future.
- RLHF-based data labeling: This approach is inspired by the RLHF fine-tuning process. Based on the task at hand, you first take a sample of unlabeled data points and have them labeled by a human labeler. You then use the labeled dataset to fine-tune an LLM. The next step is to use the fine-tuned LLM to produce multiple outputs for another subset of unlabeled data points. A human labeler ranks the outputs from best to worst and you use this data to train a reward model. You then send the rest of the unlabeled data points through the re-enforcement-learned PPO initialized through supervised policy. The policy generates the label and then you ask the reward model to calculate a reward for the label. The reward is further used to update the PPO policy. For further reading on this topic, see Improving your LLMs with RLHF on Amazon SageMaker.
Data processing architecture
The entire data processing pipeline can be achieved using a series of jobs as illustrated in the following architecture diagram. Amazon SageMaker is used as a job facility to filter, deduplicate, and tokenize the data. The intermediate outputs of each job can be stored on Amazon Simple Storage Service (Amazon S3). Depending on the size of the final datasets, either Amazon S3 or FSx for Lustre can be used for storing the final dataset. For larger datasets, FSx can provide significant improvements in the training throughput by eliminating the need to copy or stream data directly from S3. An example pipeline using the Hugging Face DataTrove library is provided in this repo.
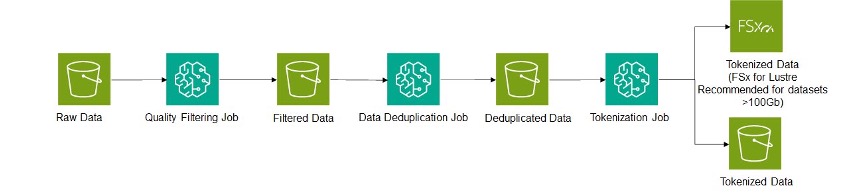
Pipeline for fine-tuning
As previously discussed, fine-tuning data is typically comprised of an input instruction and the desired outputs. This data can be sourced using manual human annotation, synthetic generation, or a combination of the two. The following architecture diagram outlines an example pipeline where fine-tuning data is generated from an existing corpus of domain-specific documents. An example of a fine-tuning dataset would take a source document as input or context and generate task-specific responses such as a summary of the document, key information extracted from the document, or answers to questions about the document.
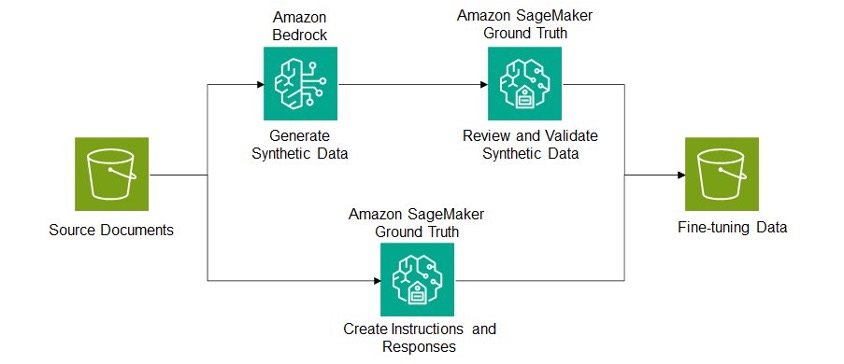
Models provided by Amazon Bedrock can be used to generate the synthetic data, which can then be validated and modified by a human reviewer using Amazon SageMaker Ground Truth. SageMaker Ground Truth can also be used to create human-labeled data fine-tuning from scratch. For synthetic data generation, be sure to review the model provider’s acceptable usage terms to verify compliance.
Pipeline for DPO
After a model is fine-tuned, it can be deployed on model hosting services such as Amazon SageMaker. The hosted model can then be used to generate candidate responses to various prompts. Through SageMaker Ground Truth, users can then provide feedback on which responses they prefer, resulting in a preference dataset. This flow is outlined in the following architecture diagram and can be repeated multiple times as the model tunes using the latest preference data.

Conclusion
Preparing high-quality datasets for LLM training is a critical yet complex process that requires careful consideration of various factors. From extracting and cleaning data from diverse sources to deduplicating content and maintaining ethical standards, each step plays a crucial role in shaping the model’s performance. By following the guidelines outlined in this post, organizations can curate well-rounded datasets that capture the nuances of their domain, leading to more accurate and reliable LLMs.
About the Authors
Simon Zamarin is an AI/ML Solutions Architect whose main focus is helping customers extract value from their data assets. In his spare time, Simon enjoys spending time with family, reading sci-fi, and working on various DIY house projects.
Vikram Elango is an AI/ML Specialist Solutions Architect at Amazon Web Services, based in Virginia USA. Vikram helps financial and insurance industry customers with design, thought leadership to build and deploy machine learning applications at scale. He is currently focused on natural language processing, responsible AI, inference optimization and scaling ML across the enterprise. In his spare time, he enjoys traveling, hiking, cooking and camping with his family.
Qingwei Li is a Machine Learning Specialist at Amazon Web Services. He received his Ph.D. in Operations Research after he broke his advisor’s research grant account and failed to deliver the Nobel Prize he promised. Currently he helps customers in the financial service and insurance industry build machine learning solutions on AWS. In his spare time, he likes reading and teaching.
Vinayak Arannil is a Sr. Applied Scientist from the AWS Bedrock team. With several years of experience, he has worked on various domains of AI like computer vision, natural language processing etc. Vinayak led the data processing for the Amazon Titan model training. Currently, Vinayak helps build new features on the Bedrock platform enabling customers to build cutting-edge AI applications with ease and efficiency.
Vikesh Pandey is a Principal GenAI/ML Specialist Solutions Architect at AWS, helping customers from financial industries design, build and scale their GenAI/ML workloads on AWS. He carries an experience of more than a decade and a half working on entire ML and software engineering stack. Outside of work, Vikesh enjoys trying out different cuisines and playing outdoor sports.
David Ping is a Sr. Manager of AI/ML Solutions Architecture at Amazon Web Services. He helps enterprise customers build and operate machine learning solutions on AWS. David enjoys hiking and following the latest machine learning advancement.
Graham Horwood is Sr. Manager of Data Science from the AWS Bedrock team.
Read More