The post Coding for the cosmos appeared first on The AI Blog.
Azure Quantum innovation: Efficient error correction of topological qubits with Floquet codes

Technological innovation that enables scaling of quantum computing underpins the Microsoft Azure Quantum program. In March of this year, we announced our demonstration of the underlying physics required to create a topological qubit—qubits that are theorized to be inherently more stable than existing ones without sacrificing size or speed. However, our quest to deliver a general-purpose quantum computer capable of addressing industrial-scale problems will require innovation across every layer of the quantum stack, from materials at the nanoscale to algorithms and applications. At Azure Quantum, our full-stack approach and broad expertise across all areas of quantum computation allows us to drive innovation in this space through tight collaboration across theory, hardware, software and systems teams.
One of the greatest challenges in building a quantum computer is that quantum states are intrinsically fragile and are quickly destroyed when a qubit couples to its environment, leading to noise. A crucial technology to overcome this fragility, which is also used in classical digital computing, is error correction. By encoding the state of a single logical qubit into many physical qubits, quantum error correction (QEC) has the ability to detect and correct most errors that occur on the physical qubits. Indeed, such error correction needs to be at the heart of any scalable quantum system. Without it, no known qubit technology can protect quantum states sufficiently long enough to perform a calculation that can deliver real-world impact. However, quantum error correction also comes at a significant cost: depending on the quality of the physical qubits, error correction can increase the space requirements of a computation by a factor of several thousand and the time requirements more than tenfold. Therefore, any improvements on error correction have enormous positive ripple effects across the entire stack.
In this post, we’ll share some exciting implications from our recent innovations toward scale—specifically how to perform quantum error correction in our topological quantum computation stack— published in the series of papers listed below. Topological qubits promise lower error rates than conventional qubits, and as such can perform scalable quantum computation at lower overhead. On top of that, in these papers we introduce a new class of quantum error correction codes, called Floquet codes, which are particularly suited to topological qubits. Our new approaches culminate in an additional tenfold or more reduction to the overhead needed for error correction on topological qubits compared to previous state of the art, opening a viable path toward scaling to a million qubits and beyond.
Explore More
-
Publication
Dynamically Generated Logical Qubits
-
Publication
Boundaries for the Honeycomb Code
Unlocking a new class of quantum codes
To optimize performance on any quantum computing platform, the circuits must be adapted to the capabilities of the hardware. This is particularly true for error correction schemes, which must be tailor-made to exploit the strengths of a given hardware platform. Unlike most other qubits, our topological qubits employ a measurement-based scheme, where direct measurements between adjacent qubits are the native set of operations. While all quantum error correction schemes use frequent measurements to identify errors, the state-of-the-art schemes require complex multi-qubit measurements that can’t be implemented directly in the hardware and must be compiled into native operations at the expense of additional auxiliary qubits and additional timesteps. The outcomes of these measurements are used to infer the occurrence of errors without destroying the encoded quantum state.
Our recent breakthroughs overcome this issue through a conceptually new perspective on quantum codes (put forward in “Dynamically Generated Logical Qubits” and “Boundaries for the Honeycomb code”), where the encoding of the quantum information is not static but rather allowed to periodically evolve in time. Many examples of physical systems are known where such periodic evolution allows new phenomena to occur (see, for example, the well-known Kapitza pendulum). The study of such systems falls under the term Floquet systems, which gives this new class of codes its name.
These codes are built entirely from two-qubit measurements referred to as “check measurements.” Just like measurements in a conventional code, these are used to check for errors. The simplicity of these checks, however, means that each time we measure a check, we change the encoding of the quantum information, leading to the Floquet nature of the code. As a consequence, the outcomes of these measurements cannot be used directly to infer which errors have occurred, but rather the full history of measurement outcomes over time must be taken into account.
The physical qubits are arranged in a lattice (such as that shown in Figure 1), represented as black dots on the vertices of this graph. Each check is associated with an edge of the graph, and one sequentially measures checks of different colors. The code state changes as the different checks are measured. There are several possible lattice arrangements of the qubits that allow for a natural implementation of a Floquet code. The lattices should have the following two properties: 1) each vertex should be attached to three edges and 2) using only three colors, it should be possible to color the plaquettes in such a way that no adjacent plaquettes have the same color (that is, the plaquettes should be “three-colorable”). While many such arrangements remain to be explored and the optimal choice will depend on details of the physical hardware, Figure 1 shows two possible Floquet-code arrangements.
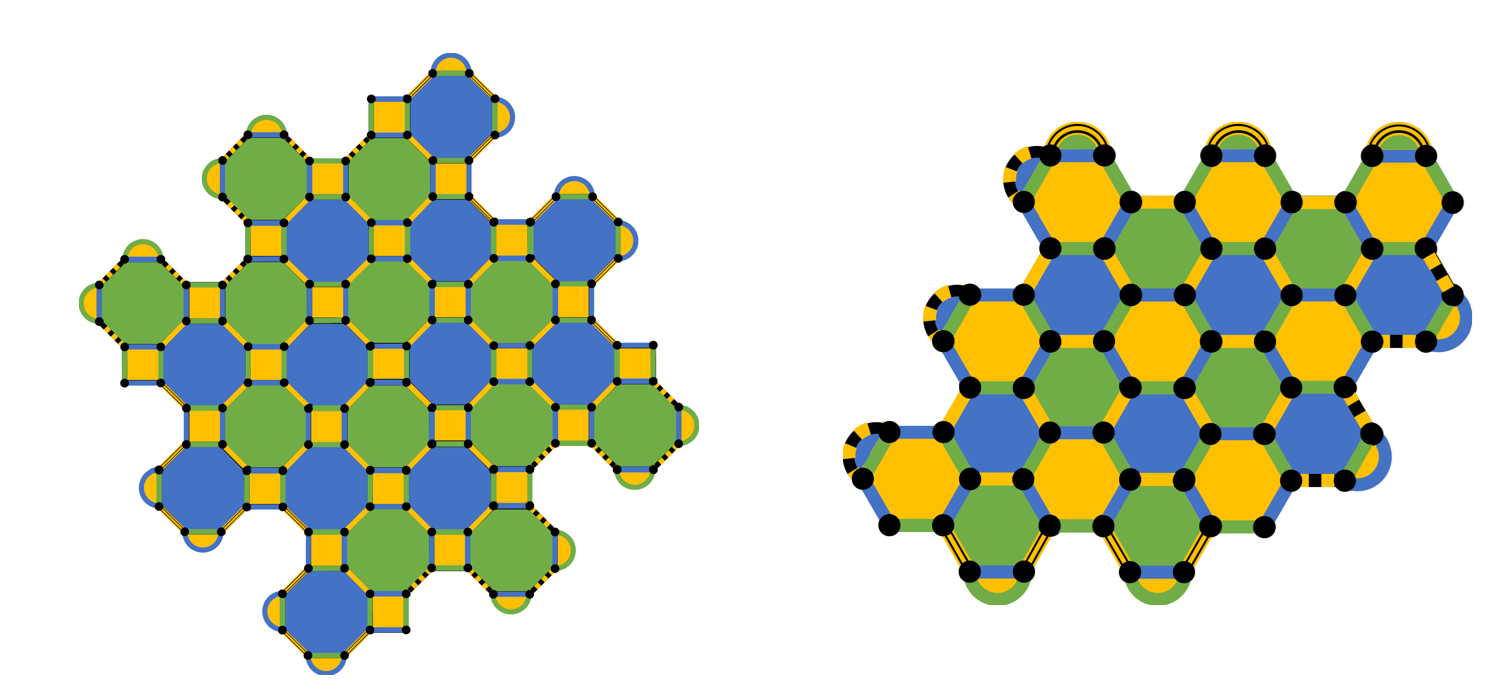
Error correction tailor-made for topological qubits
In the realm of our measurement-based topological architecture, we have identified the two arrangements shown in Figure 1 as particularly appealing when combined with a particular design of topological qubit—a “tetron” qubit—which is also a scalable design. The connectivity of these two layouts can be naturally mapped onto the connectivity of an array of such tetrons, which is shown in Figure 2. Furthermore, the majority of the two-qubit check operators that are used to construct these codes are exactly those native operations between tetrons that can be implemented with minimal error, as shown in the lower panel of Figure 2. The details of these codes, their implementation with topological qubits, and numerical studies of their performance are discussed in “Performance of planar Floquet codes with Majorana-based qubits.”
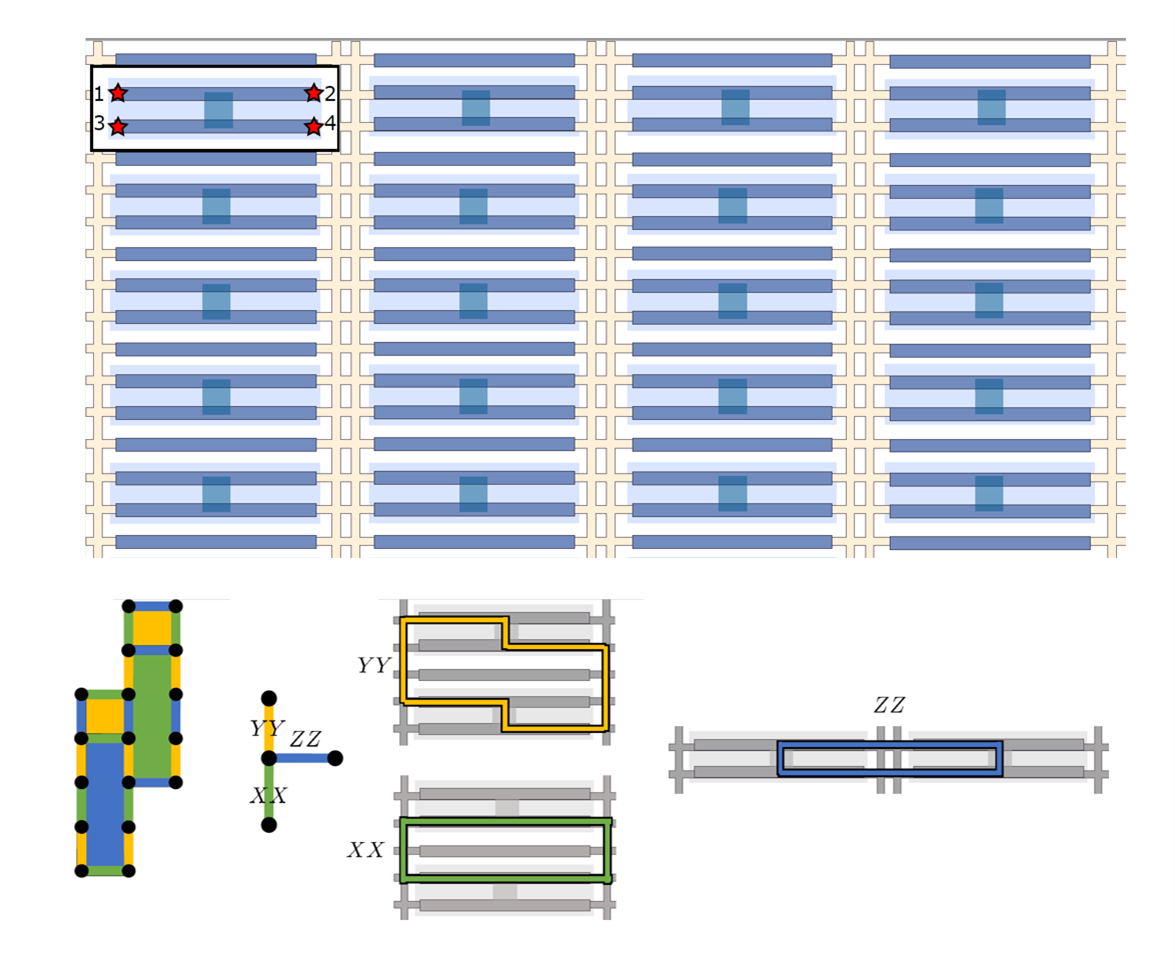
Our numerical simulations show that our Floquet codes and architecture implemented with topological “tetron” qubits help secure the path to a scalable quantum system in several ways. First, the very favorable threshold of these codes, which we estimate to be close to 1 percent, allows us to achieve quantum error correction earlier and demonstrate tangible steps on our journey toward quantum advantage. Second, in the longer run, we find that these codes reduce the overhead required for quantum error correction on topological qubits roughly tenfold compared to the previous state-of-the-art approach, which means that our scalable system can be built from fewer physical qubits and can run at a faster clock speed (see Figure 3 below).
