Large Language Models (LLMs) are trained on vast volumes of data and use billions of parameters to support tasks like answering questions, translating languages, and completing sentences. There are a few challenges when working with LLMs such as domain knowledge gaps, factuality issues, and hallucination, which affect their reliability especially for the fields that require high levels of accuracy, such as healthcare, law, or engineering. Retrieval Augmented Generation (RAG) provides a solution to mitigate some of these issues by augmenting LLMs with a specific domain or an organization’s internal knowledge base, without the need to retrain the model.
The RAG knowledge source is generally business specific databases which are typically deployed on general-purpose CPU infrastructure. So, deploying RAG on general-purpose CPU infrastructure alongside related business services is both efficient and cost-effective. With this motivation, we evaluated RAG deployment on AWS Graviton based Amazon EC2 instances which have been delivering up to 40% price-performance advantage compared to comparable instances for the majority of the workloads including databases, in-memory caches, big data analytics, media codecs, gaming servers, and machine learning inference.
In the past we published a few blog posts on how PyTorch was optimized for AWS Graviton processors to accelerate ML Inference performance for both eager mode (blog) and torch.compile
mode (blog). In this blog we cover how to deploy a typical RAG workload using PyTorch and torch.compile
, how we improved its performance up to 1.7x for embedding model and 1.3x for RAG query on AWS Graviton3-based m7g.xlarge instance compared to the default PyTorch “eager mode”, and finally a few recommendations that you can apply for your RAG use cases.
How to Optimize RAG?
Without RAG, the LLM takes the user input and creates a response based on information it was trained on (what it already knows). With RAG, an information retrieval component is introduced that utilizes the user input to first pull information from a new data source. The user query and the relevant information are both given to the LLM. The LLM uses the new knowledge and its training data to create better responses. The following diagram shows the conceptual flow of using RAG with LLMs.
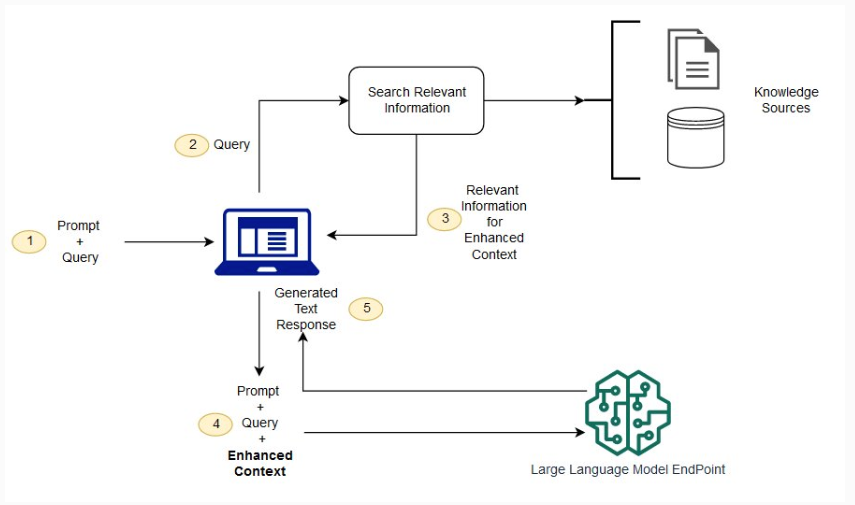
Image 1: Conceptual flow of using RAG with LLMs
Source: https://aws.amazon.com/what-is/retrieval-augmented-generation/
Embedding model
At the core of RAG is an embedding model that takes the text data and converts into a vector representation. These vectors are then stored in a vector db. When a user makes a query, the query is first converted to a vector and the RAG does a similarity search on the vector db. Hence, the first step in optimizing RAG performance is optimizing an embedding model’s inference performance. We used the AWS Graviton3-based m7g.xlarge instance and the HuggingFace sentence-transformer embedding model for the optimization work. Here is a sample script for profiling the HuggingFace sentence-transformer embedding model inference with PyTorch Eager mode.
