Inference, the work of using AI in applications, is moving into mainstream uses, and it’s running faster than ever.
NVIDIA GPUs won all tests of AI inference in data center and edge computing systems in the latest round of the industry’s only consortium-based and peer-reviewed benchmarks.
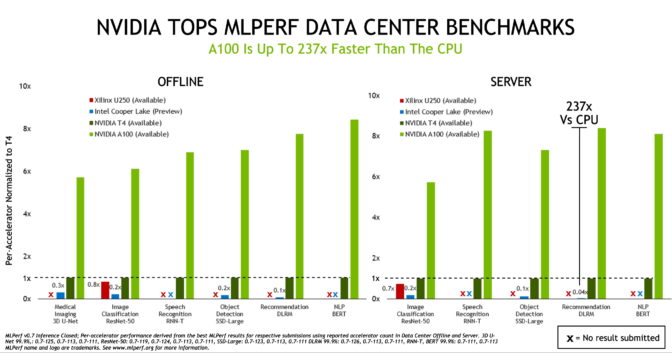
NVIDIA A100 Tensor Core GPUs extended the performance leadership we demonstrated in the first AI inference tests held last year by MLPerf, an industry benchmarking consortium formed in May 2018.
The A100, introduced in May, outperformed CPUs by up to 237x in data center inference, according to the MLPerf Inference 0.7 benchmarks. NVIDIA T4 small form factor, energy-efficient GPUs beat CPUs by up to 28x in the same tests.
To put this into perspective, a single NVIDIA DGX A100 system with eight A100 GPUs now provides the same performance as nearly 1,000 dual-socket CPU servers on some AI applications.
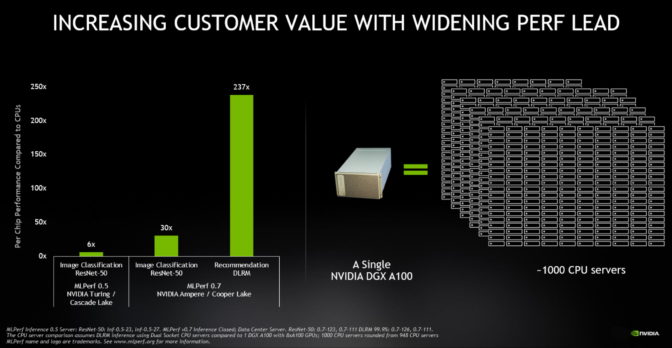
This round of benchmarks also saw increased participation, with 23 organizations submitting — up from 12 in the last round — and with NVIDIA partners using the NVIDIA AI platform to power more than 85 percent of the total submissions.
A100 GPUs, Jetson AGX Xavier Take Performance to the Edge
While A100 is taking AI inference performance to new heights, the benchmarks show that T4 remains a solid inference platform for mainstream enterprise, edge servers and cost-effective cloud instances. In addition, the NVIDIA Jetson AGX Xavier builds on its leadership position in power constrained SoC-based edge devices by supporting all new use cases.
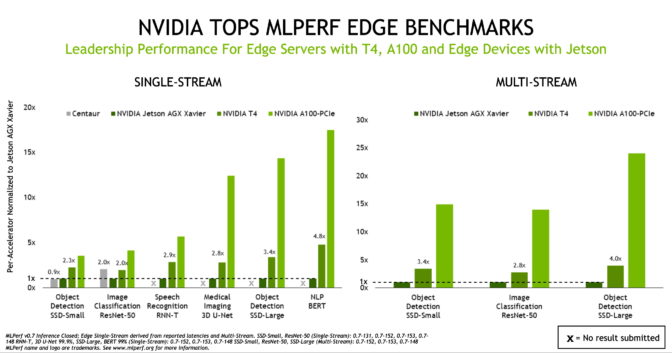
The results also point to our vibrant, growing AI ecosystem, which submitted 1,029 results using NVIDIA solutions representing 85 percent of the total submissions in the data center and edge categories. The submissions demonstrated solid performance across systems from partners including Altos, Atos, Cisco, Dell EMC, Dividiti, Fujitsu, Gigabyte, Inspur, Lenovo, Nettrix and QCT.
Expanding Use Cases Bring AI to Daily Life
Backed by broad support from industry and academia, MLPerf benchmarks continue to evolve to represent industry use cases. Organizations that support MLPerf include Arm, Baidu, Facebook, Google, Harvard, Intel, Lenovo, Microsoft, Stanford, the University of Toronto and NVIDIA.
The latest benchmarks introduced four new tests, underscoring the expanding landscape for AI. The suite now scores performance in natural language processing, medical imaging, recommendation systems and speech recognition as well as AI use cases in computer vision.
You need go no further than a search engine to see the impact of natural language processing on daily life.
“The recent AI breakthroughs in natural language understanding are making a growing number of AI services like Bing more natural to interact with, delivering accurate and useful results, answers and recommendations in less than a second,” said Rangan Majumder, vice president of search and artificial intelligence at Microsoft.
“Industry-standard MLPerf benchmarks provide relevant performance data on widely used AI networks and help make informed AI platform buying decisions,” he said.
AI Helps Saves Lives in the Pandemic
The impact of AI in medical imaging is even more dramatic. For example, startup Caption Health uses AI to ease the job of taking echocardiograms, a capability that helped save lives in U.S. hospitals in the early days of the COVID-19 pandemic.