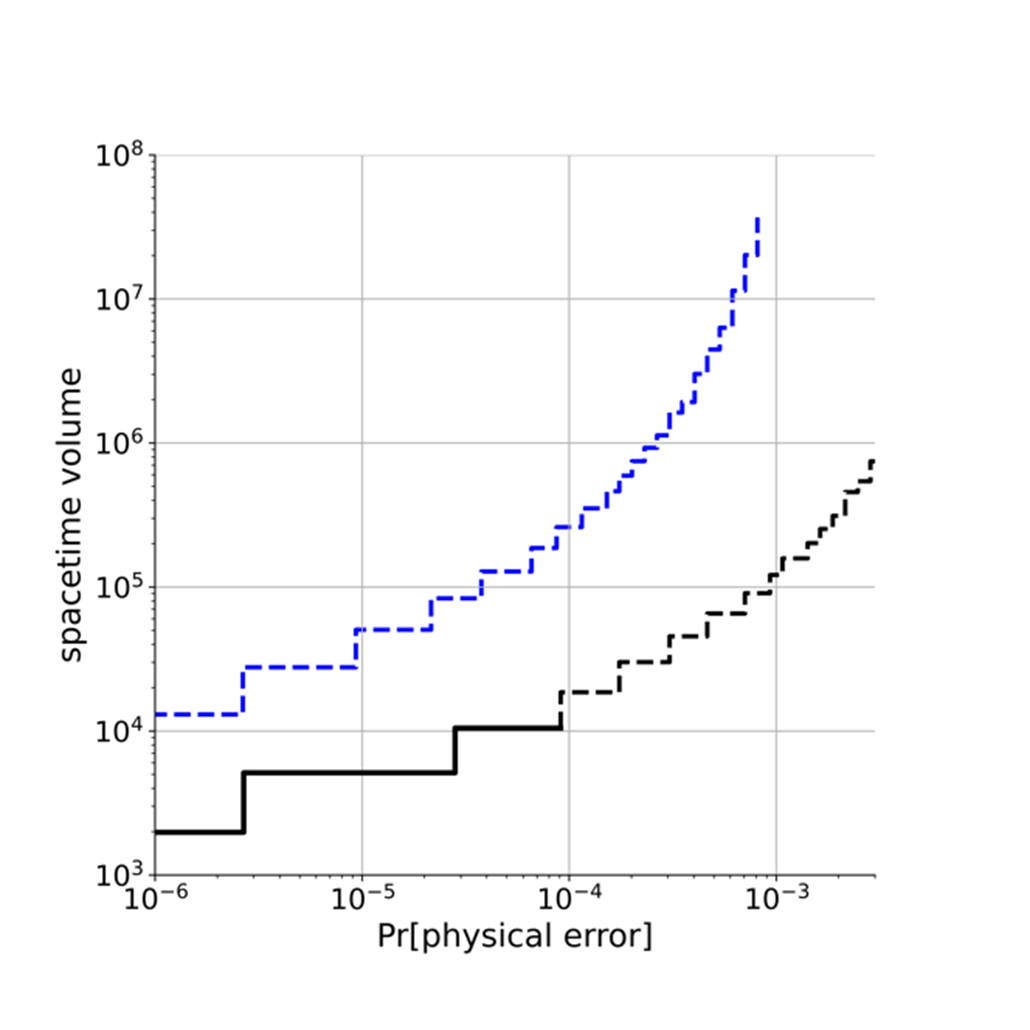
Approaching quantum computation from the unique topological perspective requires synchronized advancements across the entire Azure Quantum stack. Along with our recent demonstration of the building blocks for topological qubits, optimizing quantum error correction using Floquet codes represents a critical piece of the scientific foundation needed to achieve scaled quantum computation. These breakthroughs help establish a path and architecture for the industrial quantum machine.
The post Azure Quantum innovation: Efficient error correction of topological qubits with Floquet codes appeared first on Microsoft Research.
MoLeR: Creating a path to more efficient drug design
Drug discovery has come a long way from its roots in serendipity. It is now an increasingly rational process, in which one important phase, called lead optimization, is the stepwise search for promising drug candidate compounds in the lab. In this phase, expert medicinal chemists work to improve “hit” molecules—compounds that demonstrate some promising properties, as well as some undesirable ones, in early screening. In subsequent testing, chemists try to adapt the structure of hit molecules to improve their biological efficacy and reduce potential side effects. This process combines knowledge, creativity, experience, and intuition, and often lasts for years. Over many decades, computational modelling techniques have been developed to help predict how the molecules will fare in the lab, so that costly and time-consuming experiments can focus on the most promising compounds.

The Microsoft Generative Chemistry team is working with Novartis to improve these modelling techniques with a new model called MoLeR.
“MoLeR illustrates how generative models based on deep learning can help transform the drug discovery process and enable our colleagues at Novartis to increase the efficiency in finding new compounds.”
Christopher Bishop, Technical Fellow and Laboratory Director, Microsoft Research Cambridge
We recently focused on predicting molecular properties using machine learning methods in the FS-Mol project. To further support the drug discovery process, we are also working on methods that can automatically design compounds that better fit project requirements than existing candidate compounds. This is an extremely difficult task, as only a few promising molecules exist in the vast and largely unexplored chemical space—estimated to contain up to 1060 drug-like molecules. Just how big is that number? It would be enough molecules to reproduce the Earth billions of times. Finding them requires creativity and intuition that cannot be captured by fixed rules or hand-designed algorithms. This is why learning is crucial not only for the predictive task, as done in FS-Mol, but also for the generative task of coming up with new structures.
In our earlier work, published at the 2018 Conference on Neural Information Processing Systems (NeurIPS), we described a generative model of molecules called CGVAE. While that model performed well on simple, synthetic tasks, we noted then that further improvements required the expertise of drug discovery specialists. In collaboration with experts at Novartis, we identified two issues limiting the applicability of the CGVAE model in real drug discovery projects: it cannot be naturally constrained to explore only molecules containing a particular substructure (called the scaffold), and it struggles to reproduce key structures, such as complex ring systems, due to its low-level, atom-by-atom generative procedure. To remove these limitations, we built MoLeR, which we describe in our new paper, “Learning to Extend Molecular Scaffolds with Structural Motifs,” published at the 2022 International Conference on Learning Representations (ICLR).
The MoLeR model
In the MoLeR model, we represent molecules as graphs, in which atoms appear as vertices that are connected by edges corresponding to the bonds. Our model is trained in the auto-encoder paradigm, meaning that it consists of an encoder—a graph neural network (GNN) that aims to compress an input molecule into a so-called latent code—and a decoder, which tries to reconstruct the original molecule from this code. As the decoder needs to decompress a short encoding into a graph of arbitrary size, we design the reconstruction process to be sequential. In each step, we extend a partially generated graph by adding new atoms or bonds. A crucial feature of our model is that the decoder makes predictions at each step solely based on a partial graph and a latent code, rather than in dependence on earlier predictions. We also train MoLeR to construct the same molecule in a variety of different orders, as the construction order is an arbitrary choice.
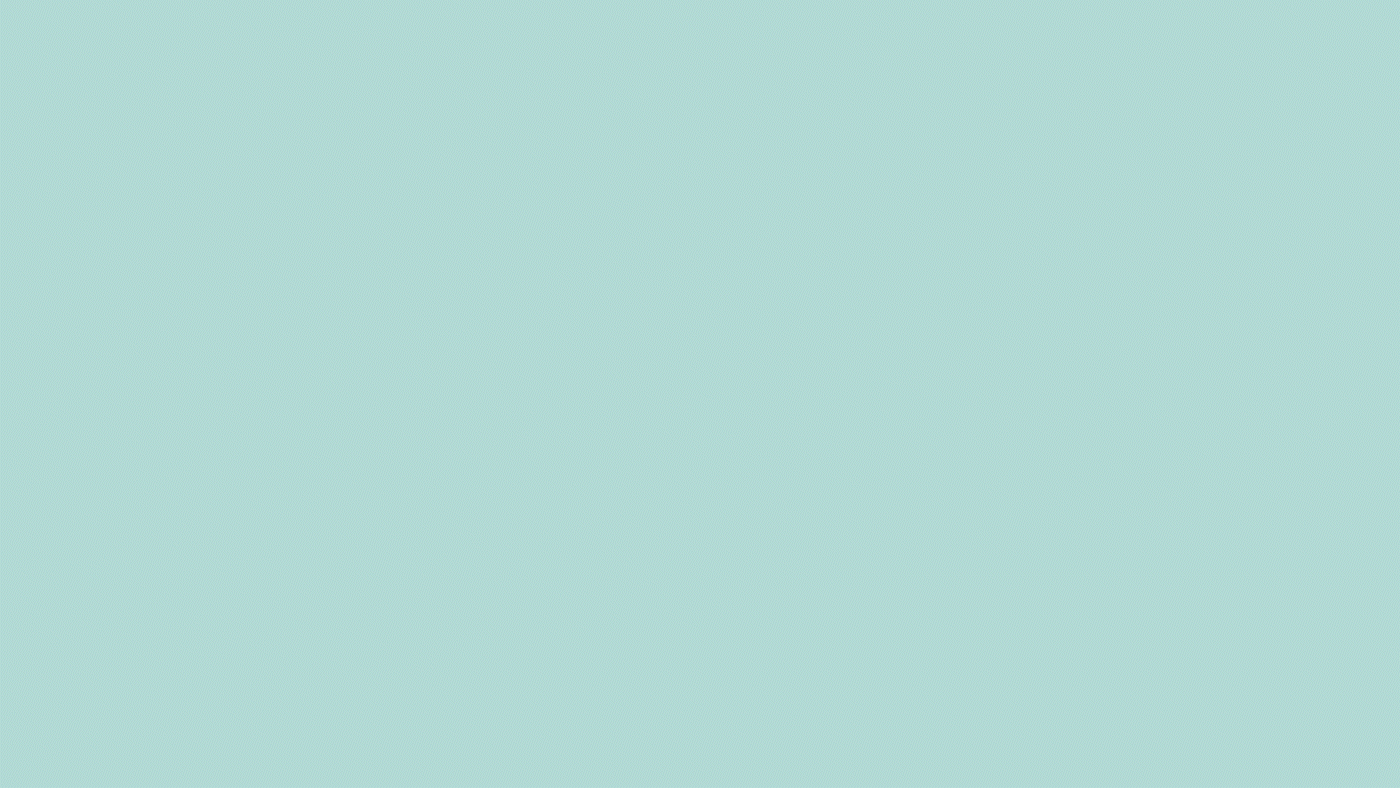
As we alluded to earlier, drug molecules are not random combinations of atoms. They tend to be composed of larger structural motifs, much like sentences in a natural language are compositions of words, and not random sequences of letters. Thus, unlike CGVAE, MoLeR first discovers these common building blocks from data, and is then trained to extend a partial molecule using entire motifs (rather than single atoms). Consequently, MoLeR not only needs fewer steps to construct drug-like molecules, but its generation procedure also occurs in steps that are more akin to the way chemists think about the construction of molecules.
