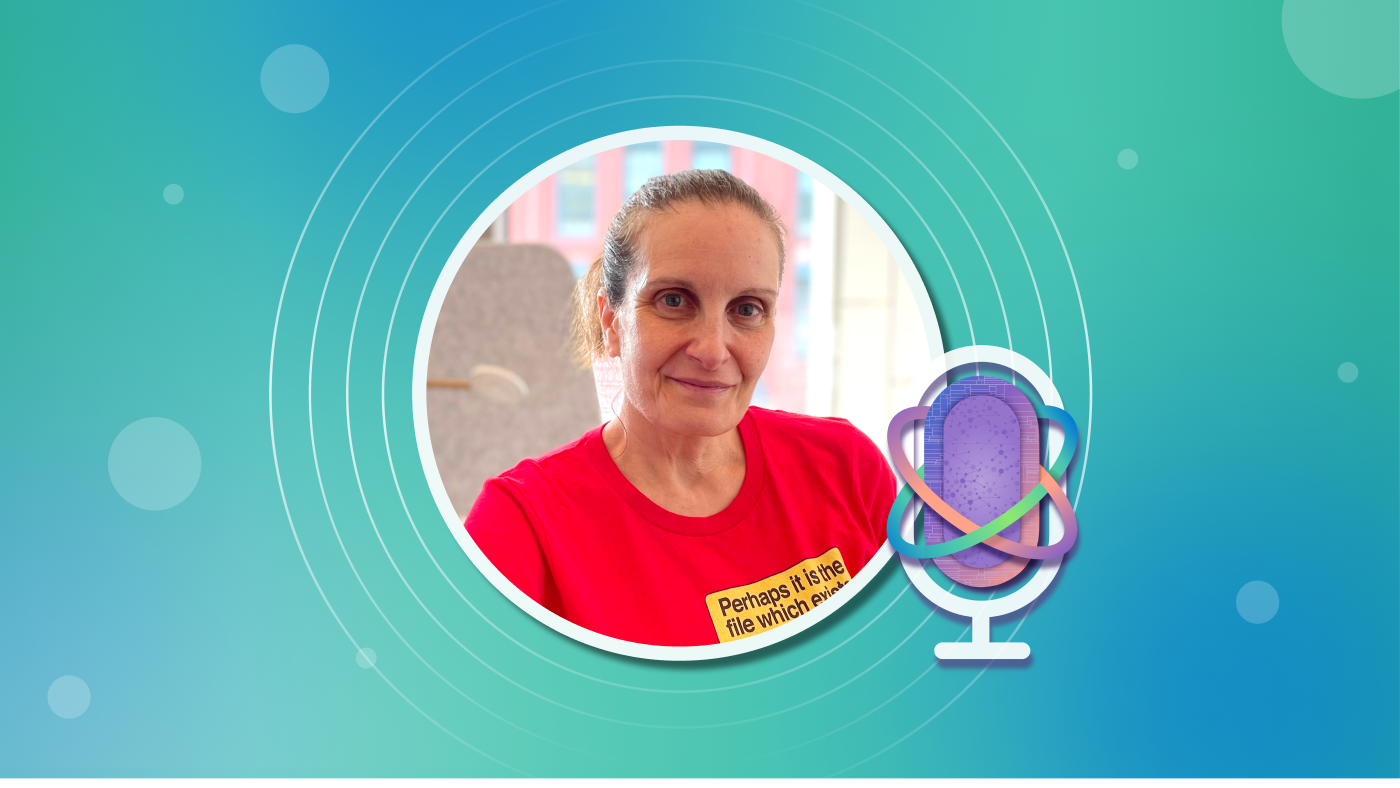
Behind every emerging technology is a great idea propelling it forward. In the new Microsoft Research Podcast series, Ideas, members of the research community at Microsoft discuss the beliefs that animate their research, the experiences and thinkers that inform it, and the positive human impact it targets.
In this episode, host Gretchen Huizinga talks with Rafah Hosn, partner, group product manager for AI Frontiers at Microsoft Research. Hosn’s professional experience spans the gamut—from research to product to engineering to research again, the discipline’s uniquely high levels of creativity, curiosity, and intellect drawing her back in. Energized by past technical disruptions she’s experienced, Hosn is on what she describes as her “most exciting adventure” yet, helping to drive scientific advancement in AI and to answer a big question: how far can we push machine intelligence while still delivering technologies people can derive value from?
