For a quality conversation between a human and a machine, responses have to be quick, intelligent and natural-sounding.
But up to now, developers of language-processing neural networks that power real-time speech applications have faced an unfortunate trade-off: Be quick and you sacrifice the quality of the response; craft an intelligent response and you’re too slow.
That’s because human conversation is incredibly complex. Every statement builds on shared context and previous interactions. From inside jokes to cultural references and wordplay, humans speak in highly nuanced ways without skipping a beat. Each response follows the last, almost instantly. Friends anticipate what the other will say before words even get uttered.
What Is Conversational AI?
True conversational AI is a voice assistant that can engage in human-like dialogue, capturing context and providing intelligent responses. Such AI models must be massive and highly complex.
But the larger a model is, the longer the lag between a user’s question and the AI’s response. Gaps longer than just three-tenths of a second can sound unnatural.
With NVIDIA GPUs, conversational AI software, and CUDA-X AI libraries, massive, state-of-the-art language models can be rapidly trained and optimized to run inference in just a couple of milliseconds — thousandths of a second — which is a major stride toward ending the trade-off between an AI model that’s fast versus one that’s large and complex.
These breakthroughs help developers build and deploy the most advanced neural networks yet, and bring us closer to the goal of achieving truly conversational AI.
GPU-optimized language understanding models can be integrated into AI applications for such industries as healthcare, retail and financial services, powering advanced digital voice assistants in smart speakers and customer service lines. These high-quality conversational AI tools can allow businesses across sectors to provide a previously unattainable standard of personalized service when engaging with customers.
How Fast Does Conversational AI Have to Be?
The typical gap between responses in natural conversation is about 300 milliseconds. For an AI to replicate human-like interaction, it might have to run a dozen or more neural networks in sequence as part of a multilayered task — all within that 300 milliseconds or less.
Responding to a question involves several steps: converting a user’s speech to text, understanding the text’s meaning, searching for the best response to provide in context, and providing that response with a text-to-speech tool. Each of these steps requires running multiple AI models — so the time available for each individual network to execute is around 10 milliseconds or less.
If it takes longer for each model to run, the response is too sluggish and the conversation becomes jarring and unnatural.
Working with such a tight latency budget, developers of current language understanding tools have to make trade-offs. A high-quality, complex model could be used as a chatbot, where latency isn’t as essential as in a voice interface. Or, developers could rely on a less bulky language processing model that more quickly delivers results, but lacks nuanced responses.
NVIDIA Jarvis is an application framework for developers building highly accurate conversational AI applications that can run far below the 300-millisecond threshold required for interactive apps. Developers at enterprises can start from state-of-the-art models that have been trained for more than 100,000 hours on NVIDIA DGX systems.
Enterprises can apply transfer learning with Transfer Learning Toolkit to fine-tune these models on their custom data. These models are better suited to understand company-specific jargon leading to higher user satisfaction. The models can be optimized with TensorRT, NVIDIA’s high-performance inference SDK, and deployed as services that can run and scale in the data center. Speech and vision can be used together to create apps that make interactions with devices natural and more human-like. Jarvis makes it possible for every enterprise to use world-class conversational AI technology that previously was only conceivable for AI experts to attempt.
What Will Future Conversational AI Sound Like?
Basic voice interfaces like phone tree algorithms (with prompts like “To book a new flight, say ‘bookings’”) are transactional, requiring a set of steps and responses that move users through a pre-programmed queue. Sometimes it’s only the human agent at the end of the phone tree who can understand a nuanced question and solve the caller’s problem intelligently.
Voice assistants on the market today do much more, but are based on language models that aren’t as complex as they could be, with millions instead of billions of parameters. These AI tools may stall during conversations by providing a response like “let me look that up for you” before answering a posed question. Or they’ll display a list of results from a web search rather than responding to a query with conversational language.
A truly conversational AI would go a leap further. The ideal model is one complex enough to accurately understand a person’s queries about their bank statement or medical report results, and fast enough to respond near instantaneously in seamless natural language.
Applications for this technology could include a voice assistant in a doctor’s office that helps a patient schedule an appointment and follow-up blood tests, or a voice AI for retail that explains to a frustrated caller why a package shipment is delayed and offers a store credit.
Demand for such advanced conversational AI tools is on the rise: an estimated 50 percent of searches will be conducted with voice by 2020, and, by 2023, there will be 8 billion digital voice assistants in use.
What Is BERT?