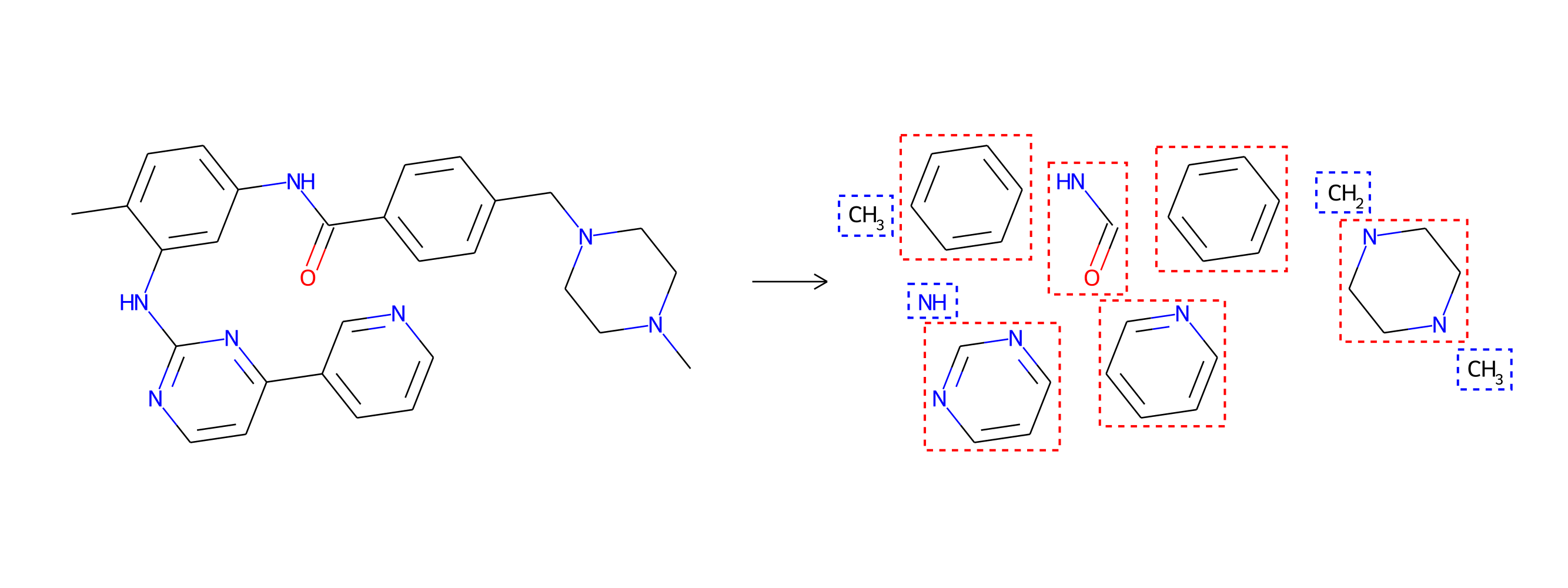
Drug-discovery projects often focus on a specific subset of the chemical space, by first defining a scaffold—a central part of the molecule that has already shown promising properties—and then exploring only those compounds that contain the scaffold as a subgraph. The design of MoLeR’s decoder allows us to seamlessly integrate an arbitrary scaffold by using it as an initial state in the decoding loop. As we randomize the generation order during training, MoLeR implicitly learns to complete arbitrary subgraphs, making it ideal for focused scaffold-based exploration.
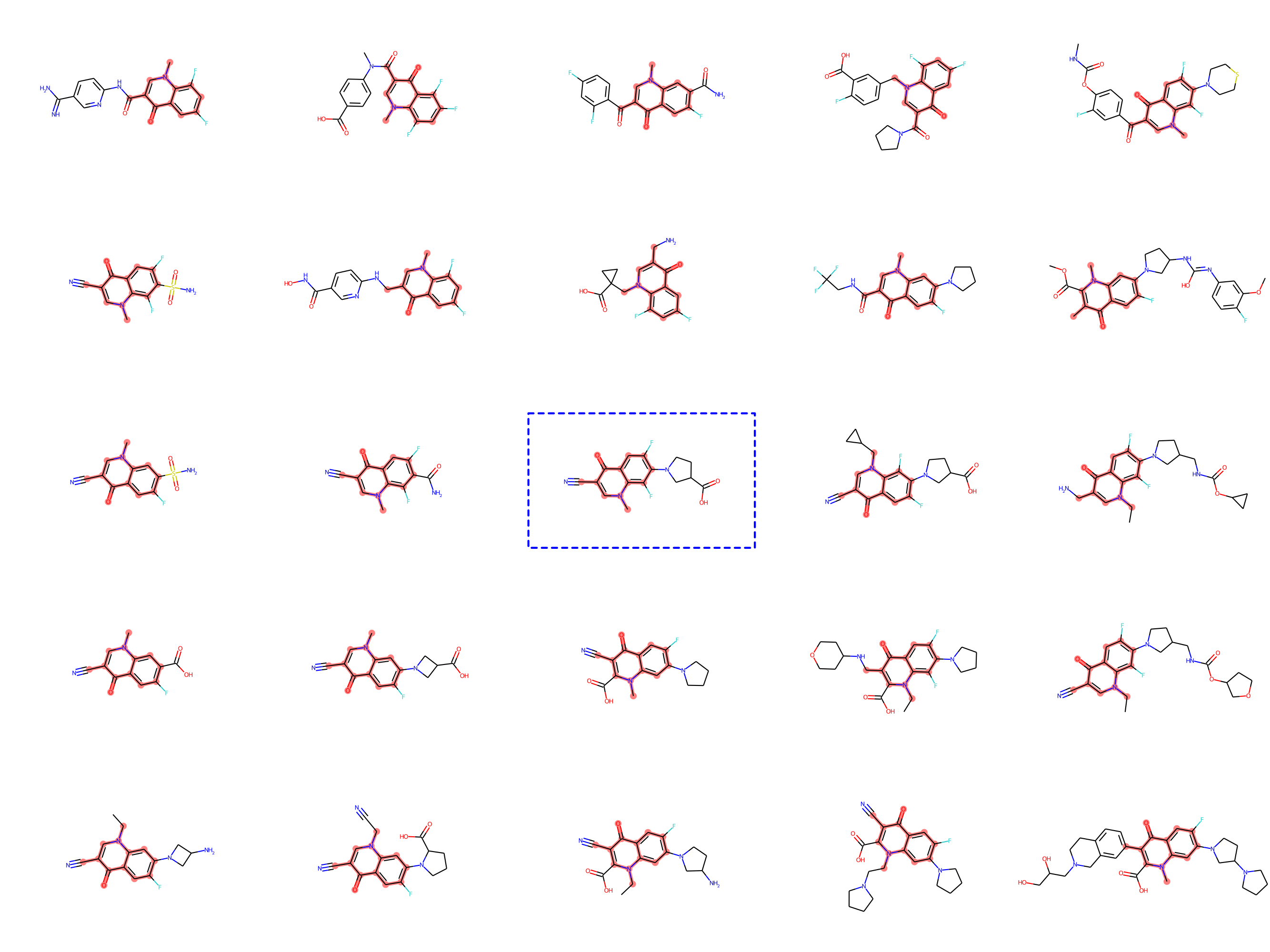
Optimization with MoLeR
Even after training our model as discussed above, MoLeR has no notion of “optimization” of molecules. However, like related approaches, we can perform optimization in the space of latent codes using an off-the-shelf black-box optimization algorithm. This was not possible with CGVAE, which used a much more complicated encoding of graphs. In our work, we opted for using Molecular Swarm Optimization (MSO), which shows state-of-the-art results for latent space optimization in other models, and indeed we found it to work very well for MoLeR. In particular, we evaluated optimization with MSO and MoLeR on new benchmark tasks that are similar to realistic drug discovery projects using large scaffolds and found this combination to outperform existing models.
Outlook
We continue to work with Novartis to focus machine learning research on problems relevant to the real-world drug discovery process. The early results are substantially better than those of competing methods, including our earlier CGVAE model. With time, we hope MoLeR-generated compounds will reach the final stages of drug-discovery projects, eventually contributing to new useful drugs that benefit humanity.
The post MoLeR: Creating a path to more efficient drug design appeared first on Microsoft Research.
PPE: A fast and provably efficient RL algorithm for exogenous noise

Picture a person walking in a park by a pond. The surrounding environment contains a number of moving objects that change the quality of the environment: clouds moving to hide the sun, altering the quality of light; ducks gliding across the pond, causing its surface to ripple; people walking along a path, their images reflecting on the water. If we’re creating an AI model for navigating to a given goal, for example, a robot navigating to a specific location in a park to deliver a package, we want this model to recognize the robot and any obstacle in its way, but not the changes in its surrounding environment that occur independently of the agent, which we define as exogenous noise.
Although reinforcement learning (RL) has proven to be a successful paradigm for training AI models in navigation tasks, often used in gaming, existing RL methods are not yet robust enough to handle exogenous noise. While they may be able to heuristically solve certain problems, such as helping a robot navigate to a specific destination in a particular environment, there is no guarantee that they can solve problems in environments they have not seen.
In this post, we introduce Path Predictive Elimination (PPE), the first RL algorithm that can solve the problem of exogenous noise with a mathematical guarantee. Specifically, for any problem that satisfies certain assumptions, the algorithm succeeds in solving the problem using a small number of episodes. We discuss this algorithm in detail in our paper, “Provable RL with Exogenous Distractors via Multistep Inverse Dynamics.”

Real-world RL and exogenous noise
To understand how PPE works, it’s important to first discuss how a real-world RL agent (the decision-maker) operates. Agents have an action space with (A) number of actions and receive information about the world in the form of an observation. In our example, the robot is the agent, and its action space contains four actions: a step forward, backward, left, or right.
After an agent takes a single action, it gets a new observation—that is, it receives more information about its environment—along with a reward. If the robot observes the park through a camera, the observation takes the form of an image. When an agent has a task to solve, such as reaching a specific destination, it must take a sequence of actions, each resulting in a reward. Its goal is to maximize the sum of rewards. When the robot takes a step forward, the camera generates a new observation of the park, and it receives a reward for this action. It may get a reward of 1 for the first action that takes it toward its goal and 0 otherwise.
Key challenges in real-world RL include how to handle complex observations and very large observation spaces. In our example, the robot in the park will have to work with an image that contains relevant information, such as the position of the destination, but this information is not directly accessible due to the exogenous noise and camera-generated image noise in the observation.
An image can be in a 500 x 500 x 3 pixel space, where each pixel takes 255 values. This would give us 255500 x 500 x 3 the number of different images which is an extremely large number of possibilities. However, the environment is much simpler to describe than this number suggests. This means the observation in an RL environment is generated from a much more compact but hidden endogenous state. In our park example, the endogenous state contains the position of the agent, the destination, and any obstacles around the agent.
In our paper, we assume that the endogenous state dynamics are near-deterministic. That is, taking a fixed action in an endogenous state always leads to the same next endogenous state in most cases. We also require that it is possible to extract the endogenous state from an observation. However, we make no assumptions about dynamics of exogenous noise or how observations are generated.
Most existing RL algorithms are either unable to solve problems containing complex observations or lack a mathematical guarantee for working on new, untried problems. This guarantee is desirable because the cost of failure in the real world can be potentially high. Many existing algorithms require an impractically large amount of data to succeed, requiring the agent to perform a large number of actions before it solves the task.