import torch
from torch.profiler import profile, ProfilerActivity, record_function
from transformers import AutoModel, AutoTokenizer
model_name = "sentence-transformers/all-mpnet-base-v2"
input_text = ["This is an example sentence", "Each sentence is converted"]
model = AutoModel.from_pretrained(model_name)
tokenizer = AutoTokenizer.from_pretrained(model_name)
encoded_input = tokenizer(
input_text, padding=True, truncation=True, return_tensors="pt"
)
warmup, actual = 100, 100
model.eval()
with torch.no_grad():
# warmup
for i in range(warmup):
embeddings = model(**encoded_input)
with profile(activities=[ProfilerActivity.CPU]) as prof:
with record_function("model_inference"):
for i in range(actual):
embeddings = model(**encoded_input)
print(prof.key_averages().table(sort_by="self_cpu_time_total"))
Eager mode
Since PyTorch eager mode was already optimized on AWS Graviton processors with the following runtime environment settings, we included them in the baseline and measured the following performance. Please refer to Optimized PyTorch 2.0 Inference with AWS Graviton processors for more details on how we optimized the PyTorch eager mode on AWS Graviton processors.
# Enable the fast math GEMM kernels, to accelerate fp32 inference with bfloat16 gemm
export DNNL_DEFAULT_FPMATH_MODE=BF16
# Enable Linux Transparent Huge Page (THP) allocations,
# to reduce the tensor memory allocation latency
export THP_MEM_ALLOC_ENABLE=1
# Set LRU Cache capacity to cache the primitives and avoid redundant
# memory allocations
export LRU_CACHE_CAPACITY=1024
--------------------------- ------------ ------------ ------------ ------------ ------------ ------------
Name Self CPU % Self CPU CPU total % CPU total CPU time avg # of Calls
--------------------------- ------------ ------------ ------------ ------------ ------------ ------------
aten::addmm 61.01% 2.638s 62.49% 2.702s 370.197us 7300
model_inference 12.01% 519.161ms 100.00% 4.324s 4.324s 1
aten::bmm 6.25% 270.084ms 11.96% 517.089ms 215.454us 2400
aten::select 3.98% 172.165ms 5.34% 230.863ms 1.331us 173500
aten::copy_ 2.11% 91.133ms 2.11% 91.133ms 6.200us 14700
--------------------------- ------------ ------------ ------------ ------------ ------------ ------------
Self CPU time total: 4.324s
Table 1: Profiler output for HuggingFace sentence-transformer embedding model inference on AWS Graviton3-based m7g.xlarge instance with PyTorch Eager mode
Next, we added torch.compile
, weights pre-packing, and torch.inference_mode
and observed around 1.7x performance improvement. The following section talks about each of these optimizations and the resulting speedup.
torch.compile
In contrast to eager mode, the torch.compile
pre-compiles the entire model into a single graph in a manner that’s optimized for running on given hardware. Please refer to Accelerated PyTorch Inference with torch.compile on AWS Graviton processors for more details on torch.compile
features and how we optimized them on AWS Graviton processors. Invoke torch.compile
as shown in the following snippet to trigger PyTorch dynamo compilation for the model. This resulted in around 1.04x performance improvement from the baseline.
model = torch.compile(model)
---------------------------- ------------ ------------ ------------ ------------ ------------ ------------
Name Self CPU % Self CPU CPU total % CPU total CPU time avg # of Calls
---------------------------- ------------ ------------ ------------ ------------ ------------ ------------
aten::addmm 64.46% 2.675s 66.66% 2.766s 378.905us 7300
Torch-Compiled Region 19.76% 820.085ms 99.04% 4.109s 41.094ms 100
aten::bmm 6.66% 276.216ms 12.52% 519.527ms 216.470us 2400
aten::select 3.98% 164.991ms 5.41% 224.488ms 1.299us 172800
aten::as_strided 1.66% 69.039ms 1.66% 69.039ms 0.383us 180100
---------------------------- ------------ ------------ ------------ ------------ ------------ ------------
Self CPU time total: 4.149s
Table 2: Profiler output for HuggingFace sentence-transformer embedding model inference on AWS Graviton3-based m7g.xlarge instance with torch.compile mode
Weights pre-packing
torch.compile
opens up opportunities like pre-packing the model weights into a format that is more suitable for the given hardware during the model compilation, thus improving the performance. Set the following config to trigger weights pre-packing. This resulted in around 1.69x improvement from the baseline.
