A guest post by Hugging Face: Pierric Cistac, Software Engineer; Victor Sanh, Scientist; Anthony Moi, Technical Lead.
Hugging Face 🤗 is an AI startup with the goal of contributing to Natural Language Processing (NLP) by developing tools to improve collaboration in the community, and by being an active part of research efforts.
Because NLP is a difficult field, we believe that solving it is only possible if all actors share their research and results. That’s why we created 🤗 Transformers, a leading NLP library with more than 2M downloads and used by researchers and engineers across many companies. It allows the amazing international NLP community to quickly experiment, iterate, create and publish new models for a variety of tasks (text/token generation, text classification, question answering…) in a variety of languages (English of course, but also French, Italian, Spanish, German, Turkish, Swedish, Dutch, Arabic and many others!) More than 300 different models are available today through Transformers.
While Transformers is very handy for research, we are also working hard on the production aspects of NLP, looking at and implementing solutions that can ease its adoption everywhere. In this blog post, we’re going to showcase one of the paths we believe can help fulfill this goal: the use of “small”, yet performant models (such as DistilBERT), and frameworks targeting ecosystems different from Python such as Node via TensorFlow.js.
The need for small models: DistilBERT
One of the areas we’re interested in is “low-resource” model with close to state-of-the-art results, while being a lot smaller and also a lot faster to run. That’s why we created DistilBERT, a distilled version of BERT: it has 40% fewer parameters, runs 60% faster while preserving 97% of BERT’s performance as measured on the GLUE language understanding benchmark.
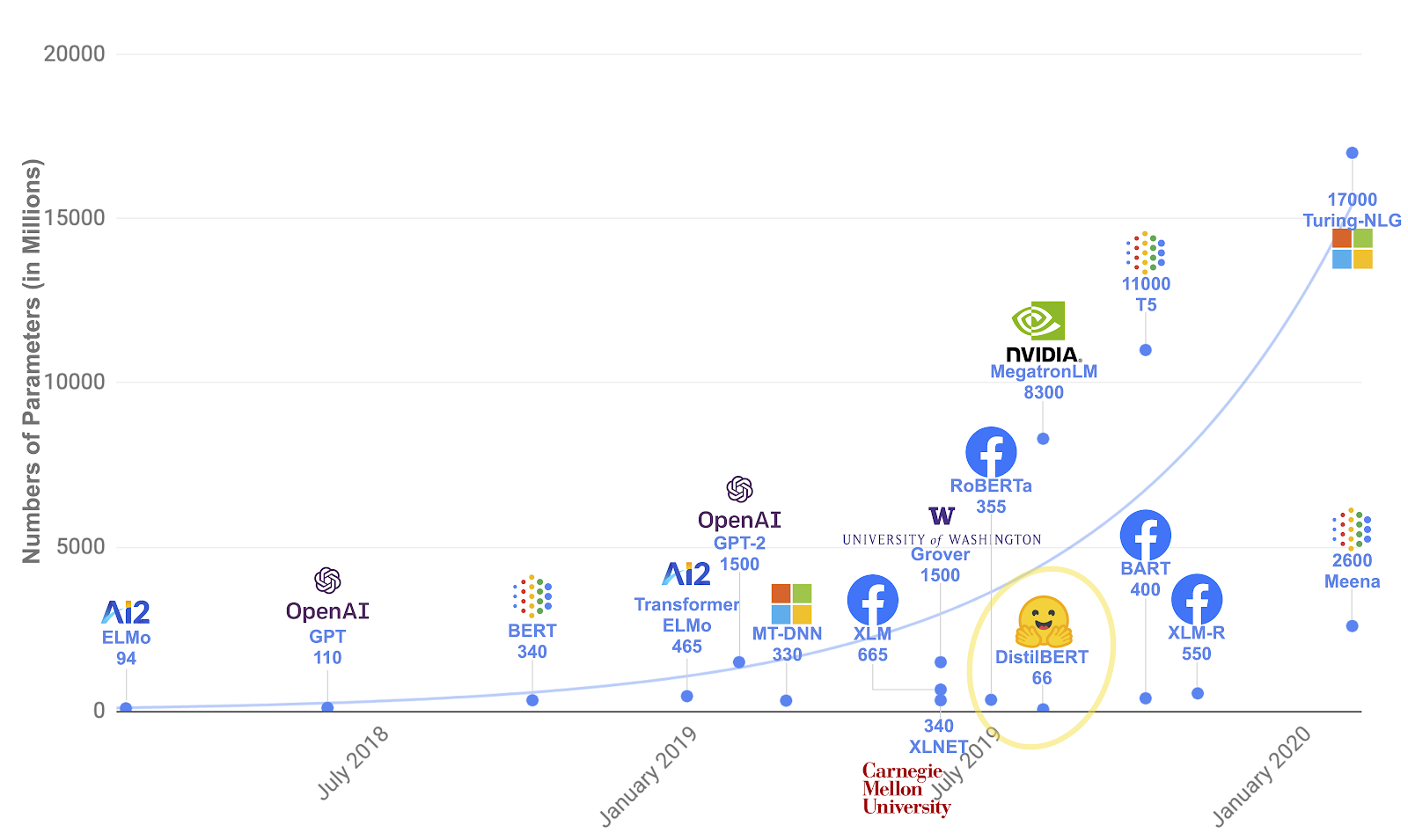 |
NLP models through time, with their number of parameters |
To create DistilBERT, we’ve been applying knowledge distillation to BERT (hence its name), a compression technique in which a small model is trained to reproduce the behavior of a larger model (or an ensemble of models), demonstrated by Hinton et al.
In the teacher-student training, we train a student network to mimic the full output distribution of the teacher network (its knowledge). Rather than training with a cross-entropy over the hard targets (one-hot encoding of the gold class), we transfer the knowledge from the teacher to the student with a cross-entropy over the soft targets (probabilities of the teacher). Our training loss thus becomes:
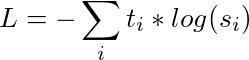 |
With t the logits from the teacher and s the logits of the student |
