Amazon IIT–Bombay AI-ML Initiative seeks to advance artificial intelligence and machine learning research within speech, language, and multimodal-AI domains.Read More
Deploy a Microsoft Teams gateway for Amazon Q, your business expert
Amazon Q is a new generative AI-powered application that helps users get work done. Amazon Q can become your tailored business expert and let you discover content, brainstorm ideas, or create summaries using your company’s data safely and securely. You can use Amazon Q to have conversations, solve problems, generate content, gain insights, and take action by connecting to your company’s information repositories, code, data, and enterprise systems. For more information, see Introducing Amazon Q, a new generative AI-powered assistant (preview).
In this post, we show you how to bring Amazon Q, your business expert, to users in Microsoft Teams. (If you use Slack, refer to Deploy a Slack gateway for Amazon Q, your business expert.)
You’ll be able converse with Amazon Q business expert using Teams direct messages (DMs) to ask questions and get answers based on company data, get help creating new content such as email drafts, summarize attached files, and perform tasks.
You can also invite Amazon Q business expert to participate in your Teams channels. In a channel, users can ask Amazon Q business expert questions in a new message, or tag it in an existing thread at any point, to provide additional data points, resolve a debate, or summarize the conversation and capture the next steps.
Solution overview
Amazon Q business expert is amazingly powerful. Check out the following demo—seeing is believing!
In the demo, our Amazon Q business expert application is populated with some Wikipedia pages. You can populate your Amazon Q business expert application with your own company’s documents and knowledge base articles, so it will be able to answer your specific questions!
Everything you need is provided as open source in our GitHub repo.
In this post, we walk you through the process to deploy Amazon Q business expert in your AWS account and add it to Microsoft Teams. When you’re done, you’ll wonder how you ever managed without it!
The following are some of the things it can do:
- Respond to messages – In DMs, it responds to all messages. In channels, it responds only to @mentions and responds in a conversation thread.
- Render answers containing markdown – This includes headings, lists, bold, italics, tables, and more.
- Track sentiment – It provides thumbs up and thumbs down buttons to track user sentiment.
- Provide source attribution – It provides references and hyperlinks to sources used by Amazon Q business expert.
- Understand conversation context – It tracks the conversation and responds based on the context.
- Stay aware of multiple users – When it’s tagged in a thread, it knows who said what, and when, so it can contribute in context and accurately summarize the thread when asked.
- Process attached files – It can process up to five attached files for document question answering, summaries, and more.
- Start new conversations – You can reset and start new conversations in DM chats by using
/new_conversation
.
In the following sections, we show how to deploy the project to your own AWS account and Teams account, and start experimenting!
Prerequisites
You need to have an AWS account and an AWS Identity and Access Management (IAM) role and user with permissions to create and manage the necessary resources and components for this application. If you don’t have an AWS account, see How do I create and activate a new Amazon Web Services account?
You also need to have an existing, working Amazon Q business expert application. If you haven’t set one up yet, see Creating an Amazon Q application.
Lastly, you need a Microsoft account and a Microsoft Teams subscription to create and publish the app using the steps outlined in this post. If you don’t have these, see if your company can create sandboxes for you to experiment, or create a new account and trial subscription as needed to complete the steps.
Deploy the solution resources
We’ve provided pre-built AWS CloudFormation templates that deploy everything you need in your AWS account.
If you’re a developer and you want to build, deploy, or publish the solution from code, refer to the Developer README.
Complete the following steps to launch the CloudFormation stack:
- Log in to the AWS Management Console.
- Choose one of the following Launch Stack buttons for your desired AWS Region to open the AWS CloudFormation console and create a new stack.
Region | Launch Stack |
---|---|
N. Virginia (us-east-1 ) |
![]() |
Oregon (us-west-2 ) |
![]() |
- For Stack name, enter a name for your app (for example,
AMAZON-Q-TEAMS-GATEWAY
). - For AmazonQAppId, enter your existing Amazon Q business expert application ID (for example,
80xxxxx9-7xx3-4xx0-bxx4-5baxxxxx2af5
). You can copy it from the Amazon Q business expert console. - For AmazonQRegion, choose the Region where you created your Amazon Q business expert application (us-east-1 or us-west-2).
- For AmazonQUserId, enter an Amazon Q business expert user ID email address (leave blank to use a Teams user email as the user ID).
- For ContextDaysToLive, enter the length of time to keep conversation metadata cached in Amazon DynamoDB (you can leave this as the default).
When your CloudFormation stack status is CREATE_COMPLETE, choose the Outputs tab, and keep it open—you’ll need it in later steps.
Register a new app in the Microsoft Azure portal
Complete the following steps to register a new app in the Microsoft Azure portal:
- Go to the Azure Portal and log in with your Microsoft account.
- Choose New registration.
- For Name, provide the name for your app. You can keep things simple by using the stack name you used for the CloudFormation stack.
- For Who can use this application or access this API?, choose Accounts in this organizational directory only (AWS only – Single tenant).
- Choose Register.
- Note down the Application (client) ID value and the Directory (tenant) ID from the Overview page. You’ll need them later when asked for
MicrosoftAppId
andMicrosoftAppTenantId
.
- Choose Select API permissions in the navigation pane.
- Choose Add a permission.
- Choose Microsoft Graph.
- Choose Application permissions.
- Select User.Read.All.
- Select ChannelMessage.Read.All.
- Select Team.ReadBasic.All.
- Select Files.Read.All.
- Choose Add permissions. This permission allows the app to read data in your organization’s directory about the signed-in user.
- Use the options menu (three dots) on the right to choose Remove permission.
- Remove the original User.Read – Delegated permission.
- Choose Grant admin consent for Default Directory.
- Choose Certificates & secrets in the navigation pane.
- Choose New client secret.
- For Description, provide a value, such as
description of my client secret
. - Choose a value for Expires. Note that in production, you’ll need to manually rotate your secret before it expires.
- Choose Add.
- Note down the value for your new secret. You’ll need it later when asked for
MicrosoftAppPassword
.
- Optionally, choose Owners to add any additional owners for the application.
Register your new app in the Microsoft Bot Framework
Complete the following steps to register your app in the Microsoft Bot Framework:
- Go to the Microsoft Bot Framework and log in with your Microsoft account.
- Optionally, you can create and upload a custom icon for your new Amazon Q business expert bot. For example, we created the following using Amazon Bedrock image playground.
- Enter your preferred display name, bot handle, and description.
- For Messaging endpoint, copy and paste the value of
TeamsEventHandlerApiEndpoint
from your stack Outputs tab. - Do not select Enable Streaming Endpoint.
- For App type, choose Single Tenant.
- For Paste your app ID below to continue, enter the
MicrosoftAppId
value you noted earlier. - For App Tenant ID, enter the
MicrosoftAppTenantId
value you noted earlier. - Leave the other values as they are, agree to the terms, and choose Register.
- On the Channels page, under Add a featured channel, choose Microsoft Teams.
- Choose Microsoft Teams Commercial (most common), then choose Save.
- Agree to the Terms of Service and choose Agree.
Configure your secrets in AWS
Let’s configure your Teams secrets in order to verify the signature of each request and post on behalf of your Amazon Q business expert bot.
In this example, we are not enabling Teams token rotation. You can enable it for a production app by implementing rotation via AWS Secrets Manager. Create an issue (or, better yet, a pull request) in the GitHub repo if you want this feature added to a future version.
Complete the following steps to configure a secret in Secrets Manager:
- On the AWS CloudFormation console, navigate to your stack Outputs tab and choose the link for
TeamsSecretConsoleUrl
to be redirected to the Secrets Manager console. - Choose Retrieve secret value.
- Choose Edit.
- Replace the values of
MicrosoftAppId
,MicrosoftAppPassword
, andMicrosoftAppTenantId
with the values you noted in the previous steps.
Deploy your app into Microsoft Teams
Complete the following steps to deploy the app to Teams:
- Go to the Developer Portal for Teams and log in with your Microsoft Teams user account.
- Choose Apps in the navigation pane, then choose New app.
- For Name, enter your bot name.
- Enter a name for Full name and both short and full descriptions (you can use the bot name for them all if you want, just don’t leave them empty).
- Enter values for Developer information and App URLs. For testing, you can make up values, and URLs like
https://www.anycompany.com/
. Use real ones for production. - For Application (client) ID*, enter the value of
MicrosoftAppId
from earlier. - Choose Save.
- Under Branding, you can upload AI-generated icons, or different icons, or none at all, it’s up to you. The following are some examples:
- Under App features, choose Bot.
- Select Enter a bot ID, and enter the
MicrosoftAppId
value from the earlier steps. - Under What can your bot do?, select Upload and download files.
- Under Select the scopes in which people can use this command, select Personal, Team, and Group chat.
- Choose Save.
- Select Enter a bot ID, and enter the
- Choose Publish.
- Choose Download the app package to download a .zip file to your computer.
- Choose Preview in Teams to launch Microsoft Teams (work or school) app.
- In the navigation pane, choose Apps, then Manage your apps, then Upload an app.
- Choose Upload an app to your orgs app catalog, and select the .zip file you downloaded. This adds the app to Teams.
- Select the card for your new app, choose Add, and wait for it to complete (10–20 seconds).
Add your bot to one or more teams
Complete the following step to add your bot to a team:
- In the Teams app, select your team and choose Manage team.
- On the Apps tab, choose the new Amazon Q business expert app, and choose Add.
Now you can test your bot in Microsoft Teams!
Start using Amazon Q business expert
Complete the following steps to start using Amazon Q business expert in Teams:
- Open your Teams client.
- Under Apps, add your new Amazon Q business expert app to a chat.
- Optionally, add your Amazon Q business expert app to one or more Teams channels.
- In the app DM chat, enter
Hello
.
You have now deployed a powerful new AI assistant into your sandbox Teams environment.
Play with it, try all the features discussed in this post, and copy the things you saw in the demo video. Most importantly, you can ask about topics related to the documents that you have ingested into your own Amazon Q business expert application. But don’t stop there. You can find additional ways to make it useful, and when you do, let us know by posting a comment.
Once you are convinced how useful it is, talk to your Teams admins (show them this post) and work with them to deploy it in your company’s Teams organizations. Your fellow employees will thank you!
Clean up
When you’re finished experimenting with this solution, delete your app in Microsoft Teams, Bot Framework, and Azure portal. Then clean up your AWS resources by opening the AWS CloudFormation console and deleting the AMAZON-Q-TEAMS-GATEWAY
stack that you deployed. This deletes the resources that you created by deploying the solution.
Conclusions
The sample Amazon Q business expert Teams application discussed in this post is provided as open source—you can use it as a starting point for your own solution, and help us make it better by contributing back fixes and features via GitHub pull requests. Explore the code, choose Watch in the GitHub repo to be notified of new releases, and check back for the latest updates. We’d also love to hear your suggestions for improvements and features.
For more information on Amazon Q business expert, refer to the Amazon Q (For Business Use) Developer Guide.
About the Authors
Gary Benattar is a Senior Software Development Manager in AWS HR. Gary started at Amazon in 2012 as an intern, focusing on building scalable, real-time outlier detection systems. He worked in Seattle and Luxembourg and is now based in Tel Aviv, Israel, where he dedicates his time to building software to revolutionize the future of Human Resources. He co-founded a startup, Zengo, with a focus on making digital wallets secure through multi-party computation. He received his MSc in Software Engineering from Sorbonne University in Paris.
Bob Strahan is a Principal Solutions Architect in the AWS Language AI Services team.
Build enterprise-ready generative AI solutions with Cohere foundation models in Amazon Bedrock and Weaviate vector database on AWS Marketplace
Generative AI solutions have the potential to transform businesses by boosting productivity and improving customer experiences, and using large language models (LLMs) with these solutions has become increasingly popular. Building proofs of concept is relatively straightforward because cutting-edge foundation models are available from specialized providers through a simple API call. Therefore, organizations of various sizes and across different industries have begun to reimagine their products and processes using generative AI.
Despite their wealth of general knowledge, state-of-the-art LLMs only have access to the information they were trained on. This can lead to factual inaccuracies (hallucinations) when the LLM is prompted to generate text based on information they didn’t see during their training. Therefore, it’s crucial to bridge the gap between the LLM’s general knowledge and your proprietary data to help the model generate more accurate and contextual responses while reducing the risk of hallucinations. The traditional method of fine-tuning, although effective, can be compute-intensive, expensive, and requires technical expertise. Another option to consider is called Retrieval Augmented Generation (RAG), which provides LLMs with additional information from an external knowledge source that can be updated easily.
Additionally, enterprises must ensure data security when handling proprietary and sensitive data, such as personal data or intellectual property. This is particularly important for organizations operating in heavily regulated industries, such as financial services and healthcare and life sciences. Therefore, it’s important to understand and control the flow of your data through the generative AI application: Where is the model located? Where is the data processed? Who has access to the data? Will the data be used to train models, eventually risking the leak of sensitive data to public LLMs?
This post discusses how enterprises can build accurate, transparent, and secure generative AI applications while keeping full control over proprietary data. The proposed solution is a RAG pipeline using an AI-native technology stack, whose components are designed from the ground up with AI at their core, rather than having AI capabilities added as an afterthought. We demonstrate how to build an end-to-end RAG application using Cohere’s language models through Amazon Bedrock and a Weaviate vector database on AWS Marketplace. The accompanying source code is available in the related GitHub repository hosted by Weaviate. Although AWS will not be responsible for maintaining or updating the code in the partner’s repository, we encourage customers to connect with Weaviate directly regarding any desired updates.
Solution overview
The following high-level architecture diagram illustrates the proposed RAG pipeline with an AI-native technology stack for building accurate, transparent, and secure generative AI solutions.