Subscribe to the Microsoft Research Podcast:
Transcript
[TEASER] [MUSIC PLAYS UNDER DIALOGUE]RAFAH HOSN: What has changed is that in the old days, we had the luxury of creating something, going and piloting for three months until we know whether it works or not, and then taking one year to productize! That … that, that doesn’t work anymore! Because guess what? In three months, this innovation is, like, topped by four other innovations, be it at Microsoft or elsewhere. So that speed is really shifting the mindset and the spirit of people.
[TEASER ENDS]GRETCHEN HUIZINGA: You’re listening to Ideas, a Microsoft Research Podcast that dives deep into the world of technology research and the profound questions behind the code. I’m Dr. Gretchen Huizinga. In this series, we’ll explore the technologies that are shaping our future and the big ideas that propel them forward.
[MUSIC FADES]
My guest today is Rafah Hosn. She’s a partner, group product manager for AI Frontiers at Microsoft Research. I’d call Rafah a sort of organizational conductor, working both with leaders to drive clarity around the mission as well as program managers to make sure they have solid operational strategies to execute on it. Rafah has mad skills in bringing research ideas from lab to life, and I’m thrilled to talk to her today. Rafah Hosn, welcome to Ideas!
RAFAH HOSN: Thank you, Gretchen. Oh, my goodness, I have to live up to this introduction now! [LAUGHTER]
HUIZINGA: Well, before we talk about research ideas, let’s talk about you and your own sort of “reason for being” in the research world. How would you describe your motivation for working in research and—assuming there was one—what was the “big idea” or animating “what if?” behind what you’re doing today?
HOSN: Yeah, you know, I don’t know. There are so many big ideas, to be honest! Every day, I wake up and I often tell my husband how lucky, like so totally lucky and humbled, I am to be where I am right now in this moment, like right now when society as we know it is being totally disrupted by this huge leap in AI. And why research? Well, I’ve tried it all, Gretchen! I’ve been in research, I went to product, I did engineering, and I did full circle and came back to research. Because, you know, for me personally, there’s no other environment that I know of, for me, that has this amount of creativity and just infinite curiosity and intellect. So working with people that are asking “what next?” and trying to imagine the next world beyond where AI is today is just … this is the big idea. This is why I’m here. This is why I’m excited to come to work every day.
HUIZINGA: Yeah. Well … and I want to drill in a little bit just, sort of, personally because sometimes there’s a story, an origin story, if you will, of some pivotal aha moment that you say, oh, that’s fascinating, that’s cool, that’s what I want to do. Anything that piqued your interest way back when you were a kid or, sort of, a pivotal moment in your educational years?
HOSN: Yeah, you know, so many different things that inspire you along the journey, right. It’s not just one thing, Gretchen. My dad was a doctor. He was my biggest inspiration growing up. And the reason is because he had a lot of depth of knowledge in his domain. And I wanted that. I wanted to have depth of knowledge in a domain. So I went engineering against his advice. He really wanted me to be a doctor. [LAUGHTER] So he was not too happy. But, you know, throughout my education, you know, I was there when smartphones came about, when the internet was a thing. And now, like with generative AI, I feel like I’ve lived through so many disruptions, and every one of those was, “Oh my gosh! Like, I am exactly where I want to be!” So multiple inspirations, and every day, I wake up and there’s new news and I’m saying to myself, “OK, that’s great.” I love it!
HUIZINGA: What a time to be alive!
HOSN: It is amazing!
HUIZINGA: Yeah. Well, you recently took on this new role in AI Frontiers at Microsoft Research. And that very word “frontiers” evokes images of unexplored, uncharted territories like the Wild West or for Trekkies, maybe “space: the final frontier.” So what does it mean to you to be working at the frontier of artificial intelligence, and what’s the big idea behind AI Frontiers?
HOSN: You know, it’s my biggest and most exciting adventure so far! Working under Ece Kamar’s leadership in this AI Frontiers is really trying to push ourselves to think, what’s beyond what there is right now in artificial intelligence? Where can we push more, from a scientific perspective? How do we translate these scientific discoveries into capabilities that people can actually use and derive value from? It’s a big responsibility, as well, because we just don’t want to push the boundaries of AI for the sake of pushing. We want to push it in a safe and responsible way. So it is a big responsibility.
