MLOps may sound like the name of a shaggy, one-eyed monster, but it’s actually an acronym that spells success in enterprise AI.
A shorthand for machine learning operations, MLOps is a set of best practices for businesses to run AI successfully.
MLOps is a relatively new field because commercial use of AI is itself fairly new.
MLOps: Taking Enterprise AI Mainstream
The Big Bang of AI sounded in 2012 when a researcher won an image-recognition contest using deep learning. The ripples expanded quickly.
Today, AI translates web pages and automatically routes customer service calls. It’s helping hospitals read X-rays, banks calculate credit risks and retailers stock shelves to optimize sales.
In short, machine learning, one part of the broad field of AI, is set to become as mainstream as software applications. That’s why the process of running ML needs to be as buttoned down as the job of running IT systems.
Machine Learning Layered on DevOps
MLOps is modeled on the existing discipline of DevOps, the modern practice of efficiently writing, deploying and running enterprise applications. DevOps got its start a decade ago as a way warring tribes of software developers (the Devs) and IT operations teams (the Ops) could collaborate.
MLOps adds to the team the data scientists, who curate datasets and build AI models that analyze them. It also includes ML engineers, who run those datasets through the models in disciplined, automated ways.
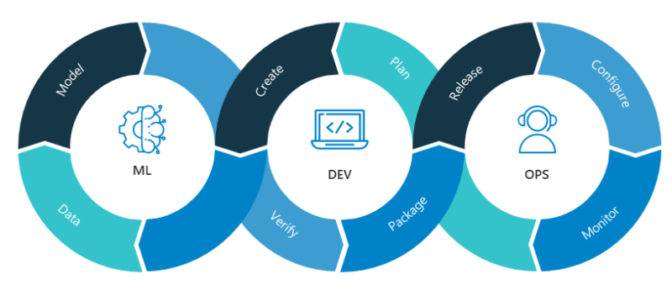
It’s a big challenge in raw performance as well as management rigor. Datasets are massive and growing, and they can change in real time. AI models require careful tracking through cycles of experiments, tuning and retraining.
So, MLOps needs a powerful AI infrastructure that can scale as companies grow. For this foundation, many companies use NVIDIA DGX systems, CUDA-X and other software components available on NVIDIA’s software hub, NGC.
Lifecycle Tracking for Data Scientists
With an AI infrastructure in place, an enterprise data center can layer on the following elements of an MLOps software stack:
- Data sources and the datasets created from them
- A repository of AI models tagged with their histories and attributes
- An automated ML pipeline that manages datasets, models and experiments through their lifecycles
- Software containers, typically based on Kubernetes, to simplify running these jobs
It’s a heady set of related jobs to weave into one process.
Data scientists need the freedom to cut and paste datasets together from external sources and internal data lakes. Yet their work and those datasets need to be carefully labeled and tracked.
Likewise, they need to experiment and iterate to craft great models well torqued to the task at hand. So they need flexible sandboxes and rock-solid repositories.
And they need ways to work with the ML engineers who run the datasets and models through prototypes, testing and production. It’s a process that requires automation and attention to detail so models can be easily interpreted and reproduced.
Today, these capabilities are becoming available as part of cloud-computing services. Companies that see machine learning as strategic are creating their own AI centers of excellence using MLOps services or tools from a growing set of vendors.
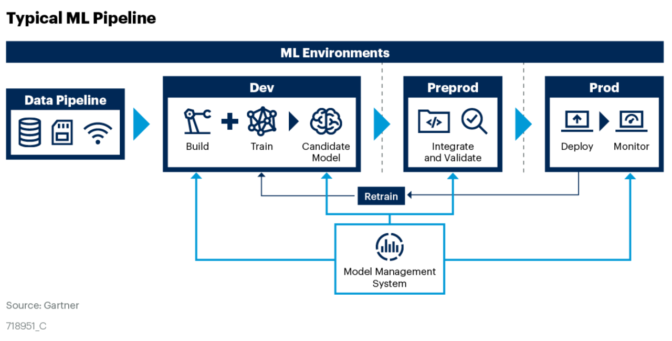
Data Science in Production at Scale
In the early days, companies such as Airbnb, Facebook, Google, NVIDIA and Uber had to build these capabilities themselves.
