Generative AI — in the form of large language model (LLM) applications like ChatGPT, image generators such as Stable Diffusion and Adobe Firefly, and game rendering techniques like NVIDIA DLSS 3 Frame Generation — is rapidly ushering in a new era of computing for productivity, content creation, gaming and more.
At the Microsoft Build developer conference, NVIDIA and Microsoft today showcased a suite of advancements in Windows 11 PCs and workstations with NVIDIA RTX GPUs to meet the demands of generative AI.
More than 400 Windows apps and games already employ AI technology, accelerated by dedicated processors on RTX GPUs called Tensor Cores. Today’s announcements, which include tools to develop AI on Windows PCs, frameworks to optimize and deploy AI, and driver performance and efficiency improvements, will empower developers to build the next generation of Windows apps with generative AI at their core.
“AI will be the single largest driver of innovation for Windows customers in the coming years,” said Pavan Davuluri, corporate vice president of Windows silicon and system integration at Microsoft. “By working in concert with NVIDIA on hardware and software optimizations, we’re equipping developers with a transformative, high-performance, easy-to-deploy experience.”
Develop Models With Windows Subsystem for Linux
AI development has traditionally taken place on Linux, requiring developers to either dual-boot their systems or use multiple PCs to work in their AI development OS while still accessing the breadth and depth of the Windows ecosystem.
Over the past few years, Microsoft has been building a powerful capability to run Linux directly within the Windows OS, called Windows Subsystem for Linux (WSL). NVIDIA has been working closely with Microsoft to deliver GPU acceleration and support for the entire NVIDIA AI software stack inside WSL. Now developers can use Windows PC for all their local AI development needs with support for GPU-accelerated deep learning frameworks on WSL.
With NVIDIA RTX GPUs delivering up to 48GB of RAM in desktop workstations, developers can now work with models on Windows that were previously only available on servers. The large memory also improves the performance and quality for local fine-tuning of AI models, enabling designers to customize them to their own style or content. And because the same NVIDIA AI software stack runs on NVIDIA data center GPUs, it’s easy for developers to push their models to Microsoft Azure Cloud for large training runs.
Rapidly Optimize and Deploy Models
With trained models in hand, developers need to optimize and deploy AI for target devices.
Microsoft released the Microsoft Olive toolchain for optimization and conversion of PyTorch models to ONNX, enabling developers to automatically tap into GPU hardware acceleration such as RTX Tensor Cores. Developers can optimize models via Olive and ONNX, and deploy Tensor Core-accelerated models to PC or cloud. Microsoft continues to invest in making PyTorch and related tools and frameworks work seamlessly with WSL to provide the best AI model development experience.
Improved AI Performance, Power Efficiency
Once deployed, generative AI models demand incredible inference performance. RTX Tensor Cores deliver up to 1,400 Tensor TFLOPS for AI inferencing. Over the last year, NVIDIA has worked to improve DirectML performance to take full advantage of RTX hardware.
On May 24, we’ll release our latest optimizations in Release 532.03 drivers that combine with Olive-optimized models to deliver big boosts in AI performance. Using an Olive-optimized version of the Stable Diffusion text-to-image generator with the popular Automatic1111 distribution, performance is improved over 2x with the new driver.
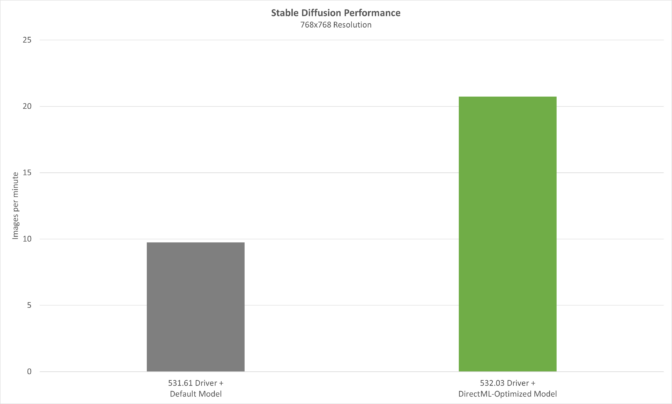
With AI coming to nearly every Windows application, efficiently delivering inference performance is critical — especially for laptops. Coming soon, NVIDIA will introduce new Max-Q low-power inferencing for AI-only workloads on RTX GPUs. It optimizes Tensor Core performance while keeping power consumption of the GPU as low as possible, extending battery life and maintaining a cool, quiet system. The GPU can then dynamically scale up for maximum AI performance when the workload demands it.
Join the PC AI Revolution Now
Top software developers — like Adobe, DxO, ON1 and Topaz — have already incorporated NVIDIA AI technology with more than 400 Windows applications and games optimized for RTX Tensor Cores.
“AI, machine learning and deep learning power all Adobe applications and drive the future of creativity. Working with NVIDIA we continuously optimize AI model performance to deliver the best possible experience for our Windows users on RTX GPUs.” — Ely Greenfield, CTO of digital media at Adobe
“NVIDIA is helping to optimize our WinML model performance on RTX GPUs, which is accelerating the AI in DxO DeepPRIME, as well as providing better denoising and demosaicing, faster.” — Renaud Capolunghi, senior vice president of engineering at DxO
“Working with NVIDIA and Microsoft to accelerate our AI models running in Windows on RTX GPUs is providing a huge benefit to our audience. We’re already seeing 1.5x performance gains in our suite of AI-powered photography editing software.” — Dan Harlacher, vice president of products at ON1
“Our extensive work with NVIDIA has led to improvements across our suite of photo- and video-editing applications. With RTX GPUs, AI performance has improved drastically, enhancing the experience for users on Windows PCs.” — Suraj Raghuraman, head of AI engine development at Topaz Labs
NVIDIA and Microsoft are making several resources available for developers to test drive top generative AI models on Windows PCs. An Olive-optimized version of the Dolly 2.0 large language model is available on Hugging Face. And a PC-optimized version of NVIDIA NeMo large language model for conversational AI is coming soon to Hugging Face.
Developers can also learn how to optimize their applications end-to-end to take full advantage of GPU-acceleration via the NVIDIA AI for accelerating applications developer site.
The complementary technologies behind Microsoft’s Windows platform and NVIDIA’s dynamic AI hardware and software stack will help developers quickly and easily develop and deploy generative AI on Windows 11.
Microsoft Build runs through Thursday, May 25. Tune into to learn more on shaping the future of work with AI.