CoreWeave today became one of the first cloud providers to bring NVIDIA GB200 NVL72 systems online for customers at scale, and AI frontier companies Cohere, IBM and Mistral AI are already using them to train and deploy next-generation AI models and applications.
CoreWeave, the first cloud provider to make NVIDIA Grace Blackwell generally available, has already shown incredible results in MLPerf benchmarks with NVIDIA GB200 NVL72 — a powerful rack-scale accelerated computing platform designed for reasoning and AI agents. Now, CoreWeave customers are gaining access to thousands of NVIDIA Blackwell GPUs.
“We work closely with NVIDIA to quickly deliver to customers the latest and most powerful solutions for training AI models and serving inference,” said Mike Intrator, CEO of CoreWeave. “With new Grace Blackwell rack-scale systems in hand, many of our customers will be the first to see the benefits and performance of AI innovators operating at scale.”
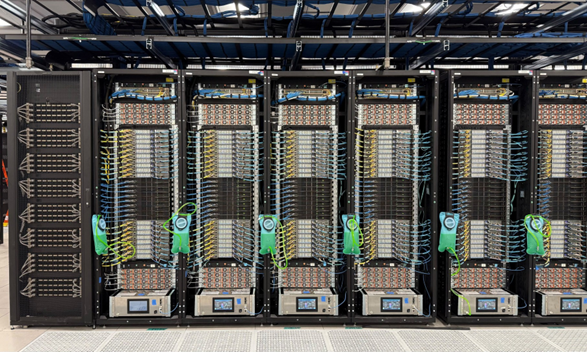
The ramp-up for customers of cloud providers like CoreWeave is underway. Systems built on NVIDIA Grace Blackwell are in full production, transforming cloud data centers into AI factories that manufacture intelligence at scale and convert raw data into real-time insights with speed, accuracy and efficiency.
Leading AI companies around the world are now putting GB200 NVL72’s capabilities to work for AI applications, agentic AI and cutting-edge model development.
Personalized AI Agents
Cohere is using its Grace Blackwell Superchips to help develop secure enterprise AI applications powered by leading-edge research and model development techniques. Its enterprise AI platform, North, enables teams to build personalized AI agents to securely automate enterprise workflows, surface real-time insights and more.
With NVIDIA GB200 NVL72 on CoreWeave, Cohere is already experiencing up to 3x more performance in training for 100 billion-parameter models compared with previous-generation NVIDIA Hopper GPUs — even without Blackwell-specific optimizations.
With further optimizations taking advantage of GB200 NVL72’s large unified memory, FP4 precision and a 72-GPU NVIDIA NVLink domain — where every GPU is connected to operate in concert — Cohere is getting dramatically higher throughput with shorter time to first and subsequent tokens for more performant, cost-effective inference.
“With access to some of the first NVIDIA GB200 NVL72 systems in the cloud, we are pleased with how easily our workloads port to the NVIDIA Grace Blackwell architecture,” said Autumn Moulder, vice president of engineering at Cohere. “This unlocks incredible performance efficiency across our stack — from our vertically integrated North application running on a single Blackwell GPU to scaling training jobs across thousands of them. We’re looking forward to achieving even greater performance with additional optimizations soon.”
AI Models for Enterprise
IBM is using one of the first deployments of NVIDIA GB200 NVL72 systems, scaling to thousands of Blackwell GPUs on CoreWeave, to train its next-generation Granite models, a series of open-source, enterprise-ready AI models. Granite models deliver state-of-the-art performance while maximizing safety, speed and cost efficiency. The Granite model family is supported by a robust partner ecosystem that includes leading software companies embedding large language models into their technologies.
Granite models provide the foundation for solutions like IBM watsonx Orchestrate, which enables enterprises to build and deploy powerful AI agents that automate and accelerate workflows across the enterprise.
CoreWeave’s NVIDIA GB200 NVL72 deployment for IBM also harnesses the IBM Storage Scale System, which delivers exceptional high-performance storage for AI. CoreWeave customers can access the IBM Storage platform within CoreWeave’s dedicated environments and AI cloud platform.
“We are excited to see the acceleration that NVIDIA GB200 NVL72 can bring to training our Granite family of models,” said Sriram Raghavan, vice president of AI at IBM Research. “This collaboration with CoreWeave will augment IBM’s capabilities to help build advanced, high-performance and cost-efficient models for powering enterprise and agentic AI applications with IBM watsonx.”
Compute Resources at Scale
Mistral AI is now getting its first thousand Blackwell GPUs to build the next generation of open-source AI models.
Mistral AI, a Paris-based leader in open-source AI, is using CoreWeave’s infrastructure, now equipped with GB200 NVL72, to speed up the development of its language models. With models like Mistral Large delivering strong reasoning capabilities, Mistral needs fast computing resources at scale.
To train and deploy these models effectively, Mistral AI requires a cloud provider that offers large, high-performance GPU clusters with NVIDIA Quantum InfiniBand networking and reliable infrastructure management. CoreWeave’s experience standing up NVIDIA GPUs at scale with industry-leading reliability and resiliency through tools such as CoreWeave Mission Control met these requirements.
“Right out of the box and without any further optimizations, we saw a 2x improvement in performance for dense model training,” said Thimothee Lacroix, cofounder and chief technology officer at Mistral AI. “What’s exciting about NVIDIA GB200 NVL72 is the new possibilities it opens up for model development and inference.”
A Growing Number of Blackwell Instances
In addition to long-term customer solutions, CoreWeave offers instances with rack-scale NVIDIA NVLink across 72 NVIDIA Blackwell GPUs and 36 NVIDIA Grace CPUs, scaling to up to 110,000 GPUs with NVIDIA Quantum-2 InfiniBand networking.
These instances, accelerated by the NVIDIA GB200 NVL72 rack-scale accelerated computing platform, provide the scale and performance needed to build and deploy the next generation of AI reasoning models and agents.