import torch._inductor.config as config
config.cpp.weight_prepack=True
config.freezing=True
----------------------------- ------------ ------------ ------------ ------------ ------------ ------------
Name Self CPU % Self CPU CPU total % CPU total CPU time avg # of Calls
----------------------------- ------------ ------------ ------------ ------------ ------------ ------------
mkldnn::_linear_pointwise 39.10% 994.821ms 41.50% 1.056s 144.628us 7300
Torch-Compiled Region 35.12% 893.675ms 98.42% 2.504s 25.043ms 100
aten::bmm 10.96% 278.859ms 21.66% 551.073ms 229.614us 2400
aten::select 7.34% 186.838ms 9.98% 253.840ms 1.469us 172800
aten::as_strided 2.63% 67.002ms 2.63% 67.002ms 0.388us 172800
----------------------------- ------------ ------------ ------------ ------------ ------------ ------------
Self CPU time total: 2.544s
Table 3: Profiler output for HuggingFace sentence-transformer embedding model inference on AWS Graviton3-based m7g.xlarge instance with torch.compile and weights pre-packing
torch.inference_mode
Additionally, use torch.inference_mode()
to get savings from turning off version control for tensors and view tracking of tensors. Please refer to the PyTorch documentation for more details.
with torch.inference_mode():
# instead of
with torch.no_grad():
----------------------------- ------------ ------------ ------------ ------------ ------------ ------------
Name Self CPU % Self CPU CPU total % CPU total CPU time avg # of Calls
----------------------------- ------------ ------------ ------------ ------------ ------------ ------------
mkldnn::_linear_pointwise 38.92% 987.276ms 41.17% 1.044s 143.056us 7300
Torch-Compiled Region 34.92% 885.895ms 98.45% 2.498s 24.975ms 100
aten::bmm 11.25% 285.292ms 22.22% 563.594ms 234.831us 2400
aten::select 7.74% 196.223ms 10.22% 259.251ms 1.500us 172800
aten::as_strided 2.48% 63.027ms 2.48% 63.027ms 0.365us 172800
----------------------------- ------------ ------------ ------------ ------------ ------------ ------------
Self CPU time total: 2.537s
Table 4: Profiler output for HuggingFace sentence-transformer embedding model inference on AWS Graviton3-based m7g.xlarge instance with torch.compile, weights pre-packing, and inference_mode
The following table shows the incremental performance improvements achieved for the standalone embedding model inference.
Optimization level
|
Latency measured (in sec)
|
Improvement over the baseline
|
PyTorch eager mode (Baseline)
|
0.04324
|
NA
|
torch.compile
|
0.04149
|
1.04x
|
weights pre-packing
|
0.02544
|
1.69x
|
torch.inference_mode
|
0.02537
|
1.70x
|
The following script is an updated example for the embedding model inference with the previously discussed optimizations included. The optimizations are highlighted in GREEN.
import torch
from torch.profiler import profile, record_function, ProfilerActivity
from transformers import AutoTokenizer, AutoModel
import torch._inductor.config as config
config.cpp.weight_prepack=True
config.freezing=True
model_name = "sentence-transformers/all-mpnet-base-v2"
input_text = ['This is an example sentence', 'Each sentence is converted']
model = AutoModel.from_pretrained(model_name)
tokenizer = AutoTokenizer.from_pretrained(model_name)
encoded_input = tokenizer(input_text, padding=True, truncation=True, return_tensors='pt')
warmup , actual = 100, 100
model.eval()
model = torch.compile(model)
with torch.inference_mode():
#instead of with torch.no_grad()
# warmup
for i in range(warmup):
embeddings = model(**encoded_input)
with profile(activities=[ProfilerActivity.CPU]) as prof:
with record_function("model_inference"):
for i in range(actual):
embeddings = model(**encoded_input)
print(prof.key_averages().table(sort_by="self_cpu_time_total"))
End-to-End RAG scenario on CPU
After optimizing the embedding model inference, we started with a PyTorch eager mode based RAG setup, mainly to validate the functionality on the CPU backend. We built the RAG solution with HuggingFaceEmbeddings from langchain_community.embeddings
, as shown in the following code snippet.
from langchain_community.embeddings import HuggingFaceEmbeddings
from langchain_community.vectorstores import FAISS
from langchain.text_splitter import RecursiveCharacterTextSplitter
from langchain_community.document_loaders.recursive_url_loader import RecursiveUrlLoader
from langchain.prompts import PromptTemplate
from langchain_core.prompts import format_document
from bs4 import BeautifulSoup as Soup
import torch
url = "https://pytorch.org/blog/pytorch2-5/"
chunk_size = 1000
chunk_overlap = 0
embedding_model = "sentence-transformers/all-mpnet-base-v2"
N = 5
question = "What's new in PyTorch 2.5?"