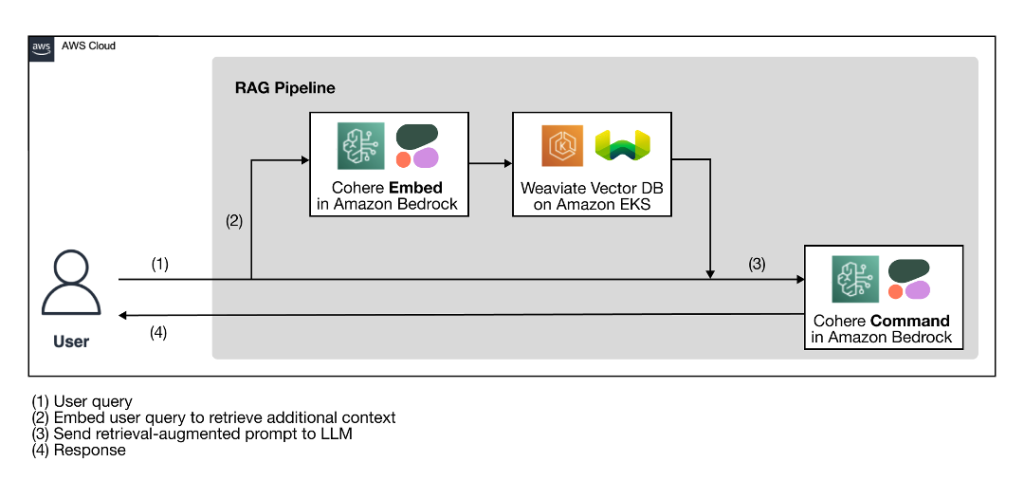
Figure 1: RAG workflow using Cohere’s language models through Amazon Bedrock and a Weaviate vector database on AWS Marketplace
As a preparation step for the RAG workflow, a vector database, which serves as the external knowledge source, is ingested with the additional context from the proprietary data. The actual RAG workflow follows the four steps illustrated in the diagram:
- The user enters their query.
- The user query is used to retrieve relevant additional context from the vector database. This is done by generating the vector embeddings of the user query with an embedding model to perform a vector search to retrieve the most relevant context from the database.
- The retrieved context and the user query are used to augment a prompt template. The retrieval-augmented prompt helps the LLM generate a more relevant and accurate completion, minimizing hallucinations.
- The user receives a more accurate response based on their query.
The AI-native technology stack illustrated in the architecture diagram has two key components: Cohere language models and a Weaviate vector database.
Cohere language models in Amazon Bedrock
The Cohere Platform brings language models with state-of-the-art performance to enterprises and developers through a simple API call. There are two key types of language processing capabilities that the Cohere Platform provides—generative and embedding—and each is served by a different type of model:
- Text generation with Command – Developers can access endpoints that power generative AI capabilities, enabling applications such as conversational, question answering, copywriting, summarization, information extraction, and more.
- Text representation with Embed – Developers can access endpoints that capture the semantic meaning of text, enabling applications such as vector search engines, text classification and clustering, and more. Cohere Embed comes in two forms, an English language model and a multilingual model, both of which are now available on Amazon Bedrock.
The Cohere Platform empowers enterprises to customize their generative AI solution privately and securely through the Amazon Bedrock deployment. Amazon Bedrock is a fully managed cloud service that enables development teams to build and scale generative AI applications quickly while helping keep your data and applications secure and private. Your data is not used for service improvements, is never shared with third-party model providers, and remains in the Region where the API call is processed. The data is always encrypted in transit and at rest, and you can encrypt the data using your own keys. Amazon Bedrock supports security requirements, including U.S. Health Insurance Portability and Accountability Act (HIPAA) eligibility and General Data Protection Regulation (GDPR) compliance. Additionally, you can securely integrate and easily deploy your generative AI applications using the AWS tools you are already familiar with.
Weaviate vector database on AWS Marketplace
Weaviate is an AI-native vector database that makes it straightforward for development teams to build secure and transparent generative AI applications. Weaviate is used to store and search both vector data and source objects, which simplifies development by eliminating the need to host and integrate separate databases. Weaviate delivers subsecond semantic search performance and can scale to handle billions of vectors and millions of tenants. With a uniquely extensible architecture, Weaviate integrates natively with Cohere foundation models deployed in Amazon Bedrock to facilitate the convenient vectorization of data and use its generative capabilities from within the database.
The Weaviate AI-native vector database gives customers the flexibility to deploy it as a bring-your-own-cloud (BYOC) solution or as a managed service. This showcase uses the Weaviate Kubernetes Cluster on AWS Marketplace, part of Weaviate’s BYOC offering, which allows container-based scalable deployment inside your AWS tenant and VPC with just a few clicks using an AWS CloudFormation template. This approach ensures that your vector database is deployed in your specific Region close to the foundation models and proprietary data to minimize latency, support data locality, and protect sensitive data while addressing potential regulatory requirements, such as GDPR.
Use case overview
In the following sections, we demonstrate how to build a RAG solution using the AI-native technology stack with Cohere, AWS, and Weaviate, as illustrated in the solution overview.
The example use case generates targeted advertisements for vacation stay listings based on a target audience. The goal is to use the user query for the target audience (for example, “family with small children”) to retrieve the most relevant vacation stay listing (for example, a listing with playgrounds close by) and then to generate an advertisement for the retrieved listing tailored to the target audience.

Figure 2: First few rows of vacation stay listings available from Inside Airbnb.
The dataset is available from Inside Airbnb and is licensed under a Creative Commons Attribution 4.0 International License. You can find the accompanying code in the GitHub repository.
Prerequisites
To follow along and use any AWS services in the following tutorial, make sure you have an AWS account.
Enable components of the AI-native technology stack
First, you need to enable the relevant components discussed in the solution overview in your AWS account. Complete the following steps:
- In the left Amazon Bedrock console, choose Model access in the navigation pane.
- Choose Manage model access on the top right.
- Select the foundation models of your choice and request access.
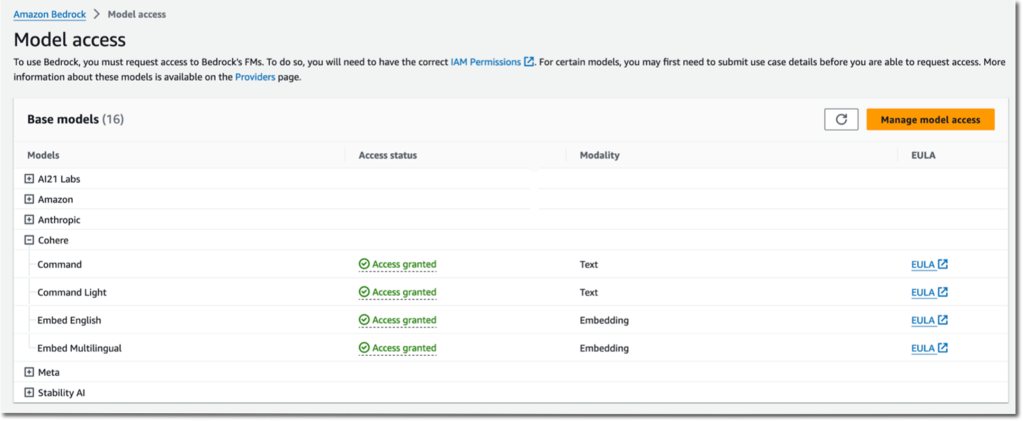
Figure 3: Manage model access in Amazon Bedrock console.
Next, you set up a Weaviate cluster.
- Subscribe to the Weaviate Kubernetes Cluster on AWS Marketplace.
- Launch the software using a CloudFormation template according to your preferred Availability Zone.
The CloudFormation template is pre-populated with default values.
- For Stack name, enter a stack name.
- For helmauthenticationtype, it is recommended to enable authentication by setting
helmauthenticationtype
toapikey
and defining a helmauthenticationapikey. - For helmauthenticationapikey, enter your Weaviate API key.
- For helmchartversion, enter your version number. It must be at least v.16.8.0. Refer to the GitHub repo for the latest version.
- For helmenabledmodules, make sure
tex2vec-aws
andgenerative-aws
are present in the list of enabled modules within Weaviate.
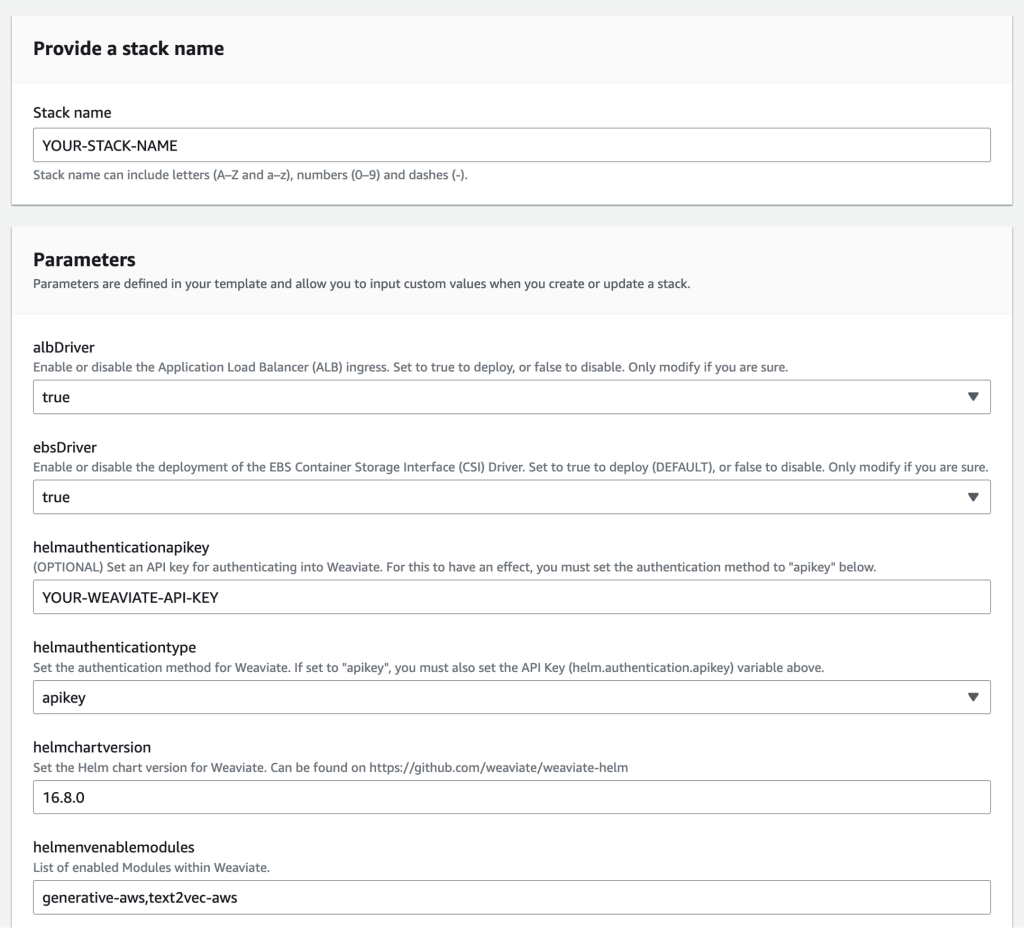
Figure 4: CloudFormation template.
This template takes about 30 minutes to complete.
Connect to Weaviate
Complete the following steps to connect to Weaviate:
- In the Amazon SageMaker console, navigate to Notebook instances in the navigation pane via Notebook > Notebook instances on the left.
- Create a new notebook instance.
- Install the Weaviate client package with the required dependencies:
- Connect to your Weaviate instance with the following code:
- Weaviate URL – Access Weaviate via the load balancer URL. In the Amazon Elastic Compute Cloud (Amazon EC2) console, choose Load balancers in the navigation pane and find the load balancer. Look for the DNS name column and add
http://
in front of it. - Weaviate API key – This is the key you set earlier in the CloudFormation template (
helmauthenticationapikey
). - AWS access key and secret access key – You can retrieve the access key and secret access key for your user in the AWS Identity and Access Management (IAM) console.
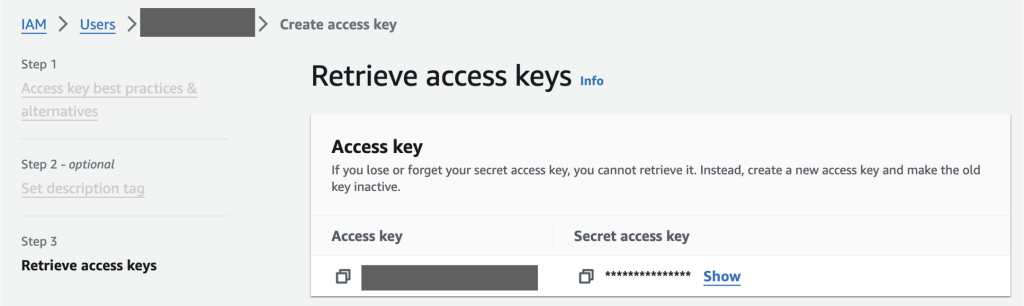
Figure 5: AWS Identity and Access Management (IAM) console to retrieve AWS access key and secret access key.
Configure the Amazon Bedrock module to enable Cohere models
Next, you define a data collection (class
) called Listings
to store the listings’ data objects, which is analogous to creating a table in a relational database. In this step, you configure the relevant modules to enable the usage of Cohere language models hosted on Amazon Bedrock natively from within the Weaviate vector database. The vectorizer (“text2vec-aws
“) and generative module (“generative-aws
“) are specified in the data collection definition. Both of these modules take three parameters:
- “service” – Use “
bedrock
” for Amazon Bedrock (alternatively, use “sagemaker
” for Amazon SageMaker JumpStart) - “Region” – Enter the Region where your model is deployed
- “model” – Provide the foundation model’s name
See the following code:
Ingest data into the Weaviate vector database
In this step, you define the structure of the data collection by configuring its properties. Aside from the property’s name and data type, you can also configure if only the data object will be stored or if it will be stored together with its vector embeddings. In this example, host_name
and property_type
are not vectorized:
Run the following code to create the collection in your Weaviate instance:
You can now add objects to Weaviate. You use a batch import process for maximum efficiency. Run the following code to import data. During the import, Weaviate will use the defined vectorizer to create a vector embedding for each object. The following code loads objects, initializes a batch process, and adds objects to the target collection one by one:
Retrieval Augmented Generation
You can build a RAG pipeline by implementing a generative search query on your Weaviate instance. For this, you first define a prompt template in the form of an f-string that can take in the user query ({target_audience}
) directly and the additional context ({{host_name}}
, {{property_type}}
, {{description}}
, and {{neighborhood_overview}}
) from the vector database at runtime:
Next, you run a generative search query. This prompts the defined generative model with a prompt that is comprised of the user query as well as the retrieved data. The following query retrieves one listing object (.with_limit(1)
) from the Listings
collection that is most similar to the user query (.with_near_text({"concepts": target_audience})
). Then the user query (target_audience
) and the retrieved listings properties (["description", "neighborhood", "host_name", "property_type"]
) are fed into the prompt template. See the following code:
In the following example, you can see that the preceding piece of code for target_audience = “Family with small children”
retrieves a listing from the host Marre. The prompt template is augmented with Marre’s listing details and the target audience:
Based on the retrieval-augmented prompt, Cohere’s Command model generates the following targeted advertisement:
Alternative customizations
You can make alternative customizations to different components in the proposed solution, such as the following:
- Cohere’s language models are also available through Amazon SageMaker JumpStart, which provides access to cutting-edge foundation models and enables developers to deploy LLMs to Amazon SageMaker, a fully managed service that brings together a broad set of tools to enable high-performance, low-cost machine learning for any use case. Weaviate is integrated with SageMaker as well.