“We tried to use open source code as much as possible, but in many cases there was no solution for what we wanted to do at scale,” said Nicolas Koumchatzky, a director of AI infrastructure at NVIDIA.
“When I first heard the term MLOps, I realized that’s what we’re building now and what I was building before at Twitter,” he added.
Koumchatzky’s team at NVIDIA developed MagLev, the MLOps software that hosts NVIDIA DRIVE, our platform for creating and testing autonomous vehicles. As part of its foundation for MLOps, it uses the NVIDIA Container Runtime and Apollo, a set of components developed at NVIDIA to manage and monitor Kubernetes containers running across huge clusters.
Laying the Foundation for MLOps at NVIDIA
Koumchatzky’s team runs its jobs on NVIDIA’s internal AI infrastructure based on GPU clusters called DGX PODs. Before the jobs start, the infrastructure crew checks whether they are using best practices.
First, “everything must run in a container — that spares an unbelievable amount of pain later looking for the libraries and runtimes an AI application needs,” said Michael Houston, whose team builds NVIDIA’s AI systems including Selene, a DGX SuperPOD recently ranked the most powerful industrial computer in the U.S.
Among the team’s other checkpoints, jobs must:
- Launch containers with an approved mechanism
- Prove the job can run across multiple GPU nodes
- Show performance data to identify potential bottlenecks
- Show profiling data to ensure the software has been debugged
The maturity of MLOps practices used in business today varies widely, according to Edwin Webster, a data scientist who started the MLOps consulting practice a year ago for Neal Analytics and wrote an article defining MLOps. At some companies, data scientists still squirrel away models on their personal laptops, others turn to big cloud-service providers for a soup-to-nuts service, he said.
Two MLOps Success Stories
Webster shared success stories from two of his clients.
One involves a large retailer that used MLOps capabilities in a public cloud service to create an AI service that reduced waste 8-9 percent with daily forecasts of when to restock shelves with perishable goods. A budding team of data scientists at the retailer created datasets and built models; the cloud service packed key elements into containers, then ran and managed the AI jobs.
Another involves a PC maker that developed software using AI to predict when its laptops would need maintenance so it could automatically install software updates. Using established MLOps practices and internal specialists, the OEM wrote and tested its AI models on a fleet of 3,000 notebooks. The PC maker now provides the software to its largest customers.
Many, but not all, Fortune 100 companies are embracing MLOps, said Shubhangi Vashisth, a senior principal analyst following the area at Gartner. “It’s gaining steam, but it’s not mainstream,” she said.
Vashisth co-authored a white paper that lays out three steps for getting started in MLOps: Align stakeholders on the goals, create an organizational structure that defines who owns what, then define responsibilities and roles — Gartner lists a dozen of them.
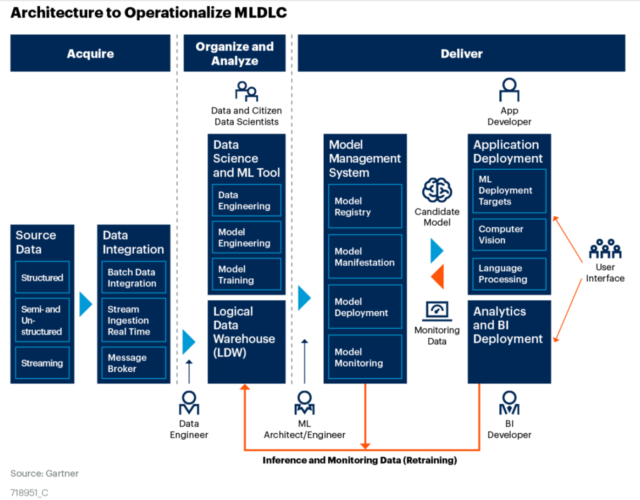
Beware Buzzwords: AIOps, DLOps, DataOps, and More
Don’t get lost in a forest of buzzwords that have grown up along this avenue. The industry has clearly coalesced its energy around MLOps.