PPE takes an approach called hidden state decoding, where the agent learns a type of ML model called a decoder to extract the hidden endogenous state from an observation. It does this in a self-supervised manner, meaning it does not require a human to provide it with labels. For example, PPE can learn a decoder to extract the robot and any obstacle’s position in the park. PPE is the first provable algorithm that can extract the endogenous state and use it to perform RL efficiently.
Path Prediction and Elimination: An RL algorithm that is robust to exogenous noise
PPE is simple to implement and is fast to run. It works by learning a small set of paths that can take the agent to all possible endogenous states. The agent can technically consider all possible paths of length (h), enabling it to visit every endogenous state. However, as there are (A^h) possible paths of length (h), the number of paths will overwhelm the agent as (h) increases. The more paths the agent has to work with, the more data it needs to solve a given task. Ideally, if there are (S) number of endogenous states, we need just (S) number of paths, with only one unique path going to each endogenous state. PPE works by eliminating redundant paths that visit the same endogenous state by solving a novel self-supervised classification task.
PPE is similar in structure to the breadth-first search algorithm in that it runs a for-loop, where, in iteration (h) of the loop, the agent learns to visit all endogenous states that can be reached by taking (h) actions. At the start of iteration, the agent maintains a list of paths of length (h). This list has a path to visit every endogenous state that’s reachable after taking (h) actions. However, this list may also contain redundant paths, i.e., multiple paths that reach the same endogenous state. When this list is simply all paths of length 1, it corresponds to every action in the agent’s action space.
The top of Figure 2 shows agent’s initial list of paths, which contains at least three paths: ( pi_1), (pi_2), and (pi_3). The first two paths reach the same destination, denoted by the endogenous state (s_1). In contrast, the last path (pi_3) reaches a different endogenous state (s_2). Figure 2 shows a sampled observation (or image) for each endogenous state.
Because PPE wants to learn a small set of paths to visit all endogenous states, it seeks to eliminate the redundant paths by collecting a dataset of observations coupled with the path that was followed to observe them. In Figure 2, both (pi_1) or (pi_2) reach the same endogenous state, so one of them can be eliminated. This is done by randomly selecting a path in its list, following this path to the end, and saving the last observation. For example, our dataset can contain a tuple ((pi_1, x)) where (pi_1) is the policy in our list and (x) is the image in top-right of Figure 2. PPE collects a dataset of many such tuples.

PPE then solves a multiclass classification problem to predict the index of the path from the last observation. The index of a path is computed with respect to the original list. This classification problem can be solved with any appropriate model class, such as deep neural networks, using PyTorch, TensorFlow, or a library of your choice. If two different paths, (pi_1) and (pi_2), reach the same endogenous state, the learned classifier won’t be able to deterministically predict which path was used to visit observations from this state. That is, the learned classifier predicts a high probability for both paths given an observation from this endogenous state. PPE uses this confusion signal to eliminate one of these paths because both paths reach the same endogenous state. PPE also learns a decoder as a result solving the classification problem described above, which maps an observation to the index of the leftover path with the highest probability under the learned classifier.
At the end of iteration (h) of the for-loop, PPE will have found a list of leftover paths that includes a unique path for every endogenous state that’s reachable after taking (h) actions. It then expands these leftover paths to create the list for the next iteration of the for-loop. For every path that’s left over, PPE creates (A) number of new paths by concatenating every action to the end of the path. The for-loop then continues with the next iteration.
Note that the above steps of PPE can be computed even in the absence of rewards. The output of these steps, namely the decoder and the learned leftover paths, can be cached and used to optimize any reward functions provided later. We discuss various strategies to optimize any given reward function in our paper, including both model-free and model-based approaches.
Proof, experiment, and code
The paper also provides a mathematical proof that PPE efficiently solves a large class of RL problems. Using a small amount of data, it can accurately explore, find a policy that achieves maximum sum of rewards, recover a decoder that maps the observation to its hidden endogenous state, and recover the dynamics of the endogenous state with a high probability. We describe various experiments where PPE successfully performs these tasks in line with its mathematical guarantee and outperforms various prior methods.
This is illustrated in Figure 3. It depicts a visual grid-world where the agent’s goal is to navigate to the slice of pizza on the other side of the pond, populated by two ducks that move independently of agent’s actions and are the source of exogenous noise. The endogenous state will consist of the position of the agent. The figure shows what PPE is expected to do in this task. It will gradually learn longer paths that reach various endogenous states in the environment. It will also learn a decoder and use it to extract the dynamics of the latent endogenous state, shown on the right.
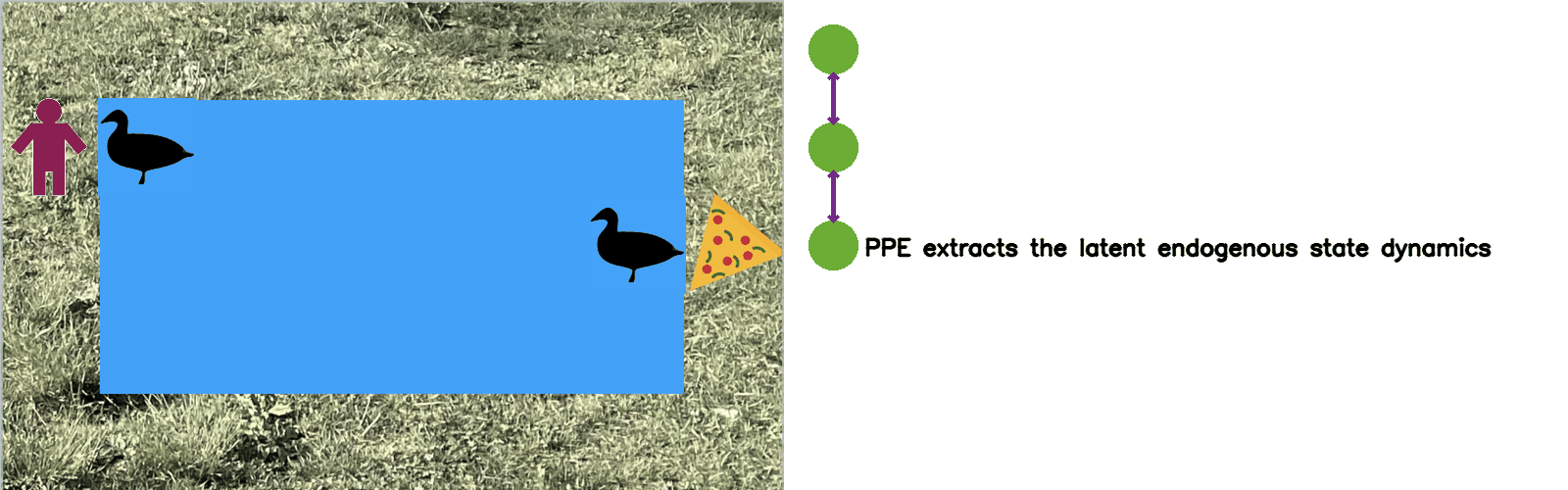
The road ahead
While PPE is the first RL algorithm that offers a mathematical guarantee in the presence of exogenous noise, there is still work to do before we can solve every RL problem that includes exogenous noise. Some of the unanswered questions that we are pursuing include:
- How can we eliminate the assumption that PPE makes, that latent endogenous state dynamics are near-deterministic?
- Can we extend PPE to work in nonepisodic settings, where the agent generates a single long episode?
- How does PPE perform on real-world problems?
- Can we make PPE a truly online algorithm, eliminating the need to collect large datasets before it improves?
RL algorithms hold great promise for improving applications in a diverse range of fields, from robotics, gaming, and software debugging, to healthcare. However, exogenous noise presents a serious challenge in unlocking the full potential of RL agents in the real world. We’re hopeful that PPE will motivate further research in RL in the presence of exogenous noise.
The post PPE: A fast and provably efficient RL algorithm for exogenous noise appeared first on Microsoft Research.
Don’t let data drift derail edge compute machine learning models

Edge computing has come of age, with deployments enabling many applications that process data from IoT sensors and cameras. In 2017, we identified the symbiotic relationship between edge computing and video analytics in an article, noting that live video analytics is the “killer app” for edge computing. Edge devices come in various shapes and sizes but are inherently resource-constrained relative to the cloud.
These resource constraints necessitate lightweight machine learning (ML) models at the edge. Using techniques for model specialization and compression, the community has obtained edge models whose compute and memory footprints are substantially lower (by 96x for object detector models). Such models are super amenable to deploy at the edge.
Smooth going so far, but the villain in the story is data drift! This is the phenomenon where the live data in the field diverges significantly from the initial training data. We achieved the phenomenally low compute footprints for edge models only because we specialized the models to be specific to the camera streams. But in the bargain, they lost their ability to generalize much beyond what they have seen during training. This lack of generality comes back to bite us when data drifts and accuracy of the models drop – by as much as 22% – when they are deployed in the field.
Ekya is a solution, developed with collaborators at University of California, Berkeley and University of Chicago, that addresses the problem of data drift on the edge compute box. Instead of sending video data to the cloud for periodic retraining of models, which is costly in its bandwidth usage and can raise privacy questions, Ekya enables both retraining and inference to co-exist on the edge box. For more details, take a look at our paper: Ekya: Continuous Learning of Video Analytics Models on Edge Compute Servers, which has been published at NSDI 2022. We are excited to release the code for Ekya as well.
Not only can you use the code to reproduce all experiments in our paper, we also hope that the code can help you easily build a continuous learning system for your edge deployment. Oh, and one more thing—we are also pointing to the raw video datasets released by the City of Bellevue. This includes 101 hours of video from five traffic intersections, all of which have also been labeled with our golden YOLOv3 model. We hope that the videos from the City of Bellevue as well as the other datasets included in the repository will aid in the building of new edge models as well as improving our pre-trained specialized models to significantly advance the state of the art.
Please reach out to Ganesh Ananthanarayanan with any questions.
Explore More
-
Abstract
Bellevue, Microsoft, UW team up to prevent traffic deaths
Leading Pedestrian Intervals – Yay or Nay? A Before-After Evaluation using Traffic Conflict-Based Peak Over Threshold Approach
-
Video
Video Analytics for Smart Cities
Microsoft Research has an on-going pilot in Bellevue, Washington for active traffic monitoring of traffic intersections live 24X7. This project is focused on is video streams from cameras at traffic intersections. Traffic-related accidents are among the top 10 reasons […]
The post Don’t let data drift derail edge compute machine learning models appeared first on Microsoft Research.
Just Tech: Centering Community-Driven Innovation at the Margins Episode 3 with Dr. Sasha Costanza-Chock

Episode 135 | April 13, 2022
In “Just Tech: Centering Community-Driven Innovation at the Margins,” Senior Principal Researcher Mary L. Gray explores how technology and community intertwine and the role technology can play in supporting community-driven innovation and community-based organizations. Dr. Gray and her team are working to bring computer science, engineering, social science, and communities together to boost societal resilience in ongoing work with Project Resolve. She’ll talk with organizers, academics, technology leaders, and activists to understand how to develop tools and frameworks of support alongside members of these communities.
In this episode of the series, Dr. Gray and Dr. Sasha Costanza-Chock, scholar, designer, and activist, explore design justice, a framework for analyzing design’s power to perpetuate—or take down—structural inequality and a community of practice dedicated to creating a more equitable and sustainable world through inclusive, thoughtful, and respectful design processes. They also discuss how critical thinkers and makers from social movements have influenced technology design and science and technology studies (STS), how challenging the assumptions that drive who tech is built for will create better experiences for most of the planet, and how a deck of tarot-inspired cards is encouraging radically wonderful sociotechnical futures.