- A powerful addition to this solution is the Cohere Rerank endpoint, available through SageMaker JumpStart. Rerank can improve the relevance of search results from lexical or semantic search. Rerank works by computing semantic relevance scores for documents that are retrieved by a search system and ranking the documents based on these scores. Adding Rerank to an application requires only a single line of code change.
- To cater to different deployment requirements of different production environments, Weaviate can be deployed in various additional ways. For example, it is available as a direct download from Weaviate website, which runs on Amazon Elastic Kubernetes Service (Amazon EKS) or locally via Docker or Kubernetes. It’s also available as a managed service that can run securely within a VPC or as a public cloud service hosted on AWS with a 14-day free trial.
- You can serve your solution in a VPC using Amazon Virtual Private Cloud (Amazon VPC), which enables organizations to launch AWS services in a logically isolated virtual network, resembling a traditional network but with the benefits of AWS’s scalable infrastructure. Depending on the classified level of sensitivity of the data, organizations can also disable internet access in these VPCs.
Clean up
To prevent unexpected charges, delete all the resources you deployed as part of this post. If you launched the CloudFormation stack, you can delete it via the AWS CloudFormation console. Note that there may be some AWS resources, such as Amazon Elastic Block Store (Amazon EBS) volumes and AWS Key Management Service (AWS KMS) keys, that may not be deleted automatically when the CloudFormation stack is deleted.

Figure 6: Delete all resources via the AWS CloudFormation console.
Conclusion
This post discussed how enterprises can build accurate, transparent, and secure generative AI applications while still having full control over their data. The proposed solution is a RAG pipeline using an AI-native technology stack as a combination of Cohere foundation models in Amazon Bedrock and a Weaviate vector database on AWS Marketplace. The RAG approach enables enterprises to bridge the gap between the LLM’s general knowledge and the proprietary data while minimizing hallucinations. An AI-native technology stack enables fast development and scalable performance.
You can start experimenting with RAG proofs of concept for your enterprise-ready generative AI applications using the steps outlined in this post. The accompanying source code is available in the related GitHub repository. Thank you for reading. Feel free to provide comments or feedback in the comments section.
About the authors
James Yi is a Senior AI/ML Partner Solutions Architect in the Technology Partners COE Tech team at Amazon Web Services. He is passionate about working with enterprise customers and partners to design, deploy, and scale AI/ML applications to derive business value. Outside of work, he enjoys playing soccer, traveling, and spending time with his family.
Leonie Monigatti is a Developer Advocate at Weaviate. Her focus area is AI/ML, and she helps developers learn about generative AI. Outside of work, she also shares her learnings in data science and ML on her blog and on Kaggle.
Meor Amer is a Developer Advocate at Cohere, a provider of cutting-edge natural language processing (NLP) technology. He helps developers build cutting-edge applications with Cohere’s Large Language Models (LLMs).
Shun Mao is a Senior AI/ML Partner Solutions Architect in the Emerging Technologies team at Amazon Web Services. He is passionate about working with enterprise customers and partners to design, deploy and scale AI/ML applications to derive their business values. Outside of work, he enjoys fishing, traveling and playing Ping-Pong.
Build a vaccination verification solution using the Queries feature in Amazon Textract
Amazon Textract is a machine learning (ML) service that enables automatic extraction of text, handwriting, and data from scanned documents, surpassing traditional optical character recognition (OCR). It can identify, understand, and extract data from tables and forms with remarkable accuracy. Presently, several companies rely on manual extraction methods or basic OCR software, which is tedious and time-consuming, and requires manual configuration that needs updating when the form changes. Amazon Textract helps solve these challenges by utilizing ML to automatically process different document types and accurately extract information with minimal manual intervention. This enables you to automate document processing and use the extracted data for different purposes, such as automating loans processing or gathering information from invoices and receipts.
As travel resumes post-pandemic, verifying a traveler’s vaccination status may be required in many cases. Hotels and travel agencies often need to review vaccination cards to gather important details like whether the traveler is fully vaccinated, vaccine dates, and the traveler’s name. Some agencies do this through manual verification of cards, which can be time-consuming for staff and leaves room for human error. Others have built custom solutions, but these can be costly and difficult to scale, and take significant time to implement. Moving forward, there may be opportunities to streamline the vaccination status verification process in a way that is efficient for businesses while respecting travelers’ privacy and convenience.
Amazon Textract Queries helps address these challenges. Amazon Textract Queries allows you to specify and extract only the piece of information that you need from the document. It gives you precise and accurate information from the document.
In this post, we walk you through a step-by-step implementation guide to build a vaccination status verification solution using Amazon Textract Queries. The solution showcases how to process vaccination cards using an Amazon Textract query, verify the vaccination status, and store the information for future use.
Solution overview
The following diagram illustrates the solution architecture.
The workflow includes the following steps:
- The user takes a photo of a vaccination card.
- The image is uploaded to an Amazon Simple Storage Service (Amazon S3) bucket.
- When the image gets saved in the S3 bucket, it invokes an AWS Step Functions workflow:
- The Queries-Decider AWS Lambda function examines the document passed in and adds information about the mime type, the number of pages, and the number of queries to the Step Functions workflow (for our example, we have four queries).
NumberQueriesAndPagesChoice
is a Choice state that adds conditional logic to a workflow. If there are between 15–31 queries and the number of pages is between 2–3,001, then Amazon Textract asynchronous processing is the only option, because synchronous APIs only support up to 15 queries and one-page documents. For all other cases, we route to the random selection of synchronous or asynchronous processing.- The
TextractSync
Lambda function sends a request to Amazon Textract to analyze the document based on the following Amazon Textract queries:- What is Vaccination Status?
- What is Name?
- What is Date of Birth?
- What is Document Number?
- Amazon Textract analyzes the image and sends the answers of these queries back to the Lambda function.
- The Lambda function verifies the customer’s vaccination status and stores the final result in CSV format in the same S3 bucket (
demoqueries-textractxxx
) in thecsv-output
folder.
Prerequisites
To complete this solution, you should have an AWS account and the appropriate permissions to create the resources required as part of the solution.
Download the deployment code and sample vaccination card from GitHub.
Use the Queries feature on the Amazon Textract console
Before you build the vaccination verification solution, let’s explore how you can use Amazon Textract Queries to extract vaccination status via the Amazon Textract console. You can use the vaccination card sample you downloaded from the GitHub repo.
- On the Amazon Textract console, choose Analyze Document in the navigation pane.
- Under Upload document, choose Choose document to upload the vaccination card from your local drive.
- After you upload the document, select Queries in the Configure Document section.
- You can then add queries in the form of natural language questions. Let’s add the following:
- What is Vaccination Status?
- What is Name?
- What is Date of Birth?
- What is Document Number?
- After you add all your queries, choose Apply configuration.
- Check the Queries tab to see the answers to the questions.
You can see Amazon Textract extracts the answer to your query from the document.
Deploy the vaccination verification solution
In this post, we use an AWS Cloud9 instance and install the necessary dependencies on the instance with the AWS Cloud Development Kit (AWS CDK) and Docker. AWS Cloud9 is a cloud-based integrated development environment (IDE) that lets you write, run, and debug your code with just a browser.
- In the terminal, choose Upload Local Files on the File menu.
- Choose Select folder and choose the
vaccination_verification_solution
folder you downloaded from GitHub. - In the terminal, prepare your serverless application for subsequent steps in your development workflow in AWS Serverless Application Model (AWS SAM) using the following command:
- Deploy the application using the
cdk deploy
command:Wait for the AWS CDK to deploy the model and create the resources mentioned in the template.
- When deployment is complete, you can check the deployed resources on the AWS CloudFormation console on the Resources tab of the stack details page.
Test the solution
Now it’s time to test the solution. To trigger the workflow, use aws s3 cp
to upload the vac_card.jpg
file to DemoQueries.DocumentUploadLocation
inside the docs folder:
The vaccination certificate file automatically gets uploaded to the S3 bucket demoqueries-textractxxx
in the uploads folder.
The Step Functions workflow is triggered via a Lambda function as soon as the vaccination certificate file is uploaded to the S3 bucket.
The Queries-Decider Lambda function examines the document and adds information about the mime type, the number of pages, and the number of queries to the Step Functions workflow (for this example, we use four queries—document number, customer name, date of birth, and vaccination status).
The TextractSync
function sends the input queries to Amazon Textract and synchronously returns the full result as part of the response. It supports 1-page documents (TIFF, PDF, JPG, PNG) and up to 15 queries. The GenerateCsvTask
function takes the JSON output from Amazon Textract and converts it to a CSV file.
The final output is stored in the same S3 bucket in the csv-output folder as a CSV file.
You can download the file to your local machine using the following command:
The format of the result is timestamp
, classification
, filename
, page number
, key name
, key_confidence
, value
, value_confidence
, key_bb_top
, key_bb_height
, key_bb.width
, key_bb_left
, value_bb_top
, value_bb_height
, value_bb_width
, value_bb_left
.
You can scale the solution to hundreds of vaccination certificate documents for multiple customers by uploading their vaccination certificates to DemoQueries.DocumentUploadLocation
. This automatically triggers multiple runs of the Step Functions state machine, and the final result is stored in the same S3 bucket in the csv-output folder.
To change the initial set of queries that are fed into Amazon Textract, you can go to your AWS Cloud9 instance and open the start_execution.py file. In the file view in the left pane, navigate to lambda, start_queries
, app
, start_execution.py
. This Lambda function is invoked when a file is uploaded to DemoQueries.DocumentUploadLocation
. The queries sent to the workflow are defined in start_execution.py
; you can change those by updating the code as shown in the following screenshot.
Clean up
To avoid incurring ongoing charges, delete the resources created in this post using the following command:
Answer the question Are you sure you want to delete: DemoQueries (y/n)?
with y.
Conclusion
In this post, we showed you how to use Amazon Textract Queries to build a vaccination verification solution for the travel industry. You can use Amazon Textract Queries to build solutions in other industries like finance and healthcare, and retrieve information from documents such as paystubs, mortgage notes, and insurance cards based on natural language questions.
For more information, see Analyzing Documents, or check out the Amazon Textract console and try out this feature.
About the Authors
Dhiraj Thakur is a Solutions Architect with Amazon Web Services. He works with AWS customers and partners to provide guidance on enterprise cloud adoption, migration, and strategy. He is passionate about technology and enjoys building and experimenting in the analytics and AI/ML space.
Rishabh Yadav is a Partner Solutions architect at AWS with an extensive background in DevOps and Security offerings at AWS. He works with ASEAN partners to provide guidance on enterprise cloud adoption and architecture reviews along with building AWS practices through the implementation of the Well-Architected Framework. Outside of work, he likes to spend his time in the sports field and FPS gaming.
Vision-language models that can handle multi-image inputs
Attention-based representation of multi-image inputs improves performance on downstream vision-language tasks.Read More
Reduce inference time for BERT models using neural architecture search and SageMaker Automated Model Tuning
In this post, we demonstrate how to use neural architecture search (NAS) based structural pruning to compress a fine-tuned BERT model to improve model performance and reduce inference times. Pre-trained language models (PLMs) are undergoing rapid commercial and enterprise adoption in the areas of productivity tools, customer service, search and recommendations, business process automation, and content creation. Deploying PLM inference endpoints is typically associated with higher latency and higher infrastructure costs due to the compute requirements and reduced computational efficiency due to the large number of parameters. Pruning a PLM reduces the size and complexity of the model while retaining its predictive capabilities. Pruned PLMs achieve a smaller memory footprint and lower latency. We demonstrate that by pruning a PLM and trading off parameter count and validation error for a specific target task, and are able to achieve faster response times when compared to the base PLM model.
Multi-objective optimization is an area of decision-making that optimizes more than one objective function, such as memory consumption, training time, and compute resources, to be optimized simultaneously. Structural pruning is a technique to reduce the size and computational requirements of PLM by pruning layers or neurons/nodes while attempting to preserve model accuracy. By removing layers, structural pruning achieves higher compression rates, which leads to hardware-friendly structured sparsity that reduces runtimes and response times. Applying a structural pruning technique to a PLM model results in a lighter-weight model with a lower memory footprint that, when hosted as an inference endpoint in SageMaker, offers improved resource efficiency and reduced cost when compared to the original fine-tuned PLM.
The concepts illustrated in this post can be applied to applications that use PLM features, such as recommendation systems, sentiment analysis, and search engines. Specifically, you can use this approach if you have dedicated machine learning (ML) and data science teams who fine-tune their own PLM models using domain-specific datasets and deploy a large number of inference endpoints using Amazon SageMaker. One example is an online retailer who deploys a large number of inference endpoints for text summarization, product catalog classification, and product feedback sentiment classification. Another example might be a healthcare provider who uses PLM inference endpoints for clinical document classification, named entity recognition from medical reports, medical chatbots, and patient risk stratification.
