Cohere, OpenAI, and AI21 Labs have developed a preliminary set of best practices applicable to any organization developing or deploying large language models.OpenAI Blog
Powering Next Generation Applications with OpenAI Codex

OpenAI Codex, a natural language-to-code system based on GPT-3, helps turn simple English instructions into over a dozen popular coding languages. Codex was released last August through our API and is the principal building block of GitHub Copilot.
Our motivation behind Codex is to supplement developers’ work and increase productivity. Codex helps computers to better understand people’s intent, which enables everyone to do more with computers. This is an integral part of our mission to build general-purpose AI that benefits all of humanity.
For enterprise customers, Microsoft’s Azure OpenAI Service provides developers with access to Codex and our other models, like GPT-3 and embeddings, along with enterprise-grade capabilities that are built into Microsoft Azure. At its Build conference today, Microsoft announced that Azure OpenAI Service—previously available by invitation only—is now available in a limited access preview. We’re already seeing new applications of Azure OpenAI Service across many industry verticals, from healthcare to financial services.
Applications and Industries
Since its release via our API, we’ve been working closely with developers to build on top of Codex. These applications utilize the system’s capabilities in a variety of categories including creativity, learning, productivity and problem solving.
Applications using Codex:
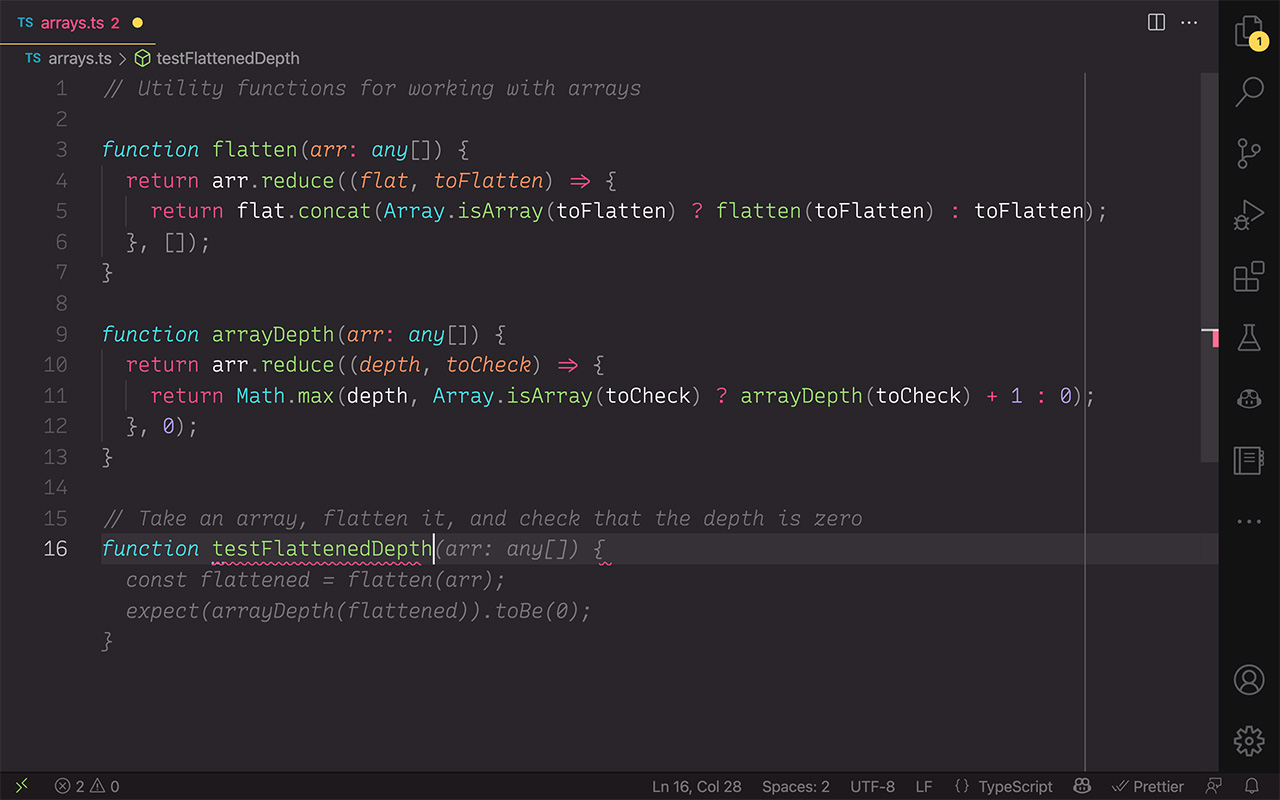
GitHub Copilot is an AI pair programmer that provides suggestions for whole lines or entire functions right inside the code editor.
