Editor’s note: This post is part of our weekly In the NVIDIA Studio series, which celebrates featured artists, offers creative tips and tricks, and demonstrates how NVIDIA Studio technology improves creative workflows. We’re also deep diving on new GeForce RTX 40 Series GPU features, technologies and resources, and how they dramatically accelerate content creation.
Gamescom, the biggest gaming event of the year, kicks off tomorrow in Cologne, Germany, but gamers and content creators can find some of the latest innovations, tools and AI-powered tech this week In the NVIDIA Studio.
On the eve of the show’s official opening, NVIDIA announced NVIDIA DLSS 3.5 featuring Ray Reconstruction — a new neural rendering AI model that creates more beautiful and realistic ray-traced visuals than traditional rendering methods — for real-time 3D creative apps and games.
NVIDIA RTX Remix, a free modding platform built on NVIDIA Omniverse and available now, gives people the tools to create and share #RTXON mods for classic games. We also announced Half-Life 2 RTX: An RTX Remix Project, a community remaster project of Valve’s Half-Life 2, one of the highest-rated games of all time.
This week’s In the NVIDIA Studio installment also features digital artist Diyor Makhmudov’s 3D work, inspired by the extraordinary gaming franchise The Witcher.
Reallusion software released an update to the iClone Omniverse Connector, including real-time synchronization of projects and enhanced import functionality for OpenUSD, enabling quicker, more efficient workflows. Learn more in the latest edition of the Into the Omniverse series.
Finally, calling all video editors to sign up for the premiere DaVinci Resolve event — ResolveCon — in Portland, Oregon, from Aug. 25-27. In-person attendees can win giveaways, including new GeForce RTX GPUs, while virtual attendees can view tutorials livestreamed by In the NVIDIA Studio artist Casey Faris.
Next-Level Graphical Fidelity With DLSS 3.5
NVIDIA DLSS 3.5 adds Ray Reconstruction, which improves ray-traced image quality for all GeForce RTX GPUs by replacing hand-tuned denoisers with an NVIDIA supercomputer-trained AI network that generates higher-quality pixels in between sampled rays.
Seeing is believing — watch the Tech Talk with NVIDIA Vice President of Applied Deep Learning Research Bryan Catanzaro to learn how DLSS 3.5 works.
Creative apps with ray-traced renderers face a wide variety of content that is difficult for traditional denoisers to handle, as they require hand-tuning for every scene. As a result, content previews return suboptimal image quality. With DLSS 3.5, the AI neural network recognizes a wide variety of scenes, producing high-quality images during preview and before committing hours to a final render.
D5 Render and Chaos Vantage, two popular professional-grade 3D apps for architects and designers, feature real-time preview modes with ray tracing. With DLSS 3.5, the AI neural network replaces the denoisers, inferring and producing higher-quality previews while building and iterating.
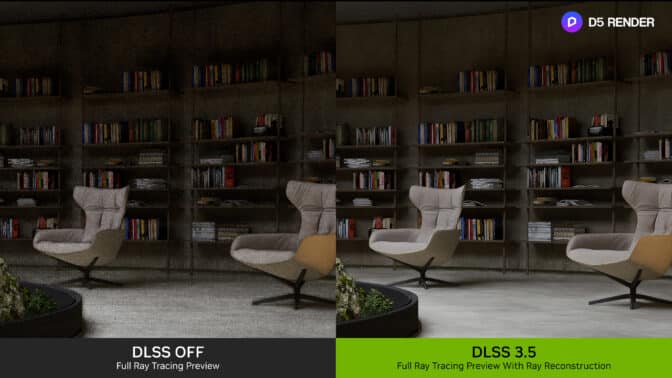
Popular creative apps Chaos Vantage, D5 Render and NVIDIA Omniverse, as well as popular gaming titles Alan Wake 2, Cyberpunk 2077, Cyberpunk 2077: Phantom Liberty and Portal with RTX, are all adding support for NVIDIA DLSS 3.5 this fall.
Developers will be able to seamlessly integrate DLSS 3.5 with the new Streamline SDK coming soon. Learn more about DLSS 3.5.
Half-Life 2 RTX: An RTX Remix Project
Half-Life 2 RTX: An RTX Remix Project is being developed by four of Half-Life 2’s top mod teams, now known as Orbifold Studios.