Solution overview
In this section, we present the overall workflow and explain the approach. First, we use an Amazon SageMaker Studio notebook to fine-tune a pre-trained BERT model on a target task using a domain-specific dataset. BERT (Bidirectional Encoder Representations from Transformers) is a pre-trained language model based on the transformer architecture used for natural language processing (NLP) tasks. Neural architecture search (NAS) is an approach for automating the design of artificial neural networks and is closely related to hyperparameter optimization, a widely used approach in the field of machine learning. The goal of NAS is to find the optimal architecture for a given problem by searching over a large set of candidate architectures using techniques such as gradient-free optimization or by optimizing the desired metrics. The performance of the architecture is typically measured using metrics such as validation loss. SageMaker Automatic Model Tuning (AMT) automates the tedious and complex process of finding the optimal combinations of hyperparameters of the ML model that yield the best model performance. AMT uses intelligent search algorithms and iterative evaluations using a range of hyperparameters that you specify. It chooses the hyperparameter values that creates a model that performs the best, as measured by performance metrics such as accuracy and F-1 score.
The fine-tuning approach described in this post is generic and can be applied to any text-based dataset. The task assigned to the BERT PLM can be a text-based task such as sentiment analysis, text classification, or Q&A. In this demo, the target task is a binary classification problem where BERT is used to identify, from a dataset that consists of a collection of pairs of text fragments, whether the meaning of one text fragment can be inferred from the other fragment. We use the Recognizing Textual Entailment dataset from the GLUE benchmarking suite. We perform a multi-objective search using SageMaker AMT to identify the sub-networks that offer optimal trade-offs between parameter count and prediction accuracy for the target task. When performing a multi-objective search, we start with defining the accuracy and parameter count as the objectives that we are aiming to optimize.
Within the BERT PLM network, there can be modular, self-contained sub-networks that allow the model to have specialized capabilities such as language understanding and knowledge representation. BERT PLM uses a multi-headed self-attention sub-network and a feed-forward sub-network. A multi-headed, self-attention layer allows BERT to relate different positions of a single sequence in order to compute a representation of the sequence by allowing multiple heads to attend to multiple context signals. The input is split into multiple subspaces and self-attention is applied to each of the subspaces separately. Multiple heads in a transformer PLM allow the model to jointly attend to information from different representation subspaces. A feed-forward sub-network is a simple neural network that takes the output from the multi-headed self-attention sub-network, processes the data, and returns the final encoder representations.
The goal of random sub-network sampling is to train smaller BERT models that can perform well enough on target tasks. We sample 100 random sub-networks from the fine-tuned base BERT model and evaluate 10 networks simultaneously. The trained sub-networks are evaluated for the objective metrics and the final model is chosen based on the trade-offs found between the objective metrics. We visualize the Pareto front for the sampled sub-networks, which contains the pruned model that offers the optimal trade-off between model accuracy and model size. We select the candidate sub-network (NAS-pruned BERT model) based on the model size and model accuracy that we are willing to trade off. Next, we host the endpoints, the pre-trained BERT base model, and the NAS-pruned BERT model using SageMaker. To perform load testing, we use Locust, an open source load testing tool that you can implement using Python. We run load testing on both endpoints using Locust and visualize the results using the Pareto front to illustrate the trade-off between response times and accuracy for both models. The following diagram provides an overview of the workflow explained in this post.
Prerequisites
For this post, the following prerequisites are required:
- An AWS account with access to the AWS Management Console
- A SageMaker domain, SageMaker user profile, and SageMaker Studio
- An IAM execution role for the SageMaker Studio Domain user
You also need to increase the service quota to access at least three instances of ml.g4dn.xlarge instances in SageMaker. The instance type ml.g4dn.xlarge is the cost efficient GPU instance that allows you to run PyTorch natively. To increase the service quota, complete the following steps:
- On the console, navigate to Service Quotas.
- For Manage quotas, choose Amazon SageMaker, then choose View quotas.
- Search for “ml-g4dn.xlarge for training job usage” and select the quota item.
- Choose Request increase at account-level.
- For Increase quota value, enter a value of 5 or higher.
- Choose Request.
The requested quota approval may take some time to complete depending on the account permissions.
- Open SageMaker Studio from the SageMaker console.
- Choose System terminal under Utilities and files.
- Run the following command to clone the GitHub repo to the SageMaker Studio instance:
- Navigate to
amazon-sagemaker-examples/hyperparameter_tuning/neural_architecture_search_llm
. - Open the file
nas_for_llm_with_amt.ipynb
. - Set up the environment with an
ml.g4dn.xlarge
instance and choose Select.
Set up the pre-trained BERT model
In this section, we import the Recognizing Textual Entailment dataset from the dataset library and split the dataset into training and validation sets. This dataset consists of pairs of sentences. The task of the BERT PLM is to recognize, given two text fragments, whether the meaning of one text fragment can be inferred from the other fragment. In the following example, we can infer the meaning of the first phrase from the second phrase:
We load the textual recognizing entailment dataset from the GLUE benchmarking suite via the dataset library from Hugging Face within our training script (./training.py
). We split the original training dataset from GLUE into a training and validation set. In our approach, we fine-tune the base BERT model using the training dataset, then we perform a multi-objective search to identify the set of sub-networks that optimally balance between the objective metrics. We use the training dataset exclusively for fine-tuning the BERT model. However, we use validation data for the multi-objective search by measuring accuracy on the holdout validation dataset.
Fine-tune the BERT PLM using a domain-specific dataset
The typical use cases for a raw BERT model include next sentence prediction or masked language modeling. To use the base BERT model for downstream tasks such as textual recognizing entailment, we have to further fine-tune the model using a domain-specific dataset. You can use a fine-tuned BERT model for tasks such as sequence classification, question answering, and token classification. However, for the purposes of this demo, we use the fine-tuned model for binary classification. We fine-tune the pre-trained BERT model with the training dataset that we prepared previously, using the following hyperparameters:
We save the checkpoint of the model training to an Amazon Simple Storage Service (Amazon S3) bucket, so that the model can be loaded during the NAS-based multi-objective search. Before we train the model, we define the metrics such as epoch, training loss, number of parameters, and validation error:
After the fine-tuning process starts, the training job takes around 15 minutes to complete.
Perform a multi-objective search to select sub-networks and visualize the results
In the next step, we perform a multi-objective search on the fine-tuned base BERT model by sampling random sub-networks using SageMaker AMT. To access a sub-network within the super-network (the fine-tuned BERT model), we mask out all the components of the PLM that are not part of the sub-network. Masking a super-network to find sub-networks in a PLM is a technique used to isolate and identify patterns of the model’s behavior. Note that Hugging Face transformers needs the hidden size to be a multiple of the number of heads. The hidden size in a transformer PLM controls the size of the hidden state vector space, which impacts the model’s ability to learn complex representations and patterns in the data. In a BERT PLM, the hidden state vector is of a fixed size (768). We can’t change the hidden size, and therefore the number of heads has to be in [1, 3, 6, 12].
In contrast to single-objective optimization, in the multi-objective setting, we typically don’t have a single solution that simultaneously optimizes all objectives. Instead, we aim to collect a set of solutions that dominate all other solutions in at least one objective (such as validation error). Now we can start the multi-objective search through AMT by setting the metrics that we want to reduce (validation error and number of parameters). The random sub-networks are defined by the parameter max_jobs
and the number of simultaneous jobs is defined by the parameter max_parallel_jobs
. The code to load the model checkpoint and evaluate the sub-network is available in the evaluate_subnetwork.py
script.
The AMT tuning job takes approximately 2 hours and 20 minutes to run. After the AMT tuning job runs successfully, we parse the job’s history and collect the sub-network’s configurations, such as number of heads, number of layers, number of units, and the corresponding metrics such as validation error and number of parameters. The following screenshot shows the summary of a successful AMT tuner job.
Next, we visualize the results using a Pareto set (also known as Pareto frontier or Pareto optimal set), which helps us identify optimal sets of sub-networks that dominate all other sub-networks in the objective metric (validation error):
First, we collect the data from the AMT tuning job. Then then we plot the Pareto set using matplotlob.pyplot
with number of parameters in the x axis and validation error in the y axis. This implies that when we move from one sub-network of the Pareto set to another, we must either sacrifice performance or model size but improve the other. Ultimately, the Pareto set provides us the flexibility to choose the sub-network that best suits our preferences. We can decide how much we want to reduce the size of our network and how much performance we are willing to sacrifice.
Deploy the fine-tuned BERT model and the NAS-optimized sub-network model using SageMaker
Next, we deploy the largest model in our Pareto set that leads to the smallest amount of performance degeneration to a SageMaker endpoint. The best model is the one that provides an optimal trade-off between the validation error and the number of parameters for our use case.
Model comparison
We took a pre-trained base BERT model, fine-tuned it using a domain-specific dataset, ran a NAS search to identify dominant sub-networks based on the objective metrics, and deployed the pruned model on a SageMaker endpoint. In addition, we took the pre-trained base BERT model and deployed the base model on a second SageMaker endpoint. Next, we ran load-testing using Locust on both inference endpoints and evaluated the performance in terms of response time.
First, we import the necessary Locust and Boto3 libraries. Then we construct a request metadata and record the start time to be used for load testing. Then the payload is passed to the SageMaker endpoint invoke API via the BotoClient to simulate real user requests. We use Locust to spawn multiple virtual users to send requests in parallel and measure the endpoint performance under the load. Tests are run by increasing the number of users for each of the two endpoints, respectively. After the tests are completed, Locust outputs a request statistics CSV file for each of the deployed models.
Next, we generate the response time plots from the CSV files downloaded after running the tests with Locust. The purpose of plotting the response time vs. the number of users is to analyze the load testing results by visualizing the impact of the response time of the model endpoints. In the following chart, we can see that the NAS-pruned model endpoint achieves a lower response time compared to the base BERT model endpoint.
In the second chart, which is an extension of the first chart, we observe that after around 70 users, SageMaker starts to throttle the base BERT model endpoint and throws an exception. However, for the NAS-pruned model endpoint, the throttling happens between 90–100 users and with a lower response time.
From the two charts, we observe that the pruned model has a faster response time and scales better when compared to the unpruned model. As we scale the number of inference endpoints, as is the case with users who deploy a large number of inference endpoints for their PLM applications, the cost benefits and performance improvement start to become quite substantial.
Clean up
To delete the SageMaker endpoints for the fine-tuned base BERT model and the NAS-pruned model, complete the following steps:
- On the SageMaker console, choose Inference and Endpoints in the navigation pane.
- Select the endpoint and delete it.
Alternatively, from the SageMaker Studio notebook, run the following commands by providing the endpoint names:
Conclusion
In this post, we discussed how to use NAS to prune a fine-tuned BERT model. We first trained a base BERT model using domain-specific data and deployed it to a SageMaker endpoint. We performed a multi-objective search on the fine-tuned base BERT model using SageMaker AMT for a target task. We visualized the Pareto front and selected the Pareto optimal NAS-pruned BERT model and deployed the model to a second SageMaker endpoint. We performed load testing using Locust to simulate users querying both the endpoints, and measured and recorded the response times in a CSV file. We plotted the response time vs. the number of users for both the models.
We observed that the pruned BERT model performed significantly better in both response time and instance throttling threshold. We concluded that the NAS-pruned model was more resilient to an increased load on the endpoint, maintaining a lower response time even as more users stressed the system compared to the base BERT model. You can apply the NAS technique described in this post to any large language model to find a pruned model that can perform the target task with significantly lower response time. You can further optimize the approach by using latency as a parameter in addition to validation loss.
Although we use NAS in this post, quantization is another common approach used to optimize and compress PLM models. Quantization reduces the precision of the weights and activations in a trained network from 32-bit floating point to lower bit widths such as 8-bit or 16-bit integers, which results in a compressed model that generates faster inference. Quantization doesn’t reduce the number of parameters; instead it reduces the precision of the existing parameters to get a compressed model. NAS pruning removes redundant networks in a PLM, which creates a sparse model with fewer parameters. Typically, NAS pruning and quantization are used together to compress large PLMs to maintain model accuracy, reduce validation losses while improving performance, and reduce model size. The other commonly used techniques to reduce the size of PLMs include knowledge distillation, matrix factorization, and distillation cascades.
The approach proposed in the blogpost is suitable for teams that use SageMaker to train and fine-tune the models using domain-specific data and deploy the endpoints to generate inference. If you’re looking for a fully managed service that offers a choice of high-performing foundation models needed to build generative AI applications, consider using Amazon Bedrock. If you’re looking for pre-trained, open source models for a wide range of business use cases and want to access solution templates and example notebooks, consider using Amazon SageMaker JumpStart. A pre-trained version of the Hugging Face BERT base cased model that we used in this post is also available from SageMaker JumpStart.
About the Authors
Aparajithan Vaidyanathan is a Principal Enterprise Solutions Architect at AWS. He is a Cloud Architect with 24+ years of experience designing and developing enterprise, large-scale and distributed software systems. He specializes in Generative AI and Machine Learning Data Engineering. He is an aspiring marathon runner and his hobbies include hiking, bike riding and spending time with his wife and two boys.
Aaron Klein is a Sr Applied Scientist at AWS working on automated machine learning methods for deep neural networks.
Jacek Golebiowski is a Sr Applied Scientist at AWS.
Fine-tune and deploy Llama 2 models cost-effectively in Amazon SageMaker JumpStart with AWS Inferentia and AWS Trainium
Today, we’re excited to announce the availability of Llama 2 inference and fine-tuning support on AWS Trainium and AWS Inferentia instances in Amazon SageMaker JumpStart. Using AWS Trainium and Inferentia based instances, through SageMaker, can help users lower fine-tuning costs by up to 50%, and lower deployment costs by 4.7x, while lowering per token latency. Llama 2 is an auto-regressive generative text language model that uses an optimized transformer architecture. As a publicly available model, Llama 2 is designed for many NLP tasks such as text classification, sentiment analysis, language translation, language modeling, text generation, and dialogue systems. Fine-tuning and deploying LLMs, like Llama 2, can become costly or challenging to meet real time performance to deliver good customer experience. Trainium and AWS Inferentia, enabled by the AWS Neuron software development kit (SDK), offer a high-performance, and cost effective option for training and inference of Llama 2 models.
In this post, we demonstrate how to deploy and fine-tune Llama 2 on Trainium and AWS Inferentia instances in SageMaker JumpStart.
Solution overview
In this blog, we will walk through the following scenarios :
- Deploy Llama 2 on AWS Inferentia instances in both the Amazon SageMaker Studio UI, with a one-click deployment experience, and the SageMaker Python SDK.
- Fine-tune Llama 2 on Trainium instances in both the SageMaker Studio UI and the SageMaker Python SDK.