Through tight integration with Codex, GitHub Copilot can convert comments to code, autofill repetitive code, suggest tests and show alternatives.
Available for Visual Studio and Visual Studio Code, among other environments, GitHub Copilot works with a broad set of frameworks and languages, and for some programming languages suggests approximately 35% of the code generated by tens of thousands of developers who use it today.
Microsoft announced at its Build developer conference that GitHub Copilot will move to general availability this summer.
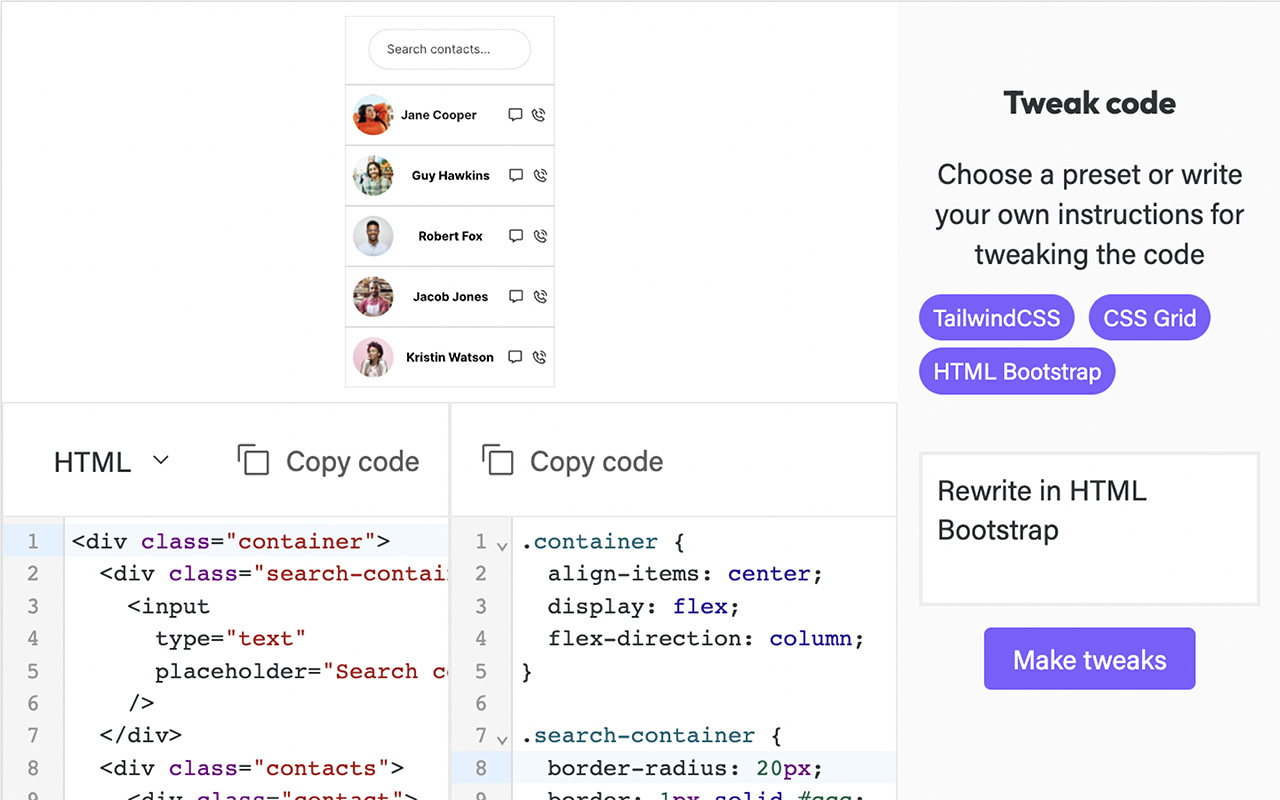
Pygma aims to turn Figma designs into high-quality code.