Our student is a small version of BERT in which we removed the token-type embeddings and the pooler (used for the next sentence classification task). We kept the rest of the architecture identical while reducing the numbers of layers by taking one layer out of two, leveraging the common hidden size between student and teacher. We trained DistilBERT on very large batches leveraging gradient accumulation (up to 4000 examples per batch), with dynamic masking, and removed the next sentence prediction objective.
With this, we were then able to fine-tune our model on the specific task of Question Answering. To do so, we used the BERT-cased model fine-tuned on SQuAD 1.1 as a teacher with a knowledge distillation loss. In other words, we distilled a question answering model into a language model previously pre-trained with knowledge distillation! That’s a lot of teachers and students: DistilBERT-cased was first taught by BERT-cased, and then “taught again” by the SQuAD-finetuned BERT-cased version in order to get the DistilBERT-cased-finetuned-squad model.
This results in very interesting performances given the size of the network: our DistilBERT-cased fine-tuned model reaches an F1 score of 87.1 on the dev set, less than 2 points behind the full BERT-cased fine-tuned model! (88.7 F1 score).
If you’re interested in learning more about the distillation process, you can read our dedicated blog post.
The need for a language-neutral format: SavedModel
Using the previous process, we end up with a 240MB Keras file (.h5) containing the weights of our DistilBERT-cased-squad model. In this format, the architecture of the model resides in an associated Python class. But our final goal is to be able to use this model in as many environments as possible (Node.js + TensorFlow.js for this blog post), and the TensorFlow SavedModel format is perfect for this: it’s a “serialized” format, meaning that all the information necessary to run the model is contained into the model files. It is also a language-neutral format, so we can use it in Python, but also in JS, C++, and Go.
To convert to SavedModel, we first need to construct a graph from the model code. In Python, we can use tf.function
to do so:
import tensorflow as tf
from transformers import TFDistilBertForQuestionAnswering
distilbert = TFDistilBertForQuestionAnswering.from_pretrained('distilbert-base-cased-distilled-squad')
callable = tf.function(distilbert.call)
Here we passed to tf.function
the function called in our Keras model, call
. What we get in return is a callable that we can in turn use to trace our call function with a specific signature and shapes thanks to get_concrete_function
:
concrete_function = callable.get_concrete_function([tf.TensorSpec([None, 384], tf.int32, name="input_ids"), tf.TensorSpec([None, 384], tf.int32, name="attention_mask")])
By calling get_concrete_function
, we trace-compile the TensorFlow operations of the model for an input signature composed of two Tensors of shape [None, 384]
, the first one being the input ids and the second one the attention mask.