- Compare the performance of the fine-tuned Llama 2 model with that of pre-trained model to show the effectiveness of fine-tuning.
To get hands on, see the GitHub example notebook.
Deploy Llama 2 on AWS Inferentia instances using the SageMaker Studio UI and the Python SDK
In this section, we demonstrate how to deploy Llama 2 on AWS Inferentia instances using the SageMaker Studio UI for a one-click deployment and the Python SDK.
Discover the Llama 2 model on the SageMaker Studio UI
SageMaker JumpStart provides access to both publicly available and proprietary foundation models. Foundation models are onboarded and maintained from third-party and proprietary providers. As such, they are released under different licenses as designated by the model source. Be sure to review the license for any foundation model that you use. You are responsible for reviewing and complying with any applicable license terms and making sure they are acceptable for your use case before downloading or using the content.
You can access the Llama 2 foundation models through SageMaker JumpStart in the SageMaker Studio UI and the SageMaker Python SDK. In this section, we go over how to discover the models in SageMaker Studio.
SageMaker Studio is an integrated development environment (IDE) that provides a single web-based visual interface where you can access purpose-built tools to perform all machine learning (ML) development steps, from preparing data to building, training, and deploying your ML models. For more details on how to get started and set up SageMaker Studio, refer to Amazon SageMaker Studio.
After you’re in SageMaker Studio, you can access SageMaker JumpStart, which contains pre-trained models, notebooks, and prebuilt solutions, under Prebuilt and automated solutions. For more detailed information on how to access proprietary models, refer to Use proprietary foundation models from Amazon SageMaker JumpStart in Amazon SageMaker Studio.
From the SageMaker JumpStart landing page, you can browse for solutions, models, notebooks, and other resources.
If you don’t see the Llama 2 models, update your SageMaker Studio version by shutting down and restarting. For more information about version updates, refer to Shut down and Update Studio Classic Apps.
You can also find other model variants by choosing Explore All Text Generation Models or searching for llama
or neuron
in the search box. You will be able to view the Llama 2 Neuron models on this page.
Deploy the Llama-2-13b model with SageMaker Jumpstart
You can choose the model card to view details about the model such as license, data used to train, and how to use it. You can also find two buttons, Deploy and Open notebook, which help you use the model using this no-code example.
When you choose either button, a pop-up will show the End User License Agreement and Acceptable Use Policy (AUP) for you to acknowledge.
After you acknowledge the policies, you can deploy the endpoint of the model and use it via the steps in the next section.
Deploy the Llama 2 Neuron model via the Python SDK
When you choose Deploy and acknowledge the terms, model deployment will start. Alternatively, you can deploy through the example notebook by choosing Open notebook. The example notebook provides end-to-end guidance on how to deploy the model for inference and clean up resources.
To deploy or fine-tune a model on Trainium or AWS Inferentia instances, you first need to call PyTorch Neuron (torch-neuronx) to compile the model into a Neuron-specific graph, which will optimize it for Inferentia’s NeuronCores. Users can instruct the compiler to optimize for lowest latency or highest throughput, depending on the objectives of the application. In JumpStart, we pre-compiled the Neuron graphs for a variety of configurations, to allow users to sip compilation steps, enabling faster fine-tuning and deploying models.
Note that the Neuron pre-compiled graph is created based on a specific version of the Neuron Compiler version.
There are two ways to deploy LIama 2 on AWS Inferentia-based instances. The first method utilizes the pre-built configuration, and allows you to deploy the model in just two lines of code. In the second, you have greater control over the configuration. Let’s start with the first method, with the pre-built configuration, and use the pre-trained Llama 2 13B Neuron Model, as an example. The following code shows how to deploy Llama 13B with just two lines:
To perform inference on these models, you need to specify the argument accept_eula
to be True
as part of the model.deploy()
call. Setting this argument to be true, acknowledges you have read and accepted the EULA of the model. The EULA can be found in the model card description or from the Meta website.
The default instance type for Llama 2 13B is ml.inf2.8xlarge. You can also try other supported models IDs:
meta-textgenerationneuron-llama-2-7b
meta-textgenerationneuron-llama-2-7b-f
(chat model)meta-textgenerationneuron-llama-2-13b-f
(chat model)
Alternatively, if you want have more control of the deployment configurations, such as context length, tensor parallel degree, and maximum rolling batch size, you can modify them via environmental variables, as demonstrated in this section. The underlying Deep Learning Container (DLC) of the deployment is the Large Model Inference (LMI) NeuronX DLC. The environmental variables are as follows:
- OPTION_N_POSITIONS – The maximum numbers of input and output tokens. For example, if you compile the model with
OPTION_N_POSITIONS
as 512, then you can use an input token of 128 (input prompt size) with a maximum output token of 384 (the total of the input and output tokens has to be 512). For the maximum output token, any value below 384 is fine, but you can’t go beyond it (for example, input 256 and output 512). - OPTION_TENSOR_PARALLEL_DEGREE – The number of NeuronCores to load the model in AWS Inferentia instances.
- OPTION_MAX_ROLLING_BATCH_SIZE – The maximum batch size for concurrent requests.
- OPTION_DTYPE – The date type to load the model.
The compilation of Neuron graph depends on the context length (OPTION_N_POSITIONS
), tensor parallel degree (OPTION_TENSOR_PARALLEL_DEGREE
), maximum batch size (OPTION_MAX_ROLLING_BATCH_SIZE
), and data type (OPTION_DTYPE
) to load the model. SageMaker JumpStart has pre-compiled Neuron graphs for a variety of configurations for the preceding parameters to avoid runtime compilation. The configurations of pre-compiled graphs are listed in the following table. As long as the environmental variables fall into one of the following categories, compilation of Neuron graphs will be skipped.
LIama-2 7B and LIama-2 7B Chat | ||||
Instance type | OPTION_N_POSITIONS | OPTION_MAX_ROLLING_BATCH_SIZE | OPTION_TENSOR_PARALLEL_DEGREE | OPTION_DTYPE |
ml.inf2.xlarge | 1024 | 1 | 2 | fp16 |
ml.inf2.8xlarge | 2048 | 1 | 2 | fp16 |
ml.inf2.24xlarge | 4096 | 4 | 4 | fp16 |
ml.inf2.24xlarge | 4096 | 4 | 8 | fp16 |
ml.inf2.24xlarge | 4096 | 4 | 12 | fp16 |
ml.inf2.48xlarge | 4096 | 4 | 4 | fp16 |
ml.inf2.48xlarge | 4096 | 4 | 8 | fp16 |
ml.inf2.48xlarge | 4096 | 4 | 12 | fp16 |
ml.inf2.48xlarge | 4096 | 4 | 24 | fp16 |
LIama-2 13B and LIama-2 13B Chat | ||||
ml.inf2.8xlarge | 1024 | 1 | 2 | fp16 |
ml.inf2.24xlarge | 2048 | 4 | 4 | fp16 |
ml.inf2.24xlarge | 4096 | 4 | 8 | fp16 |
ml.inf2.24xlarge | 4096 | 4 | 12 | fp16 |
ml.inf2.48xlarge | 2048 | 4 | 4 | fp16 |
ml.inf2.48xlarge | 4096 | 4 | 8 | fp16 |
ml.inf2.48xlarge | 4096 | 4 | 12 | fp16 |
ml.inf2.48xlarge | 4096 | 4 | 24 | fp16 |
The following is an example of deploying Llama 2 13B and setting all the available configurations.
Now that we have deployed the Llama-2-13b model, we can run inference with it by invoking the endpoint. The following code snippet demonstrates using the supported inference parameters to control text generation:
- max_length – The model generates text until the output length (which includes the input context length) reaches
max_length
. If specified, it must be a positive integer. - max_new_tokens – The model generates text until the output length (excluding the input context length) reaches
max_new_tokens
. If specified, it must be a positive integer. - num_beams – This indicates the number of beams used in the greedy search. If specified, it must be an integer greater than or equal to
num_return_sequences
. - no_repeat_ngram_size – The model ensures that a sequence of words of
no_repeat_ngram_size
is not repeated in the output sequence. If specified, it must be a positive integer greater than 1. - temperature – This controls the randomness in the output. A higher temperature results in an output sequence with low-probability words; a lower temperature results in an output sequence with high-probability words. If
temperature
equals 0, it results in greedy decoding. If specified, it must be a positive float. - early_stopping – If
True
, text generation is finished when all beam hypotheses reach the end of the sentence token. If specified, it must be Boolean. - do_sample – If
True
, the model samples the next word as per the likelihood. If specified, it must be Boolean. - top_k – In each step of text generation, the model samples from only the
top_k
most likely words. If specified, it must be a positive integer. - top_p – In each step of text generation, the model samples from the smallest possible set of words with a cumulative probability of
top_p
. If specified, it must be a float between 0–1. - stop – If specified, it must be a list of strings. Text generation stops if any one of the specified strings is generated.
The following code shows an example:
Output:
For more information on the parameters in the payload, refer to Detailed parameters.
You can also explore the implementation of the parameters in the notebook to add more information about the link of the notebook.
Fine-tune Llama 2 models on Trainium instances using the SageMaker Studio UI and SageMaker Python SDK
Generative AI foundation models have become a primary focus in ML and AI, however, their broad generalization can fall short in specific domains like healthcare or financial services, where unique datasets are involved. This limitation highlights the need to fine-tune these generative AI models with domain-specific data to enhance their performance in these specialized areas.
Now that we have deployed the pre-trained version of the Llama 2 model, let’s look at how we can fine-tune this to domain-specific data to increase the accuracy, improve the model in terms of prompt completions, and adapt the model to your specific business use case and data. You can fine-tune the models using either the SageMaker Studio UI or SageMaker Python SDK. We discuss both methods in this section.
Fine-tune the Llama-2-13b Neuron model with SageMaker Studio
In SageMaker Studio, navigate to the Llama-2-13b Neuron model. On the Deploy tab, you can point to the Amazon Simple Storage Service (Amazon S3) bucket containing the training and validation datasets for fine-tuning. In addition, you can configure deployment configuration, hyperparameters, and security settings for fine-tuning. Then choose Train to start the training job on a SageMaker ML instance.
To use Llama 2 models, you need to accept the EULA and AUP. It will show up when you when you choose Train. Choose I have read and accept EULA and AUP to start the fine-tuning job.
You can view the status of your training job for the fine-tuned model under on the SageMaker console by choosing Training jobs in the navigation pane.
You can either fine-tune your Llama 2 Neuron model using this no-code example, or fine-tune via the Python SDK, as demonstrated in the next section.
Fine-tune the Llama-2-13b Neuron model via the SageMaker Python SDK
You can fine-tune on the dataset with the domain adaptation format or the instruction-based fine-tuning format. The following are the instructions for how the training data should be formatted before being sent into fine-tuning:
- Input – A
train
directory containing either a JSON lines (.jsonl) or text (.txt) formatted file.- For the JSON lines (.jsonl) file, each line is a separate JSON object. Each JSON object should be structured as a key-value pair, where the key should be
text
, and the value is the content of one training example. - The number of files under the train directory should equal to 1.
- For the JSON lines (.jsonl) file, each line is a separate JSON object. Each JSON object should be structured as a key-value pair, where the key should be
- Output – A trained model that can be deployed for inference.
In this example, we use a subset of the Dolly dataset in an instruction tuning format. The Dolly dataset contains approximately 15,000 instruction-following records for various categories, such as, question answering, summarization, and information extraction. It is available under the Apache 2.0 license. We use the information_extraction
examples for fine-tuning.
- Load the Dolly dataset and split it into
train
(for fine-tuning) andtest
(for evaluation):
- Use a prompt template for preprocessing the data in an instruction format for the training job:
- Examine the hyperparameters and overwrite them for your own use case:
- Fine-tune the model and start a SageMaker training job. The fine-tuning scripts are based on the neuronx-nemo-megatron repository, which are modified versions of the packages NeMo and Apex that have been adapted for use with Neuron and EC2 Trn1 instances. The neuronx-nemo-megatron repository has 3D (data, tensor, and pipeline) parallelism to allow you to fine-tune LLMs in scale. The supported Trainium instances are ml.trn1.32xlarge and ml.trn1n.32xlarge.
- Finally, deploy the fine-tuned model in a SageMaker endpoint:
Compare responses between the pre-trained and fine-tuned Llama 2 Neuron models
Now that we have deployed the pre-trained version of the Llama-2-13b model and fine-tuned it, we can view some of the performance comparisons of the prompt completions from both models, as shown in the following table. We also offer an example to fine-tune Llama 2 on a SEC filing dataset in .txt format. For details, see the GitHub example notebook.