from transformers import AutoTokenizer, AutoModel
from typing import Any, List
loader = RecursiveUrlLoader(
url=url, max_depth=3, extractor=lambda x: Soup(x, "html.parser").text
)
docs = loader.load()
# Split the document into chunks with a specified chunk size
text_splitter = RecursiveCharacterTextSplitter(chunk_size=chunk_size, chunk_overlap=chunk_overlap)
all_splits = text_splitter.split_documents(docs)
# Store the document into a vector store with a specific embedding model
model = HuggingFaceEmbeddings(model_name=embedding_model)
warmup , actual = 100, 100
with torch.inference_mode():
vectorstore = FAISS.from_documents(all_splits, model)
for i in range(warmup):
searchDocs = vectorstore.similarity_search(question, k=N)
import time
start = time.time()
for i in range(actual):
searchDocs = vectorstore.similarity_search(question, k=N)
end = time.time()
print(f"Time for 1 inference is {(end-start)/actual} seconds")
doc_prompt = PromptTemplate.from_template("{page_content}")
context = ""
for i, doc in enumerate(searchDocs):
context += f"n{format_document(doc, doc_prompt)}n"
Next, our goal was to optimize the end-to-end RAG use case with torch.compile and weights pre-packing that gave 1.7x improvement for the standalone embedding model inference. However, the optimizations didn’t work out of the box for the RAG scenario.
What are the challenges and solutions to achieve similar gains in an end-to-end RAG scenario?
Challenge 1: model handle
There was no way to get the model handle that was instantiated with HuggingFaceEmbeddings
, and the wrapper class doesn’t provide compile APIs. So, there was no way for our application to invoke torch.compile
to trigger the PyTorch dynamo compilation process.
Solution
We implemented our custom embedding class so that we can get a handle for the model. This instantiated the embedding model from sentence-transformers
, and maintained the handle for immediate compilation or compilation at a later stage. With this, we were able to trigger torch.compile
and hence the dynamo compilation.
class CustomEmbedding(HuggingFaceEmbeddings):
def __init__(self, **kwargs: Any):
"""Initialize the sentence_transformer."""
super().__init__(**kwargs)
# Load model from HuggingFace Hub
self.client = AutoModel.from_pretrained(self.model_name)
class Config:
arbitrary_types_allowed = True
def embed_documents(self, texts: List[str]) -> List[List[float]]:
"""Compute doc embeddings using a HuggingFace transformer model.
Args:
texts: The list of texts to embed.
Returns:
List of embeddings, one for each text.
"""
texts = list(map(lambda x: x.replace("n", " "), texts))
# Tokenize sentences
tokenizer = AutoTokenizer.from_pretrained(self.model_name)
encoded_input = tokenizer(texts, padding=True, truncation=True, return_tensors='pt')
embeddings = self.client(
**encoded_input, output_hidden_states=True
)
embeddings = embeddings.pooler_output.detach().numpy()
return embeddings.tolist()
# instead of model = HuggingFaceEmbeddings(model_name=embedding_model)
model = CustomEmbedding(model_name=embedding_model)
# torch.compile the model
model.client = torch.compile(model.client)
Challenge 2: triggering the optimization
For a typical inference scenario where the graph is frozen and gradient calculations are disabled, Torch inductor (the compiler backend we used for CPUs) invokes hardware specific optimizations like graph rewrite into more performant operators, operator fusion, and weights pre-packing. Though Torch dynamo was able to see the model and trigger generic compilation, it failed to trigger these additional Fx passes in the Torch inductor.
There were two main reasons for Torch inductor not triggering the optimization passes: (1) The application didn’t set no_grad()
or inference_mode()
for torch inductor to detect that the graph was frozen; and (2) We hit a limitation with the torch.compile framework, where, if the no_grad
is set just at the beginning of the compiled region, torch.compile
wouldn’t be able to detect it while invoking the inductor Fx
passes because it would not have hit the no_grad
region by then. Please refer to this GitHub issue for more details.