Learn more:
- Design Justice: Community-Led Practices to Build the Worlds We Need
- Algorithmic Justice League
- Coded Bias documentary
- “Bug Bounties for Algorithmic Harms? Lessons from cybersecurity vulnerability disclosure for algorithmic harms discovery, disclosure, and redress”
- The Oracle for Transfeminist Technologies card deck
Subscribe to the Microsoft Research Podcast:
iTunes | Email | Android | Spotify | RSS feed
Transcript
[MUSIC PLAYS UNDER DIALOGUE]
MARY GRAY: Welcome to the Microsoft Research Podcast series “Just Tech: Centering Community-Driven Innovation at the Margins.” I’m Mary Gray, a Senior Principal Researcher at our New England lab in Cambridge, Massachusetts. I use my training as an anthropologist and communication media scholar to study people’s everyday uses of technology. In March 2020, I took all that I’d learned about app-driven services that deliver everything from groceries to telehealth to study how a coalition of community-based organizations in North Carolina might develop better tech to deliver the basic needs and health support to those hit hardest by the pandemic. Our research together, called Project Resolve, aims to create a new approach to community-driven innovation—one that brings computer science, engineering, the social sciences, and community expertise together to accelerate the roles that communities and technologies could play in boosting societal resilience. For this podcast, I’ll be talking with researchers, activists, and nonprofit leaders about the promises and challenges of what it means to build technology with rather than for society.
[MUSIC ENDS]
My guest for this episode is Dr. Sasha Costanza-Chock, a researcher, activist, and designer who works to support community-led processes that build shared power, dismantle the matrix of domination, and advance ecological survival. They are the director of research and design at Algorithmic Justice League, a faculty associate with the Berkman Klein Center for Internet & Society at Harvard University, and a member of the steering committee of the Design Justice Network. Sasha’s most recent book, Design Justice: Community-Led Practices to Build the Worlds We Need, was recently a 2021 Engineering and Technology PROSE Award finalist and has been cited widely across disciplines. Welcome, Sasha.
SASHA COSTANZA-CHOCK: Thanks, Mary. I’m excited to be here.
GRAY: Can you tell us a little bit about how you define design justice?
COSTANZA-CHOCK: Design justice is a term—you know, I didn’t create this term; it comes out of a community of practice called the Design Justice Network. But I have kind of chronicled the emergence of this community of practice and some of the ways of thinking about design and power and technology that have sort of come out of that community. And I’ve also done some work sort of tracing the history of different ways that people have thought about design and social justice, really. So, in the book, I did offer a tentative definition, kind of a two-part definition. So, on the one hand, design justice is a framework for analysis about how design distributes benefits and burdens between various groups of people. And in particular, design justice is a way to focus explicitly on the ways that design can reproduce or challenge the matrix of domination, which is Patricia Hill Collins’ term for white supremacy, heteropatriarchy, capitalism, ableism, settler colonialism, and other forms of structural inequality. And also, design justice is a growing community of practice of people who are focused on ensuring more equitable distribution of design’s benefits and burdens, more meaningful participation in design decisions and processes, and also recognition of already existing, community-based, Indigenous, and diasporic design traditions and knowledge and practices.
GRAY: Yeah. What are those disciplines we’re missing when we think about building and building for and with justice at the center of our attention?
COSTANZA-CHOCK: It’s interesting. I think for me, um, so design and technology design in particular, I think, for me, practice came first. So, you know, learning the basics of how to code, building websites, working with the Indymedia network. Indymedia was a kind of global network of hackers and activists and social movement networks who leveraged the power of what was then the nascent internet, um, to try and create a globalized news network for social movements. I became a project manager for various open-source projects for a while. I had a lot of side gigs along my educational pathway. So that was sort of more sort of practice. So, that’s where I learned, you know, how do you run a software project? How do you motivate and organize people? I came later to reading about and learning more about sort of that long history of design theory and history. And then, sort of technology design stuff, I was always looking at it along the way, but started diving deeper more recently. So, my—my first job after my doctorate was, you know, I—I received a position at MIT. Um, and so I came to MIT to the comparative media studies department, set up my collaborative design studio, and I would say, yeah, at MIT, I became more exposed to the HCI literature, spent more time reading STS work, and, in particular, was drawn to feminist science and technology studies. You know, MIT’s a very alienating place in a lot of ways and there’s a small but excellent, you know, community of scholars there who take, you know, various types of critical approaches to thinking about technology design and development and—and sort of the histories of—of technology and sociotechnical systems. And so, kind of through that period, from 2011 up until now, I spent more time engaging with—with that work, and yeah, got really inspired by feminist STS. I also—parallel to my academic formation and training—was always reading theory and various types of writing from within social movement circles, stuff that sometimes is published in academic presses or in peer-review journals and sometimes totally isn’t, but, to me, is often equally or even more valuable if you’re interested in theorizing social movement activity than the stuff that comes sort of primarily from the academy or from social movement studies as a subfield of sociology.
GRAY: Mm-hmm.
COSTANZA-CHOCK: Um, so I was like, you know, always reading all kinds of stuff that I thought was really exciting that came out of movements. So, reading everything that AK Press publishes, reading stuff from Autonomia, and sort of the—the Italian sort of autonomous Marxist tradition. But also in terms of pedagogy, I’m a big fan of Freire. And I didn’t encounter Freire through the academy; it was through, you know, community organizing work. So, community organizers that I was connected to were all reading Freire and reading other sort of critical and radical thinkers and scholars.
GRAY: So, wait. Hold the phone.
COSTANZA-CHOCK: OK. [LAUGHS]
GRAY: You didn’t actually—I mean, there wasn’t a class where Pedagogy of the Oppressed was taught in your training? I’m just, now, am like “Really?” That’s—
COSTANZA-CHOCK: I don’t think so. Yeah.
GRAY: Wow.
COSTANZA-CHOCK: Yeah, because I didn’t have formal training in education. It was certainly referenced, but the place where I did, you know, study group on it was in movement spaces, not in the academy. Same with bell hooks. I mean, bell hooks, there would be, like, the occasional essay in, like—I did undergraduate cultural studies stuff. Marjorie Garber, you know, I think—
GRAY: Yeah.
COSTANZA-CHOCK: had like an essay or two on her syllabus, um—
GRAY: Yeah.
COSTANZA-CHOCK: —of bell hooks. Um so, I remember encountering bell hooks early on, but reading more of her work came later and through movement spaces. And so, then, what I didn’t see was a lot of people—although, increasingly now, I think this is happening—you know, putting that work into dialogue with design studies and with science and technology studies. And so, that’s what I—that’s what I get really excited by, is the evolution of that.
GRAY: And—and maybe to that point, I feel like you have, dare I say, “mainstreamed” Patricia Hill Collins in computer science and engineering circles that I travel. Like, to hear colleagues say “the matrix of domination,” they’re reading it through you, which is wonderful. They’re reading—they’re reading what that means. And design justice really puts front and center this critical approach. Can you tell us about how you came to that framework and put it in the center of your work for design justice?
COSTANZA-CHOCK: Patricia Hill Collins develops the term in the ’90s. Um, the “matrix of domination” is her phrase. Um, she elaborates on it in, you know, her text, uh, Black Feminist Thought. And of course, she’s the past president of the American Sociological Association. Towering figure, um, in some fields, but, you know, maybe not as much in computer science and HCI, and other, you know, related fields. But I think unjustly so. And so, part of what I’m really trying to do at the core of the Design Justice book was put insights from her and other Black feminist thinkers and other critical scholars in dialogue with some core, for me, in particular, HCI concepts, um, although I think it does, you know, go broader than that. The matrix of domination was really useful to me when I was learning to think about power and resistance, how does power and privilege operate. You know, this is a concept that says you can’t only think about one axis of inequality at a time. You can’t just talk about race or just talk about gender—you can’t just talk about class—because they operate together. Of course, another key term that connects with matrix of domination is “intersectionality” from Kimberlé Crenshaw. She talks about it in the context of legal theory, where she’s looking at how the legal system is not set up to actually protect people who bear the brunt of oppression. And she talks about these, you know, classic cases where Black women can’t claim discrimination under the law at a company which defends itself by saying, “Well, we’ve hired Black people.” And what they mean is they’ve hired some Black men. And they say, “And we’ve also hired women.” But they mean white women. And so, it’s not legally actionable. The Black women have no standing or claim to discrimination because Black women aren’t protected under anti-discrimination law in the United States of America. And so that is sort of like a grounding that leads to this, you know, the conversation. The matrix of domination is an allied concept. And to me, it’s just incredibly useful because I thought that it could translate well, in some ways, into technical fields because there’s a geometry and there’s a mental picture. There’s an image that it’s relatively easy to generate for engineers, I think, of saying, “OK, well, OK, your x-axis is class. [LAUGHS] Your y-axis is gender. Your z-axis is race. This is a field. And somewhere within that, you’re located. And also, everyone is located somewhere in there, and where you’re located has an influence on how difficult the climb is.” And so when we’re designing technologies—and whether it’s, you know, interface design, or it’s an automated decision system—you know, you have to think about if this matrix is set up to unequally distribute, through its topography, burdens and benefits to different types of people depending on how they are located in this matrix, at this intersection. Is that correct? You know, do you want to keep doing that, or do you want to change it up so that it’s more equitable? And I think that that’s been a very useful and powerful concept. And I think, for me, part of it maybe did come through pedagogy. You know, I was teaching MIT undergraduates—most of them are majoring in computer science these days—and so I had to find ways to get them to think about power using conceptual language that they could connect with, and I found that this resonated.
GRAY: Yeah. And since the book has come out—and I, you know, it’s been received by many different scholarly communities and activist communities—has your own definition of design justice changed at—at all? Or even the ways you think about that matrix?
COSTANZA-CHOCK: That’s a great question. I think that one of the things that happened for me in the process of writing the book is I went a lot deeper into reading and listening and thinking more about disability and how crucial, you know, disability and ableism are, how important they are as sort of axes of power and resistance, also as sources of knowledge. So, like, disability justice and disabled communities of various kinds being key places for innovation, both of devices and tools and also of processes of care. And just, there’s so much phenomenal sort of work that’s coming, you know, through the disability justice lens that I really was influenced by in the writing of the book.
GRAY: So another term that seems central in the book is “codesign.” And I think for many folks listening, they might already have an idea of what that is. But can you say a bit more about what you mean by codesign, and just how that term relates to design justice for you?
COSTANZA-CHOCK: I mean, to be entirely honest with you, I think that when I arrived at MIT, I was sort of casting around for a term that I could use to frame a studio course that I wanted to set up that would both signal what the approach was going to be while also being palatable to the administration and not scaring people away. Um, and so I settled on “codesign” as a term that felt really friendly and inclusive and was a broad enough umbrella to enable the types of partnerships with community-based organizations and social movement groups, um, that I wanted to provide scaffolding for in that class. It’s not that I think “codesign” is bad. You know, there’s a whole rich history of writing and thinking and practice, you know, in codesign. I think I just worry that like so many things—I don’t know if it’s that the term is loose enough that it allows for certain types of design practices that I don’t really believe in or support or that I’m critical of or if it’s just that it started meaning more of one thing, um, and then, over time, it became adopted—as many things do become adopted—um, by the broader logics of multinational capitalist design firms and their clients. But I don’t necessarily use the term that much in my own practice anymore.
GRAY: I want to understand what you felt was useful about that term when you first started applying it to your own work and why you’ve moved away from it. What are good examples of, for you, a practice of codesign that stays committed to design justice, and what are some examples of what worries you about the ambiguity of what’s expected of somebody doing codesign?