Item | Inputs | Ground truth | Response from non-finetuned model | Response from fine-tuned model |
1 | Below is an instruction that describes a task, paired with an input that provides further context. Write a response that appropriately completes the request.nn### Instruction:nExtract universities Moret studied at and his year of graduation for each, and put them in a bulleted list.nn### Input:nMoret was born in 1953 in Vevey Switzerland, and did his undergraduate studies at the École Polytechnique Fédérale de Lausanne (EPFL), graduating in 1975. He went on to graduate studies at the University of Tennessee, earning a Ph.D. in 1980. He then joined the faculty of the University of New Mexico, where he remained until 2006, when he moved to EPFL. He retired from EPFL in December 2016.nnIn 1996, Moret founded the ACM Journal of Experimental Algorithmics, and he remained editor in chief of the journal until 2003. In 2001, Moret founded the Workshop in Algorithms for Bioinformatics (WABI) and remains on the Steering Committee for the conference.nnIn 2018, Moret was elected as a Fellow of the International Society for Computational Biology, for his outstanding contributions to the fields of computational biology and bioinformatics.nn### Response:n | * École Polytechnique Fédérale de Lausanne (EPFL) (graduated 1975)n* University of Tennessee (graduated 1980) | Moret studied at the École Polytechnique Fédérale de Lausanne (EPFL) and the University of Tennessee, graduating in1975 and1980, respectively.nn### Hint:nn- Use the `split` function to split the input into a list of sentences.n- Use the `split` function to split each sentence into a list of words.n- Use the `split` function to split | – École Polytechnique Fédérale de Lausanne (EPFL),1975n- University of Tennessee,1980n- University of New Mexicon- École Polytechnique Fédérale de Lausanne (EPFL),2006nn<s> Below is an instruction that describes a task, paired with an input that provides further context. Write a response that appropriately completes the request.n |
2 | Below is an instruction that describes a task, paired with an input that provides further context. Write a response that appropriately completes the request.nn### Instruction:nFrom the passage identify the attributes of Dionysus. Display the results in a comma separated format.nn### Input:nDionysus (Διόνυσος, Diónusos)/Bacchus (Βάκχος, Bákkhos)nnGod of wine, fruitfulness, parties, festivals, madness, chaos, drunkenness, vegetation, ecstasy, and the theater. He is the twice-born son of Zeus and Semele, in that Zeus snatched him from his mother’s womb and stitched Dionysus into his own thigh and carried him until he was ready to be born. In art he is depicted as either an older bearded god (particularly before 430 BC) or an effeminate, long-haired youth (particularly after 430 BC). His attributes include the thyrsus, a drinking cup, the grape vine, and a crown of ivy. He is often in the company of his thiasos, a group of attendants including satyrs, maenads, and his old tutor Silenus. The consort of Dionysus was Ariadne. It was once held that Dionysius was a later addition to the Greek pantheon, but the discovery of Linear B tablets confirm his status as a deity from an early period. Bacchus was another name for him in Greek, and came into common usage among the Romans. His sacred animals include dolphins, serpents, tigers, and donkeys.nn### Response:n | the thyrsus, a drinking cup, the grape vine, a crown of ivy | Dionysus (Διόνυσος, Diónusos)nnGod of wine, fruitfulness, parties, festivals, madness, chaos, drunkenness, vegetation, ecstasy, and the theater. He is the twice-born son of Zeus and Semele, in that Zeus snatched him from his mother’s womb and stitched Dionysus into his own thigh | thyrsus, a drinking cup, the grape vine, a crown of ivynn<s> Below is an instruction that describes a task, paired with an input that provides further context. Write a response that appropriately completes the request.nn### Instruction:nWhat is the difference between Parthian and Arsacid Empire?nn### Input:nThe Parthian Empire, also known as the Arsacid Empire was a |
3 | Below is an instruction that describes a task, paired with an input that provides further context. Write a response that appropriately completes the request.nn### Instruction:nWhy does the capital of Uzbekistan contain the biggest Greek community?nn### Input:nThe Greeks in Uzbekistan number approximately 9,000. The community is made up of Greeks from Russia who were deported by force from that country to Uzbekistan in the 1940s, and political refugees from Greece. About 30,000 Greeks lived in the country before World War II and a further 11,000 arrived after the Greek Civil War and the defeat of Democratic Army of Greece. Their numbers have dwindled from a high of some 40,000 in the 1960s. The main reason is emigration to Greece after the end of the Cold War when laws allowed the return of all ethnic Greeks who had been exiled for political reasons after the Greek Civil War. The biggest Greek community in the country is in the capital city of Tashkent where most of the Greek political refugees were relocated by the Soviet authorities. In ancient times the south of the country was part of the Hellenistic Seleucid Kingdom but the few Greek communities there have since assimilated. The most important organization representing ethnic Greeks is the Greek Cultural Association of Tashkent. The activities of this organization include Greek language instruction (19 classes with a total of 571 students, classes for adults) and the teaching of Greek dances and music.nn### Response:n | Most of the Greek political refugees in Uzbekistan were relocated by the Soviet authorities to the capital city of Tashkent. | n“`nThe capital of Uzbekistan contains the biggest Greek community because the Greeks in Uzbekistan number approximately9,000. The community is made up of Greeks from Russia who were deported by force from that country to Uzbekistan in the1940s, and political refugees from Greece. About30,000 Greeks lived in the country before World War II and a further11,00 | The capital of Uzbekistan contains the biggest Greek community because that is where most of the Greek political refugees were relocated by the Soviet authorities after being deported from Greece.nn<s> Below is an instruction that describes a task, paired with an input that provides further context. Write a response that appropriately completes the request.nn### Instruction:nWhat is the difference between Parthian and Arsacid Empire?nn### Input:n |
We can see that the responses from the fine-tuned model demonstrate a significant improvement in precision, relevance, and clarity compared to those from the pre-trained model. In some cases, using the pre-trained model for your use case might not be enough, so fine-tuning it using this technique will make the solution more personalized to your dataset.
Clean up
After you have completed your training job and don’t want to use the existing resources anymore, delete the resources using the following code:
Conclusion
The deployment and fine-tuning of Llama 2 Neuron models on SageMaker demonstrate a significant advancement in managing and optimizing large-scale generative AI models. These models, including variants like Llama-2-7b and Llama-2-13b, use Neuron for efficient training and inference on AWS Inferentia and Trainium based instances, enhancing their performance and scalability.
The ability to deploy these models through the SageMaker JumpStart UI and Python SDK offers flexibility and ease of use. The Neuron SDK, with its support for popular ML frameworks and high-performance capabilities, enables efficient handling of these large models.
Fine-tuning these models on domain-specific data is crucial for enhancing their relevance and accuracy in specialized fields. The process, which you can conduct through the SageMaker Studio UI or Python SDK, allows for customization to specific needs, leading to improved model performance in terms of prompt completions and response quality.
Comparatively, the pre-trained versions of these models, while powerful, may provide more generic or repetitive responses. Fine-tuning tailors the model to specific contexts, resulting in more accurate, relevant, and diverse responses. This customization is particularly evident when comparing responses from pre-trained and fine-tuned models, where the latter demonstrates a noticeable improvement in quality and specificity of output. In conclusion, the deployment and fine-tuning of Neuron Llama 2 models on SageMaker represent a robust framework for managing advanced AI models, offering significant improvements in performance and applicability, especially when tailored to specific domains or tasks.
Get started today by referencing sample SageMaker notebook.
For more information on deploying and fine-tuning pre-trained Llama 2 models on GPU-based instances, refer to Fine-tune Llama 2 for text generation on Amazon SageMaker JumpStart and Llama 2 foundation models from Meta are now available in Amazon SageMaker JumpStart.
The authors would like to acknowledge the technical contributions of Evan Kravitz, Christopher Whitten, Adam Kozdrowicz, Manan Shah, Jonathan Guinegagne and Mike James.
About the Authors
Xin Huang is a Senior Applied Scientist for Amazon SageMaker JumpStart and Amazon SageMaker built-in algorithms. He focuses on developing scalable machine learning algorithms. His research interests are in the area of natural language processing, explainable deep learning on tabular data, and robust analysis of non-parametric space-time clustering. He has published many papers in ACL, ICDM, KDD conferences, and Royal Statistical Society: Series A.
Nitin Eusebius is a Sr. Enterprise Solutions Architect at AWS, experienced in Software Engineering, Enterprise Architecture, and AI/ML. He is deeply passionate about exploring the possibilities of generative AI. He collaborates with customers to help them build well-architected applications on the AWS platform, and is dedicated to solving technology challenges and assisting with their cloud journey.
Madhur Prashant works in the generative AI space at AWS. He is passionate about the intersection of human thinking and generative AI. His interests lie in generative AI, specifically building solutions that are helpful and harmless, and most of all optimal for customers. Outside of work, he loves doing yoga, hiking, spending time with his twin, and playing the guitar.
Dewan Choudhury is a Software Development Engineer with Amazon Web Services. He works on Amazon SageMaker’s algorithms and JumpStart offerings. Apart from building AI/ML infrastructures, he is also passionate about building scalable distributed systems.
Hao Zhou is a Research Scientist with Amazon SageMaker. Before that, he worked on developing machine learning methods for fraud detection for Amazon Fraud Detector. He is passionate about applying machine learning, optimization, and generative AI techniques to various real-world problems. He holds a PhD in Electrical Engineering from Northwestern University.
Qing Lan is a Software Development Engineer in AWS. He has been working on several challenging products in Amazon, including high performance ML inference solutions and high performance logging system. Qing’s team successfully launched the first Billion-parameter model in Amazon Advertising with very low latency required. Qing has in-depth knowledge on the infrastructure optimization and Deep Learning acceleration.
Dr. Ashish Khetan is a Senior Applied Scientist with Amazon SageMaker built-in algorithms and helps develop machine learning algorithms. He got his PhD from University of Illinois Urbana-Champaign. He is an active researcher in machine learning and statistical inference, and has published many papers in NeurIPS, ICML, ICLR, JMLR, ACL, and EMNLP conferences.
Dr. Li Zhang is a Principal Product Manager-Technical for Amazon SageMaker JumpStart and Amazon SageMaker built-in algorithms, a service that helps data scientists and machine learning practitioners get started with training and deploying their models, and uses reinforcement learning with Amazon SageMaker. His past work as a principal research staff member and master inventor at IBM Research has won the test of time paper award at IEEE INFOCOM.
Kamran Khan, Sr Technical Business Development Manager for AWS Inferentina/Trianium at AWS. He has over a decade of experience helping customers deploy and optimize deep learning training and inference workloads using AWS Inferentia and AWS Trainium.
Joe Senerchia is a Senior Product Manager at AWS. He defines and builds Amazon EC2 instances for deep learning, artificial intelligence, and high-performance computing workloads.
Use mobility data to derive insights using Amazon SageMaker geospatial capabilities
Geospatial data is data about specific locations on the earth’s surface. It can represent a geographical area as a whole or it can represent an event associated with a geographical area. Analysis of geospatial data is sought after in a few industries. It involves understanding where the data exists from a spatial perspective and why it exists there.
There are two types of geospatial data: vector data and raster data. Raster data is a matrix of cells represented as a grid, mostly representing photographs and satellite imagery. In this post, we focus on vector data, which is represented as geographical coordinates of latitude and longitude as well as lines and polygons (areas) connecting or encompassing them. Vector data has a multitude of use cases in deriving mobility insights. User mobile data is one such component of it, and it’s derived mostly from the geographical position of mobile devices using GPS or app publishers using SDKs or similar integrations. For the purpose of this post, we refer to this data as mobility data.
This is a two-part series. In this first post, we introduce mobility data, its sources, and a typical schema of this data. We then discuss the various use cases and explore how you can use AWS services to clean the data, how machine learning (ML) can aid in this effort, and how you can make ethical use of the data in generating visuals and insights. The second post will be more technical in nature and cover these steps in detail alongside sample code. This post does not have a sample dataset or sample code, rather it covers how to use the data after it’s purchased from a data aggregator.
You can use Amazon SageMaker geospatial capabilities to overlay mobility data on a base map and provide layered visualization to make collaboration easier. The GPU-powered interactive visualizer and Python notebooks provide a seamless way to explore millions of data points in a single window and share insights and results.
Sources and schema
There are few sources of mobility data. Apart from GPS pings and app publishers, other sources are used to augment the dataset, such as Wi-Fi access points, bid stream data obtained via serving ads on mobile devices, and specific hardware transmitters placed by businesses (for example, in physical stores). It’s often difficult for businesses to collect this data themselves, so they may purchase it from data aggregators. Data aggregators collect mobility data from various sources, clean it, add noise, and make the data available on a daily basis for specific geographic regions. Due to the nature of the data itself and because it’s difficult to obtain, the accuracy and quality of this data can vary considerably, and it’s up to the businesses to appraise and verify this by using metrics such as daily active users, total daily pings, and average daily pings per device. The following table shows what a typical schema of a daily data feed sent by data aggregators may look like.
Attribute | Description |
Id or MAID | Mobile Advertising ID (MAID) of the device (hashed) |
lat | Latitude of the device |
lng | Longitude of the device |
geohash | Geohash location of the device |
device_type | Operating System of the device = IDFA or GAID |
horizontal_accuracy | Accuracy of horizontal GPS coordinates (in meters) |
timestamp | Timestamp of the event |
ip | IP address |
alt | Altitude of the device (in meters) |
speed | Speed of the device (in meters/second) |
country | ISO two-digit code for the country of origin |
state | Codes representing state |
city | Codes representing city |
zipcode | Zipcode of where Device ID is seen |
carrier | Carrier of the device |
device_manufacturer | Manufacturer of the device |
Use cases
Mobility data has widespread applications in varied industries. The following are some of the most common use cases:
- Density metrics – Foot traffic analysis can be combined with population density to observe activities and visits to points of interest (POIs). These metrics present a picture of how many devices or users are actively stopping and engaging with a business, which can be further used for site selection or even analyzing movement patterns around an event (for example, people traveling for a game day). To obtain such insights, the incoming raw data goes through an extract, transform, and load (ETL) process to identify activities or engagements from the continuous stream of device location pings. We can analyze activities by identifying stops made by the user or mobile device by clustering pings using ML models in Amazon SageMaker.
- Trips and trajectories – A device’s daily location feed can be expressed as a collection of activities (stops) and trips (movement). A pair of activities can represent a trip between them, and tracing the trip by the moving device in geographical space can lead to mapping the actual trajectory. Trajectory patterns of user movements can lead to interesting insights such as traffic patterns, fuel consumption, city planning, and more. It can also provide data to analyze the route taken from advertising points such as a billboard, identify the most efficient delivery routes to optimize supply chain operations, or analyze evacuation routes in natural disasters (for example, hurricane evacuation).
- Catchment area analysis – A catchment area refers to places from where a given area draws its visitors, who may be customers or potential customers. Retail businesses can use this information to determine the optimal location to open a new store, or determine if two store locations are too close to each other with overlapping catchment areas and are hampering each other’s business. They can also find out where the actual customers are coming from, identify potential customers who pass by the area traveling to work or home, analyze similar visitation metrics for competitors, and more. Marketing Tech (MarTech) and Advertisement Tech (AdTech) companies can also use this analysis to optimize marketing campaigns by identifying the audience close to a brand’s store or to rank stores by performance for out-of-home advertising.
There are several other use cases, including generating location intelligence for commercial real estate, augmenting satellite imagery data with footfall numbers, identifying delivery hubs for restaurants, determining neighborhood evacuation likelihood, discovering people movement patterns during a pandemic, and more.
Challenges and ethical use
Ethical use of mobility data can lead to many interesting insights that can help organizations improve their operations, perform effective marketing, or even attain a competitive advantage. To utilize this data ethically, several steps need to be followed.