HUIZINGA: Yeah …
HOSN: And fundamentally, you know, the unifying big idea in this team is to explore, you know, how far can we push intelligence further into models and encapsulations of those models so that we can, you know, have not just sort of an assistant but really a personal assistant, an agent that can, kind of, do tasks for us, with us, seamlessly across multiple domains? So this is what we’re trying to push for.
HUIZINGA: Mmm. Rafah, do you feel like you’re at the frontier of artificial intelligence? I mean, what are the emotions that crop up when you are dealing with these things—that you and your teams basically know about but the rest of us don’t?
HOSN: For most days, it’s excitement. Sometimes it’s [LAUGHTER] … it ranges, to be honest. I would say there’s a spectrum of emotions. The dominating one is really just excitement. There’s so much that has happened with GenAI, but I feel like it has opened up so many different paths, as well, for us to explore, and that’s the excitement. And then every time the world accomplishes something, you’re like in astonishment. You’re like, wow, wow.
HUIZINGA: Yeah …
HOSN: And then, and then, oh my gosh, what’s next? And so, it’s a range of emotions …
HUIZINGA: Right …
HOSN: … but I would say the dominating one is enthusiasm.
HUIZINGA: Yeah. Well, I’ve heard other people on your teams use words like surprise, sometimes even shock …
HOSN: Yeah, yeah, there are a lot of “wow” factors. Every day, every day, I wake up, I read like my three favorite AI tweets or things like that, and I’m like, “Oh my gosh. I wouldn’t have imagined that this model could do this thing,” so [LAUGHS] … um, but it’s exciting.
HUIZINGA: We may have to get those accounts in the show notes so that we can follow along with your surprise and amazement in the mornings!
HOSN: [LAUGHS] Yes!
HUIZINGA: Well, listen, when we talk about measuring the success of an AI system, we often use the common convention of what we call benchmarks. But I want to zoom out from AI systems for a minute and ask how you might measure the success of an AI lab, which is what you’re working in. What are your benchmarks or key performance indicators—we call them KPIs—for the work going on at AI Frontiers?
HOSN: Yeah, so I’m going to start by something that may sound surprising maybe to some, but I think it’s the culture first. It’s the culture of endless curiosity, of enthusiasm coupled with a bit of skepticism, to be honest, to ask the questions, the right questions, and this drive to push further. So I would say one KPI of success for me, personally, is, you know, can we maintain this culture of enthusiasm coupled with skepticism so we can ask hard questions and an envelope of enthusiasm and drive for everyone? So that’s one. I would say the other three are … one is around how much can we push scientifically as a community, right? This is a team of people that are getting together with a mission to push the boundaries of our understanding of artificial intelligence. So are we pushing that scientific boundaries? Are we creating insights, not just for the scientific community, but also for Microsoft and the world, so that we know how to derive value from these discoveries, right? At the end of the day, it is awesome to push scientifically. It’s even more awesome if you take this and translate it into something a human being can use …
HUIZINGA: Yeah …
HOSN: … or an enterprise can use. And I think … that’s kind of my KPIs of success. Culture first, pushing on the scientific boundaries, creating insights for the scientific community as well as for Microsoft so we can derive value for us as a society, right.
HUIZINGA: Yeah. Well, continuing on this idea of success, and you’ve alluded to this already in terms of characteristics of curiosity and so on, part of your job, as you put it, was “enabling brilliant minds to find success.” So talk a little bit about the personal qualities of these brilliant minds and how you help them find success.
HOSN: Yeah, you know, everybody I work with brings different aspects of brilliance to the table—every day. So in our community of engineers, PMs, researchers, everybody is present with their ideas and their strengths. And they’re pulling together to push harder and faster on our key priorities. And I find folks working in AI these days, you know, to have a renewed fire. It’s really amazing to see. And I talk a lot about curiosity, but, you know, I cannot emphasize how much this is driving a lot of our community to explore new paths that they hadn’t thought about prior to this GenAI coming along. And so everybody is showing up, present, asking these questions and trying to solve new scenarios, new problems that are emerging. And from my perspective, you know, as you mentioned, I just try to unblock, basically. My team and I are here to [LAUGHTER] … well, two things I would say. First is bring the outside-in perspective. That’s so important because science is amazing, but unless you can derive value from it, it remains an awesome paper and an awesome equation, right. So asking, who can use this? What are the scenarios it could, you know, light up? How can we derive value? So those are the questions that my team and I can contribute to, and we are trying to participate from ideation all the way to basically delivering on key milestones. And that last mile is so important. Like, once you know what you want to do, how do you structure? How do you have an operational strategy that is amenable to these times, which is fast, fast, fast, and faster? So that’s, kind of, what we’re trying to do here.