COSTANZA-CHOCK: So, I mean, there—there’s lots of terms in, like, a related conceptual space, right? So, there’s codesign, participatory design, human-centered design, design justice. I think if we really get into it, each has its own history and sort of there are conferences associated with each. There are institutions connected to each. And there are internal debates within those communities about, you know, what counts and what doesn’t. I think, for me, you know, codesign remains broad enough to include both what I would consider to be sort of design justice practice, where, you know, a community is actually leading the process and people with different types of design and engineering skills might be supporting or responding to that community leadership. But it’s also broad enough to include what I call in the book, you know, more extractive design processes, where what happens is, you know, typically a design shop or consultant working for a multinational brand parachutes into a place, a community, a group of people, runs some design workshops, maybe—maybe does some observation, maybe does some focus groups, generates a whole bunch of ideas about the types of products or product changes that people would like to see, and then gathers that information and extracts it from that community, brings it back to headquarters, and then maybe there are some product changes or some new features or a rollout of something new that gets marketed back to people. And so in that modality, you know, some people might call an extractive process where you’re just doing one or a few workshops with people “codesign” because you have community collaborators, you have community input of some kind; you’re not only sitting in the lab making something. But the community participation is what I would call thin. It’s potentially extractive. The benefit may be minimal to the people who have been involved in that process. And most of the benefits accrue back either to the design shop that’s getting paid really well to do this or ultimately back to headquarters—to the brand that decided to sort of initiate the process. And I’m interested in critiquing extractive processes, but I’m most interested in trying to learn from people who are trying to do something different, people who are already in practice saying, “I don’t want to just be doing knowledge extraction. I want to think about how my practice can contribute to a more just and equitable and sustainable world.” And in some ways, people are, you know, figuring it out as we go along, right? Um, but I’m trying to be attentive to people trying to create other types of processes that mirror, in the process, the kinds of worlds that we want to create.
GRAY: So, it seems like one of the challenges that you bring up in the book is precisely design at—at some point is thinking about particular people and particular—often referred to as “users’”— journeys. And I wanted to—to step back and ask you, you know, you note in the book that there’s a—a default in design that tends to think about the “unmarked user.” And I’m quoting you here. That’s a “(cis)male, white, heterosexual, ‘able-bodied,’ literate, college educated, not a young child, not elderly.” Definitely, they have broadband access. They’ve got a smartphone. Um, maybe they have a personal jet, I don’t know. That part was not a quote of you. [LAUGHTER] But, you know, you’re really clear that there’s this—this default, this presumed user, ubiquitous user. Um, what are the limits for you to designing for an unmarked user, but then how do you contend with this thinking so specifically about people can also be quite, to your earlier point about intersectionality, quite flattening?
COSTANZA-CHOCK: Well, I think the unmarked user is a really well-known and well-documented problem. Unfortunately, it often, it—it applies—you don’t have to be a member of all those categories as an unmarked user to design for the unmarked user when you’re in sort of a professional design context. And that’s for a lot of different reasons that we don’t have that much time to get into, but basically hegemony. [LAUGHTER] So, um—and the problem with that—like, there’s lots of problems with that—one is that it means that we’re organizing so much time and energy and effort in all of our processes to kind of, like, design and build everything from, you know, industrial design and new sort of, you know, objects to interface design to service design, and, you know, if we build everything for the already most privileged group of people in the world, then the matrix of domination just kind of continues to perpetuate itself. Then we don’t move the world towards a more equitable place. And we create bad experiences, frankly, for the majority of people on the planet. Because the majority of people on planet Earth don’t belong to that sort of default, unmarked user that’s hegemonic. Most people on planet Earth aren’t white; they’re actually not cis men. Um, at some point most people on planet Earth will be disabled or will have an impairment. They may not identify as Disabled, capital D. Most people on planet Earth aren’t college educated. Um, and so on and so forth. So, we’re really excluding the majority of people if we don’t actively and regularly challenge the assumption of who we should be building things for.
GRAY: So, what do you say to the argument that, “Well, tech companies, those folks who are building, they just need to hire more diverse engineers, diverse designers—they need a different set of people at the table—and then they’ll absolutely be able to anticipate what a—a broader range of humanity needs, what more people on Earth might need.”
COSTANZA-CHOCK: I think this is a “yes, and” answer. So, absolutely, tech companies [LAUGHS] need to hire more diverse engineers, designers, CEOs; investors need to be more diverse, et cetera, et cetera, et cetera. You know, the tech industry still has pretty terrible statistics, and the further you go up the corporate hierarchy, the worse it gets. So that absolutely needs to change, and unfortunately, right now, it’s just, you know, every few years, everyone puts out their diversity numbers. There’s a slow crawl sometimes towards improvement; sometimes it backslides. But we’re not seeing the shifts that we—we need to see, so it’s like hiring, retention, promotion, everything. I am a huge fan of all those things. They do need to happen. And a—a much more diverse and inclusive tech industry will create more diverse and inclusive products. I wouldn’t say that’s not true. I just don’t think that employment diversity is enough to get us towards an equitable, just, and ecologically sustainable planet. And the reason why is because the entire tech industry right now is organized around the capitalist system. And unfortunately, the capitalist system is a resource-extractive system, which is acting as if we have infinite resources on a finite planet. And so, we’re just continually producing more stuff and more things and building more server farms and creating more energy-intensive products and software tools and machine learning models and so on and so on and so on. So at some point, we’re going to have to figure out a way to organize our economic system in a way that’s not going to destroy the planet and result in the end of homo sapiens sapiens along with most of the other species on the planet. And so unfortunately, employment diversity within multicultural, neoliberal capitalism will not address that problem.
GRAY: I could not agree more. And I don’t want this conversation to end. I really hope you’ll come back and join me for another conversation, Sasha. It’s been unbelievable to be able to spend even a little bit of time with you. So, thank you for—for sharing your thoughts with us today.
COSTANZA-CHOCK: Well, thank you so much for having me. I always enjoy talking with you, Mary. And I hope that, yeah, we’ll continue this either in a podcast or just over a cup of tea.
[MUSIC PLAYS UNDER DIALOGUE]
GRAY: Looking forward to it. And as always, thanks to our listeners for tuning in. If you’d like to learn more—wait, wait, wait, wait! There’s just so much to talk about. [MUSIC IS WARPED AND ENDS] Not long after our initial conversation, Sasha said she was willing to have more discussion. Sasha, thanks for rejoining us.
COSTANZA-CHOCK: Of course. It’s always a pleasure to talk with you, Mary.
GRAY: In our first conversation, we had a chance to explore design justice as a framework and a practice and your book of the same name, which has inspired many. I’d love to know how your experience in design justice informs your current role with the Algorithmic Justice League.
COSTANZA-CHOCK: So I am currently the director of research and design at the Algorithmic Justice League. The Algorithmic Justice League, or AJL for short, is an organization that was founded by Dr. Joy Buolamwini, and our mission is to raise awareness about the impacts of AI, equip advocates with empirical research, build the voice and choice of the most impacted communities, and galvanize researchers, policymakers, and industry practitioners to mitigate AI harms and biases, and so we like to talk about how we’re building a movement to shift the AI ecosystem towards more equitable and accountable AI. And my role in AJL is to lead up our research efforts and also, at the moment, product design. Uh, we’re a small team. We’re sort of in start-up mode. Uh, we’re hiring various, you know, director-level roles and building out the teams that are responsible for different functions, and so it’s a very exciting time to be part of the organization. I’m very proud of the work that we’re doing.
GRAY: So you have both product design and research happening under the same roof in what sounds like a super-hero setting. That’s what we should take away—and that you’re hiring. I think listeners need to hear that. How do you keep research and product design happening in a setting that usually you have to pick one or the other in a nonprofit. How are you making those come together?
COSTANZA-CHOCK: Well, to be honest, most nonprofits don’t really have a product design arm. I mean, there are some that do, but it’s not necessarily a standard, you know, practice. I think what we are trying to do, though, as an organization—you know, we’re very uniquely positioned because we play a storytelling role, and so we’re influencing the public conversation about bias and harms in algorithmic decision systems, and probably the most visible place that that, you know, has happened is in the film Coded Bias. It premiered at Sundance, then it aired on PBS, and it’s now available on Netflix, and that film follows Dr. Buolamwini’s journey from, you know, a grad student at the MIT Media Lab who has an experience of facial recognition technology basically failing on her dark skin, and it follows her journey as she learns more about how the technology works, how it was trained, why it’s failing, and ultimately is then sort of, you know, testifying in U.S. Congress about the way that these tools are systematically biased against women and people with darker skin tones, skin types, and also against trans and gender nonconforming people, and that these tools should not be deployed in production environments, especially where it’s going to cause significant impacts to people’s lives. Over the past couple years, we’ve seen a lot of real-world examples of the harms that facial recognition technologies, or FRTs, can create. These types of bias and harm are happening constantly not only in facial recognition technologies but in automated decision systems of many different kinds, and there are so many scholars and advocacy organizations and, um, community groups that are now kind of emerging to make that more visible and to organize to try and block the deployment of systems when they’re really harmful or at the very least try and ensure that there’s more community oversight of these tools and also to set some standards in place, best practices, external auditing and impact assessment so that especially as public agencies start to purchase these systems and roll them out, you know, we have oversight and accountability.
GRAY: So, April 15 is around the corner, Tax Day, and there was a recent bit of news around what seems like a harmless use of technology and use of identification for taxes that you very much, um, along with other activists and organizations, uh, brought public attention to the concerns over sharing IDs as a part of our—of our tax process. Can you just tell the audience a little bit about what happened, and what did you stop?
COSTANZA-CHOCK: Absolutely. So, um, ID.me is a, uh, private company that sells identity verification services, and they have a number of different ways that they do identity verification, including, uh, facial recognition technology where they compare basically a live video or selfie to a picture ID that’s previously been uploaded and stored in the system. They managed to secure contracts with many government agencies, including a number of federal agencies and about 30 state agencies, as well. And a few weeks ago, it came out that the IRS had given a contract to ID.me and that people were going to have to scan our faces to access our tax records. Now, the problem with this—there are a lot of problems with this, but one of the problems is that we know that facial recognition technology is systematically biased against some groups of people who are protected by the Civil Rights Act, so, uh, against Black people and people with darker skin tones in general, uh, against women, and the systems perform least well on darker skinned type women. And so what this means is that if you’re, say, a Black woman or if you’re a trans person, it would be more likely that the verification process would fail for you in a way that is very systematic and has—you know, we have pretty good documentation about the failure rates, both in false positives and false negatives. The best science shows that these tools are systematically biased against some people, and so for it to be deployed in contracts by a public agency for something that’s going to affect everybody in the United States of America and is going to affect Black people and Black women specifically most, uh, is really, really problematic and opens the ground to civil rights lawsuits, to Federal Trade Commission action, among a number of other, you know, possible problems. So when we at the Algorithmic Justice League learned that ID.me had this partnership with the IRS and that this was all going to roll out in advance of this year’s tax season, uh, we thought this is really a problem and maybe this is something that we could move the needle on, and so we got together with a whole bunch of other organizations like Fight for the Future and the Electronic Privacy Information Center, and basically, all of these organizations started working with all cylinders firing, including public campaigns, op-eds, social media, and back channeling to various people who work inside different agencies in the federal government like the White House Office of Science and Technology Policy, the Federal Trade Commission, other contacts that we have in different agencies kind of saying, “Did you know that this system—this multi-million-dollar contract for verification that the IRS is about to unleash on all taxpayers—is known to have outcomes that disproportionately disadvantage Black people and women and trans and gender nonconforming people?” And in a nutshell, it worked to a degree. So the IRS announced that they would not be using the facial recognition verification option that ID.me offers, and a number of other federal agencies announced that they would be looking more closely at the contracts and exploring whether they wanted to actually roll this out, and what’s happening now is that at the state level through public records requests and other actions, um, you know, different organizations are now looking state by state and finding and turning up all these examples of how this same tool was used to basically deny access to unemployment benefits for people, to deny access to services for veterans. There are now, I think, around 700 documented examples that came from public records requests of people saying that they tried to verify their access, um, especially to unemployment benefits using the ID.me service, and they could not verify, and when they were told to take the backup option, which is to talk with a live agent, the company, you know, was rolling out this system with contracts so quickly that they hadn’t built up their human workforce, so when people’s automated verification was failing, there were these extremely long wait times like weeks or, in some cases, months for people to try and get verified.