BERT (Bidirectional Encoder Representations from Transformers) is a large, computationally intensive model that set the state of the art for natural language understanding when it was released last year. With fine-tuning, it can be applied to a broad range of language tasks such as reading comprehension, sentiment analysis or question and answer.
Trained on a massive corpus of 3.3 billion words of English text, BERT performs exceptionally well — better than an average human in some cases — to understand language. Its strength is its capability to train on unlabeled datasets and, with minimal modification, generalize to a wide range of applications.
The same BERT can be used to understand several languages and be fine-tuned to perform specific tasks like translation, autocomplete or ranking search results. This versatility makes it a popular choice for developing complex natural language understanding.
At BERT’s foundation is the Transformer layer, an alternative to recurrent neural networks that applies an attention technique — parsing a sentence by focusing attention on the most relevant words that come before and after it.
The statement “There’s a crane outside the window,” for example, could describe either a bird or a construction site, depending on whether the sentence ends with “of the lakeside cabin” or “of my office.” Using a method known as bidirectional or nondirectional encoding, language models like BERT can use context cues to better understand which meaning applies in each case.
Leading language processing models across domains today are based on BERT, including BioBERT (for biomedical documents) and SciBERT (for scientific publications).
How Does NVIDIA Technology Optimize Transformer-Based Models?
The parallel processing capabilities and Tensor Core architecture of NVIDIA GPUs allow for higher throughput and scalability when working with complex language models — enabling record-setting performance for both the training and inference of BERT.
Using the powerful NVIDIA DGX SuperPOD system, the 340 million-parameter BERT-Large model can be trained in under an hour, compared to a typical training time of several days. But for real-time conversational AI, the essential speedup is for inference.
NVIDIA developers optimized the 110 million-parameter BERT-Base model for inference using TensorRT software. Running on NVIDIA T4 GPUs, the model was able to compute responses in just 2.2 milliseconds when tested on the Stanford Question Answering Dataset. Known as SQuAD, the dataset is a popular benchmark to evaluate a model’s ability to understand context.
The latency threshold for many real-time applications is 10 milliseconds. Even highly optimized CPU code results in a processing time of more than 40 milliseconds.
By shrinking inference time down to a couple milliseconds, it’s practical for the first time to deploy BERT in production. And it doesn’t stop with BERT — the same methods can be used to accelerate other large, Transformer-based natural language models like GPT-2, XLNet and RoBERTa.
To work toward the goal of truly conversational AI, language models are getting larger over time. Future models will be many times bigger than those used today, so NVIDIA built and open-sourced the largest Transformer-based AI yet: GPT-2 8B, an 8.3 billion-parameter language processing model that’s 24x bigger than BERT-Large.
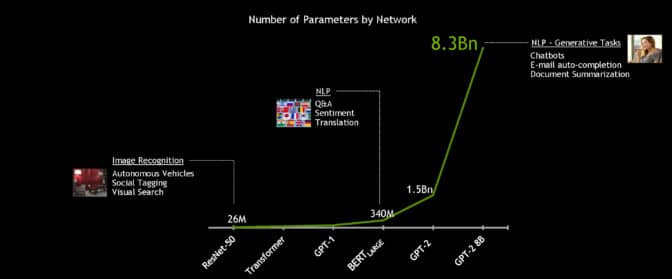
Learn How to Build Your Own Transformer-Based Natural Language Processing Applications
The NVIDIA Deep Learning Institute offers instructor-led, hands-on training on the fundamental tools and techniques for building Transformer-based natural language processing models for text classification tasks, such as categorizing documents. Taught by an expert, this in-depth, 8-hour workshop instructs participants in being able to:
- Understand how word embeddings have rapidly evolved in NLP tasks, from Word2Vec and recurrent neural network-based embeddings to Transformer-based contextualized embeddings.
- See how Transformer architecture features, especially self-attention, are used to create language models without RNNs.
- Use self-supervision to improve the Transformer architecture in BERT, Megatron and other variants for superior NLP results.
- Leverage pre-trained, modern NLP models to solve multiple tasks such as text classification, NER and question answering.
- Manage inference challenges and deploy refined models for live applications.
Earn a DLI certificate to demonstrate subject-matter competency and accelerate your career growth. Take this workshop at an upcoming GTC or request a workshop for your organization.
For more information on conversational AI, training BERT on GPUs, optimizing BERT for inference and other projects in natural language processing, check out the NVIDIA Developer Blog.