Only a dream just a few years ago, real-time ray tracing has become the new reality in graphics because of NVIDIA RTX — and it’s just getting started.
The world’s top gaming franchises, the most popular gaming engines and scores of creative applications across industries are all onboard for real-time ray tracing.
Leading studios, design firms and industry luminaries are using real-time ray tracing to advance content creation and drive new possibilities in graphics, including virtual productions for television, interactive virtual reality experiences, and realistic digital humans and animations.
The Future Group and Riot Games used NVIDIA RTX to deliver the world’s first ray-traced broadcast. Rob Legato, the VFX supervisor for Disney’s recent remake of The Lion King, referred to real-time rendering with GPUs serving as the future of creativity. And developers have adopted real-time techniques to create cinematic video game graphics, like ray-traced reflections in Battlefield V, ray-traced shadows in Shadow of the Tomb Raider and path-traced lighting in Minecraft.
These are just a few of many examples.
In early 2018, ILMxLAB, Epic Games and NVIDIA released a cinematic called Star Wars: Reflections. We revealed that the demo was rendered in real time using ray-traced reflections, area light shadows and ambient occlusion — all on a $70,000 NVIDIA DGX workstation packed with four NVIDIA Volta GPUs. This major advancement captured global attention, as real-time ray tracing with this level of fidelity could only be done offline on gigantic server farms.
Fast forward to August 2018, when we announced the GeForce RTX 2080 Ti at Gamescom and showed Reflections running on just one $1,200 GeForce RTX GPU, with the NVIDIA Turing architecture’s RT Cores accelerating ray tracing performance in real time.
Today, over 50 content creation and design applications, including 20 of the leading commercial renderers, have added support for NVIDIA RTX. Real-time ray tracing is more widely available, allowing professionals to have more time for iterating designs and capturing accurate lighting, shadows, reflections, translucence, scattering and ambient occlusion in their images.
RTX Ray Tracing Continues to Change the Game
From product and building designs to visual effects and animation, real-time ray tracing is revolutionizing content creation. RTX allows creative decisions to be made sooner, as designers no longer need to play the waiting game for renders to complete.
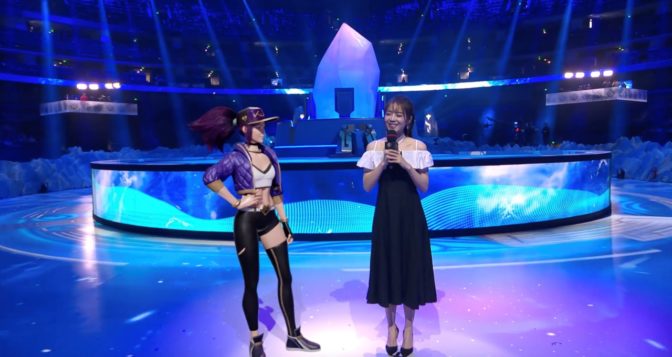
What was once considered impossible just two years ago has now become a reality for anyone with an RTX GPU — NVIDIA’s Turing architecture delivers new capabilities that made real-time ray tracing achievable. Its RT Cores accelerate two of the most computationally intensive tasks: bounding volume hierarchy traversal and ray-triangle intersection testing. This allows the streaming multiprocessors, which perform the computations, to improve programmable shading instead of spending thousands of instruction slots for each ray cast.
Turing’s Tensor Cores enable users to leverage and enhance AI denoising for generating clean images quickly. All of these new features combined are what make real-time ray tracing possible. Creative teams can render images faster, complete more iterations and finish projects with cinematic, photorealistic graphics.
“Ray tracing, especially real-time ray tracing, brings the ground truth to an image and allows the viewer to make immediate, sometimes subconscious decisions about the information,” said Jon Peddie, president of Jon Peddie Research. “If it’s entertainment, the viewer is not distracted and taken out of the story by artifacts and nagging suspension of belief. If it’s engineering, the user knows the results are accurate and can move closer and more quickly to a solution.”