Pygma utilizes Codex to turn Figma designs into different frontend frameworks and match the coding style and preferences of the developer. Codex enables Pygma to help developers do tasks instantly that previously could have taken hours.
“Codex has allowed me to integrate innovative features into my app with very little coding. As someone without a strong machine learning background, certain features like flexible code-tweaking would be incredibly difficult to build in-house. With Codex, it works almost out of the box.”
—Emile Paffard-Wray, Founder, Pygma
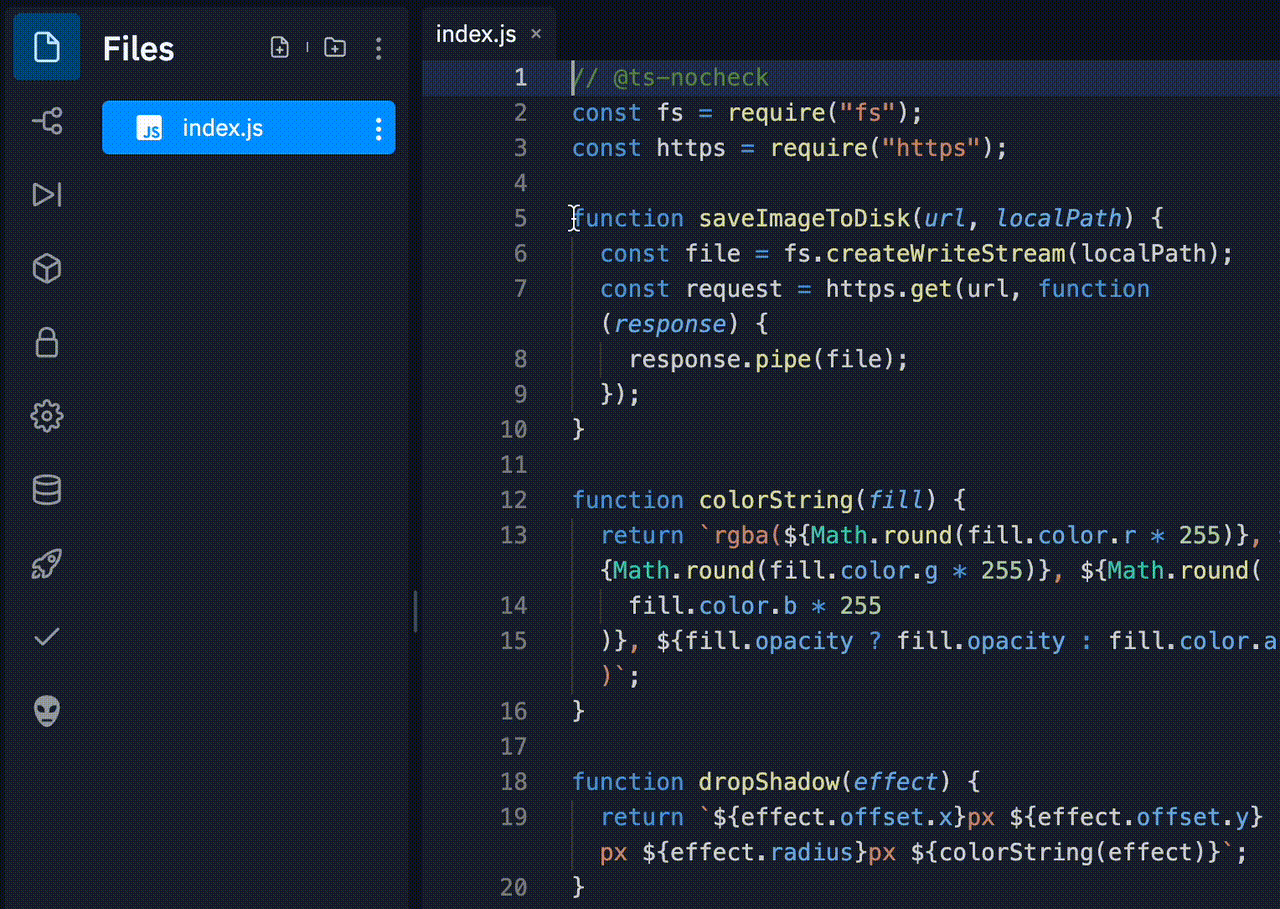
Replit is a programming platform for any programming language that lets users collaborate live on projects, learn about code and share work with a community of learners and builders.