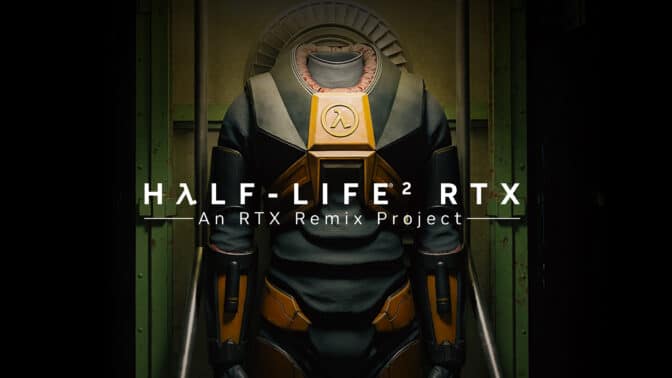
Using the latest version of RTX Remix, Orbifold Studios is rebuilding materials with physically based rendering properties, adding extra geometric detail with Valve’s Hammer editor and using the full range of NVIDIA technologies, including NVIDIA DLSS, NVIDIA RTX IO and NVIDIA Reflex, to breathe new life into the critically acclaimed title.
As with Portal with RTX, a high-fidelity reimagining of Valve’s timeless classic, and Portal: Prelude RTX, built by community modders, nearly every asset in Half-Life 2 RTX: An RTX Remix Project is being reconstructed in high fidelity and with full ray tracing (otherwise known as path tracing, enabling advanced rendering techniques. Compared to the original, some assets feature 20x the geometric detail.
Half-Life 2 RTX: An RTX Remix Project is early in development and is a community effort looking to galvanize talented modders and artists everywhere. To join the project, apply via the Orbifold Studios website.
Eat. Sleep. Game. Create. Repeat.
Already building 3D scenes in immaculate detail, 19-year-old digital creator and 3D lighting artist Diyorbek Makhmudov has the savvy skills of an industry veteran and a bright future ahead.
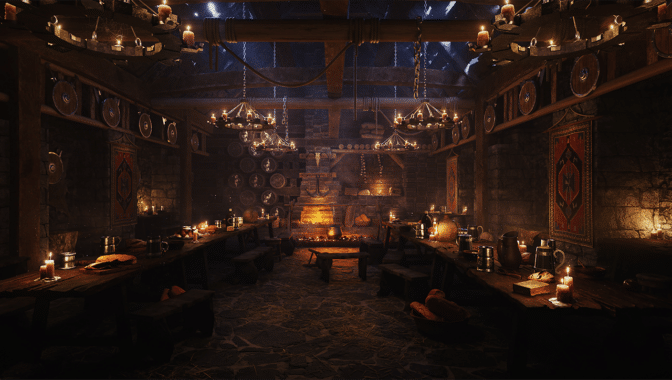
Makhmudov has always had a deep-rooted passion for gaming, gaining inspiration from the 3D worlds of his favorite games. Most notably, The Witcher franchise has fueled him to create 3D worlds that showcase his own signature look and feel.
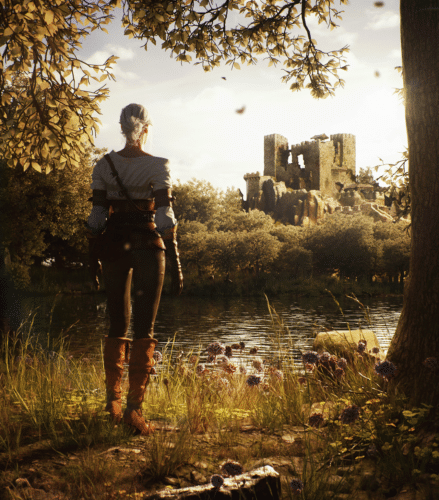
Unlike many content creators featured In the NVIDIA Studio, Makhmudov doesn’t like to bring his own life experience into the creative process, enjoying the escapism offered by world-building.
“I like to immerse myself in another universe,” said Makhmudov. “I don’t like to express my feelings, thoughts or emotions in my creations.”