Then we can finally save our model to the SavedModel format:
tf.saved_model.save(distilbert, 'distilbert_cased_savedmodel', signatures=concrete_function)
A conversion in 4 lines of code, thanks to TensorFlow! We can check that our resulting SavedModel contains the correct signature by using the
saved_model_cli:
$ saved_model_cli show --dir distilbert_cased_savedmodel --tag_set serve --signature_def serving_default
Output:
The given SavedModel SignatureDef contains the following input(s):
inputs['attention_mask'] tensor_info:
dtype: DT_INT32
shape: (-1, 384)
name: serving_default_attention_mask:0
inputs['input_ids'] tensor_info:
dtype: DT_INT32
shape: (-1, 384)
name: serving_default_input_ids:0
The given SavedModel SignatureDef contains the following output(s):
outputs['output_0'] tensor_info:
dtype: DT_FLOAT
shape: (-1, 384)
name: StatefulPartitionedCall:0
outputs['output_1'] tensor_info:
dtype: DT_FLOAT
shape: (-1, 384)
name: StatefulPartitionedCall:1
Method name is: tensorflow/serving/predict
Perfect! You can play with the conversion code yourself by opening this colab notebook. We are now ready to use our SavedModel with TensorFlow.js!
The need for ML in Node.js: TensorFlow.js
Here at Hugging Face we strongly believe that in order to reach its full adoption potential, NLP has to be accessible in other languages that are more widely used in production than Python, with APIs simple enough to be manipulated with software engineers without a Ph.D. in Machine Learning; one of those languages is obviously Javascript.
Thanks to the API provided by TensorFlow.js, interacting with the SavedModel we created previously in Node.js is very straightforward. Here is a slightly simplified version of the Typescript code in our NPM Question Answering package:
const model = await tf.node.loadSavedModel(path); // Load the model located in path
const result = tf.tidy(() => {
// ids and attentionMask are of type number[][]
const inputTensor = tf.tensor(ids, undefined, "int32");
const maskTensor = tf.tensor(attentionMask, undefined, "int32");
// Run model inference
return model.predict({
// “input_ids” and “attention_mask” correspond to the names specified in the signature passed to get_concrete_function during the model conversion
“input_ids”: inputTensor, “attention_mask”: maskTensor
}) as tf.NamedTensorMap;
});
// Extract the start and end logits from the tensors returned by model.predict
const [startLogits, endLogits] = await Promise.all([
result[“output_0"].squeeze().array() as Promise,
result[“output_1”].squeeze().array() as Promise
]);
tf.dispose(result); // Clean up memory used by the result tensor since we don’t need it anymore
Note the use of the very helpful TensorFlow.js function tf.tidy
, which takes care of automatically cleaning up intermediate tensors like inputTensor
and maskTensor
while returning the result of the model inference.
How do we know we need to use "ouput_0"
and "output_1"
to extract the start and end logits (beginning and end of the possible spans answering the question) from the result returned by the model? We just have to look at the output names indicated by the saved_model_cli
command we ran previously after exporting to SavedModel.
The need for fast and easy to use tokenizer: 🤗 Tokenizers
Our goal while building our Node.js library was to make the API as simple as possible. As we just saw, running model inference once we have our SavedModel is quite simple, thanks to TensorFlow.js. Now, the most difficult part is passing the data in the right format to the input ids and attention mask tensors. What we collect from a user is usually a string, but the tensors require arrays of numbers: we need to tokenize the user input.
Enter 🤗 Tokenizers: a performant library written in Rust that we’ve been working on at Hugging Face. It allows you to play with different tokenizers such as BertWordpiece very easily, and it works in Node.js too thanks to the provided bindings:
const tokenizer = await BertWordPieceTokenizer.fromOptions({
vocabFile: vocabPath, lowercase: false
});
tokenizer.setPadding({ maxLength: 384 }); // 384 matches the shape of the signature input provided while exporting to SavedModel
// Here question and context are in their original string format
const encoding = await tokenizer.encode(question, context);
const { ids, attentionMask } = encoding;
That’s it! In just 4 lines of code, we are able to convert the user input to a format we can then use to feed our model with TensorFlow.js.