Replit leverages Codex to describe what a selection of code is doing in simple language so everyone can get quality explanation and learning tools. Users can highlight selections of code and click “Explain Code” to use Codex to understand its functionality.
“Codex helps learning on Replit better understand code they encounter. We’ve only scratched the surface of what semantic code understanding can offer those who want to get from idea to working code quickly.”
—Amjad Masad, Founder, Replit
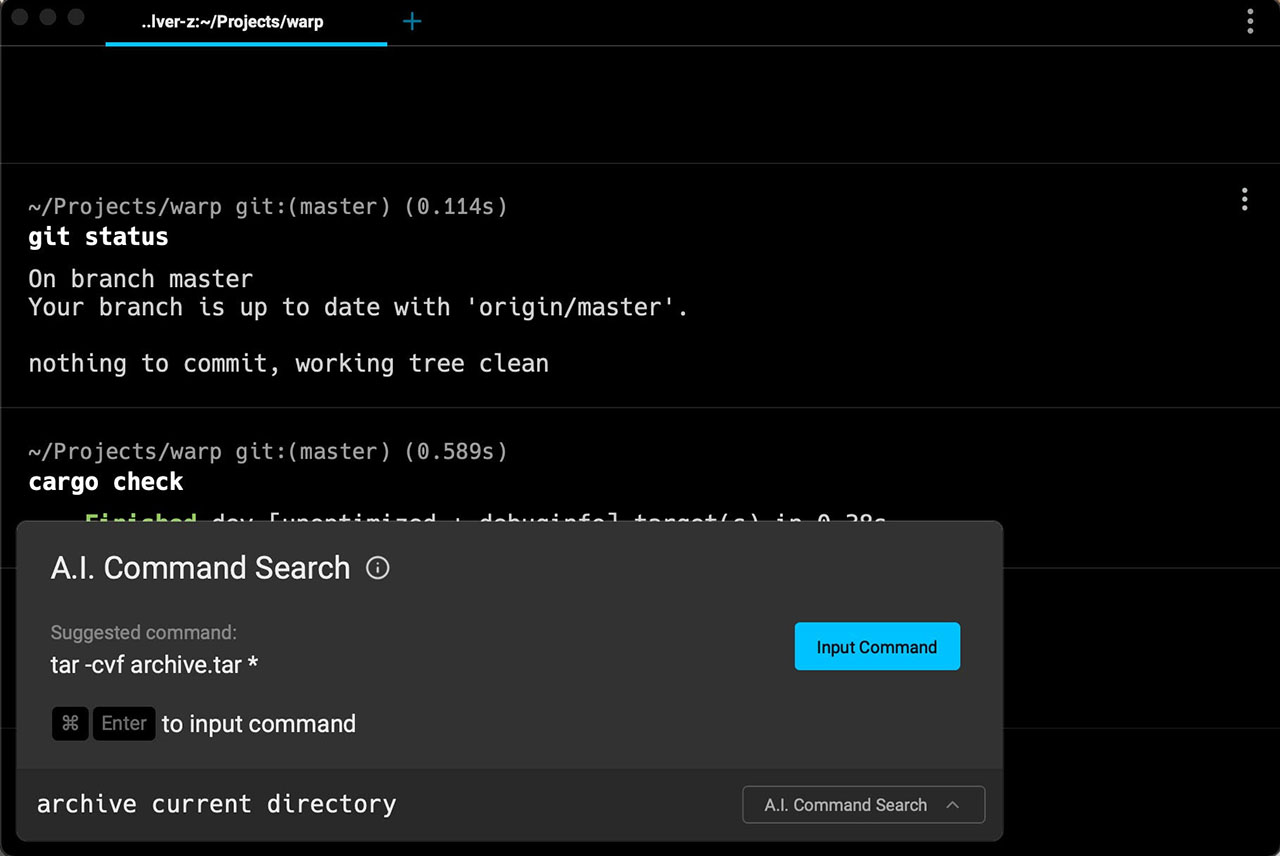
Warp is a Rust-based terminal, reimagined from the ground up to help both individuals and teams be more productive in the command-line.