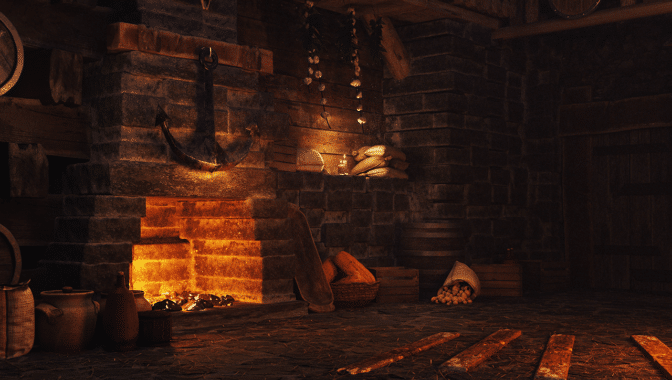
Makhmudov follows standard 3D creative workflow practices: gathering reference material, prepping materials, shaping environments and tinkering with materials, textures and colors. But it’s in 3D creation where he really shines.
Makhmudov uses his preferred 3D app — Cinema 4D — to achieve smooth interactivity while working with complex 3D models thanks to the NVIDIA GPU-accelerated viewport. It’s powered by a GeForce RTX 3090 graphics card, which offers considerable increases in efficiency while fueling creativity.
In the video above, Makhmudov is able to move within the scene, tinkering while the scene renders in real time.
Cinema 4D also supports several popular GPU-accelerated renderers such as Chaos V-Ray, OTOY OctaneRender and Maxon’s Redshift. This flexibility allows Makhmudov to use whichever best suits his needs.
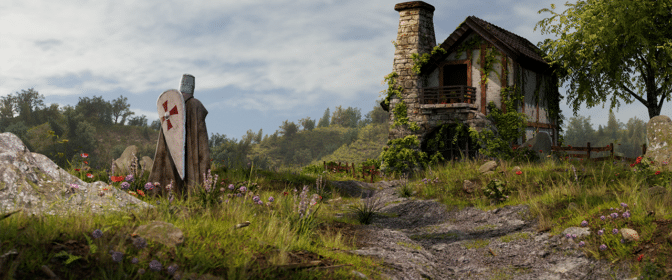
“Redshift is fast, has a good light-linking system and I have almost full control over everything,” said Makhmudov. He prefers OctaneRender for exporting ultra-realistic renders quickly. The built-in Cinema 4D render is also a speedy option. The only thing he can’t do is work on a CPU alone because, to quote Makhmudov, “It’s very slow.”
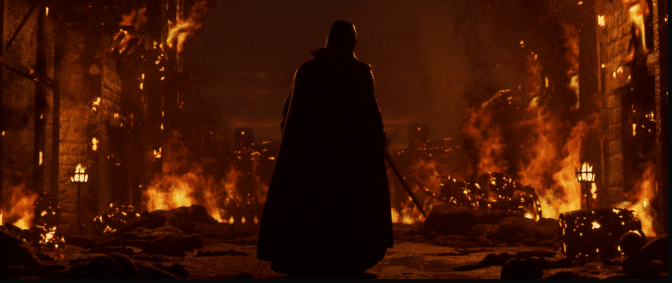
“When you do personal work, it pushes you more to achieve a good result,” said Makhmudov. “As a bonus, that big portfolio will be a major advantage in your job search.”
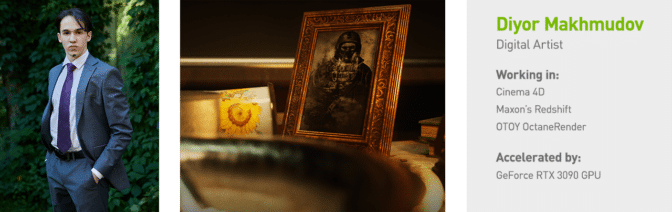
Check out Makhmudov on ArtStation.
Follow NVIDIA Studio on Instagram, Twitter and Facebook. Access tutorials on the Studio YouTube channel and get updates directly in your inbox by subscribing to the Studio newsletter.
Get started with NVIDIA Omniverse by downloading the standard license free, or learn how Omniverse Enterprise can connect your team. Developers can get started with Omniverse resources. Stay up to date on the platform by subscribing to the newsletter, and follow NVIDIA Omniverse on Instagram, Medium and Twitter.
For more, join the Omniverse community and check out the Omniverse forums, Discord server, Twitch and YouTube channels.