That’s why thought leaders in healthcare AI view models like 3D U-Net, used in the latest MLPerf benchmarks, as key enablers.
“We’ve worked closely with NVIDIA to bring innovations like 3D U-Net to the healthcare market,” said Klaus Maier-Hein, head of medical image computing at DKFZ, the German Cancer Research Center.
“Computer vision and imaging are at the core of AI research, driving scientific discovery and representing core components of medical care. And industry-standard MLPerf benchmarks provide relevant performance data that helps IT organizations and developers accelerate their specific projects and applications,” he added.
Commercially, AI use cases like recommendation systems, also part of the latest MLPerf tests, are already making a big impact. Alibaba used recommendation systems last November to transact $38 billion in online sales on Singles Day, its biggest shopping day of the year.
Adoption of NVIDIA AI Inference Passes Tipping Point
AI inference passed a major milestone this year.
NVIDIA GPUs delivered a total of more than 100 exaflops of AI inference performance in the public cloud over the last 12 months, overtaking inference on cloud CPUs for the first time. Total cloud AI Inference compute capacity on NVIDIA GPUs has been growing roughly tenfold every two years.
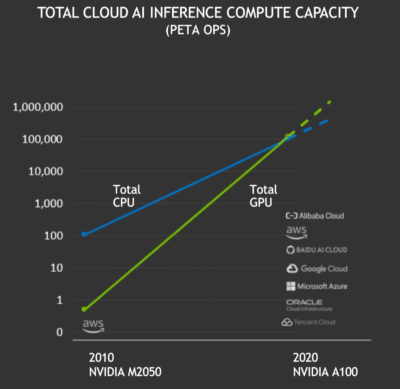
With the high performance, usability and availability of NVIDIA GPU computing, a growing set of companies across industries such as automotive, cloud, robotics, healthcare, retail, financial services and manufacturing now rely on NVIDIA GPUs for AI inference. They include American Express, BMW, Capital One, Dominos, Ford, GE Healthcare, Kroger, Microsoft, Samsung and Toyota.
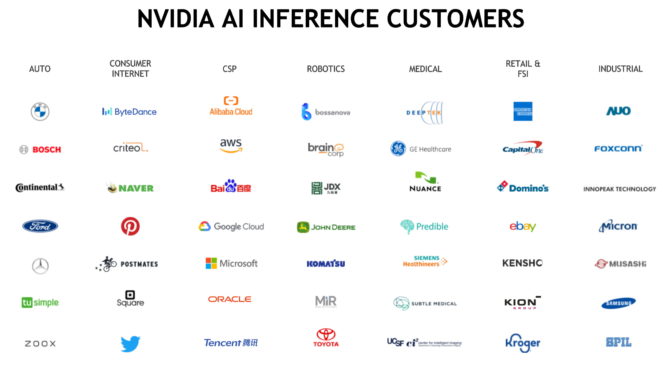
Why AI Inference Is Hard
Use cases for AI are clearly expanding, but AI inference is hard for many reasons.
New kinds of neural networks like generative adversarial networks are constantly being spawned for new use cases and the models are growing exponentially. The best language models for AI now encompass billions of parameters, and research in the field is still young.
These models need to run in the cloud, in enterprise data centers and at the edge of the network. That means the systems that run them must be highly programmable, executing with excellence across many dimensions.
NVIDIA founder and CEO Jensen Huang compressed the complexities in one word: PLASTER. Modern AI inference requires excellence in Programmability, Latency, Accuracy, Size of model, Throughput, Energy efficiency and Rate of learning.
To power excellence across every dimension, we’re focussed on constantly evolving our end-to-end AI platform to handle demanding inference jobs.
AI Requires Performance, Usability
An accelerator like the A100, with its third-generation Tensor Cores and the flexibility of its multi-instance GPU architecture, is just the beginning. Delivering leadership results requires a full software stack.
NVIDIA’s AI software begins with a variety of pretrained models ready to run AI inference. Our Transfer Learning Toolkit lets users optimize these models for their particular use cases and datasets.
NVIDIA TensorRT optimizes trained models for inference. With 2,000 optimizations, it’s been downloaded 1.3 million times by 16,000 organizations.
The NVIDIA Triton Inference Server provides a tuned environment to run these AI models supporting multiple GPUs and frameworks. Applications just send the query and the constraints — like the response time they need or throughput to scale to thousands of users — and Triton takes care of the rest.
These elements run on top of CUDA-X AI, a mature set of software libraries based on our popular accelerated computing platform.
Getting a Jump-Start with Applications Frameworks
Finally, our application frameworks jump-start adoption of enterprise AI across different industries and use cases.
Our frameworks include NVIDIA Merlin for recommendation systems, NVIDIA Jarvis for conversational AI, NVIDIA Maxine for video conferencing, NVIDIA Clara for healthcare, and many others available today.
These frameworks, along with our optimizations for the latest MLPerf benchmarks, are available in NGC, our hub for GPU-accelerated software that runs on all NVIDIA-certified OEM systems and cloud services.
In this way, the hard work we’ve done benefits the entire community.
The post NVIDIA Inference Performance Surges as AI Use Crosses Tipping Point appeared first on The Official NVIDIA Blog.