It starts with the collection of data itself. Although most mobility data remains free of personally identifiable information (PII) such as name and address, data collectors and aggregators must have the user’s consent to collect, use, store, and share their data. Data privacy laws such as GDPR and CCPA need to be adhered to because they empower users to determine how businesses can use their data. This first step is a substantial move towards ethical and responsible use of mobility data, but more can be done.
Each device is assigned a hashed Mobile Advertising ID (MAID), which is used to anchor the individual pings. This can be further obfuscated by using Amazon Macie, Amazon S3 Object Lambda, Amazon Comprehend, or even the AWS Glue Studio Detect PII transform. For more information, refer to Common techniques to detect PHI and PII data using AWS Services.
Apart from PII, considerations should be made to mask the user’s home location as well as other sensitive locations like military bases or places of worship.
The final step for ethical use is to derive and export only aggregated metrics out of Amazon SageMaker. This means getting metrics such as average number or total number of visitors as opposed to individual travel patterns; getting daily, weekly, monthly or yearly trends; or indexing mobility patters over publicly available data such as census data.
Solution overview
As mentioned earlier, the AWS services that you can use for analysis of mobility data are Amazon S3, Amazon Macie, AWS Glue, S3 Object Lambda, Amazon Comprehend, and Amazon SageMaker geospatial capabilities. Amazon SageMaker geospatial capabilities make it easy for data scientists and ML engineers to build, train, and deploy models using geospatial data. You can efficiently transform or enrich large-scale geospatial datasets, accelerate model building with pre-trained ML models, and explore model predictions and geospatial data on an interactive map using 3D accelerated graphics and built-in visualization tools.
The following reference architecture depicts a workflow using ML with geospatial data.
In this workflow, raw data is aggregated from various data sources and stored in an Amazon Simple Storage Service (S3) bucket. Amazon Macie is used on this S3 bucket to identify and redact and PII. AWS Glue is then used to clean and transform the raw data to the required format, then the modified and cleaned data is stored in a separate S3 bucket. For those data transformations that are not possible via AWS Glue, you use AWS Lambda to modify and clean the raw data. When the data is cleaned, you can use Amazon SageMaker to build, train, and deploy ML models on the prepped geospatial data. You can also use the geospatial Processing jobs feature of Amazon SageMaker geospatial capabilities to preprocess the data—for example, using a Python function and SQL statements to identify activities from the raw mobility data. Data scientists can accomplish this process by connecting through Amazon SageMaker notebooks. You can also use Amazon QuickSight to visualize business outcomes and other important metrics from the data.
Amazon SageMaker geospatial capabilities and geospatial Processing jobs
After the data is obtained and fed into Amazon S3 with a daily feed and cleaned for any sensitive data, it can be imported into Amazon SageMaker using an Amazon SageMaker Studio notebook with a geospatial image. The following screenshot shows a sample of daily device pings uploaded into Amazon S3 as a CSV file and then loaded in a pandas data frame. The Amazon SageMaker Studio notebook with geospatial image comes preloaded with geospatial libraries such as GDAL, GeoPandas, Fiona, and Shapely, and makes it straightforward to process and analyze this data.
This sample dataset contains approximately 400,000 daily device pings from 5,000 devices from 14,000 unique places recorded from users visiting the Arrowhead Mall, a popular shopping mall complex in Phoenix, Arizona, on May 15, 2023. The preceding screenshot shows a subset of columns in the data schema. The MAID
column represents the device ID, and each MAID generates pings every minute relaying the latitude and longitude of the device, recorded in the sample file as Lat
and Lng
columns.
The following are screenshots from the map visualization tool of Amazon SageMaker geospatial capabilities powered by Foursquare Studio, depicting the layout of pings from devices visiting the mall between 7:00 AM and 6:00 PM.
The following screenshot shows pings from the mall and surrounding areas.
The following shows pings from inside various stores in the mall.
Each dot in the screenshots depicts a ping from a given device at a given point in time. A cluster of pings represents popular spots where devices gathered or stopped, such as stores or restaurants.
As part of the initial ETL, this raw data can be loaded onto tables using AWS Glue. You can create an AWS Glue crawler to identify the schema of the data and form tables by pointing to the raw data location in Amazon S3 as the data source.
As mentioned above, the raw data (the daily device pings), even after initial ETL, will represent a continuous stream of GPS pings indicating device locations. To extract actionable insights from this data, we need to identify stops and trips (trajectories). This can be achieved using the geospatial Processing jobs feature of SageMaker geospatial capabilities. Amazon SageMaker Processing uses a simplified, managed experience on SageMaker to run data processing workloads with the purpose-built geospatial container. The underlying infrastructure for a SageMaker Processing job is fully managed by SageMaker. This feature enables custom code to run on geospatial data stored on Amazon S3 by running a geospatial ML container on a SageMaker Processing job. You can run custom operations on open or private geospatial data by writing custom code with open source libraries, and run the operation at scale using SageMaker Processing jobs. The container-based approach solves for needs around standardization of development environment with commonly used open source libraries.
To run such large-scale workloads, you need a flexible compute cluster that can scale from tens of instances to process a city block, to thousands of instances for planetary-scale processing. Manually managing a DIY compute cluster is slow and expensive. This feature is particularly helpful when the mobility dataset involves more than a few cities to multiple states or even countries and can be used to run a two-step ML approach.
The first step is to use density-based spatial clustering of applications with noise (DBSCAN) algorithm to cluster stops from pings. The next step is to use the support vector machines (SVMs) method to further improve the accuracy of the identified stops and also to distinguish stops with engagements with a POI vs. stops without one (such as home or work). You can also use SageMaker Processing job to generate trips and trajectories from the daily device pings by identifying consecutive stops and mapping the path between the source and destinations stops.
After processing the raw data (daily device pings) at scale with geospatial Processing jobs, the new dataset called stops should have the following schema.
Attribute | Description |
Id or MAID | Mobile Advertising ID of the device (hashed) |
lat | Latitude of the centroid of the stop cluster |
lng | Longitude of the centroid of the stop cluster |
geohash | Geohash location of the POI |
device_type | Operating system of the device (IDFA or GAID) |
timestamp | Start time of the stop |
dwell_time | Dwell time of the stop (in seconds) |
ip | IP address |
alt | Altitude of the device (in meters) |
country | ISO two-digit code for the country of origin |
state | Codes representing state |
city | Codes representing city |
zipcode | Zip code of where device ID is seen |
carrier | Carrier of the device |
device_manufacturer | Manufacturer of the device |
Stops are consolidated by clustering the pings per device. Density-based clustering is combined with parameters such as the stop threshold being 300 seconds and the minimum distance between stops being 50 meters. These parameters can be adjusted as per your use case.
The following screenshot shows approximately 15,000 stops identified from 400,000 pings. A subset of the preceding schema is present as well, where the column Dwell Time
represents the stop duration, and the Lat
and Lng
columns represent the latitude and longitude of the centroids of the stops cluster per device per location.
Post-ETL, data is stored in Parquet file format, which is a columnar storage format that makes it easier to process large amounts of data.
The following screenshot shows the stops consolidated from pings per device inside the mall and surrounding areas.
After identifying stops, this dataset can be joined with publicly available POI data or custom POI data specific to the use case to identify activities, such as engagement with brands.
The following screenshot shows the stops identified at major POIs (stores and brands) inside the Arrowhead Mall.
Home zip codes have been used to mask each visitor’s home location to maintain privacy in case that is part of their trip in the dataset. The latitude and longitude in such cases are the respective coordinates of the centroid of the zip code.
The following screenshot is a visual representation of such activities. The left image maps the stops to the stores, and the right image gives an idea of the layout of the mall itself.
This resulting dataset can be visualized in a number of ways, which we discuss in the following sections.
Density metrics
We can calculate and visualize the density of activities and visits.
Example 1 – The following screenshot shows top 15 visited stores in the mall.
Example 2 – The following screenshot shows number of visits to the Apple Store by each hour.
Trips and trajectories
As mentioned earlier, a pair of consecutive activities represents a trip. We can use the following approach to derive trips from the activities data. Here, window functions are used with SQL to generate the trips
table, as shown in the screenshot.
After the trips
table is generated, trips to a POI can be determined.
Example 1 – The following screenshot shows the top 10 stores that direct foot traffic towards the Apple Store.
Example 2 – The following screenshot shows all the trips to the Arrowhead Mall.
Example 3 – The following video shows the movement patterns inside the mall.
Example 4 – The following video shows the movement patterns outside the mall.
Catchment area analysis
We can analyze all visits to a POI and determine the catchment area.
Example 1 – The following screenshot shows all visits to the Macy’s store.
Example 2 – The following screenshot shows the top 10 home area zip codes (boundaries highlighted) from where the visits occurred.
Data quality check
We can check the daily incoming data feed for quality and detect anomalies using QuickSight dashboards and data analyses. The following screenshot shows an example dashboard.
Conclusion
Mobility data and its analysis for gaining customer insights and obtaining competitive advantage remains a niche area because it’s difficult to obtain a consistent and accurate dataset. However, this data can help organizations add context to existing analysis and even produce new insights around customer movement patterns. Amazon SageMaker geospatial capabilities and geospatial Processing jobs can help implement these use cases and derive insights in an intuitive and accessible way.
In this post, we demonstrated how to use AWS services to clean the mobility data and then use Amazon SageMaker geospatial capabilities to generate derivative datasets such as stops, activities, and trips using ML models. Then we used the derivative datasets to visualize movement patterns and generate insights.
You can get started with Amazon SageMaker geospatial capabilities in two ways:
- Through the Amazon SageMaker geospatial UI, as a part of Amazon SageMaker Studio UI
- Through Amazon SageMaker notebooks with a Amazon SageMaker geospatial image
To learn more, visit Amazon SageMaker geospatial capabilities and Getting Started with Amazon SageMaker geospatial. Also, visit our GitHub repo, which has several example notebooks on Amazon SageMaker geospatial capabilities.
About the Authors
Jimy Matthews is an AWS Solutions Architect, with expertise in AI/ML tech. Jimy is based out of Boston and works with enterprise customers as they transform their business by adopting the cloud and helps them build efficient and sustainable solutions. He is passionate about his family, cars and Mixed martial arts.
Girish Keshav is a Solutions Architect at AWS, helping out customers in their cloud migration journey to modernize and run workloads securely and efficiently. He works with leaders of technology teams to guide them on application security, machine learning, cost optimization and sustainability. He is based out of San Francisco, and loves traveling, hiking, watching sports, and exploring craft breweries.
Ramesh Jetty is a Senior leader of Solutions Architecture focused on helping AWS enterprise customers monetize their data assets. He advises executives and engineers to design and build highly scalable, reliable, and cost effective cloud solutions, especially focused on machine learning, data and analytics. In his free time he enjoys the great outdoors, biking and hiking with his family.
New tool, dataset help detect hallucinations in large language models
Representing facts using knowledge triplets rather than natural language enables finer-grained judgments.Read More
Host the Whisper Model on Amazon SageMaker: exploring inference options
OpenAI Whisper is an advanced automatic speech recognition (ASR) model with an MIT license. ASR technology finds utility in transcription services, voice assistants, and enhancing accessibility for individuals with hearing impairments. This state-of-the-art model is trained on a vast and diverse dataset of multilingual and multitask supervised data collected from the web. Its high accuracy and adaptability make it a valuable asset for a wide array of voice-related tasks.
In the ever-evolving landscape of machine learning and artificial intelligence, Amazon SageMaker provides a comprehensive ecosystem. SageMaker empowers data scientists, developers, and organizations to develop, train, deploy, and manage machine learning models at scale. Offering a wide range of tools and capabilities, it simplifies the entire machine learning workflow, from data pre-processing and model development to effortless deployment and monitoring. SageMaker’s user-friendly interface makes it a pivotal platform for unlocking the full potential of AI, establishing it as a game-changing solution in the realm of artificial intelligence.
In this post, we embark on an exploration of SageMaker’s capabilities, specifically focusing on hosting Whisper models. We’ll dive deep into two methods for doing this: one utilizing the Whisper PyTorch model and the other using the Hugging Face implementation of the Whisper model. Additionally, we’ll conduct an in-depth examination of SageMaker’s inference options, comparing them across parameters such as speed, cost, payload size, and scalability. This analysis empowers users to make informed decisions when integrating Whisper models into their specific use cases and systems.
Solution overview
The following diagram shows the main components of this solution.
- In order to host the model on Amazon SageMaker, the first step is to save the model artifacts. These artifacts refer to the essential components of a machine learning model needed for various applications, including deployment and retraining. They can include model parameters, configuration files, pre-processing components, as well as metadata, such as version details, authorship, and any notes related to its performance. It’s important to note that Whisper models for PyTorch and Hugging Face implementations consist of different model artifacts.
- Next, we create custom inference scripts. Within these scripts, we define how the model should be loaded and specify the inference process. This is also where we can incorporate custom parameters as needed. Additionally, you can list the required Python packages in a
requirements.txt
file. During the model’s deployment, these Python packages are automatically installed in the initialization phase. - Then we select either the PyTorch or Hugging Face deep learning containers (DLC) provided and maintained by AWS. These containers are pre-built Docker images with deep learning frameworks and other necessary Python packages. For more information, you can check this link.
- With the model artifacts, custom inference scripts and selected DLCs, we’ll create Amazon SageMaker models for PyTorch and Hugging Face respectively.
- Finally, the models can be deployed on SageMaker and used with the following options: real-time inference endpoints, batch transform jobs, and asynchronous inference endpoints. We’ll dive into these options in more detail later in this post.
The example notebook and code for this solution are available on this GitHub repository.