GRAY: Well, and I mean, this is—I feel like the past always comes back to haunt us, right, because we have so many cases where it’s, in hindsight, seems really obvious that we’re going to have a system that will fail because of the training data that might have created the model. We are seeing so many cases where training datasets that have been the tried-and-true standards are now being taken off the shelf because we can tell that there are too many errors and too few theories to understand the models we have to keep using the same models the same way that we have used them in the past, and I’m wondering what you make of this continued desire to keep reaching for the training data and pouring more data in or seeing some way to offset the bias. What’s the value of looking for the bias versus setting up guardrails for where we apply a decision-making system in the first place?
COSTANZA-CHOCK: Sure. I mean, I think—let me start by saying that I do think it’s useful and valuable for people to do research to try and better understand the ways in which automated decision systems are biased, the different points in the life cycle where bias creeps in. And I do think it’s useful and valuable for people to look at bias and try and reduce it. And also, that’s not the be all and end all, and at the Algorithmic Justice League, we are really trying to get people to shift the conversation from bias to harm because bias is one but not the only way that algorithmic systems can be harmful to people. So a good example of that would be, we could talk about recidivism risk prediction, which there’s been a lot of attention to that, you know, ever since the—the ProPublica articles and the analysis of—that’s come out about, uh, COMPAS, which is, you know, the scoring system that’s used when people are being detained pre-trial and a court is making a decision about whether the person should be allowed out on bail or whether they should be detained until their trial. And these risk scoring tools, it turns out that they’re systematically biased against Black people, and they tend to overpredict the rate at which Black people will recidivate or will—will re-offend during the, you know, the period that they’re out and underpredict the rate at which white people, you know, would do so. So there’s one strand of researchers and advocates who would say, “Well, we need to make this better. We need to fix that system, and it should be less biased, and we want a system that more perfectly—more perfectly does prediction and also more equitably distributes both false positives and false negatives.” You can’t actually maximize both of those things. You kind of have to make difficult decisions about do you want it to, um, have more false positives or more false negatives. You have to sort of make decisions about that. But then there’s a whole nother strand of people like, you know, the Carceral Technology Resistance Network, who would just say, “Hold on a minute. Why are we talking about reducing bias in a pre-trial detention risk-scoring tool? We should be talking about why are we locking people up at all, and especially why are we locking people up before they’ve been sentenced for anything?” So rather than saying let’s build a better tool that can help us, you know, manage pre-trial detention, we should just be saying we should absolutely minimize pre-trial detention to only the most extreme cases that—where there’s clear evidence and a clear, you know, present danger that the person will immediately be harming themselves or—or—or someone else, and that should be something that, you know, a judge can decide without the need of a risk score.
GRAY: When you’re describing the consequences of a false positive or a false negative, I’m struck by, um, how cold the calculation can sound, and then when I think about the implications, you’re saying we have to decide do we let more people we might suspect could create harms leave a courtroom or put in jail people we could not possibly know how many more of them would not versus would commit some kind of act between now and when they’re sentenced. And so, I’m just really struck by the weightiness of that, uh, if I was trying to think about developing a technology that was going to try and reduce that harm and deliberate which is more harmful. I’m just saying that out loud because I—I feel like those are those moments where I see two strands of works you’re calling out and two strands of work you’re pointing out that sometimes do seem in fundamental tension, right? That we would not want to build systems that perpetuate an approach that tries to take a better guess at whether to retain someone before they’ve been convicted of anything.
COSTANZA-CHOCK: Yeah, so I think, like, in certain cases, like in criminal, you know, in the criminal legal system, you know, we want to sort of step out from the question that’s posed to us, where people are saying, “Well, what approach should we use to make this tool less biased or even less harmful,” if they’re using that frame. And we want to step back and say, “Well, what are the other things that we need to invest in to ensure that we can minimize the number of people who are being locked up in cages?” Because that’s clearly a horrible thing to do to people, and it’s not making us safer or happier or better, and it’s systematically and disproportionately deployed against people of color. In other domains, it’s very different, and this is why I think, you know, it can be very tricky. We don’t want to collapse the conversation about AI and algorithmic decision systems, and there are some things that we can say, you know, at a very high level about these tools, but at the end of the day, a lot of the times, I think that it comes down to the specific domain and context and tool that we’re talking about. So then we could say, well, let’s look at another field like dermatology, right? And you would say, well, there’s a whole bunch of researchers working hard to try and develop better diagnostic tools for skin conditions, early detection of cancer. And so it turns out that the existing datasets of skin conditions heavily undersample the wide diversity of human skin types that are out there in the world and overrepresent white skin, and so these tools perform way better, um, you know, for people who are, uh, raced as white, uh, under the current, you know, logic of the construction of—of racial identities. So there’s a case where we could say, “Well, yeah, here inclusion makes sense.” Not everybody would say this, but a lot of us would say this is a case where it is a good idea to say, “Well, what we need to do is go out and create much better, far more inclusive datasets of various skin conditions across many different skin types, you know, should be people from all across the world and different climates and locations and skin types and conditions, and we should better train these diagnostic tools, which potentially could really both democratize access to, you know, dermatology diagnostics and could also help with earlier detection of, you know, skin conditions that people could take action on, you know. Now, we could step out of that logic for a moment and say, “Well, no, what we should really do is make sure that there’s enough resources so that there are dermatologists in every community that people can easily see for free because they’re always going to do, you know, a better job than, you know, these apps could ever do,” and I wouldn’t disagree with that statement, and also, to me, this is a case where that’s a “both/and” proposition, you know. If we have apps that people can use to do self-diagnostic and if they reach a certain threshold of accuracy and they’re equitable across different skin types, then that could really save a lot of people’s lives, um, and then in the longer run, yes, we need to dramatically overhaul our—our medical system and so on and so forth. But I don’t think that those goals are incompatible, whereas in another domain like the criminal legal system, I think that investing heavily in the development of so-called predictive crime technologies of various kinds, I don’t think that that’s compatible with decarceration and the long-term project of abolition.
GRAY: I love that you’ve reframed it as a matter of compatibility cause I—what I really appreciate about your work is that you’re—you keep the tension. I mean you—that you really insist on us being willing to grapple with and stay vigilant about what could go wrong without saying don’t do it at all, and I’ve found that really inspiring. Um …
COSTANZA-CHOCK: Well—
GRAY: Yeah, please.
COSTANZA-CHOCK: Can I—can I say one more thing about that, though? I mean, I do—yes, and also there’s a whole nother question here, right? So, you know, is—is this tool harmful? And then there’s also—there’s a democracy question, which is, were people consulted? Do people want this thing? Even if it does a good job, you know, um, and even if it is equitable. And because there’s a certain type of harm, which is, uh, a procedural harm, which is if an automated decision system is deployed against people’s consent or against people’s idea about what they think should be happening in a just interaction with the decision maker, then that’s a type of harm that’s also being done. And so, we really need to think about not only how can we make AI systems less harmful and less biased, among the various types of harm that can happen, but also more accountable, and how can we ensure that there is democratic and community oversight over whether systems are deployed at all, whether these contracts are entered into by public agencies, and whether people can opt out if they want to from the automated decision system or whether it’s something that’s being forced on us.
GRAY: Could you talk a little bit about the work you’re doing around bounties as a way of thinking about harms in algorithmic systems?
COSTANZA-CHOCK: So at the Algorithmic Justice League, one of the projects I’ve been working on over the last year culminated in a recently released report, which is called “Bug Bounties for Algorithmic Harms? Lessons from cybersecurity vulnerability disclosure for algorithmic harms discovery, disclosure, and redress,” and it’s a co-authored paper by AJL researchers Josh Kenway, Camille François, myself, Deb Raji, and Dr. Joy Buolamwini. And so, basically, we got some resources from the Sloan and Rockefeller foundations to explore this question of could we apply bug bounty programs to areas beyond cybersecurity, including algorithmic harm discovery and disclosure? In the early days of cybersecurity, hackers were often in this position of finding bugs in software, and they would then tell the companies about it, and then the companies would sue them or deny that it was happening or try and shut them down in—in various ways. And over time, that kind of evolved into what we have now, which is a system where, you know, it was once considered a radical new thing to pay hackers to find and tell you about bugs in your—in your systems, and now it’s a quite common thing, and most major tech companies, uh, do this. And so very recently, a few companies have started adopting that model to look beyond security bugs. So, for example, you know, we found an early example where Rockstar Games offered a bounty for anyone who could demonstrate how their cheat detection algorithms might be flawed because they didn’t want to mistakenly flag people as cheating in game if they weren’t. And then there was an example where Twitter basically observed that Twitter users were conducting a sort of open participatory audit on Twitter’s image saliency and cropping algorithm, which was sort of—when you uploaded an image to Twitter, it would crop the image in a way that it thought would generate the most engagement, and so people noticed that there were some problems with that. It seemed to be cropping out Black people to favor white people, um, and a number of other things. So Twitter users kind of demonstrated this, and then Twitter engineers replicated those findings and published a paper about it, and then a few months later, they ran a bounty program, um, in partnership with the platform HackerOne, and they sort of launched it at—at DEF CON and said, “We will offer prizes to people who can demonstrate the ways that our image crop system, um, might be biased.” So this was a biased bounty. So we explored the whole history of bug bounty programs. We explored these more recent attempts to apply bug bounties to algorithmic bias and harms, and we interviewed key people in the field, and we developed a design framework for better vulnerability disclosure mechanisms. We developed a case study of Twitter’s bias bounty pilot. We developed a set of 25 design lessons for people to create improved bug bounty programs in the future. And you can read all about that stuff at ajl.org/bugs.
GRAY: I—I feel like you’ve revived a certain, um, ’90s sentiment of “this is our internet; let’s pick up the trash.” It just has a certain, um, kind of collaborative feel to it that I—that I really appreciate. So, with the time we have left, I would love to hear about oracles and transfeminism. What’s exciting you about oracles and transfeminist technologies these days?
COSTANZA-CHOCK: So it can be really overwhelming to constantly be working to expose the harms of these systems that are being deployed everywhere, in every domain of life, all the time, to uncover the harms, to get people to talk about what’s happened, to try and push back against contracts that have already been signed, and to try and get, you know, lawmakers that are concerned with a thousand other things to pass bills that will rein in the worst of these tools. So I think for me, personally, it’s really important to also find spaces for play and for visioning and for speculative design and for radical imagination. And so, one of the projects that I’m really enjoying lately is called the Oracle for Transfeminist Technologies, and it’s a partnership between Coding Rights, which is a Brazil-based hacker feminist organization, and the Design Justice Network, and the Oracle is a hands-on card deck that we designed to help us use as a tool to collectively envision and share ideas for transfeminist technologies from the far future. And this idea kind of bubbled up from conversations between Joana Varon, who’s the directoress of Coding Rights, and myself and a number of other people who are in kind of transnational hacker feminist networks, and we were kind of thinking about how, throughout history, human beings have always used a number of different divination techniques, like tarot decks, to understand the present and to reshape our destiny, and so we created a card deck called the Oracle for Transfeminist Technologies that has values cards, objects cards, bodies and territories cards, and situations cards, and the values are various transfeminist values, like autonomy and solidarity and nonbinary thought and decoloniality and a number of other transfeminist values. The objects are everyday objects like backpacks or bread or belts or lipstick, and the bodies and territories cards, well, that’s a spoiler, so I can’t tell you what’s in them.