HUIZINGA: Yeah, yeah. Well, two things came to my mind in terms of what kinds of people would end up working in this area. And one would be agility, or agile. And that would, to me, represent in a researcher that the person would be able to spin or pivot if something didn’t work out. And the other one is sort of a risk-reward mentality. It’s like, where are you willing to push to get that reward versus what might keep you from even trying?
HOSN: Yeah, so definitely in this AI Frontiers community, I’m finding a lot of adaptability. So people willing to try, failing fast when they fail, and pivoting. And you have to, nowadays, in this atmosphere that we are living in. And because we have the privilege of working in research—and it’s really an honor and a privilege, and I’m not saying it just lightly—but it is the place where you can take risks, Gretchen. It is the place where failing is totally fine because you’re learning and you’re pivoting in a way that allows you to progress on the next thing you tackle. So I feel like most of the people I work with in this community, AI Frontiers, we are risk takers. We want to push, and it’s OK to fail, and it’s OK to adapt. So, I think, as an aggregate, that’s kind of the spirit I’m seeing.
HUIZINGA: In the past, Rafah, you’ve stressed the importance of both teams and timing. And so we’ve been talking about the teams and the minds and the kinds of qualities in those people. But what about the “when” of research? How does timing impact what gets done in your world?
HOSN: Well, in this new era, Gretchen, everything is yesterday! [LAUGHS] I mean, it is true. AI research is moving at such speeds that I feel like we need to get accustomed to a timing of now. And if it’s not now, it’s yesterday. So the timing is important, but the leeway has shrunk so significantly that I feel like we have to really just be present in the moment and just move as fast as we can because everybody else is moving at the highest speed. So timing is “now,” is what I would say.
HUIZINGA: On that note, with so many innovations in AI coming out every day, every minute, what you’ve just expressed is that research horizons are shorter than ever. But as one of your team members noted in a recent panel, it still takes a lot of time to translate a research artifact, maybe a noteworthy finding or a published paper or an equation, an algorithm, into a useful product for humans. So how are you then dealing with these newly compressed timelines of “it needs to be done yesterday to keep up,” and how has the traditional research-to-product pipeline changed?
HOSN: Yeah, it’s an awesome question. It is so true that going from research to a production-quality algorithm or capability takes time. But what I’m seeing is that the research-to-capabilities is accelerating, meaning if you look at the world today in generative AI and its surrounding, folks even in research are creating assets as they are creating their research. And so they are thinking as well, how do I showcase this? And of course, these assets are not production ready. But here’s the kicker. I think that the product teams are also adapting to this generative AI era, and they are changing to meet this disruptive moment. They are changing the way they think, and they are accelerating the way they productize and look at hardening and securing the assets so that they can put them in the hands of even a limited set of users just to get a feel of what it means to have them in the hands of end users and quickly iterating so that they can further harden and further improve the design until it’s production ready. And I feel like our product partners are meeting the moments, meaning they also are really adapting their processes such that they can get these assets and put them in the hands of users and test them out before they actually release them.
HUIZINGA: Right. Let’s drill in a little bit more on that and talk about the traditional research-to-product pipeline, where you would have a researcher working on something and then an RSDE. What does RSDE stand for?
HOSN: A research software development engineer. It’s a mouthful.
HUIZINGA: Right. And then to the PM, or program manager, and then to the engineer. And you’ve said this provocative statement: now everyone is a PM!
HOSN: Everyone is a PM! [LAUGHTER]
HUIZINGA: What do you mean by that?
HOSN: I just, I just feel like if we are to meet the moment, we need to be thinking outside-in, inside-out simultaneously. And I believe that the spirit of program management, which is looking at the design from a user-centric perspective, is embedded as we are ideating, as we are trying to explore new methodologies, new algorithms, new assets. And so what has changed is that in the old days, we had the luxury of creating something, going and piloting for three months until we know whether it works or not, and then taking one year to productize! That … that, that doesn’t work anymore. [LAUGHTER]
HUIZINGA: Right.