Terminal commands are typically difficult to remember, find and construct. Users often have to leave the terminal and search the web for answers and even then the results might not give them the right command to execute. Warp uses Codex to allow users to run a natural language command to search directly from within the terminal and get a result they can immediately use.
“Codex allows Warp to make the terminal more accessible and powerful. Developers search for entire commands using natural language rather than trying to remember them or assemble them piecemeal. Codex-powered command search has become one of our game changing features.
—Zach Lloyd, Founder, Warp
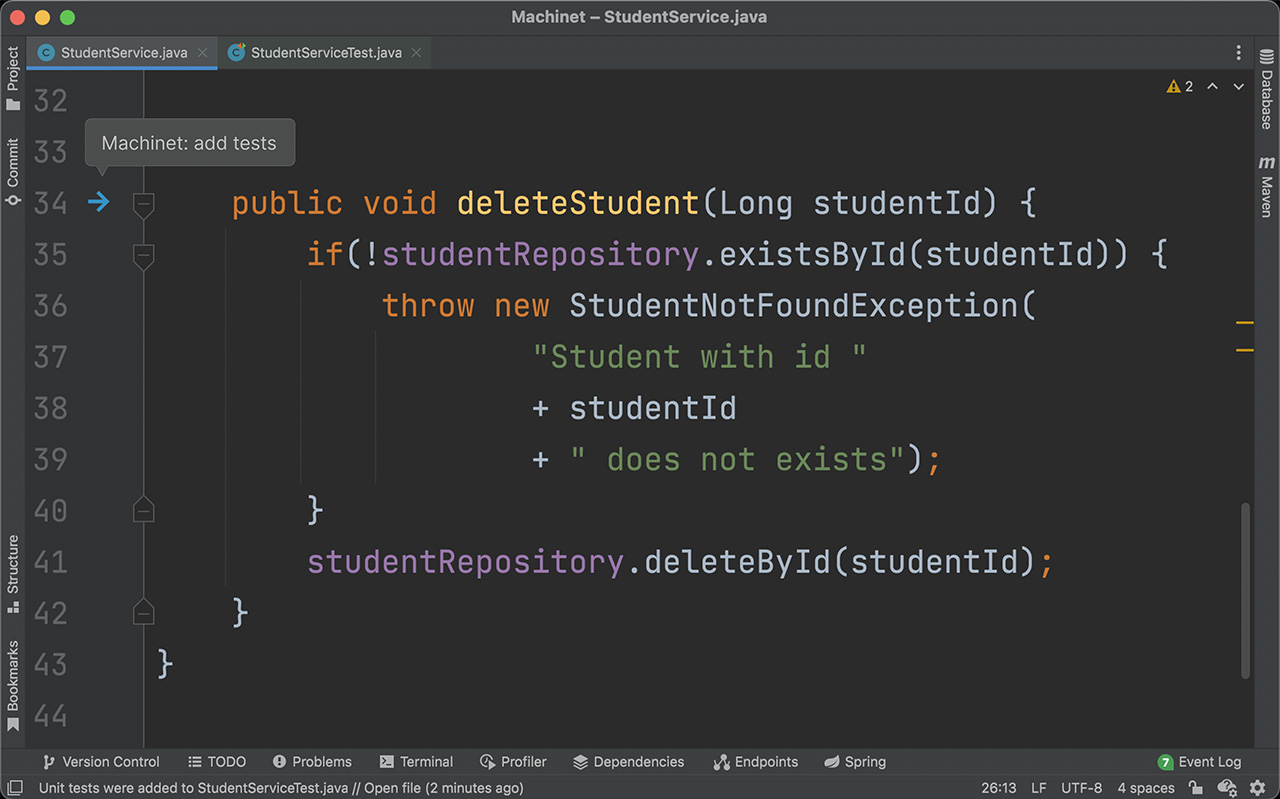
Machinet helps professional Java developers write quality code by using Codex to generate intelligent unit test templates.
Machinet was able to accelerate their development several-fold by switching from building their own machine learning systems to using Codex. The flexibility of Codex allows for the ability to easily add new features and capabilities saving their users time and helping them be more productive.
“Codex is an amazing tool in our arsenal. Not only does it allow us to generate more meaningful code, but it has also helped us find a new design of product architecture and got us out of a local maximum.”