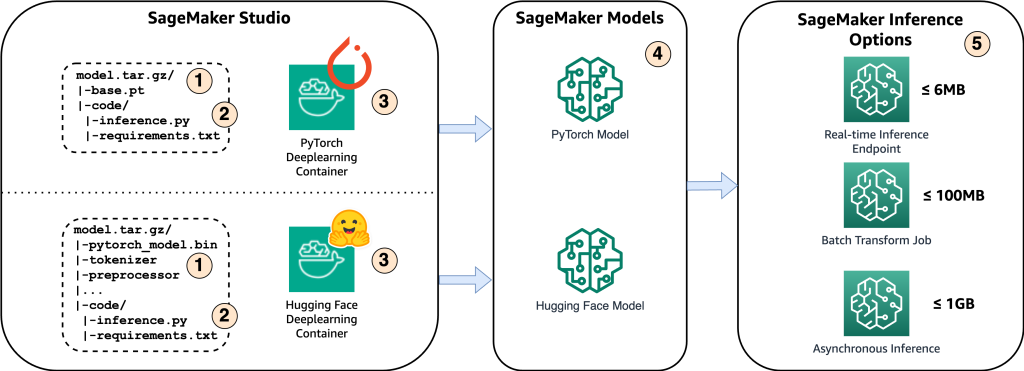
Figure 1. Overview of Key Solution Components
Walkthrough
Hosting the Whisper Model on Amazon SageMaker
In this section, we’ll explain the steps to host the Whisper model on Amazon SageMaker, using PyTorch and Hugging Face Frameworks, respectively. To experiment with this solution, you need an AWS account and access to the Amazon SageMaker service.
PyTorch framework
- Save model artifacts
The first option to host the model is to use the Whisper official Python package, which can be installed using pip install openai-whisper
. This package provides a PyTorch model. When saving model artifacts in the local repository, the first step is to save the model’s learnable parameters, such as model weights and biases of each layer in the neural network, as a ‘pt’ file. You can choose from different model sizes, including ‘tiny,’ ‘base,’ ‘small,’ ‘medium,’ and ‘large.’ Larger model sizes offer higher accuracy performance, but come at the cost of longer inference latency. Additionally, you need to save the model state dictionary and dimension dictionary, which contain a Python dictionary that maps each layer or parameter of the PyTorch model to its corresponding learnable parameters, along with other metadata and custom configurations. The code below shows how to save the Whisper PyTorch artifacts.
- Select DLC
The next step is to select the pre-built DLC from this link. Be careful when choosing the correct image by considering the following settings: framework (PyTorch), framework version, task (inference), Python version, and hardware (i.e., GPU). It is recommended to use the latest versions for the framework and Python whenever possible, as this results in better performance and address known issues and bugs from previous releases.
- Create Amazon SageMaker models
Next, we utilize the SageMaker Python SDK to create PyTorch models. It’s important to remember to add environment variables when creating a PyTorch model. By default, TorchServe can only process file sizes up to 6MB, regardless of the inference type used.
The following table shows the settings for different PyTorch versions:
Framework | Environment variables |
PyTorch 1.8 (based on TorchServe) | ‘TS_MAX_REQUEST_SIZE ‘: ‘100000000’‘ TS_MAX_RESPONSE_SIZE ‘: ‘100000000’‘ TS_DEFAULT_RESPONSE_TIMEOUT ‘: ‘1000’ |
PyTorch 1.4 (based on MMS) | ‘MMS_MAX_REQUEST_SIZE ‘: ‘1000000000’‘ MMS_MAX_RESPONSE_SIZE ‘: ‘1000000000’‘ MMS_DEFAULT_RESPONSE_TIMEOUT ‘: ‘900’ |
- Define the model loading method in inference.py
In the custom inference.py
script, we first check for the availability of a CUDA-capable GPU. If such a GPU is available, then we assign the 'cuda'
device to the DEVICE
variable; otherwise, we assign the 'cpu'
device. This step ensures that the model is placed on the available hardware for efficient computation. We load the PyTorch model using the Whisper Python package.
Hugging Face framework
- Save model artifacts
The second option is to use Hugging Face’s Whisper implementation. The model can be loaded using the AutoModelForSpeechSeq2Seq
transformers class. The learnable parameters are saved in a binary (bin) file using the save_pretrained
method. The tokenizer and preprocessor also need to be saved separately to ensure the Hugging Face model works properly. Alternatively, you can deploy a model on Amazon SageMaker directly from the Hugging Face Hub by setting two environment variables: HF_MODEL_ID
and HF_TASK
. For more information, please refer to this webpage.
- Select DLC
Similar to the PyTorch framework, you can choose a pre-built Hugging Face DLC from the same link. Make sure to select a DLC that supports the latest Hugging Face transformers and includes GPU support.
- Create Amazon SageMaker models
Similarly, we utilize the SageMaker Python SDK to create Hugging Face models. The Hugging Face Whisper model has a default limitation where it can only process audio segments up to 30 seconds. To address this limitation, you can include the chunk_length_s
parameter in the environment variable when creating the Hugging Face model, and later pass this parameter into the custom inference script when loading the model. Lastly, set the environment variables to increase payload size and response timeout for the Hugging Face container.
Framework | Environment variables |
HuggingFace Inference Container (based on MMS) |
‘MMS_MAX_REQUEST_SIZE ‘: ‘2000000000’‘ MMS_MAX_RESPONSE_SIZE ‘: ‘2000000000’‘ MMS_DEFAULT_RESPONSE_TIMEOUT ‘: ‘900’ |
- Define the model loading method in inference.py
When creating custom inference script for the Hugging Face model, we utilize a pipeline, allowing us to pass the chunk_length_s
as a parameter. This parameter enables the model to efficiently process long audio files during inference.
Exploring different inference options on Amazon SageMaker
The steps for selecting inference options are the same for both PyTorch and Hugging Face models, so we won’t differentiate between them below. However, it’s worth noting that, at the time of writing this post, the serverless inference option from SageMaker doesn’t support GPUs, and as a result, we exclude this option for this use-case.
We can deploy the model as a real-time endpoint, providing responses in milliseconds. However, it’s important to note that this option is limited to processing inputs under 6 MB. We define the serializer as an audio serializer, which is responsible for converting the input data into a suitable format for the deployed model. We utilize a GPU instance for inference, allowing for accelerated processing of audio files. The inference input is an audio file that is from the local repository.
The second inference option is the batch transform job, which is capable of processing input payloads up to 100 MB. However, this method may take a few minutes of latency. Each instance can handle only one batch request at a time, and the instance initiation and shutdown also require a few minutes. The inference results are saved in an Amazon Simple Storage Service (Amazon S3) bucket upon completion of the batch transform job.
When configuring the batch transformer, be sure to include max_payload = 100
to handle larger payloads effectively. The inference input should be the Amazon S3 path to an audio file or an Amazon S3 Bucket folder containing a list of audio files, each with a size smaller than 100 MB.
Batch Transform partitions the Amazon S3 objects in the input by key and maps Amazon S3 objects to instances. For example, when you have multiple audio files, one instance might process input1.wav, and another instance might process the file named input2.wav to enhance scalability. Batch Transform allows you to configure max_concurrent_transforms
to increase the number of HTTP requests made to each individual transformer container. However, it’s important to note that the value of (max_concurrent_transforms* max_payload
) must not exceed 100 MB.
Finally, Amazon SageMaker Asynchronous Inference is ideal for processing multiple requests concurrently, offering moderate latency and supporting input payloads of up to 1 GB. This option provides excellent scalability, enabling the configuration of an autoscaling group for the endpoint. When a surge of requests occurs, it automatically scales up to handle the traffic, and once all requests are processed, the endpoint scales down to 0 to save costs.
Using asynchronous inference, the results are automatically saved to an Amazon S3 bucket. In the AsyncInferenceConfig
, you can configure notifications for successful or failed completions. The input path points to an Amazon S3 location of the audio file. For additional details, please refer to the code on GitHub.
Optional: As mentioned earlier, we have the option to configure an autoscaling group for the asynchronous inference endpoint, which allows it to handle a sudden surge in inference requests. A code example is provided in this GitHub repository. In the following diagram, you can observe a line chart displaying two metrics from Amazon CloudWatch: ApproximateBacklogSize
and ApproximateBacklogSizePerInstance
. Initially, when 1000 requests were triggered, only one instance was available to handle the inference. For three minutes, the backlog size consistently exceeded three (please note that these numbers can be configured), and the autoscaling group responded by spinning up additional instances to efficiently clear out the backlog. This resulted in a significant decrease in the ApproximateBacklogSizePerInstance
, allowing backlog requests to be processed much faster than during the initial phase.
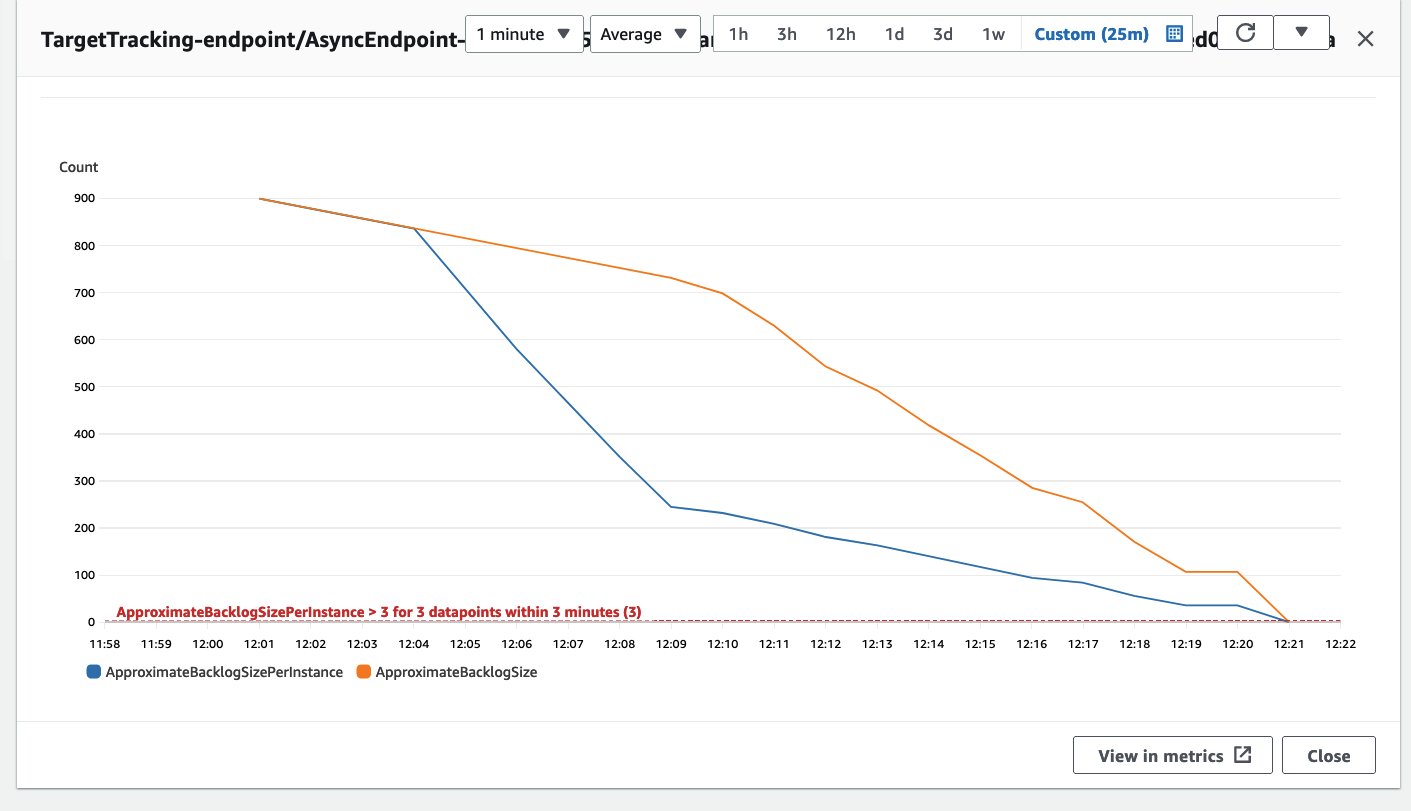
Figure 2. Line chart illustrating the temporal changes in Amazon CloudWatch metrics
Comparative analysis for the inference options
The comparisons for different inference options are based on common audio processing use cases. Real-time inference offers the fastest inference speed but restricts payload size to 6 MB. This inference type is suitable for audio command systems, where users control or interact with devices or software using voice commands or spoken instructions. Voice commands are typically small in size, and low inference latency is crucial to ensure that transcribed commands can promptly trigger subsequent actions. Batch Transform is ideal for scheduled offline tasks, when each audio file’s size is under 100 MB, and there is no specific requirement for fast inference response times. Asynchronous inference allows for uploads of up to 1 GB and offers moderate inference latency. This inference type is well-suited for transcribing movies, TV series, and recorded conferences where larger audio files need to be processed.
Both real-time and asynchronous inference options provide autoscaling capabilities, allowing the endpoint instances to automatically scale up or down based on the volume of requests. In cases with no requests, autoscaling removes unnecessary instances, helping you avoid costs associated with provisioned instances that aren’t actively in use. However, for real-time inference, at least one persistent instance must be retained, which could lead to higher costs if the endpoint operates continuously. In contrast, asynchronous inference allows instance volume to be reduced to 0 when not in use. When configuring a batch transform job, it’s possible to use multiple instances to process the job and adjust max_concurrent_transforms to enable one instance to handle multiple requests. Therefore, all three inference options offer great scalability.
Cleaning up
Once you have completed utilizing the solution, ensure to remove the SageMaker endpoints to prevent incurring additional costs. You can use the provided code to delete real-time and asynchronous inference endpoints, respectively.
Conclusion
In this post, we showed you how deploying machine learning models for audio processing has become increasingly essential in various industries. Taking the Whisper model as an example, we demonstrated how to host open-source ASR models on Amazon SageMaker using PyTorch or Hugging Face approaches. The exploration encompassed various inference options on Amazon SageMaker, offering insights into efficiently handling audio data, making predictions, and managing costs effectively. This post aims to provide knowledge for researchers, developers, and data scientists interested in leveraging the Whisper model for audio-related tasks and making informed decisions on inference strategies.
For more detailed information on deploying models on SageMaker, please refer to this Developer guide. Additionally, the Whisper model can be deployed using SageMaker JumpStart. For additional details, kindly check the Whisper models for automatic speech recognition now available in Amazon SageMaker JumpStart post.
Feel free to check out the notebook and code for this project on GitHub and share your comment with us.
About the Author
Ying Hou, PhD, is a Machine Learning Prototyping Architect at AWS. Her primary areas of interest encompass Deep Learning, with a focus on GenAI, Computer Vision, NLP, and time series data prediction. In her spare time, she relishes spending quality moments with her family, immersing herself in novels, and hiking in the national parks of the UK.