Solution
We work around this limitation by moving the no_grad()
context into the application code from within the model class. With this, the model compilation happened as expected and gave around 1.3x performance improvement when we profiled the stable inference pass for eager and compiled versions.
Challenge 3: extra compilation
With the previous fixes, the query lookup inference performance was improved, but not the total execution time of the benchmarking script. We root-caused it to redundant compilation for the model during the RAG inference. Further deep diving revealed that it was because of the batch size mismatch between the word embedding and the RAG query stages. For example, in our benchmarking script, when the database was vectorized and stored in vector db, we used the batch size of 16, hence the model was compiled with shapes of 16xNxK. Whereas, the RAG query lookup is usually a single request of shape 1xNxK. So, there was a batch size mismatch (dimension “0” of these tensors) that triggered the recompilation for the query lookup stage. We confirmed it with the following Torch logging: TORCH_LOGS="recompiles"
TORCH_LOGS="recompiles" python rag_compile.py
V1103 02:48:08.805986 34281 site-packages/torch/_dynamo/guards.py:2813] [0/1] [__recompiles] Recompiling function forward in site-packages/transformers/models/mpnet/modeling_mpnet.py:502
V1103 02:48:08.805986 34281 site-packages/torch/_dynamo/guards.py:2813] [0/1] [__recompiles] triggered by the following guard failure(s):
V1103 02:48:08.805986 34281 site-packages/torch/_dynamo/guards.py:2813] [0/1] [__recompiles] - 0/0: tensor 'L['input_ids']' size mismatch at index 0. expected 16, actual 1
Solution
Torch dynamo provides a decorator to mark the dimension of a given tensor as dynamic and specify an expected value for the same, so that re-compilation is not triggered. For example, specifying dimension “0” of input_ids
and attention_mask
as dynamic, and specifying that value of “1” is allowed in that dimension (as shown in the following code snippet), should have avoided the redundant compilations.
torch._dynamo.decorators.mark_unbacked(encoded_input['input_ids'], 0)
torch._dynamo.mark_dynamic(encoded_input['input_ids'], 1)
torch._dynamo.decorators.mark_unbacked(encoded_input['attention_mask'], 0)
torch._dynamo.mark_dynamic(encoded_input['attention_mask'], 1)
However, the Torch dynamo decorator and marking didn’t work in this particular case. Moreover, using the decorator created graph breaks. So, we added some warmup iterations to hide the compilation latency, and profiled the query lookup performance in the steady state. However, the good news is that, in practice, this re-compilation is triggered only for the first query, so it might not affect the production scenario if the database size is fixed. Moreover, PyTorch AOT Inductor (a new feature in PyTorch) addresses re-compilation and warm up challenges with torch.compile. In a follow-up blog we will address how in a production environment we can use AOT Inductor to address these challenges.
With these solutions we were able to apply torch.compile, weights pre-packing and the AWS Graviton specific optimizations for an end-end RAG scenario and improve the performance by 1.3x from the baseline eager mode.
Deployment
A detailed guide on how to deploy torch compiled RAG on AWS Graviton-based Amazon EC2 instances and how to deploy it in conjunction with Llama using TorchServe can be found on the PyTorch website.
Conclusion
In this blog, we covered how we optimized embedding model inference performance on AWS Graviton3-based EC2 instances. We also shared the challenges faced, the solutions we implemented to bring those optimizations for a RAG use case, and the resulting speedups. We hope that you will give it a try! If you need any support with ML software on Graviton, please open an issue on the AWS Graviton Technical Guide GitHub.
We would like to express our gratitude to Eli Uriegas for the support in making this blog post happen.
Authors
Sunita Nadampalli is a Principal Engineer and AI/ML expert at AWS. She leads AWS Graviton software performance optimizations for AI/ML and HPC workloads. She is passionate about open source software development and delivering high-performance and sustainable software solutions for SoCs based on the Arm ISA.
Ankith Gunapal is an AI Partner Engineer at Meta (PyTorch). He leads customer support, evangelizing & release engineering of TorchServe. He is passionate about solving production problems in model inference and model serving. He also enjoys distilling technically complex material in a user friendly format.
Hamid Shojanazeri leads the AI Frameworks Partner Engineering team at Meta. He is passionate about building scalable AI solutions and specializes in working with PyTorch to tackle the challenges of large-scale distributed training, inference, model serving, and optimization.
Read More