NVIDIA and AWS are bringing the future of XR streaming to the cloud.
Announced today, the NVIDIA CloudXR platform will be available on Amazon EC2 P3 and G4 instances, which support NVIDIA V100 and T4 GPUs, allowing cloud users to stream high-quality immersive experiences to remote VR and AR devices.
The CloudXR platform includes the NVIDIA CloudXR software development kit, NVIDIA Virtual Workstation software and NVIDIA AI SDKs to deliver photorealistic graphics, with the mobile convenience of all-in-one XR headsets. XR is a collective term for VR, AR and mixed reality.
With the ability to stream from the cloud, professionals can now easily set up, scale and access immersive experiences from anywhere — they no longer need to be tethered to expensive workstations or external VR tracking systems.
The growing availability of advanced tools like CloudXR is paving the way for enhanced collaboration, streamlined workflows and high fidelity virtual environments. XR solutions are also introducing new possibilities for adding AI features and functionality.
With the CloudXR platform, many early access customers and partners across industries like manufacturing, media and entertainment, healthcare and others are enhancing immersive experiences by combining photorealistic graphics with the mobility of wireless head-mounted displays.
Lucid Motors recently announced the new Lucid Air, a powerful and efficient electric vehicle that users can experience through a custom implementation of the ZeroLight platform. Lucid Motors is developing a virtual design showroom using the CloudXR platform. By streaming the experience from AWS, shoppers can enter the virtual environment and see the advanced features of Lucid Air.
“NVIDIA CloudXR allows people all over the world to experience an incredibly immersive, personalized design with the new Lucid Air,“ said Thomas Orenz, director of digital interactive marketing at Lucid Motors. “By using the AWS cloud, we can save on infrastructure costs by removing the need for onsite servers, while also dynamically scaling the VR configuration experiences for our customers.”
Another early adopter of CloudXR on AWS is The Gettys Group, a hospitality design, branding and development company based in Chicago. Gettys frequently partners with visualization company Theia Interactive to turn the design process into interactive Unreal Engine VR experiences.
When the coronavirus pandemic hit, Gettys and Theia used NVIDIA CloudXR to deliver customer projects to a local Oculus Quest HMD, streaming from the AWS EC2 P3 instance with NVIDIA Virtual Workstations.
“This is a game changer — by streaming collaborative experiences from AWS, we can digitally bring project stakeholders together on short notice for quick VR design alignment meetings,” said Ron Swidler, chief innovation officer at The Gettys Group. “This is going to save a ton of time and money, but more importantly it’s going to increase client engagement, understanding and satisfaction.”
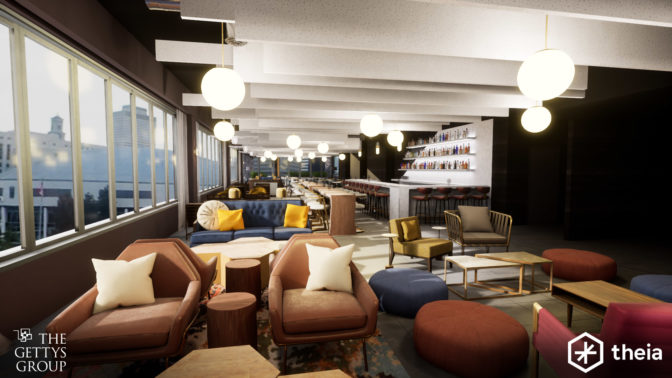
Next-Level Streaming from the Cloud
CloudXR is built on NVIDIA RTX GPUs to allow streaming of immersive AR, VR or mixed reality experiences from anywhere.
The platform includes:
- NVIDIA CloudXR SDK, which provides support for all OpenVR apps and includes broad client support for phones, tablets and HMDs. Its adaptive streaming protocol delivers the richest experiences with the lowest perceived latency by constantly adapting to network conditions.
- NVIDIA Virtual Workstations to deliver the most immersive, highest quality graphics at the fastest frame rates. It’s available from cloud providers such as AWS, or can be deployed from an enterprise data center.
- NVIDIA AI SDKs to accelerate performance and enhance immersive presence.
With the NVIDIA CloudXR platform on Amazon EC2 G4 and P3 instances supporting NVIDIA T4 and V100 GPUs, companies can deliver high-quality virtual experiences to any user, anywhere in the world.
Availability Coming Soon
NVIDIA CloudXR on AWS will be generally available early next year, with a private beta available in the coming months. Sign up now to get the latest news and updates on upcoming CloudXR releases, including the private beta.
The post NVIDIA Delivers Streaming AR and VR from the Cloud with AWS appeared first on The Official NVIDIA Blog.