HOSN: Because guess what? In three months, this innovation is, like, topped by four other innovations, be it at Microsoft or elsewhere. So that speed is really shifting the mindset and the, and the spirit of people. I have colleagues and friends, researchers, that are asking me, oh, scenarios, users … I mean it’s amazing to see. So, yes, everybody has gotten a little PM in them now. [LAUGHTER]
HUIZINGA: Yeah, I did a podcast with Shamsi Iqbal and Jina Suh. And Shamsi was talking about this concept, this old concept, of the researcher being in their lab and saying, well, I’ve done this work; now go see what you want to do with it. I don’t think you have that affordance anymore as a researcher.
HOSN: No …
HUIZINGA: You’ve got to work much more tightly with other team members and think like a PM.
HOSN: Totally.
HUIZINGA: So let’s talk about how the big general idea behind AI Frontiers is giving birth to smaller, more specific ideas. What are some of the research directions and projects that you could tell us about that illustrate this vision here?
HOSN: Yeah, and I’m sure you’ve heard some of it come from Ece Kamar as she spoke on this community that we have. In AI Frontiers, we’re exploring, I would say, three major areas of research. And I want you to imagine a stack. At the bottom of the stack, we’re asking ourselves questions around, what are some new architectures we can be thinking about for these foundational models? How do we create them? What kind of data we need to train them, to pre-train them. And then on top of that stack, which starts with a foundation model, we’re asking ourselves, OK great, you have a pretrained model. In a lot of cases, when you’re creating especially small models, you need to fine-tune them. So what is this methodology and data generation pipeline that we’re going to use to fine-tune these models and specialize them for both across domains and across skill set? And on top of that—so now we’re on the third layer—we have a final layer that encapsulates these models and orchestrates among them to allow them the ability to do, you know, complex tasks. And we don’t want to stop there because for us it’s … we don’t want to have an agent that just does things and doesn’t learn. So that learnability, that learning on the job, like we do as humans, is something we’re asking ourselves, as well. Like, how do we encapsulate these models? We orchestrate among them. And we allow these encapsulated things, we call them agents, to learn on the job so that they can accomplish more complex tasks. So those are the three things. And then cutting across these three layers, imagine there’s a thing that cuts across them, is doing everything in a way that allows us to rigorously evaluate and to ensure that we’re doing things in a safe and responsible way. So those are the main things that we’re working on. Does that make sense?
HUIZINGA: That’s … yes, it does. And I imagine, you know, if you go to the website and you see those, kind of, three main areas, I imagine that even under there, there are specific projects on, you know, how then do we iterate? How then do we explore?
HOSN: That’s right. That’s a good plug for people to visit the AI Frontiers website! Thank you, Gretchen! [LAUGHS]
HUIZINGA: Well, I’ve been intrigued for a while by this idea of what you’ve called bi-directional enrichment, which represents both how research informs product but also how product informs research, but you’ve recently talked about how this idea has expanded to embrace what you call multi-directional enrichment and co-innovation. So what do you mean by that, and what does it look like for you?
HOSN: So we talked just moments ago how the time has shrunk tremendously in artificial intelligence and the speed at which innovations are coming out. So what does that mean when you are sitting in research and you’re trying to derive value for Microsoft, for example? It means that now, rather than going on a journey to try out you know different things, what you want is for product to come on a co-innovation journey with you. And not every team has the capability or the time or the resources to do it. But sometimes product teams have applied scientists that are asking themselves very similar questions. And so now we have this huge synergistic effect by which, you know, researchers can come and explore their research but anchor them in a real-world scenario that the product team is, you know, asking themselves about. And that’s what I mean by co-innovation. And we look for co-innovation, so these are product teams or applied scientists in product teams that are not looking at something I can ship tomorrow. Because that’s not … that’s not frontiers. That’s feature-function that they can deliver right now to their customers. When we co-innovate, we have to co-innovate on a bit of a longer timespan. Now it’s no longer years, right? With generative AI, everything is months, but nonetheless, this is not next week. This is in a few months. And so … but this is really, really great because, again, I keep saying this and I have maybe a huge bias, but I do believe that research, without it being anchored in real-world scenario, just doesn’t have the same effect. So I have a bias for that. It’s my PM hat, what can I say? I love real-world scenarios! [LAUGHTER]
HUIZINGA: What you just referred to is an interesting flow. I’ve noticed in my years doing this podcast that some people that started in research ended up over in product—and we’ll call them embedded researchers, if you will—and then some people that were in a product scenario come back over to research. And so, there’s this flow, multi-directional, bi-directional, and also where they’re placed within the company. How do you see that flow and the value of that flow between these organizations?