—Vladislav Yanchenko, Founder, Machinet
OpenAI
Powering next generation applications with OpenAI Codex
Codex is now powering 70 different applications across a variety of use cases through the OpenAI API.OpenAI Blog
DALL·E 2 Research Preview Update
Last month, we started previewing DALL·E 2 to a limited number of trusted users to learn about the technology’s capabilities and limitations.
Since then, we’ve been working with our users to actively incorporate the lessons we learn. As of today:
- Our users have collectively created over 3 million images with DALL·E.
- We’ve enhanced our safety system, improving the text filters and tuning the automated detection & response system for content policy violations.
- Less than 0.05% of downloaded or publicly shared images were flagged as potentially violating our content policy. About 30% of those flagged images were confirmed by human reviewers to be policy violations, leading to an account deactivation.
- As we work to understand and address the biases that DALL·E has inherited from its training data, we’ve asked early users not to share photorealistic generations that include faces and to flag problematic generations. We believe this has been effective in limiting potential harm, and we plan to continue the practice in the current phase.
Learning from real-world use is an important part of our commitment to develop and deploy AI responsibly, so we’re starting to widen access to users who joined our waitlist, slowly but steadily.
We intend to onboard up to 1,000 people every week as we iterate on our safety system and require all users to abide by our content policy. We hope to increase the rate at which we onboard new users as we learn more and gain confidence in our safety system. We’re inspired by what our users have created with DALL·E so far, and excited to see what new users will create.
OpenAI
OpenAI Leadership Team Update
Greg Brockman is becoming President, a new role which reflects his unique combination of personal coding contributions on our critical path together with company strategy. He is currently focused on training our flagship AI systems.
Brad Lightcap has been pivotal in OpenAI’s growth, scaling our structure, team, and capital base through his oversight of our Finance, Legal, People, and Operations organizations. He will become Chief Operating Officer and expand his focus, working with our Applied AI teams to sharpen our business and commercial strategies. He will also continue to manage the OpenAI Startup Fund.
Mira Murati has done a tremendous job leading our research, product, and partnership functions over the past 18 months. Most recently, she was instrumental in bringing these functions together for the successful release of our DALL·E research. Mira is taking on the role of Chief Technology Officer, reflecting her leadership across these critical areas within OpenAI.
Chris Clark is becoming Head of Nonprofit and Strategic Initiatives. He will lead the operations of OpenAI’s nonprofit parent and key strategic projects including our relationships with mission-aligned partners.
These executives are supported by world-class teams who are the lifeblood of OpenAI, constantly advancing the state of the art in artificial intelligence research and deployment. It’s a pleasure to work alongside such incredible talent and leadership across our company. We are all very excited for the future. (And we’re hiring!)
OpenAI
Measuring Goodhart’s Law
Goodhart’s law famously says: “When a measure becomes a target, it ceases to be a good measure.” Although originally from economics, it’s something we have to grapple with at OpenAI when figuring out how to optimize objectives that are difficult or costly to measure. It’s often necessary to introduce some proxy objective that’s easier or cheaper to measure, but when we do this, we need to be careful not to optimize it too much.
For example, as part of our work to align models like GPT-3 with human intent and values, we would like to optimize things like “How helpful is this response?”, or “How factually accurate is this claim?”. These are complex objectives that require humans to carefully check things over. For this reason, we train a model to predict these human preferences, known as a reward model, and use the reward model’s predictions as a proxy objective. But it’s important to keep track of how well the true objective is being optimized.
In this post we’ll look at some of the mathematics behind how we do this. We’ll focus on a setting that is particularly clean to analyze, in which we have access to the true objective. In practice, even human preferences can fail to measure what we really care about, but we’re setting that issue aside in this post.
Best-of-$n$ sampling
There are many ways in which one could optimize the proxy objective, but perhaps the simplest is best-of-$n$ sampling, also known as rejection sampling or reranking. We simply sample $n$ times and take the one that scores the highest according to the proxy objective.
Although this method is very simple, it can actually be competitive with more advanced techniques such as reinforcement learning, albeit at the cost of more inference-time compute. For example, in WebGPT, our best-of-$64$ model outperformed our reinforcement learning model, perhaps in part because the best-of-$64$ model got to browse many more websites. Even applying best-of-$4$ provided a significant boost to human preferences.
