Greetings, Traveler.
Prepare for adventure. Genshin Impact, the popular open-world action role-playing game, is leaving limited beta and launching for all GeForce NOW members next week.
Gamers can get their game on today with the six total games joining the GeForce NOW library.
As announced last week, Warhammer 40,000: Darktide is coming to the cloud at launch — with GeForce technology. This September, members will be able to leap thousands of years into the future to the time of the Space Marines, streaming on GeForce NOW with NVIDIA DLSS and more.
Plus, the 2.0.41 GeForce NOW app update brings a highly requested feature: in-stream copy-and-paste support from the clipboard while streaming from the PC and Mac apps — so there’s no need to enter a long, complex password for the digital store. Get to your games even faster with this new capability.
GeForce NOW is also giving mobile gamers more options by bringing the perks of RTX 3080 memberships and PC gaming at 120 frames per second to all devices with support for 120Hz phones. The capability is rolling out in the coming weeks.
Take a Trip to Teyvat
After the success of a limited beta and receiving great feedback from members, Genshin Impact is coming next week to everyone streaming on GeForce NOW.
Embark on a journey as a traveler from another world, stranded in the fantastic land of Teyvat. Search for your missing sibling in a vast continent made up of seven nations. Master the art of elemental combat and build a dream team of over 40 uniquely skilled playable characters – like the newest additions of Yelan and Kuki Shinobu – each with their own rich stories, personalities and combat styles.
Experience the immersive campaign, dive deep into rich quests alongside iconic characters and complete daily challenges. Charge head-on into battles solo or invite friends to join the adventures. The world is constantly expanding, so bring it wherever you go across devices, streaming soon to underpowered PCs, Macs and Chromebooks on GeForce NOW.
RTX 3080 members can level up their gaming for the best experience by streaming in 4K resolution and 60 frames per second on the PC and Mac apps.
Let the Gaming Commence
All of the action this GFN Thursday kicks off with six new games arriving on the cloud. Members can also gear up for Rainbow Six Siege Year 7 Season 2.
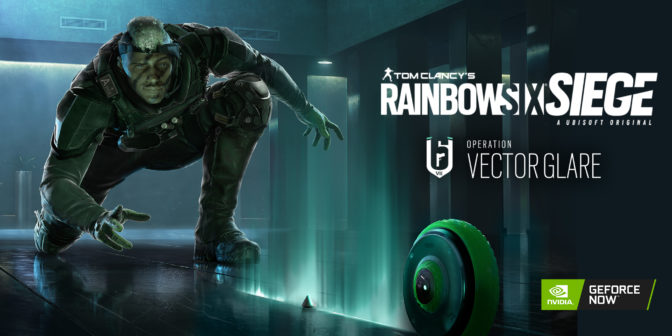
Members can look for the following streaming this week:
- Chivalry 2 (New release on Steam)
- Starship Troopers – Terran Command (New release on Steam and Epic Games Store)
- Builder Simulator (Steam)
- Supraland (Free on Epic Games Store)
- The Legend of Heroes: Trails of Cold Steel II (Steam)
- POSTAL: Brain Damaged (Steam)
Finally, members still have a chance to stream the PC Building Simulator 2 open beta before it ends on Monday, June 20. Experience deeper simulation, an upgraded career mode and powerful new customization features to bring your ultimate PC to life.
To start your weekend gaming adventures, we’ve got a question. Let us know your thoughts on Twitter or in the comments below.
What are there more of in video games?
NPCs or Quests?
—
NVIDIA GeForce NOW (@NVIDIAGFN) June 15, 2022
The post Get Your Wish: Genshin Impact Coming to GeForce NOW appeared first on NVIDIA Blog.