This week’s GFN Thursday packs a prehistoric punch with the release of Jurassic World Evolution 2. It also gets infinitely brighter with the release of Bright Memory: Infinite.
Both games feature NVIDIA RTX technologies and are part of the six titles joining the GeForce NOW library this week.
GeForce NOW RTX 3080 members will get the peak cloud gaming experience in these titles and more. In addition to RTX ON, they’ll stream both games at up to 1440p and 120 frames per second on PC and Mac; and up to 4K on SHIELD.
Preorders for six-month GeForce NOW RTX 3080 memberships are currently available in North America and Europe for $99.99. Sign up today to be among the first to experience next-generation gaming.
The Latest Tech, Streaming From the Cloud
GeForce RTX GPUs give PC gamers the best visual quality and highest frame rates. They also power NVIDIA RTX technologies. And with GeForce RTX 3080-class GPUs making their way to the cloud in the GeForce NOW SuperPOD, the most advanced platform for ray tracing and AI is now available across nearly any low-powered device.
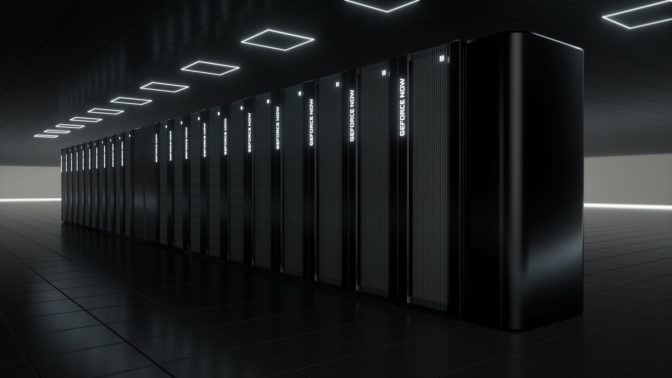
Real-time ray tracing creates the most realistic and immersive graphics in supported games, rendering environments in cinematic quality. NVIDIA DLSS gives games a speed boost with uncompromised image quality, thanks to advanced AI.
With GeForce NOW’s Priority and RTX 3080 memberships, gamers can take advantage of these features in numerous top games, including new releases like Jurassic World Evolution 2 and Bright Memory: Infinite.
The added performance from the latest generation of NVIDIA GPUs also means GeForce NOW RTX 3080 members have exclusive access to stream at up to 1440p at 120 FPS on PC, 1600p at 120 FPS on most MacBooks, 1440p at 120 FPS on most iMacs, 4K HDR at 60 FPS on NVIDIA SHIELD TV and up to 120 FPS on select Android devices.
Welcome to …
Immerse yourself in a world evolved in a compelling, original story, experience the chaos of “what-if” scenarios from the iconic Jurassic World and Jurassic Park films and discover over 75 awe-inspiring dinosaurs, including brand-new flying and marine reptiles. Play with support for NVIDIA DLSS this week on GeForce NOW.
GeForce NOW gives your low-end rig the power to play Jurassic World Evolution 2 with even higher graphics settings thanks to NVIDIA DLSS, streaming from the cloud.
Blinded by the (Ray-Traced) Light
FYQD-studio, a one-man development team that released Bright Memory in 2020, is back with a full-length sequel, Bright Memory: Infinite, streaming from the cloud with RTX ON.
Bright Memory: Infinite combines the FPS and action genres with dazzling visuals, amazing set pieces and exciting action. Mix and match available skills and abilities to unleash magnificent combos on enemies. Cut through the opposing forces with your sword, or lock and load with ranged weaponry, customized with a variety of ammunition. The choice is yours.
Priority and GeForce NOW RTX 3080 members can experience every moment of the action the way FYQD-studio intended, gorgeously rendered with ray-traced reflections, ray-traced shadows, ray-traced caustics and dazzling RTX Global Illumination. And GeForce NOW RTX 3080 members can play at up to 1440p and 120 FPS on PC and Mac.
Never Run Out of Gaming
GFN Thursday always means more games.
Members can find these six new games streaming on the cloud this week:
- Bright Memory: Infinite (new game launch on Steam)
- Epic Chef (new game launch on Steam)
- Jurassic World Evolution 2 (new game on launch on Steam and Epic Games Store)
- MapleStory (Steam)
- Severed Steel (Steam)
- Tale of Immortal (Steam)
We make every effort to launch games on GeForce NOW as close to their release as possible, but, in some instances, games may not be available immediately.
What are you planning to play this weekend? Let us know on Twitter or in the comments below.
The post Catch Some Rays This GFN Thursday With ‘Jurassic World Evolution 2’ and ‘Bright Memory: Infinite’ Game Launches appeared first on The Official NVIDIA Blog.