In addition, best-of-$n$ sampling has reliable performance and is straightforward to analyze mathematically, making it well-suited to empirical studies of Goodhart’s law and related phenomena.
The mathematics of best-of-$n$ sampling
Let’s study best-of-$n$ sampling more formally. Suppose we have some sample space $S$ (such as the set of possible question-answer pairs), some probability distribution $P$ over $S$, a true objective (or “reward”) $R_{text{true}}:Stomathbb R$, and a proxy objective $R_{text{proxy}}:Stomathbb R$. Let’s say that we somehow optimize $R_{text{proxy}}$ and thereby obtain some new distribution $P^prime$. Then:
- The expectation $mathbb E_{x^primesim P^prime}left[R_{text{true}}left(x^primeright)right]$ measures how well we have optimized the true objective.
- The KL divergence $D_{text{KL}}left(P^primeparallel Pright)$ measures how much optimization we have done. For example, if $P^prime$ is obtained by taking the first sample from $P$ that lies in some subset $S^primesubseteq S$, then this KL divergence is just the negative log probability that a sample from $P$ lies in $S^prime$.
It turns out that in the case of best-of-$n$ sampling, both of these quantities can be estimated efficiently using samples from $P$.
Let’s look at the expectation first. The naive approach is to use a Monte Carlo estimator: run best-of-$n$ sampling many times, measure the true objective on those samples, and average the results. However, there is a better estimator. If we have $Ngeq n$ samples from $P$ overall, then we can simultaneously consider every possible subset of these samples of size $n$, weight each sample by the number of subsets for which it is the best according to the proxy objective, and then take the weighted average true objective score. This weight is just the binomial coefficient $binom{k-1}{n-1}$, where $k$ is the rank of the sample under the proxy objective, from $1$ (worst) up to $N$ (best).[1] As well as using samples more efficiently, this also allows us to reuse samples for different values of $n$.
As for the KL divergence, surprisingly, this turns out to have an exact formula that works for any continuous probability distribution $P$ (i.e., as long as $P$ has no point masses). One might naively guess that the answer is $log n$, since best-of-$n$ is doing something like taking the top $frac 1n$ of the distribution, and this is roughly correct: the exact answer is $log n-frac{n-1}n$.[2]
Together, these estimators allow us to easily analyze how the true objective varies with the amount of optimization applied to the proxy objective.
Here’s a real-life example from WebGPT:
Best-of-$n$ performance for WebGPT 175B
Best-of-$n$ performance for WebGPT, with shaded regions representing $pm 1$ standard error, and the KL axis following a square root scale. Here, the original distribution ($P$) is given by the 175B model trained using behavior cloning, the proxy objective used to compute best-of-$n$ ($R_{text{proxy}}$) is given by the training reward model, and we consider three putatively “true” objectives ($R_{text{true}}$): the training reward model itself, a validation reward model trained on held-out data, and actual human preferences. There isn’t much over-optimization of the proxy objective, but we would expect there to be at higher KLs.
Going beyond best-of-$n$ sampling
The main limitation of best-of-$n$ sampling is that the KL divergence grows logarithmically with $n$, so it is only suitable for applying a small amount of optimization.
To apply more optimization, we typically use reinforcement learning. In the settings we’ve studied so far, such as summarization, we’ve typically been able to reach a KL of around 10 nats using reinforcement learning before the true objective starts to decrease due to Goodhart’s law. We’d have to take $n$ to be around 60,000 to reach this KL using best-of-$n$, and we hope to be able to reach much larger KLs than this with improvements to our reward modeling and reinforcement learning practices.
However, not all nats are equal. Empirically, for small KL budgets, best-of-$n$ better optimizes both the proxy and the true objectives than reinforcement learning. Intuitively, best-of-$n$ is the “brute force” approach, making it more information-theoretically efficient than reinforcement learning, but less computationally efficient at large KLs.[3]
We’re actively studying the scaling properties of proxy objectives as part of our work to align our models with human intent and values. If you’d like to help us with this research, we’re hiring!
OpenAI
Measuring Goodhart’s law
Goodhart’s law famously says: “When a measure becomes a target, it ceases to be a good measure.” Although originally from economics, it’s something we have to grapple with at OpenAI when figuring out how to optimize objectives that are difficult or costly to measure.OpenAI Blog