The Final Result: Powerful Question Answering in Node.js
Thanks to the powers of the SavedModel format, TensorFlow.js for inference, and Tokenizers for tokenization, we’ve reached our goal to offer a very simple, yet very powerful, public API in our NPM package:
import { QAClient } from "question-answering"; // If using Typescript or Babel
// const { QAClient } = require("question-answering"); // If using vanilla JS
const text = `
Super Bowl 50 was an American football game to determine the champion of the National Football League (NFL) for the 2015 season.
The American Football Conference (AFC) champion Denver Broncos defeated the National Football Conference (NFC) champion Carolina Panthers 24–10 to earn their third Super Bowl title. The game was played on February 7, 2016, at Levi's Stadium in the San Francisco Bay Area at Santa Clara, California.
As this was the 50th Super Bowl, the league emphasized the "golden anniversary" with various gold-themed initiatives, as well as temporarily suspending the tradition of naming each Super Bowl game with Roman numerals (under which the game would have been known as "Super Bowl L"), so that the logo could prominently feature the Arabic numerals 50.
`;
const question = "Who won the Super Bowl?";
const qaClient = await QAClient.fromOptions();
const answer = await qaClient.predict(question, text);
console.log(answer); // { text: 'Denver Broncos', score: 0.3 }
Powerful? Yes! Thanks to the native support of SavedModel format in TensorFlow.js, we get very good performances: here is a benchmark comparing our Node.js package and our popular transformers Python library, running the same DistilBERT-cased-squad model. As you can see, we achieve a 2X speed gain! Who said Javascript was slow?
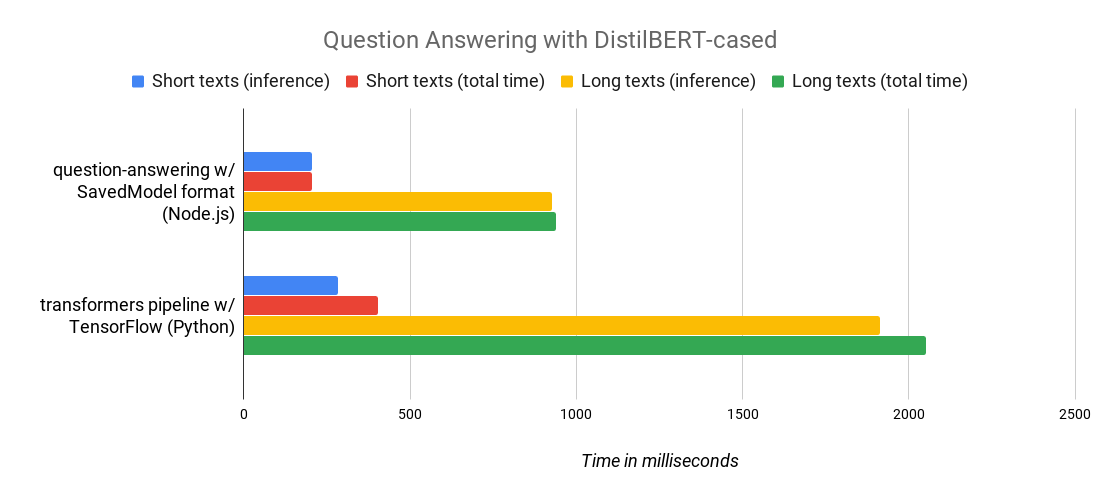 |
Short texts are texts between 500 and 1000 characters, long texts are between 4000 and 5000 characters. You can check the Node.js benchmark script here (the Python one is equivalent). Benchmark run on a standard 2019 MacBook Pro running on macOS 10.15.2. |
It’s a very interesting time for NLP: big models such as GPT2 or T5 keep getting better and better, and research on how to “minify” those good but heavy and costly models is also getting more and more traction, with distillation being one technique among others. Adding to the equation tools that allow big developer communities to be part of the revolution (such as TensorFlow.js with the Javascript ecosystem), only makes the future of NLP more exciting and more production-ready than ever!
For further reading, feel free to check our Github repositories:
https://github.com/huggingfaceRead More