Artists can now use a single GPU for real-time ray tracing to create high-quality imagery, and they can harness the power of RTX through numerous ways. Popular game engines Unity and Unreal Engine are leveraging RTX. GPU renderers like V-Ray, Redshift and Octane are adopting OptiX for RTX acceleration. And workstation vendors like BOXX, Dell, HP, Lenovo and Supermicro offer real-time ray tracing-capable systems, allowing users to harness the power of RTX in a single, flexible desktop or mobile workstation.
RTX GPUs also provide the memory required for handling massive datasets, whether it’s complex geometry or large numbers of high-resolution textures. The NVIDIA Quadro RTX 8000 GPU provides a 48GB frame buffer, and with NVLink high-speed interconnect technology doubling that capacity, users can easily manipulate massive, complex scenes without spending time constantly decimating or optimizing their datasets.
“DNEG’s virtual production department has taken on an ever increasing amount of work, particularly over recent months where practical shoots have become more difficult,” said Stephen Willey, head of technology at DNEG. “NVIDIA’s RTX and Quadro Sync solutions, coupled with Epic’s Unreal Engine, have allowed us to create far larger and more realistic real-time scenes and assets. These advances help us offer exciting new possibilities to our clients.”
More recently, NVIDIA introduced techniques to further improve ray tracing and rendering. With Deep Learning Super Sampling, users can enhance real-time rendering through AI-based super resolution. NVIDIA DLSS allows them to render fewer pixels and use AI to construct sharp, higher-resolution images.
At SIGGRAPH this month, one of our research papers dives deep into how to render dynamic direct lighting and shadows from millions of area lights in real time using a new technique called reservoir-based spatiotemporal importance resampling, or ReSTIR.
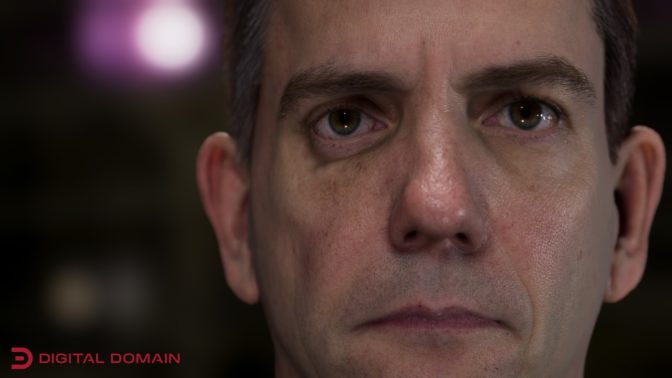
Real-Time Ray Tracing Opens New Possibilities for Graphics
RTX ray tracing is transforming design across industries today.
In gaming, the quality of RTX ray tracing creates new dynamics and environments in gameplay, allowing players to use reflective surfaces strategically. For virtual reality, RTX ray tracing brings new levels of realism and immersiveness for professionals in healthcare, AEC and automotive design. And in animation, ray tracing is changing the pipeline completely, enabling artists to easily manage and manipulate light geometry in real time.
Real-time ray tracing is also paving the way for virtual productions and believable digital humans in film, television and immersive experiences like VR and AR.
And with NVIDIA Omniverse — the first real-time ray tracer that can scale to any number of GPUs — creatives can simplify collaborative studio workflows with their favorite applications like Unreal Engine, Autodesk Maya and 3ds Max, Substance Painter by Adobe, Unity, SideFX Houdini, and many others. Omniverse is pushing ray tracing forward, enabling users to create visual effects, architectural visualizations and manufacturing designs with dynamic lighting and physically based materials.
Explore the Latest in Ray Tracing and Graphics
Join us at the SIGGRAPH virtual conference to learn more about the latest advances in graphics, and get an exclusive look at some of our most exciting work.
Be part of the NVIDIA community and show us what you can create by participating in our real-time ray tracing contest. The selected winner will receive the latest Quadro RTX graphics card and a free pass to discover what’s new in graphics at NVIDIA GTC, October 5-9.
The post Real-Time Ray Tracing Realized: RTX Brings the Future of Graphics to Millions appeared first on The Official NVIDIA Blog.