HOSN: Yeah, you know, like, I think that the flow is important because that’s how cross-pollination happens. And you talked about brilliant minds. In product teams, there are brilliant minds, as well, right. And although their focus area is more around the product they live and breathe every day, this is enriching to researchers and continues to be enriching because when you deploy research capabilities in a real-world setting, there are surprising new research questions that come up, not just engineering. A lot of times people think of research, OK, yeah, you scale it, you harden it, you secure it, and it’s good to go. But that’s not always the case. In a lot of cases, because of the interactivity that happens with real-world scenarios, it opened up brand-new paths for research. And so I think that flow continues to happen even now. It’s just compressed. It’s just that researchers are no longer thinking six years. Researchers are thinking three months. Like, what am I going to do in three months? Because in three months, there will be a hundred other researchers that are coming up with innovation on the same question. So I think the flow still exists. I think that time has shrunk. And I think the mobility from researchers and research going to product and vice versa is enriching for the people that do it because you gain different perspectives.
HUIZINGA: Well, and let’s push in even there a little bit. Researchers like everyone else can get comfortable looking at things through a particular lens. I would say that’s a human trait, not just a research trait …
HOSN: Absolutely.
HUIZINGA: … until a disruption challenges their status quo. So you’ve talked about LLMs, which we’ve called large language models, as being a good forcing function for researchers to think differently, even about the questions they’re asking. Can you elaborate on that a little bit?
HOSN: Yeah, yeah, so, you know, the large language models and this disruption that we are living in at the moment is lighting fire underneath a lot of people’s intellect, I’m going to say. And so I think that people have to adapt quickly to change. And this is key. Adaptability, I believe, is just a key ingredient in doing research nowadays. Why? Because a lot of people are thinking directionally the same. And so, you know, if you’re not the first, you’re going to have to adapt to what came out. And then you have to think of, how do I differentiate? So the second point I would say is differentiation. And this mindset of, you know, how do I adapt to what just came out? How do I differentiate? And then—Rafah’s bias—how do I anchor in real-world scenario? This is the home run. And I would say you package all of this and focus, focus, focus … and you get a gold mine.
HUIZINGA: I’m hearing “yes, and …” in this response in the sense of not everyone’s going to be first, but then, what else? This is back to the frontiers. It’s like, how do I differentiate? Yes, that’s awesome. And we’ve got this …
HOSN: Exactly. And how do I build on what has just been discovered and give it a little bit of an edge or push it a little further or take it in a brand-new direction? I mean, so many different possibilities, but it does take adaptability, like a flexibility in the mindset, I would say.
HUIZINGA: Yeah. Well, let’s go back to what you alluded to earlier, this idea of responsible AI. This is a big deal at Microsoft. And researchers are very thoughtful about the question of what could possibly go wrong if we got everything right. But how does that translate practically, and what concrete steps are you taking at what I’ll call the “frontier of responsibility?”
HOSN: Yeah, and as I mentioned, you know, being at the frontiers is amazing. It also holds a big responsibility. We have so many different, I would say, checks and balances that we use, in model training and fine-tuning, to ensure that we are on top of all the regulatory, the policymaker suggestions, and we are abiding by Microsoft values first and foremost and responsibility in creating these innovations. So practically and tactically, what happens is that there are processes for how you actually even release any type of model. And this is just research. And when it goes to product, they have their own compliance, you know, a stricter even compliance, I would say, process that they go through. So we try, and I try particularly, to partner with our privacy champions, with our legal champions, with our people that are looking at this from a responsible AI perspective, so that we bring them in early on, and we say, hey, we’re thinking of doing this. And they tell us, well, you know, if you’re thinking about it this way, you might want to consider this. So we’re trying to bring them in as early as possible so that also we don’t go all the way and then we discover we did something wrong, so we have to backtrack. So I would say, you know, having these partners and colleagues come in early in the game just saves everybody a lot of time. And all this responsible AI for us, it’s ingrained with how we work, meaning we bring our champions early on and then we have them advise us as we move along the journey to create these innovations. So by the time we’re done, we know we’re good, right. And even by the time we’re done, we recheck everything, we run a lot of evaluation benchmarks, and, you know, we do the right thing per policies at Microsoft. So we take it very, very seriously.