By contrast, AIOps is a narrower practice of using machine learning to automate IT functions. One part of AIOps is IT operations analytics, or ITOA. Its job is to examine the data AIOps generate to figure out how to improve IT practices.
Similarly, some have coined the terms DataOps and ModelOps to refer to the people and processes for creating and managing datasets and AI models, respectively. Those are two important pieces of the overall MLOps puzzle.
Interestingly, every month thousands of people search for the meaning of DLOps. They may imagine DLOps are IT operations for deep learning. But the industry uses the term MLOps, not DLOps, because deep learning is a part of the broader field of machine learning.
Despite the many queries, you’d be hard pressed to find anything online about DLOps. By contrast, household names like Google and Microsoft as well as up-and-coming companies like Iguazio and Paperspace have posted detailed white papers on MLOps.
MLOps: An Expanding Software and Services Smorgasbord
Those who prefer to let someone else handle their MLOps have plenty of options.
Major cloud-service providers like Alibaba, AWS and Oracle are among several that offer end-to-end services accessible from the comfort of your keyboard.
For users who spread their work across multiple clouds, DataBricks’ MLFlow supports MLOps services that work with multiple providers and multiple programming languages, including Python, R and SQL. Other cloud-agnostic alternatives include open source software such as Polyaxon and KubeFlow.
Companies that believe AI is a strategic resource they want behind their firewall can choose from a growing list of third-party providers of MLOps software. Compared to open-source code, these tools typically add valuable features and are easier to put into use.
NVIDIA certified products from six of them as part of its DGX-Ready Software program-:
- Allegro AI
- cnvrg.io
- Core Scientific
- Domino Data Lab
- Iguazio
- Paperspace
All six vendors provide software to manage datasets and models that can work with Kubernetes and NGC.
It’s still early days for off-the-shelf MLOps software.
Gartner tracks about a dozen vendors offering MLOps tools including ModelOp and ParallelM now part of DataRobot, said analyst Vashisth. Beware offerings that don’t cover the entire process, she warns. They force users to import and export data between programs users must stitch together themselves, a tedious and error-prone process.
The edge of the network, especially for partially connected or unconnected nodes, is another underserved area for MLOps so far, said Webster of Neal Analytics.
Koumchatzky, of NVIDIA, puts tools for curating and managing datasets at the top of his wish list for the community.
“It can be hard to label, merge or slice datasets or view parts of them, but there is a growing MLOps ecosystem to address this. NVIDIA has developed these internally, but I think it is still undervalued in the industry.” he said.
Long term, MLOps needs the equivalent of IDEs, the integrated software development environments like Microsoft Visual Studio that apps developers depend on. Meanwhile Koumchatzky and his team craft their own tools to visualize and debug AI models.
The good news is there are plenty of products for getting started in MLOps.
In addition to software from its partners, NVIDIA provides a suite of mainly open-source tools for managing an AI infrastructure based on its DGX systems, and that’s the foundation for MLOps. These software tools include:
- Foreman and MAAS (Metal as a Service) for provisioning individual systems
- Ansible and Git for cluster configuration management
- Data Center GPU Manager (DCGM) and NVIDIA System Management (NVSM) for monitoring and reporting
- NVIDIA Container Runtime to launch GPU-aware containers and NVIDIA GPU Operator to simplify GPU management in Kubernetes
- Triton Inference Server and TensorRT to deploy AI models in production
- And DeepOps for scripts and instructions on how to deploy and orchestrate all of the elements above
Many are available on NGC and other open source repositories. Pulling these ingredients into a recipe for success, NVIDIA provides a reference architecture for creating GPU clusters called DGX PODs.
In the end, each team needs to find the mix of MLOps products and practices that best fits its use cases. They all share a goal of creating an automated way to run AI smoothly as a daily part of a company’s digital life.
The post What Is MLOps? appeared first on The Official NVIDIA Blog.