GRAY: [LAUGHS]
COSTANZA-CHOCK: Um, and the situations cards are kind of scenarios that you might have to confront. And so what happens is basically people take this card deck—and there’s both a physical version of the card deck, and there’s also a virtual version of this that we developed using a—a Miro board, a virtual whiteboard, but we created the cards inside the whiteboard—and people get dealt a hand, um, and either individually or in small groups, you get one or several values, an object, a people/places card, or a bodies/territory card and a situation, and then what you have to do is create a technology rooted in your values and—that somehow engages with the object that you’re dealt that will help people deal with the situation, um, from the future. And so people come up with all kinds of really wonderful things that, um—and—and they illustrate these. So they create kind of hand-drawn blueprints or mockups for what these technologies are like and then short descriptions of them and how they work. And so people have created things like community compassion probiotics that connect communities through a mycelial network and the bacteria develop a horizontal governance in large groups, where each bacteria is linked to a person to maintain accountability to the whole, and it measures emotional and affective temperature and supports equitable distribution of care by flattening hierarchies. Or people created, um, a—
GRAY: [LAUGHS] Right now, every listener is, like, Googling, looking feverishly online for these—for the, the Oracle. Where—where do we find this deck? Where—please, tell us.
COSTANZA-CHOCK: So you can—you can just Google “the Oracle for Transfeminist Technologies” or you can go to transfeministech.codingrights.org. So people create these fantastic technologies, and what’s really fun, right, is that a lot of them, of course, you know, we could create something like that now. And so our dream with the Oracle in its next stage would be to move from the completely speculative design, you know, on paper piece to a prototyping lab, where we would start prototyping some of the transfeminist technologies from the future and see how soon we can bring them into the present.
GRAY: I remember being so delighted by a very, very, very early version of this, and it was the tactileness of it was just amazing, like, to be able to play with the cards and dream together. So that’s—I’m so excited to hear that you’re doing that work. That’s—that is inspiring. I’m just smiling. I don’t know if you can hear it through the radio, but, uh—wow, I just said “radio.” [LAUGHTER]
[MUSIC PLAYS UNDER DIALOGUE]
COSTANZA-CHOCK: It is a radio. A radio in another name.
GRAY: I guess it is a radio. That’s true. A radio by another name. Oh, Sasha, I could—I could really spend all day talking with you. Thank you for wandering back into the studio.
COSTANZA-CHOCK: Thank you. It’s really a pleasure. And next time, it’ll be in person with tea.
GRAY: Thanks to our listeners for tuning in. If you’d like to learn more about community-driven innovation, check out the other episodes in our “Just Tech” series. Also, be sure to subscribe for new episodes of the Microsoft Research Podcast wherever you listen to your favorite shows.
[MUSIC ENDS]
The post Just Tech: Centering Community-Driven Innovation at the Margins Episode 3 with Dr. Sasha Costanza-Chock appeared first on Microsoft Research.
Jigsaw fixes bugs in machine-written software

Large pre-trained language models such as GPT-3, Codex, and others can be tuned to generate code from natural language specifications of programmer intent. Such automated models have the potential to improve productivity for every programmer in the world. But since the models can struggle to understand program semantics, the quality of the resulting code can’t be guaranteed.
In our research paper, Jigsaw: Large Language Models meet Program Synthesis, which has been accepted at the International Conference on Software Engineering (ICSE 2022), we introduce a new tool that can improve the performance of these large language models. Jigsaw deploys post-processing techniques that understand the programs’ syntax and semantics and then leverages user feedback to improve future performance. Jigsaw is designed to synthesize code for Python Pandas API using multi-modal inputs.
Our experience suggests that as these large language models evolve for synthesizing code from intent, Jigsaw can play an important role in improving the accuracy of the systems.
The promise, and perils, of machine-written software
Large language models like OpenAI’s Codex are redefining the landscape of programming. A software developer, while solving a programming task, can provide a description in English for an intended code fragment and Codex can synthesize the intended code in languages like Python or JavaScript. However, the synthesized code might be incorrect and might even fail to compile or run. Codex users are responsible for vetting the code before using it. With Project Jigsaw, we aim to automate some of this vetting to boost the productivity of developers who are using large language models like Codex for code synthesis.
-
Project
Project Jigsaw
Suppose Codex provides a code fragment to a software developer. The developer might then undertake a basic vetting by checking whether the code compiles. If it doesn’t compile, then the developer might be able to use the error messages of the compiler to repair it. Once the code eventually does compile, a typical developer will test it on an input to check whether the code is producing the intended output or not. Again, the code might fail (raise an exception or produce incorrect output) and the developer would need to repair it further. We show that this process can be completely automated. Jigsaw takes as input an English description of the intended code, as well as an I/O example. In this way, it pairs an input with the associated output, and provides the quality assurance that the output Python code will compile and generate the intended output on the provided input.
In our ICSE 2022 paper, Jigsaw: Large Language Models meet Program Synthesis, we evaluate this approach on Python Pandas. Pandas is a widely used API in data science, with hundreds of functions for manipulating dataframes, or tables with rows and columns. Instead of asking a developer to memorize the usage of all these functions, an arguably better approach is to use Jigsaw. With Jigsaw, the user provides a description of the intended transformation in English, an input dataframe, and the corresponding output dataframe, and then lets Jigsaw synthesize the intended code. For example, suppose a developer wants to remove the prefix “Name: ” from the column “country” in the table below. Using Pandas, this can be solved performing the following operation:
df['c'] = df['c'].str.replace('Name: ', '')

A developer who is new to Pandas will need to figure out the functions and their arguments to put together this code fragment or post the query and example to a forum like StackOverflow and wait for a good Samaritan to respond. In addition, they might have to tweak the response, at times considerably, based on the context. In contrast, it is much more convenient to provide the English query with an input-output table (or dataframe).
How Jigsaw works
Jigsaw takes the English query and pre-processes it with appropriate context to build an input that can be fed to a large language model. The model is treated as a black box and Jigsaw has been evaluated both with GPT-3 and Codex. The advantage of this design is that it enables plug-and-play with the latest and greatest available models. Once the model generates an output code, Jigsaw checks whether it satisfies the I/O example. If so, then Jigsaw is done! The model output is already correct. In our experiments, we found this happened about 30% of the time. If the code fails, then the repair process starts in a post-processing phase.
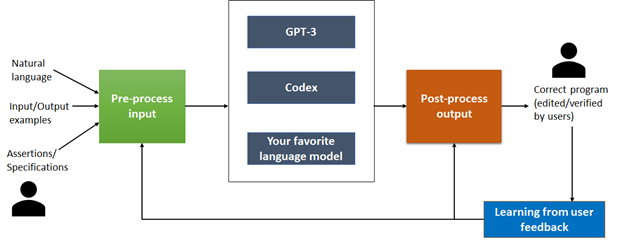
During post-processing, Jigsaw applies three kinds of transformations to repair the code. Each of these transformations is motivated by the failure modes that we have observed in GPT-3 and Codex. Surprisingly, both GPT-3 and Codex fail in similar ways and hence Jigsaw’s post-processing to address these failure modes is useful for both.
Variable transformations
We have observed that Codex can produce output that uses incorrect variable names. For example, most publicly available code uses names like df1, df2, etc. for dataframes. So, the Codex output also uses these names. Now, if the developer uses g1, g2, etc. as dataframe names, the Codex output is probably going to use df1, df2, etc. and fail. Other times Codex confuses variable names provided to it. For instance, it produces df2.merge(df1)instead of df1.merge(df2)
. To fix these kinds of errors, Jigsaw replaces names in Codex generated code with all possible names in the scope until it finds a program that satisfies the I/O example. We find this simple transformation to be quite useful in many cases.
Argument transformations
Sometimes Codex generated code calls the expected API functions but with some of the arguments incorrect. For example:
a.) Query – Drop all the rows that are duplicated in column ‘inputB’
dfout = dfin.drop_duplicates(subset=['inputB']) # Model
dfout = dfin.drop_duplicates(subset=['inputB'],keep=False) # Correct
b.) Query – Replace Canada with CAN
in column country of df
df = df.replace({'Canada':'CAN'}) # Model
df = df.replace({'country':{'Canada':'CAN'}) # Correct
To fix such errors, Jigsaw systematically enumerates over all possible arguments, using the function and argument sequences generated by Codex as a starting point, until it finds a program that satisfies the I/O example.
AST-to-AST transformations
An AST (abstract-syntax-tree) is a representation of code in the form of a tree. Since models like Codex work at a syntactic level, they might produce code which is syntactically very close to the intended program, but some characters might be incorrect. For example:
a.) Query – Select rows of dfin where value in bar is or
>60
dfout = dfin[dfin['bar']60] # Model
dfout = dfin[(dfin['bar'])|(dfin['bar']>60)] # Correct
Mistake – missing parentheses change precedence and cause exception
b.) Query – Count the number of duplicated rows in df
out = df.duplicated() # Model
out = df.duplicated().sum() # Correct
Mistake – missing required summation to get the count
To fix this failure mode, Jigsaw provides AST-to-AST transformations that are learned over time. The user would need to fix the code themselves — then the Jigsaw UI will capture the edit, generalize the edit to a more widely applicable transformation, and learn this transformation. With usage, the number of transformations increases, and Jigsaw becomes more and more effective.
Evaluation
We evaluated Codex and Jigsaw (with Codex) on various datasets and measured accuracy, which is the percentage of tasks in the dataset where the system produces the intended result. Codex gives an accuracy of about 30% out-of-the-box, which is what is expected from OpenAI’s paper as well. Jigsaw improves the accuracy to >60% and, through user feedback, the accuracy improves to >80%.
The road ahead
We have released the datasets that we used to evaluate Jigsaw in the public domain. Each dataset includes multiple tasks, where each task has an English query and an I/O example. Solving a task requires generating a Pandas code that maps the input dataframe provided to the corresponding output dataframe. We hope that this dataset will help evaluate and compare other systems. Although there are datasets where the tasks have only English queries or only I/O examples, the Jigsaw datasets are the first to contain both English queries and the associated I/O examples.
As these language models continue to evolve and become more powerful, we believe that Jigsaw will still be required for providing the guardrails and making these models viable in real-world scenarios. This is just addressing the tip of the iceberg for research problems in this area and many questions remain to be answered:
- Can these language models be trained to learn semantics associated with code?
- Can better preprocessing and postprocessing steps be integrated into Jigsaw? For example, we are looking at static analysis techniques to improve the post-processing.
- Are I/O examples effective for other APIs apart from Python Pandas? How do we tackle scenarios where I/O examples are not available? How do we adapt Jigsaw for languages like JavaScript and general code in Python?
- The developer overhead of providing an example over just providing a natural language query needs further evaluation and investigation.
These are some of the interesting directions we are pursuing. As we refine and improve Jigsaw, we believe it can play an important role in improving programmer productivity through automation. We continue to work on generalizing our experience with the Python Pandas API to work across other APIs and other languages.
Other contributors:
Naman Jain, Research fellow at Microsoft Research India Lab
Skanda Vaidyanath, Intern at Microsoft Research India Lab, currently pursuing master’s degree at Stanford
The post Jigsaw fixes bugs in machine-written software appeared first on Microsoft Research.