HUIZINGA: Well, let’s go back to this idea of research horizons for a second and anchor it in the way that we approach research. So many ideas are basically iterative steps on existing work, and they make a lot of sense … this is the next step … but then there are those out-of-the-box ideas that feel like maybe bigger swings—some might even call them outrageous—and in organizations like Microsoft Research, they might get the green light, too. Where do you find this idea of the outrageous or maybe longer-term idea finding a home or a place in an organization like Microsoft Research, and have you ever worked on something that felt outrageous to you?
HOSN: Umm, you know, we like outrageous! That’s why we’re in research, right? So outrageous is good. I haven’t, to be honest, worked on an outrageous, but I am confident I will be. So … [LAUGHTER] I just have this belief that in AI Frontiers, we are going to have outrageous ideas, and we’re going to work on them, and we’re going to make bets that basically are hard to make in other parts of the company because we have the privilege of taking them and pursuing them. And, yes, they may fail, but if we have a breakthrough, it will be a significant breakthrough. So, so I think that outrageous is good. We need to think big. We need to take big leaps, big ideas. We also need to know how to fail gracefully and pivot fast!
HUIZINGA: Hmmm. Mmm. You know, it strikes me, and I’m laughing to myself, it strikes me, even as we’re talking, that the idea that you work in AI Frontiers, that’s outrageous to most people and, and it’s normal to you. So maybe this idea of, “I haven’t worked on anything outrageous” is like, no, you live in outrageous, it just doesn’t seem like it! [LAUGHTER]
HOSN: Maybe. It’s my day-to-day job, so, yes, I guess you’re right.
HUIZINGA: Right. I mean, yeah, you say, we love outrageous, and that’s where it is right now. Every day that I follow, sort of, AI Twitter also and find myself going, seriously? That happened yesterday? What next?
HOSN: Yeah, in two hours, there’ll be yet another thing. So, yeah, I guess I am living in outrageous, and I love it! It’s amazing! [LAUGHS]
HUIZINGA: Yeah, maybe the idea of outrageous is just changed.
HOSN: You know, you’re so right. I think that it’s become the norm. And it is, once we anchor in generative AI, and we push further on this idea, maybe we will go back in a cycle where outrageous is outrageous, but today it’s our life. It’s where we live. It’s what we breathe every day. So it’s become a norm.
HUIZINGA: Yeah. Well, as we close, Rafah, I want to ask a question anchored on the big idea behind AI Frontiers. What do you believe might be true in say 10 to 15 years, and what should we be doing about it now? In other words, how does what we believe about the future influence how we conceptualize and execute on ideas today?
HOSN: Yeah, you know, it’s … I can’t even predict what I’m going to be doing tomorrow! But … [LAUGHTER] here’s, here’s what I think. I think that we are truly approaching a moment in human history where a lot of unsurmountable problems, like very hard-to-tackle diseases that have been so hard, I think we are approaching a moment, you know, soon, I hope it’s even sooner than 10 years, where generative AI and innovations on top of it could lead to a lot of resolution for things that today … that cause unsurmountable pain and suffering. I’m very hopeful that with what we are creating that we can, you know, take inefficiencies out of so many different things that we see today that take time so that we liberate ourselves to think about the “what next” societally, right? I think what we need to be doing right now, to be honest, to influence the future is think about our curricula. What are we going to teach our kids? What are they going to work in? This is where I’m hoping that we pour some of our creativity, education system. How are we preparing the next generation? What are the paths that we are going to forge for them, knowing what we know today, knowing what this technology can bring forth? So my hope is that we put some brain power into that.
HUIZINGA: Rafah Hosn, it’s always a pleasure to talk to you. A sincere pleasure, a delight. Thanks for joining us today on Ideas.
[MUSIC PLAYS]HOSN: Thank you so much for having me, Gretchen.
[MUSIC FADES]
The post Ideas: Exploring AI frontiers with Rafah Hosn appeared first on Microsoft Research.